NCBI Bookshelf. A service of the National Library of Medicine, National Institutes of Health.
Dalal SR, Shekelle PG, Hempel S, et al. A Pilot Study Using Machine Learning and Domain Knowledge To Facilitate Comparative Effectiveness Review Updating [Internet]. Rockville (MD): Agency for Healthcare Research and Quality (US); 2012 Sep.
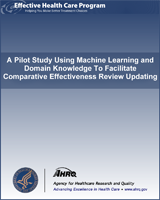
A Pilot Study Using Machine Learning and Domain Knowledge To Facilitate Comparative Effectiveness Review Updating [Internet].
Show detailsLiterature Characteristics
Table 1 shows the characteristics of the original and updated AAP literature searches; each column (original and update) represents both excluded and relevant studies. We compared the proportions of each variable within the original and update search results using Fisher’s exact test. Substantial and statistically significant differences were observed between the means of variables in the AAP original and updated searches. This finding suggests that the composition of the search results (if not necessarily the included studies) differed substantially between the update and original searches.
Table 1
AAP characteristics: original versus update.
Table 2 shows the characteristics for the AAP original search by category (excluded, included for AE analysis, included only for the efficacy/effectiveness analyses, included for both analyses). There are obviously substantial differences, as revealed by the one-way Anova test comparing means in all four groups; these differences were highly significant for most key variables including “RCT.” The importance of each variable is unknown, but the differences suggest that combinations of variables could be useful in distinguishing between included and excluded studies.
Table 2
Characteristics of the original AAP review (by category of article).
Table 3 shows select characteristics of the LBD literature; we show the same characteristics as in the AAP update (Tables 1 and 2) to demonstrate how characteristics may vary between different review topics. The original search results were published from 1966 to 2009 (articles published after 2006 were electronically published in 2006). The updated search results were predominantly published from 2007 to 2010, with some articles published from 1997 to 2006 and in 2011. Roughly 10 percent of the retrieved studies were classified as RCTs in MEDLINE in both the original and updated literature searches. As noted in the third column of Table 3, the presence of several key variables differed substantially between the original and updated searches in univariate comparisons. In particular, the update included non-human studies and proportionally fewer articles in which the outcome was associated with drug therapy. This finding suggests that the original and updated data were somewhat different, which made creation of a generalizable model more difficult.
Table 3
Characteristics of LBD search results (original vs. updated).
Table 4 shows the original literature search results for LBD in greater detail, and compares characteristics among four categories (excluded studies, considered only for efficacy/effectiveness analyses, considered only for AE analysis, and considered for both AE and efficacy/effectiveness analyses). As is clear from the table, none of the predictors function perfectly. However, substantial differences exist for multiple variables, which make modeling based on some combination of these variables feasible via a regression approach. As expected, the vast majority of relevant studies were either meta-analyses or RCTs; in contrast, the results in irrelevant studies were occasionally tagged as in vitro or animal studies (not shown). Furthermore, large majorities of studies in every included category (efficacy, AE, or both analyses) contained indexing information that described the therapeutic use of a preferred intervention or the treatment of a preferred outcome. By contrast, relatively few excluded studies contained indexing information that linked the therapeutic use of a preferred intervention (0.257) or the treatment of a preferred outcome (0.192).
Table 4
Characteristics of the original LBD review (by category of article).
Performance Predicting Efficacy/Effectiveness Results
Predicting Articles Relevant to Efficacy/Effectiveness for AAP Review
We developed a model for predicting the inclusion of efficacy/effectiveness articles using the original search results. Figure 3 shows the relative weights of different variables for GBM; variables with larger relative weights account for large fractions of the total explanatory power. In keeping with some of the differences in frequency distributions between included and excluded studies, “RCT” contains a substantial portion of the model’s explanatory power. Weights for GLMnet were similar, with “RCT” providing the greatest explanatory power.
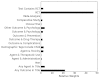
Figure 3
Relative weights for variables in AAP efficacy analysis.
Table 5 shows efficacy/effectiveness results for all models (GLMnet, GBM, and hybrid) at multiple thresholds. For AAP, all models achieved high sensitivity when predicting on the original sample at relatively high thresholds (p≤0.02). For example, the GLMnet-based predictive model achieved a sensitivity of 1 and PPV of 0.38 using a threshold of 0.02 for predicting relevant articles in the original sample. Achieving good results on the original sample was expected because the underlying model was derived from the same outcomes and explanatory variables. Applying the GLMnet model to the updated AAP literature search results yielded a sensitivity of 0.921 and PPV of 0.185; GBM and hybrid models performed similarly.
Table 5
Model performance for efficacy/effectiveness.
Figure 4 shows these results graphically using a histogram of the prediction probabilities for the update, divided according to whether the article met final inclusion criteria. Excluded articles were predominantly given probabilities very close to zero, while articles considered for efficacy/effectiveness had probabilities that spanned the entire spectrum. Of note, this histogram displays densities; even small densities of false positive articles (from the much larger group of negative articles) entail a relatively high proportion of false positives among model predictions, which limits the PPV to 0.185.
