From: Results
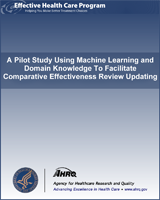
A Pilot Study Using Machine Learning and Domain Knowledge To Facilitate Comparative Effectiveness Review Updating [Internet].
Dalal SR, Shekelle PG, Hempel S, et al.
Rockville (MD): Agency for Healthcare Research and Quality (US); 2012 Sep.
NCBI Bookshelf. A service of the National Library of Medicine, National Institutes of Health.