Included under terms of UK Non-commercial Government License.
NCBI Bookshelf. A service of the National Library of Medicine, National Institutes of Health.
Evans DG, Astley S, Stavrinos P, et al. Improvement in risk prediction, early detection and prevention of breast cancer in the NHS Breast Screening Programme and family history clinics: a dual cohort study. Southampton (UK): NIHR Journals Library; 2016 Aug. (Programme Grants for Applied Research, No. 4.11.)
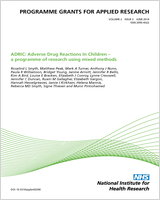
Improvement in risk prediction, early detection and prevention of breast cancer in the NHS Breast Screening Programme and family history clinics: a dual cohort study.
Show detailsThis section reports the aims, methods and results of three studies used to address the overarching research question outlined in the original proposal to understand the relative cost-effectiveness of using risk-based algorithms to inform the appropriate screening intervals in the NHSBSP. The early work in the programme was used to provide a mechanism (risk-based algorithm) for dividing a population of women into distinct categories that predicted their risk of breast cancer, which was then used to infer prespecified screening intervals to use as part of a risk-based national breast screening programme. The risk prediction studies, PROCAS and the study designed to assess the predictive value of new genetic variants (reported in Chapters 2, 3 and 4, respectively) informed the development of a risk-based algorithm which is used to select the appropriate screening interval for women participating in a national breast screening programme.
Aim
The focus of the cost-effectiveness work was to identify the relevant costs and patient benefits affected by using risk-based algorithms to inform the appropriate length of screening interval compared with the current NHSBSP.
Objectives
There were two initial objectives:
- 1.
to identify and critically appraise the existing economic evidence supporting the use of a national breast screening programme and individual screening modalities in the UK
- 2.
to identify the potential incremental costs and patient benefits associated with a risk-based screening programme as part of the NHSBSP and identify the expected cost-effectiveness.
At the request of external reviewers, a third objective was added:
- 3.
to quantify the impact of women attending the UK breast screening programme on their out-of-pocket expenses.
These three objectives were addressed using three distinct studies:
- 4.
systematic review of economic evaluations of breast screening programmes and screening modalities
- 5.
quantifying the impact of a national breast screening programme on women’s out-of-pocket expenses
- 6.
preliminary model-based cost-effectiveness analysis of a risk-based screening strategy for breast cancer as part of the NHSBSP.
The aim, methods and results from these three studies are now described.
Study 1: systematic review of economic evaluations of breast screening programmes and screening modalities
Background
A key stage in structuring an economic model, suitable to inform resource allocation decisions, is to identify and critically appraise existing published economic evaluations related to the intervention (breast screening programmes) of interest.
Aim
The primary aim is to identify published articles which have conducted an economic evaluation of breast cancer screening programmes and to summarise and critically appraise these studies.
Objectives of review
- Identify the number of published economic evaluations of breast cancer screening programmes.
- Identify the comparators, specifically if an option of no screening was evaluated and, if not, what screening intervention comparator was used.
- Identify the interventions evaluated and classify according to screening modality, age range and screening interval and studies which have examined risk-based screening interventions.
- Classify the evaluations as either trial or model based.
Methods
A systematic review was conducted of economic evaluations relevant to breast screening in a general population of women and published in peer-reviewed journals.
Literature search
The electronic databases Ovid MEDLINE, Ovid EMBASE and NHS Economic Evaluations Database (accessed via the Centre for Reviews and Dissemination databases) were systematically searched to identify economic evaluations published in peer-reviewed journals. Electronic search strategies were designed for each respective database and combined index and free-text terms for breast cancer screening and associated screening modalities (e.g. mammography) with the Centre for Reviews and Dissemination economics filter (see Appendices 2–4). In addition, the reference lists of relevant studies were hand-searched to identify further studies meeting the inclusion criteria. All searches were performed on 30 January 2014.
Inclusion criteria and study selection for critical review
Two reviewers (IJ and EG) independently screened all retrieved titles and abstracts to identify the studies eligible for inclusion in the critical review. Studies were included if they met the inclusion criteria summarised in Table 66.
