NCBI Bookshelf. A service of the National Library of Medicine, National Institutes of Health.
Saldanha IJ, Skelly AC, Ley KV, et al. Inclusion of Nonrandomized Studies of Interventions in Systematic Reviews of Intervention Effectiveness: An Update [Internet]. Rockville (MD): Agency for Healthcare Research and Quality (US); 2022 Sep.
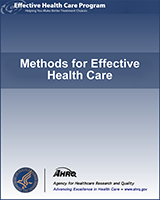
Inclusion of Nonrandomized Studies of Interventions in Systematic Reviews of Intervention Effectiveness: An Update [Internet].
Show detailsIn NRSIs, data sources vary and can include (1) routinely collected data, such as clinic records, electronic medical records, administrative claims data, and disease registries, and (2) customized data, such as study-specific visit data and patient-generated data, e.g., from fitness trackers or home medical equipment.
“Big data” is an ill-defined term that is increasingly used to describe large volumes of either routinely collected or customized data, as listed above. Studies that are conducted using big data offer the obvious advantage of very large sample sizes (often with many thousands of patients), potentially representing routine clinical practice well. Such data may permit evaluation of rare health outcomes or rare diseases and provide contextual information regarding effectiveness or harms. Because of large sample sizes, high precision of treatment effect sizes is often attained, which may or may not be clinically relevant. However, studies using big data are usually subject to the same sorts of threats to validity (e.g., confounding, selection bias) as studies using other data sources. Moreover, studies using big data are often prone to inaccuracies in diagnostic and intervention coding (i.e., misclassification of interventions and/or outcomes), inconsistent and/or incomplete follow-up data, and variability in reporting and interpretation.51–54 For reviewers, an additional challenge is that subsets of the population in one big data study may overlap with other studies included in the SR. Reviewers may find it hard to detect and handle the potential double-counting arising from such overlap.
Understanding data sources and the context in which the data were generated can greatly help interpret the findings of NRSIs. For example, controlled clinical trials, in which participants are prospectively assigned to treatment groups by researchers without the use of randomization, usually obtain customized data using similar methods as in RCTs. However, NRSIs using administrative claims data, which are usually not gathered for the purposes of research, may lack important information regarding potential confounders. Additionally, there may be a substantial amount of missing data when sources such as patient-generated data are used. When such missing data are “informative,” e.g., missing not at random (MNAR),55 this can lead to findings that are subject to emigrative selection biases (i.e., bias due to post-baseline exclusion of some participants from the study for reasons related to both the exposure and outcome).14, 56
- Various Data Sources for NRSIs - Inclusion of Nonrandomized Studies of Intervent...Various Data Sources for NRSIs - Inclusion of Nonrandomized Studies of Interventions in Systematic Reviews of Intervention Effectiveness: An Update
Your browsing activity is empty.
Activity recording is turned off.
See more...