NCBI Bookshelf. A service of the National Library of Medicine, National Institutes of Health.
Saldanha IJ, Skelly AC, Ley KV, et al. Inclusion of Nonrandomized Studies of Interventions in Systematic Reviews of Intervention Effectiveness: An Update [Internet]. Rockville (MD): Agency for Healthcare Research and Quality (US); 2022 Sep.
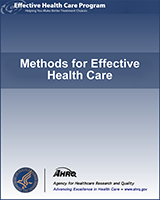
Inclusion of Nonrandomized Studies of Interventions in Systematic Reviews of Intervention Effectiveness: An Update [Internet].
Show detailsPrevious guidance on the inclusion of NRSIs in EPC SRs recommended their inclusion as being conditional on RCTs being insufficient to address the research question and required NRSIs to fill the perceived gap.1, 2 In effect, this approach handles NRSIs as a secondary source of information. Recent advances in causal inference call this handling into question. Specifically, improvements in design elements and analytic methods can support more sophisticated analyses. These methods may include trial emulation approaches, in which NRSIs carefully specify criteria to make the potential sources of bias transparent and clarify the concordance between results from NRSIs and RCTs, and causal inference analytic approaches, such as propensity scoring, instrumental variable, regression discontinuity, and difference-in-difference approaches, which facilitate causal inferences despite lack of randomization. This section explores these advanced analytic methods for comparative NRSIs.
9.1. Advanced Analytic Approaches: Trial Emulation Efforts
Trial emulation efforts aim to analyze NRSI data using designs that simulate a targeted, hypothetical RCT. Every attempt is made to emulate the features of the targeted RCT, except that randomization is not conducted.57, 58 Early emulation studies have shown that discrepancies in effect estimates between RCTs and NRSIs that address a similar question are to a lesser extent the result of unmeasured confounding and can be largely explained by differences in study design and analytic methods, such as time since disease onset and duration of followup.59–62 However, unmeasured confounding and other issues are still important and may not be adequately addressed in some trial emulation studies.63, 64
The Randomized Controlled Trials Duplicated Using Prospective Longitudinal Insurance Claims: Applying Techniques of Epidemiology (RCT DUPLICATE) Initiative is a large systematic evaluation of the ability of NRSIs using routine clinical data to replicate RCTs.65 The initiative aims to quantify differences between NRSIs that use routine clinical data and RCTs as well as the factors that may explain the differences. Results from the first 10 emulations focused on insurance claims data on cardiovascular outcomes of antidiabetic or antiplatelet medications. The results pertaining to the agreement between RCTs and NRSIs were mixed; 80 percent of the emulations achieved agreement in estimates66. Preliminary results in this limited clinical area support the conclusion that selection of active comparator therapies with similar indications and use patterns increases agreement between results of NRSIs and RCTs.66
Trial emulation studies have shown that NRSIs can be a supplemental source of high-quality evidence for answering questions of intervention effectiveness. Franklin and colleagues have provided general recommendations for evaluating quality and potential biases in trial emulation studies.65 However, reliably distinguishing high-quality trial emulation studies from low-quality ones remains a challenge. Key considerations include the availability of data regarding confounders that may be imbalanced between study groups, whether the outcomes are defined similarly in each group, and whether the study power is sufficient to detect clinically meaningful differences. Confidence in the validity of trial emulation studies is increased if they report sensitivity analyses demonstrating minimal impact of the chosen design and analytic decisions.65
The inclusion of trial emulation studies in SRs is consistent with the goal of including the highest quality evidence. Some regulatory bodies, healthcare payors, health systems, and guideline developers consider trial emulation studies for drug approvals, drug labeling, formulary decisions, and evidence-based practice.66–69 As data sources and statistical approaches improve, the opportunities for incorporating high-quality NRSIs that emulate the results of RCTs will continue to increase.68 By understanding when and why results between NRSIs and RCTs might differ, reviewers can conduct “cross-design synthesis” by conducting meta-analyses across study designs to provide stakeholders with more pragmatic conclusions and valuable insights about the effectiveness of interventions that may not be gleaned by using RCT evidence alone.68, 70
9.2. Advanced Analytic Approaches: Causal Inference Methods
As noted in Section 3, randomization serves to prevent biases. Yet, NRSIs, under specific assumptions in which quasi-randomness occurs, can be analyzed in ways that facilitate causal inference. We discuss herein four approaches that, in our opinion, have the most merit for SRs: propensity scoring, instrumental variables, regression discontinuity, and difference-indifferences. The last three approaches capitalize on the existence of specific conditions that create quasi-random assignment of treatments, which allows for the estimation of causal effects even in the presence of selection bias and confounding. We highlight these methods because they are now commonly used advanced methods to evaluate causality. For each approach, we provide an explanation, the main assumption(s), and an example.