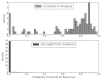
Figure 4
Histogram AAP efficacy analysis: distribution of predictions.
Predicting Articles Relevant to Efficacy/Effectiveness for LBD Review
Figure 5 shows the relative weights of variables included in the GBM model of efficacy for LBD (weights for GLMnet were similar, in that RCT contained the greatest explanatory power). As in the AAP analysis, terms such as RCT and meta-analysis are important. Clearly, other variables carried different weights in the AAP analysis, suggesting that predictive models may need to be topic-specific.
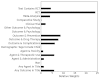
Figure 5
Relative weights for variables in LBD efficacy analysis.
The efficacy/effectiveness results were similar for the LBD review (Table 5.) The GLMnet-based predictive model achieved sensitivity of 0.982 and PPV of 0.174 using a threshold of 0.02 for predicting relevant articles in the original sample. We then tested these results on the updated literature search results; GLMnet yielded sensitivity of 0.905 and PPV of 0.102.
Figure 6 shows model prediction performance on the LBD updated search graphically using a histogram of the prediction probabilities. Excluded articles were generally assigned very low probabilities. As in Figure 4 (for AAP), the small percentage of false positive articles reduced the PPV to 0.102 due to the much greater number of negative articles overall.
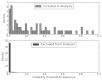
Figure 6
Histogram LBD efficacy analysis: distribution of predictions.
Performance Retrieving Articles Considered for AE Analysis
Predicting AE-Relevant Articles for AAP Update
We empirically developed a model for predicting AE articles using the original search results. We show the relative importance of the same select variables in Figure 7 for GBM (though GLMnet produced similar weights). Again, the “RCT” variable remains extremely important, even as the importance of the remaining explanatory variables differs from the efficacy/effectiveness models.
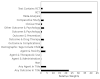
Figure 7
Relative weights for variables in AAP AE analysis.
We show results from all models in Table 6. The GLMnet-based predictive model achieved a sensitivity of 0.978 and PPV of 0.215 using a threshold of 0.02 for predicting articles relevant to AEs in the original sample. Applying the GLMnet-based model to the updated literature search results yielded a sensitivity of 0.981 and PPV of 0.09. The GBM-based model performed better in the original (sensitivity, 1; PPV, 0.274) but worse in the update (sensitivity, 0.895; PPV, 0.11). The hybrid model yielded similar sensitivity to the GLMnet model, but worse PPV.
Table 6
Model performance for AEs.
Figure 8 shows these results graphically using a histogram of the prediction probabilities, divided according to whether the article met final inclusion criteria. Articles not considered for AE analyses were predominantly assigned probabilities very close to zero; included articles had probabilities that spanned the entire spectrum including the 2 percent that were assigned a probability of inclusion <0.02.
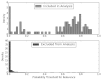
Figure 8
Histogram AAP AE analysis: distribution of predictions.
Predicting AE-Relevant Articles for LBD Update
Figure 9 shows key variables for this analysis (GBM only, though weights for GLMnet were similar, in that RCT contained the greatest explanatory power). By inspection, these importance weights do not appear extremely dissimilar to those from the AAP analysis.
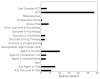
Figure 9
Relative weights for variables in LBD AE analysis.
The GLMnet-based predictive model achieved a sensitivity of 0.964 and PPV of 0.21 using a threshold of 0.02 for predicting articles relevant for the AE analysis in the original LBD review (Table 6.) However, we were able to predict AE-relevant articles with a substantially reduced sensitivity (0.685) when compared to the AAP results. Reducing the threshold substantially (i.e., retaining all articles with p ≥0.001) would increase sensitivity to 0.946 but decrease PPV to 0.04. Our results for GBM-based and hybrid models were not substantially better at threshold p ≥0.02, with the hybrid model achieving sensitivity of 0.707 and PPV of 0.112.
Figure 10 shows these results graphically as many AE articles relevant to the LBD update were assigned relatively low prediction probabilities. In fact, 11.6 percent of AE-relevant articles were assigned probabilities <0.005. When we examined missed AE articles, we noted that there were relatively few relevant large observational studies (cohort and case-control studies) in the original review. As a result, the both the GLMnet- and GBM-based models assigned lower probabilities to observational studies in the LBD update as well. However, observational studies were more important in the update because the SCEPC researchers focused on several newly identified AEs that were largely studied in cohort and case-control studies.
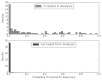
Figure 10
Histogram LBD AE analysis: distribution of predictions.
