NCBI Bookshelf. A service of the National Library of Medicine, National Institutes of Health.
Saldanha IJ, Skelly AC, Ley KV, et al. Inclusion of Nonrandomized Studies of Interventions in Systematic Reviews of Intervention Effectiveness: An Update [Internet]. Rockville (MD): Agency for Healthcare Research and Quality (US); 2022 Sep.
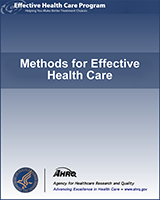
Inclusion of Nonrandomized Studies of Interventions in Systematic Reviews of Intervention Effectiveness: An Update [Internet].
Show details10.1. Planning for the Inclusion of NRSIs
When making the decision of whether to include NRSIs in an SR, traditional evidence hierarchies are not as relevant as specific study design features. As noted in Section 4, we agree with other methodologists that when considering NRSIs, reviewers should, instead of relying on study design labels (e.g., cohort studies), evaluate study methods and analytic approaches.3, 4
At a minimum, decisions to include or exclude NRSIs should be explained or justified as part of the SR protocol. The Cochrane Handbook for Systematic Reviews of Interventions describes some of the leading reasons to include NRSIs in SRs, many of which are related to the common limitations of RCTs or their absence.4 For example, as we discuss in Section 3, large RCTs with long-term outcomes may not be conducted for ethical, practical, and/or resource-related reasons. Sometimes, even when RCTs are conducted, there may not be an adequate number of well-conducted RCTs addressing rare diseases or certain subpopulations. Even when well-conducted RCTs are available, they may not replicate typical clinical practice and outcomes as closely as NRSIs might. Low-quality evidence may often be better than no evidence at all for important clinical and policy decision making. However, as we discuss in Section 7, NRSIs have a higher susceptibility to confounding and other biases. A heavily biased estimate can be worse than no estimate because it could lead to erroneous conclusions and preclude higher quality and more reliable research. On the other hand, well-conducted NRSIs, such as those that are well-analyzed or use the advanced methods described in Section 9 carefully and with relevant assumptions fulfilled, may be less prone to confounding and other biases.
10.2. Developing Searches for NRSIs
“Hedges,” also known as filters, are standardized search strategies that can be used to help retrieve relevant articles from electronic databases. Hedges are applied to improve the retrieval of various kinds of evidence, such as NRSIs. They are often used to identify study designs and, to a lesser extent, clinical concepts, such as treatment, diagnosis, and prognosis. To our knowledge, there are no published NRSI hedges with greater than 92-percent sensitivity,87 so they should be used with some caution. See Appendix A for a sample of hedges for some common NRSI designs.
Another option is to use a hedge that eliminates unwanted publication types. These hedges retrieve records describing a broad range of study types while filtering out those that do not include primary research. We are not aware of published hedges for this type of exclusion. To maximize sensitivity, it may be best to not use hedges. In this case, machine-learning based screening tools, such as Abstrackr (http://abstrackr.cebm.brown.edu), that prioritize unscreened records based on the manual labels of previously screened citations, may be a particularly useful alternative.88
Often, NRSIs are used to identify evidence providing data on long-term adverse events. Adverse events hedges limit not by publication types but to studies reporting any type of adverse event. If NRSIs are being used only to evaluate harms, these hedges can be a good option. Appendix A includes examples of published adverse event hedges.
10.3. Assessing the Risk of Bias in NRSIs
Existing instruments for assessing the risk of bias in NRSIs89, 90 vary in their (1) theoretical and empirical foundations; (2) comprehensiveness when considering sources of bias for a range of study designs and analytic approaches; (3) validation, documentation, and ease of use (length/complexity); and (4) presentation and transparency of the risk of bias assessments. Notably, although current AHRQ guidance does not recommend a specific tool for use in SRs, the guidance suggests that the chosen tool should:
- Be specifically designed for the study designs being evaluated
- Allow transparency in how assessments are made
- Be based on theory and supported by empirical evidence
- Avoid the presentation of risk of bias assessments as a numerical score.43
Sources of bias that are uniquely relevant to comparative NRSIs are described in Section 7 of this guidance. They include the potential for selection bias, confounding, and misclassification of interventions. It is worth noting that risk of bias assessments should focus on domains that contribute to bias and, as such, a well-conducted NRSI may sometimes be of better methodological quality than a poorly conducted RCT.