9.2.1. Propensity Scoring Methods
9.2.1.1. Explanation
Propensity scoring is a set of analytic methods to adjust for observed confounders in an NRSI. The propensity score is defined as the conditional probability of receiving a certain intervention, given a set of covariates.71 Yet, rather than maximizing the conditional probability of receiving the intervention, the primary purpose of propensity scoring is to balance the set of confounders between the two intervention groups.72–76 Like all probabilities, the propensity score ranges from 0 to 1. The closer to 1, the stronger the probability that the participant would be in the intervention group; likewise, the closer to 0, the stronger the probability the participant would be in the control group.
There are two steps to a propensity score calculation. In the first step, an appropriate set of baseline covariates must be identified. Identification of relevant baseline covariates requires careful thought and should not include covariates simply because they are available in the dataset. Once the relevant covariates are identified, the second step involves estimation of each participant’s probability of being treated (the propensity score). This can be done using such methods as binomial regression (using a logistic or probit model), statistical learning algorithms (classification trees or ensemble methods),77, 78 and covariate balancing (which predicts treatment assignment while simultaneously optimizing covariate balance).79
Once the propensity score is calculated, one can use it as a covariate when estimating the treatment effect in a regression, matching, stratification, or weighting approach. A discussion of the strengths and weaknesses of each of these approaches is beyond the scope of this guidance.
9.2.1.2. Assumptions
The major assumption required of a propensity score analysis is that of strongly ignorable treatment assignment (ignorability assumption). This assumption means that the treatment assignment and potential outcomes are conditionally independent, given the observed covariates.71, 72 In other words, if the important confounders are identified, the only difference between the treatment and control groups is the treatment. If this assumption holds, the propensity score analysis is considered to produce unbiased estimates of the treatment effect. The ignorability assumption is fulfilled if all important covariates are identified (i.e., if there are no important unobserved confounders). Failure to identify all important confounders is a major limitation of propensity score analyses.71, 72
9.2.1.3. Example
Assessment of the effects of mental health treatments in pregnancy serves as a common example of the use of propensity score analysis.80, 81 RCTs of psychotropic medications in pregnant women are rare. As a result, the evidence base relies on NRSIs. As discussed in Section 7, confounding is an important threat to validity of NRSIs. Propensity score analysis attempt to address confounding by modeling receipt of treatment on a wide range of covariates that are carefully selected. A key factor in predicting treatment receipt that may often be absent from large databases is the severity of the underlying condition. As a result, propensity score analyses may lack adjustment for severity or require the use of severity proxies, such as number of diagnoses. As with other analytic approaches, propensity score analyses are limited by the availability and completeness of data representing all confounders.
9.2.2. Instrumental Variable Approach
9.2.2.1. Explanation
NRSIs may choose to use an instrumental variable (IV) analytic approach when randomization is not feasible. In regression analysis of such studies, an IV refers to a variable that meaningfully relates to the intervention but affects the outcome only through the intervention (i.e., the IV has no direct effect on the outcome).82 In such a context, an IV approach can simulate randomization because any variation in the outcome that is associated with variation of the IV is effectively due to the intervention. Randomization can be considered as the quintessential IV; random assignment occurs for the subset of the sample that received the intervention due to variation in the instrument and thus provides a causal treatment effect for this subset.
9.2.2.2. Assumptions
The IV analytic approach requires two main assumptions.83 The relevance assumption refers to the existence of an association between the IV and the intervention variable. The exclusion assumption refers to the lack of a direct association between the IV and the outcome variable.83
9.2.2.3. Example
The effect of cardiac catheterization on mortality from acute myocardial infarction (AMI) is a well-known example of use of the IV analytic approach.82, 84 The IV used was the additional distance that a patient must travel beyond the nearest hospital to get to a hospital that performs catheterization (“distance difference”). The relevance assumption holds because a smaller distance difference also makes it more likely that the patient received catheterization because the additional barrier of travel is lower. The exclusion assumption holds because the IV (i.e., the distance difference) is considered unrelated to mortality in the study, except through catheterization. Because the distance difference thus quasi-randomly “assigns” the intervention to patients, the IV approach can estimate the effect of catheterization on mortality in the subset of patients that received catheterization because they were closer to a catheterization hospital.
Regarding the assumptions in this example, the relevance assumption is fulfilled because a meaningful relationship can be demonstrated between the distance difference and catheterization. However, one cannot test the exclusion assumption due to the same reason that an IV is required, i.e., the relationship between the intervention variable and the outcome may be confounded by unobserved variables. Therefore, any test for a direct effect of the instrument on the outcome will also be confounded by these same unobserved variables. Here, expert knowledge should attempt to rule out the existence of any direct effect of differential distance on AMI mortality.