Performance Predicting Any Relevant Result and Potential Workload Reductions
The workflow in many AHRQ comparative effectiveness reviews includes a first step in which reviewers select all articles that might be relevant to AEs or efficacy, and as the second step, a process that reviews the full text of articles to determine their relevance to efficacy/effectiveness or AE analyses. To simulate how our approach might improve the workflow for updates, we determined the GLMnet-based model’s sensitivity and PPV at various thresholds for retrieving all AE and efficacy/effectiveness analyses. Sensitivity and PPV for a particular threshold were determined by selecting articles if the maximum predicted relevance from either model (efficacy/effectiveness or AE) exceeded the threshold. We show how sensitivity and the number needed to screen change as the threshold changes in Table 7. (We do not show sensitivities < 0.75 as these results are unlikely to be useful to comparative effectiveness review researchers.) We selected a threshold of p≥0.01 based on the performance of the model in the original search results, in which a threshold of p≥0.01 yielded perfect sensitivity with 58.1 percent of screening saved. When we applied this threshold to the update predictions, the projected sensitivity model exceeded 0.99, whereas the proportion of title/abstract screening saved was 55.4 percent. In other words, the total number of articles to be screened would have been reduced from 3,591 to 1,601. By contrast, the hybrid model had identical sensitivity, but more limited workload reductions at the same threshold (p≥0.01).
Table 7
GLMnet model performance in retrieving any relevant article (AAP Update).
The GLMnet-based model for LBD performed worse, in that the model selected articles for the update with a sensitivity of 0.795 at a threshold of p≥0.02 (compared to 0.974 for AAP). (Tables 7 and 8.) However, this approach still provided potential benefits once we selected a suitable threshold. We chose a threshold of p≥0.001 based on the performance of the model in the original search results, in which a threshold of p≥0.001 yielded perfect sensitivity with 66.8 percent of screening saved. Using the same threshold when evaluating results in the update yielded perfect sensitivity accompanying the drop in the projected article screening burden from 7,051 to 2,597 (63.2%). While the probability thresholds differed between the AAP and LBD updates (0.001 in LBD and 0.01 in AAP), both thresholds could be derived from the original modeling process.
Table 8
GLMnet model performance in retrieving any relevant article (LBD update).
We show these results graphically using ROC curves (Figures 11 and 12). The AUC for the GLMnet method (in the AAP study) was 0.943 (95% CI: 0.927 to 0.960) versus 0.925 (95% CI: 0.899 to 0.950) with GBM. The p-value for null hypothesis of equality was 0.007. Similarly, the AUC for the GLMnet method (in the LBD study) was 0.954 (95% CI: 0.943 to 0.965) versus 0.947 (95% CI: 0.933 to 0.961) for GBM. In the LBD study, p-value for null hypothesis of equality was 0.06. Both results suggest that the ROC curves differed between the two studies; in addition, GLMnet seems to perform somewhat better than GBM visually as well. Still, it would be difficult to establish GLMnet’s superiority in this context (comparative effectiveness reviewing updating) without further studies.
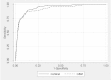
Figure 11
ROC curve for classifying AAP articles. AAP = antipsychotic systematic review; GBM = gradient boosting machine; GLMnet = generalized linear models with convex penalties; ROC = receiver operating characteristic
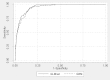
Figure 12
ROC curve for classifying LBD articles. GBM = gradient boosting machine; GLMnet = generalized linear models with convex penalties; LBD = low bone density systematic review; ROC = receiver operating characteristic
Evaluation of Model Prediction Errors
SCEPC researchers independently evaluated articles in the update that were included in the final reports but were assigned low probability scores by the statistical classifiers. We initially chose a probability threshold (p≥0.02) that reduced workload substantially; however, this threshold entailed 29 false negatives. Nearly all false negatives were non-RCT studies (along with an RCT that was not tagged as such by MEDLINE). Of the 29 false negatives (at threshold p≥0.02 from both updates), 26 were from the LBD update. The GLMnet model for LBD missed one RCT because the drug of interest (“raloxifene”) was tagged with “pharmacology” and not a more revealing subheading. The remaining LBD false-negatives were non-RCT studies (including meta-analyses, case-control studies, retrospective analyses of claims databases, case-control studies, and analyses of government registries). It is difficult to determine whether similar studies were present in the original data without actually re-reading all earlier studies, but we did note that words such as “cohort” and “database” were poorly represented among both included and excluded studies in the original LBD report.
In considering the models used to predict inclusion of any relevant articles (Tables 7 and 8), just one article (from the AAP update) would have been excluded.42 This article was likely assigned a low probability because it was tagged as a letter although it reported on a clinical trial. Of note, despite missing this trial using machine learning, EPC researchers might have been able to retrieve this trial because it was referenced in a relevant article and would plausibly have been caught using the researchers’ analyses of references accepted in the final reports.43
EPC researchers also evaluated several citations that were assigned high relevance probabilities but were deemed irrelevant by the original comparative effectiveness review researchers; none of these decisions changed on re-evaluation. These studies included one small RCT on calcitriol (that did not report fracture outcomes) and another RCT in a modest sized specialized population (Parkinson’s patients).44,45
- Results - A Pilot Study Using Machine Learning and Domain Knowledge To Facilitat...Results - A Pilot Study Using Machine Learning and Domain Knowledge To Facilitate Comparative Effectiveness Review Updating
Your browsing activity is empty.
Activity recording is turned off.
See more...