A key consideration in assessing the risk of bias in NRSIs is that topic-specific expertise is required to identify relevant confounders. Therefore, reviewer teams should include a mix of methodologic and content-specific expertise.
10.4. Interpreting Results From NRSIs
As discussed in Section 7, when interpreting results of NRSIs, it is important to remember that confounding is a key threat to validity. Across NRSI designs and analytic approaches, the ability to successfully account for confounding can vary greatly. For example, a before-after study (with a historic control group) may not be able to disentangle temporal changes of outcomes independent of the intervention being tested, while a prospective cohort study (with a concurrent control group) does not have the same level of challenge. The ability of an individual NRSI to adjust for confounding also depends on availability of data on the confounders, their precise and valid measurement, and analytic approaches used. As a result, poor adjustment (i.e., inadequate or overadjustment) of confounding in NRSIs can lead to bias, which can overestimate or underestimate the treatment effect, sometimes greatly.13 Therefore, when including NRSIs, it is important to evaluate the extent to which confounding has been considered and effectively addressed. A statistic, known as the E-value, has been proposed to indicate the potential for an unmeasured confounder to have impacted the results for a given outcome in a study. The E-value has been defined as the minimum magnitude of association that an unmeasured confounder would need to have with both the intervention and the outcome to fully explain away a treatment effect, conditional on the other measured covariates.91 The larger the E-value the larger the magnitude of unmeasured confounding would need to be to explain away an effect estimate.91
10.5. Incorporating Data From NRSIs Into Meta-Analyses
When interpreting data regarding a treatment effect from an NRSI for the purpose of metaanalysis, reviewers should consider: (1) whether the estimate was adjusted; (2) whether and how important confounders were handled in the design and analysis; (3) whether the confounders were measured in a precise and valid way; and (4) whether underlying assumptions of the analytic approach were evaluated and validated. Experts, such as clinical experts, statisticians, and SR methodologists, should assess whether the NRSI adequately adjusted for important confounding and whether estimates from NRSIs should be combined with those from other NRSIs and RCTs.
There sometimes is heterogeneity in estimates of treatment effect between RCTs and NRSIs. This methodological heterogeneity can be due to many factors, including differences in risks of selection bias, confounding, and other biases, potential treatment effect modifiers, and sampling error. However, empirical evaluation of such heterogeneity is limited. Heterogeneity statistical indicators (e.g., I2, H2) and statistical tests (e.g., Cochran’s Q test) only evaluate statistical variations of observed treatment effects and do not capture the true uncertainty of the underlying true treatment effect.92, 93 Investigating sources of heterogeneity through subgroup analysis or meta-regression is, by nature, exploratory and suffers from multiple potential shortcomings, such as the lack of sufficient detail reported in the included studies, small numbers of studies, and (in the case of meta-regression) collinearity.92, 94
When meta-analysis is deemed appropriate, reviewers should be cautious of combining different NRSI designs and/or analytic approaches or comparing NRSIs with RCTs. The advantages of conducting meta-analysis include the attainment of a singular overall estimate of the treatment effect that is relatively precise and based on a broader set of participants, with a potentially greater strength of evidence.95 However, it is more likely than for meta-analyses of only RCTs that studies at higher risk of bias will be included with studies at lower risk of bias in the SR, which could lead to more biased results. Moreover, because of their generally large sample sizes, effect size estimates from individual NRSIs will generally be more precise and therefore will be assigned greater weights in a meta-analysis that weights studies using the inverse variance method.95
As an initial step, reviewers should examine the consistency of study findings between different NRSI designs/analytic approaches and between NRSIs and RCTs. Graphical displays, such as forest plots without an overall summary estimate, can be used to visually assess consistency in the direction and magnitude of treatment effect estimates and their confidence intervals. If applied, formal statistical tests, such as meta-regression and Cochran’s Q test, should be used in concert with the considerations listed above (rather than as litmus tests to indicate the presence or absence of notable heterogeneity). Sensitivity analyses around study quality may be important.