Two challenges with the IV approach worth noting are (1) it is often difficult to find an appropriate IV for a given research question, and (2) the results of IV analyses may be biased if the assumptions, which are often unverifiable, are not fulfilled.
9.2.3. Regression Discontinuity Design Approach
9.2.3.1. Explanation
A regression discontinuity design uses a threshold or cutoff point to assign an intervention to those on one side of the threshold and no intervention to those immediately on the other side of the threshold.
9.2.3.2. Assumption
The assumption is that study participants who are close to the threshold, on either side, are comparable in factors other than receipt of the intervention, making the intervention assignment arguably random.
9.2.3.3. Example
To investigate the effect of the human papilloma virus (HPV) vaccine on adolescent sexual behavior, researchers assessed a policy in Ontario, Canada, which made girls born after December 31, 1993, eligible for the vaccine and girls born on or before that date ineligible.85 Girls born on or close to the date threshold (on either side) are arguably similar because small differences in birth date are not expected to affect sexual behavior. Because of the comparability in the populations before and after the policy change date, the regression discontinuity design could robustly estimate the effect of the HPV vaccine on health outcomes by comparing those born just before and just after the date threshold.
A limitation of this approach is that although known confounders (i.e., observable characteristics) can be compared between the two groups, unknown confounders (i.e., unobserved characteristics) cannot be compared to rule out unmeasured confounding. In this study, researchers tested whether girls born on either side of the date were dissimilar on observable characteristics but could not rule out the possibility of dissimilarities between the groups on unobservable characteristics.
9.2.4. Difference-in-Difference Approach
9.2.4.1. Explanation
A difference-in-difference (DiD) approach compares the changes in an outcome over time between a group that received the intervention and a group that did not receive it. The approach adjusts the treatment effect estimate for factors other than the intervention that may also impact the outcome. The first difference (within-group) controls for time-invariant differences within the intervention group. The second difference (between-group) controls for time-varying factors that are common across groups.
9.2.4.2. Assumption
The important assumption is that the outcome changes that occurred within the intervention group would have been the same for the control group had the intervention group not been treated (i.e., the counterfactual). If this assumption, known as the common trends assumption, is not fulfilled, the DiD approach would lead to a biased estimate of the treatment effect.
9.2.4.3. Example
An example of use of the DiD approach was the estimation of the effect of the 2014 state-level expansions in Medicaid insurance coverage under the Affordable Care Act on clinical outcomes.86 Researchers found that expanded coverage decreased the proportion of men with high prostate-specific antigen (PSA) results at the time of cancer diagnosis, which suggested that Medicaid expansion improved access to screening. Some states expanded Medicaid coverage, while others did not. The researchers estimated the difference between the proportion of men with high PSA scores after versus before 2014 and compared that difference between states that did and did not expand Medicaid coverage. While it is not possible to directly test the counterfactual, i.e., whether trends would be the same in Medicaid non-expansion states and expansion states in the absence of the expansion, it is possible to indirectly test the common trends assumption. For instance, a visual inspection of a graph of PSA trends for expansion and non-expansion states in the years before the policy implementation suggested common trends.
9.3. Summary of Considerations Regarding Advanced Methods
The advanced methods for NRSIs described above largely attempt to simulate randomization by balancing population characteristics for the intervention and the comparator groups on observed confounders (e.g., trial replication, propensity scores) and/or unobserved confounders (e.g., IV approach). In theory, such methods support making causal inferences regarding the impact of an intervention on outcomes of interest. As discussed, validity of the inferences made using these methods depends on the validity and robustness of underlying assumptions (e.g., the exclusion assumption in the IV approach) and modeling used. In practice, not all confounders are available or can be measured. Authors of NRSIs may lack the requisite expertise to appropriately apply the often-complex analytic methods and to verify all assumptions, some of which may be unverifiable. Moreover, evaluation of these assumptions and methods is often challenging, if not impossible, for most reviewers because it requires advanced knowledge of specific statistical modeling methods and a deep understanding of the study context and data structure. Another important consideration for reviewers is that modeling assumptions are often unverifiable due to lack of access to original datasets and/or inadequate reporting. We are not aware of risk of bias assessment tools that specifically evaluate the potential flaws of these advanced designs. Reviewers should acquire expertise or consult experts in these methods when including these types of NRSIs in an SR.
- Advanced Analytic Methods for NRSIs - Inclusion of Nonrandomized Studies of Inte...Advanced Analytic Methods for NRSIs - Inclusion of Nonrandomized Studies of Interventions in Systematic Reviews of Intervention Effectiveness: An Update
Your browsing activity is empty.
Activity recording is turned off.
See more...