TABLE 66
Criteria for inclusion in the critical review
Data collection and extraction
Data were extracted from the identified studies by two reviewers (Sean Gavan and KP) using a structured data collection form. The de novo data extraction form was developed based on the CHEERS (Consolidated Health Economic Evaluation Reporting Standards)237 checklist and supplemented by criteria suggested by Karnon et al.238
The data extracted encompassed:
- viewpoint – societal/health system/health service/payer
- type of alternative (technology/screening programme/screening interval)
- comparator (current screening programme or no screening)
- study population (eligible age)
- type of evaluation (model or trial based)
- model type: decision tree/Markov model
- whether or not calibration was performed
- whether or not model validation was performed
- uncertainty analysis included (one way and/or probabilistic)
- method used to estimate effectiveness and data source (meta-analysis/RCT/cohort study/published studies)
- whether or not screening was linked to treatment
- primary measure of benefit (clinical effectiveness/life-years/QALYs)
- resources included
- price year (currency)
- time horizon (discount rate)
- incremental cost-effectiveness ratio results
- key parameters driving cost-effectiveness.
Results were tabulated and then summarised in a narrative synthesis.
Results
This section reports the main findings from the systematic review of published economic evaluations of breast screening programmes.
Study identification
Figure 41 summarises the study identification and inclusion process. The search of electronic databases identified 2959 published studies, which were screened for eligibility. A total of 71 studies were identified and included in the review. A further 15 studies may be added at a later date if the hard copies of the manuscripts can be sourced.

FIGURE 41
Study identification and inclusion. BSP, breast screening programme.
Table 67 provides an overview of these studies. The full data extraction table is available from the authors on request. Three other key modelling studies were identified: Tan et al.,310 the Wisconsin simulation model311 and Breastscreen Australia Evaluation (2009).312 Tan et al.310 developed a Markov model to characterise breast cancer progression but did not attach cost data, and this study is not, therefore, an economic evaluation. The Wisconsin model311 was developed as part of the National Cancer Institute Cancer Intervention and Surveillance Modelling Network. It is a patient-level simulation model, continually updated, that uses data from Wisconsin State, USA. The model simulates breast cancer in a population over time, generating cancer registry-like data sets. The model uses parametric input assumptions about natural history, screening and treatment, and can be used to evaluate the relative cost-effectiveness of different breast screening-related policies. The Australian cost-effectiveness analysis312 compared the current BreastScreen Australia programme and various alternative screening scenarios with a hypothetical no-screening scenario using a Markov model. BreastScreen Australia actively targets all women aged 50–69 years; however, women aged 40–49 years and > 70 years are also eligible to attend. The model consists of two separate components: the natural history of disease component and the BreastScreen Australia screening pathway. These models were not included in the review as they were not published in a peer-reviewed journal, but they are mentioned here as they were subsequently used to inform the final model structure.
TABLE 67
Summary of included studies
Study overview
There were a total of 71 economic evaluations included in the review. Of these, 52 were model-based evaluations, 10 were retrospective cohort-based evaluations, six were prospective cohort-evaluations and three were trial-based evaluations. The majority (n = 69) conducted cost-effectiveness analysis and there were two cost–benefit analyses.
The review covered studies up to the end of December 2013 and the first study277 was published in 1979. The analyses were based in a number of countries including the USA (n = 18239,257,263,267,271,272,274,275,277,278,283,289–293,295,298), the UK (n = 15241,243,246,247,249,253,261,265,273,276,279,287,297,299,303), the Netherlands (n = 4242,244,256,259), Hong Kong (n = 4304–307), Australia (n = 3254,262,302), Italy (n = 3245,251,252), Japan (n = 2284,286), Korea (n = 2266,268), Finland (n = 2269,270), Spain (n = 2250,258) and Switzerland (n = 2255,280); and there was one study in each of France,240 Brazil,294 Ghana,308 Germany,303 New Zealand,296 Vietnam,281 Peru,309 Norway,282 Slovenia,288 Canada,264 India285 and Denmark.260 One study300 compared programmes across four countries (the UK, the Netherlands, Spain and France) and one study248 compared programmes across two countries (the USA and Sweden).