Regardless of the study designs being analyzed, when deciding to combine study findings quantitatively (i.e., in a meta-analysis), considerations should include, but should not be limited to, similarity of the included studies in terms of population, intervention, comparator, outcome, timing, and settings (PICOTS) and type of NRSI design and analytic approach. When meta-analyses include studies of different designs, reviewers should present subgroup analyses by study design (at a minimum, RCTs vs. NRSIs). It may also be appropriate to conduct sensitivity analyses that exclude high-risk of bias NRSIs to avoid overestimating the strength of evidence. As a general rule, reviewers should not use statistical tests or indicators of heterogeneity (e.g., I2, H2, Cochran’s Q test) purely as litmus tests to determine the appropriateness of conducting a meta-analysis.95
If different NRSI designs and analytic approaches present consistent effect estimates and confidence intervals, and if they are generally consistent with RCT effect estimates and confidence intervals, it may be appropriate to meta-analyze all the studies. However, reviewers should also present meta-analyzed results within subgroups by study design.92
If different NRSIs designs and analytic approaches and/or RCTs present inconsistent effect estimates and confidence intervals, in most instances, reviewers should avoid meta-analyzing estimates across study designs. Instead, evidence and associated heterogeneity should be reported separately, and the sources of inconsistency should be investigated, if possible, through subgroup analysis (or meta-regression). The “best evidence” approach is useful to select a body of evidence for investigation; investigators should decide whether RCTs, NRSIs in general, or specific NRSI design and analytic approaches represent lower risk of bias and better applicability to clinical practice. AHRQ’s 2011 Methods Report–A Framework for “Best Evidence” Approaches in Systematic Reviews–provides detailed discussion regarding this approach.38
10.6. Grading the Strength of the Body of Evidence That Includes NRSIs
10.6.1. GRADE
The Grading of Recommendations Assessment, Development, and Evaluation (GRADE) system is widely used to rate the certainty of evidence identified in SRs.96 Domains of evidence considered in this system include study design, risk of bias, indirectness, inconsistency, imprecision, publication bias, dose-response, and magnitude of effect. Ratings for each domain feed into ratings for the certainty of the overall body of evidence for a given outcome; certainty may be rated as high, moderate, low, or very low.
10.6.2. AHRQ EPC Program Approach to Grading the Strength of a Body of Evidence That Includes NRSIs
The AHRQ EPC Program adopted a modified version of the GRADE system for use in EPC SRs.97 The main modification is that applicability is separated from the indirectness domain to be an independent domain. This decision stems from the wide remit of EPC Program SRs that may have a diverse set of end-users with potentially unique parameters for judging applicability. The Program also uses the term “insufficient” rather than “very low” to describe the lowest level of evidence.97
Information in the rest of this section summarizes guidance specific to NRSIs that was provided in the 2013 AHRQ EPC Methods Guide Update.97
10.6.2.1. Developing the Protocol
When developing the SR protocol, reviewers should establish a priori criteria to identify studies with design elements that would constitute an unacceptably high risk of bias (e.g., lack of adjustment for confounders). In addition to specifying the rationale, procedures, and decision rules, reviewers should explicitly describe the processes for synthesizing evidence from RCTs and NRSIs and determining overall strength of evidence.
10.6.2.2. Rating Strength of Evidence Domains
For each outcome and intervention comparison of interest, when both RCTs and NRSIs are identified, reviewers should describe whether evidence from NRSIs agrees or conflicts with evidence from RCTs, provide potential reasons for any differences, and note pertinent limitations in both types of evidence. Reviewers do not need to assess the publication bias domain for NRSIs because methods to detect this among NRSIs are less certain than among RCTs. However, NRSIs may be susceptible to publication and other reporting biases because NRSIs are usually not registered a priori. The Real World Evidence Registry is a new registry that attempts to address this problem.98
The 2013 guidance also recommends the consideration of three additional domains for NRSIs: dose-response relationship, magnitude of treatment effect, and potential confounding that could impact the observed treatment effect (see Table 3 of the 2013 EPC Methods Guide).97
10.6.2.3. Establishing an Overall Strength of Evidence
According to the 2013 guidance, evidence from NRSIs is generally assumed to suffer from a relatively higher risk of bias due to the lack of randomization and higher potential for confounding.97 Thus, an initial provisional grade of low strength of evidence is assigned to evidence from NRSIs. Reviewers may increase the grade to moderate strength of evidence (although rarely high) if the evidence from NRSIs is rated as low for the Study Limitations domain (based on study conduct or analysis) or after assessing the additional domains.
When both NRSI and RCT evidence exist, reviewers may combine those design-specific strength of evidence grades into one overall strength of evidence grade or rely on one study design if it clearly provides stronger evidence. In general, the guidance allows reviewers the flexibility of using varied approaches to incorporate multiple domains into an overall strength of evidence grade as long as the rationale is clear and consistent and adheres to the important general principles of AHRQ EPC methods guidance.97
10.6.3. Other Approaches to Grading the Strength of a Body of Evidence
In addition to GRADE and the AHRQ EPC approach, various systems have been used for specific health topics or settings (e.g., Strength of Recommendation Taxonomy [SORT] for primary care,99 Highest Attainable Standard of Evidence [HASTE] for HIV/AIDS,100 Let Evidence Guide Every New Decision [LEGEND] for point-of-care101). A review of these systems is beyond the scope of the current guidance.
10.7. Reporting NRSI Evidence
When reporting findings from a synthesis of evidence that involves NRSIs, reviewers should be cautious and provide sufficient context regarding the strengths and limitations of all included studies. In general, making causal inferences from NRSIs should be done cautiously. We suggest that, unless there is substantial confidence in the NRSI design and analytic methods, their results should be interpreted as associations between an intervention and outcome and not as effects of the intervention on the outcome. Although well-designed NRSIs with adequate analytic methods (including multivariable regression or any of the advanced methods discussed in Section 9) reduce the potential impact of confounding and may come close to emulating an RCT, reviewers may be unlikely to encounter advanced analytic methods for most topics. Moreover, specialized expertise may be required for carefully interpreting findings of advanced methods. However, such methods as multivariable regression may be more common and will often be adequate for control of confounding. These approaches rely on the assumption that the full set of confounders is known and validly measured.
In general, NRSIs and, if any, RCTs, should be reported together when reporting findings for a given outcome for a given Key Question. In doing so, evidence with lower risk of bias and greater applicability to the population of interest should be prioritized. Regardless of whether meta-analysis is conducted, it is important to report consistency of the findings (in terms of direction and magnitude of treatment effects) among NRSI designs and between NRSIs and RCTs. Where inconsistencies are detected, their likely sources should be explored and discussed. Reviewers should also describe the extent to which NRSIs may have used appropriate analytic methods to address confounders and other important threats to validity.
- Incorporating NRSIs in Systematic Reviews - Inclusion of Nonrandomized Studies o...Incorporating NRSIs in Systematic Reviews - Inclusion of Nonrandomized Studies of Interventions in Systematic Reviews of Intervention Effectiveness: An Update
Your browsing activity is empty.
Activity recording is turned off.
See more...