The number of different interventions and varied jurisdictions for the analyses limits the comparability between the programmes and ability to provide an overall statement of relative cost-effectiveness owing to issues with generalisability.313 Van Ineveld et al.300 explicitly concluded, in their comparison of breast screening programmes for four European countries (the UK, France, Spain and the Netherlands), that ‘no uniform policy recommendations for breast cancer screening can be made for all countries in the European Community’. In terms of the remaining 15 analyses that focused on the UK, eight studies concluded that the programme or technology was potentially cost-effective but that more robust evidence was needed before a definitive conclusion could be reached,243,249,254,287,299 five studies suggested an optimal programme based on relative cost-effectiveness242,246,247,279,301 and two studies, both evaluating computer-aided detection, concluded that the technology was not likely to be a cost-effective use of resources but that more evidence was needed to support this conclusion.292,298
Interventions and comparators
A number of interventions were evaluated in the identified studies: introducing a screening programme using mammography; using a mobile screening unit; different screening programmes that may vary by screening modality, screening interval or screening age; screening interval; screening age; and new technologies (two-view reading, double reading, computer-aided detection, digital mammography, ultrasonography). Some studies included multiple interventions for evaluation and hence reported a range of strategies. One study309 reported that 94 strategies were evaluated. Two studies248,300 compared screening programmes across countries. Three studies explicitly covered some form of stratified screening strategy (risk and/or density based).292,298,299
A study had to state that a comparator was included in the analysis to be defined as an economic evaluation and hence to be eligible for the review. However, on some occasions, a comparator, although alluded to, was not reported explicitly. On the occasions when a comparator was reported explicitly, those used were no screening, opportunistic screening and the existing screening programme or technology (in terms of modality, screening interval and screening age).
Study population
The age range of the women included in the eligible study population depended on the country base for the analysis and the screening programme under evaluation. A number of studies specifically evaluated the choice of age range for the screening programme in terms of the impact on the relative costs and outcomes. Not every study reported the eligible age range for the screening programme. The earliest reported starting age for a screening programme that was evaluated was 30 years (Japan) and the oldest included age was 85 years (UK), with one study evaluating lifetime screening (USA).
The majority of the modelling studies described pre-clinical disease incidence and disease progression rates, which were sourced from longitudinal observational databases. For some studies, historical incidence data for breast cancer were incorporated into the model because either opportunistic screening or a population-based screening programme was already available in the study setting.
Validation and calibration
Validation and calibration of decision-analytic and mathematical models of screening interventions are important aspects to include in the design of a robust model-based economic analysis. Validation has always been recognised to be a key component of developing a model structure which accurately reflects the stated decision problem.314 In contrast, calibration emerged into recognition as a key component around 2010 onwards.315 However, owing to word constraints set by journal article lengths, the process of model validation or calibration is not generally reported in detail.
Three studies described a method of model validation. de Koning et al.256 compare the predicted results with the actual results from the first 7500 women screened in the Netherlands in 1989. The study presents six model outputs: attendance rate, positive screens, biopsy, breast cancer detection rate, positive predictive value, and malignant diagnosis with biopsy and lymph node metastases. The predicted estimates from the model match very closely those observed. However, it is worth noting that the outputs chosen for validation represent a short time period. The performance of the model, in terms of long-term predictions, cannot be ascertained. Both Schousboe et al.292 and the Australian Department of Health Review (not included in the review as not in a peer-reviewed journal) compare the predicted age-adjusted breast cancer incidence and mortality data for their respective countries.
Stout et al.295 was the only identified study that explicitly described a process of model calibration for unobservable input parameters. The study utilised breast cancer incidence data from the USA to calibrate a lag time parameter representing the period between the start of biological growth and the detection of a tumour. In addition, the model parameter determining the growth rate of a tumour is also calibrated. In furthering the robustness of their model, once calibrated the model outputs were validated using cancer registry data. This study remains the only robust description of modelling calibration and validation for any of the studies identified.
Overview of key model-based evaluations
There were 52 model-based evaluations. The most commonly reproduced model structure was the Microsimulation Screening Analysis (MISCAN) model, which was used in nine studies. The MISCAN model was applied in a range of country settings outside the Netherlands, for which it was originally designed, but in all cases these models still relied on Dutch data to populate key aspects of the model structure. The MISCAN model is not publicly available. Therefore, a range of model types including decision trees, Markov state transition models, individual patient-level simulation models and mathematical models were used in the studies identified. In general, each individual study developed a de novo model relevant to a specific research question.
Overview of evaluations of stratified screening strategies
Three studies explicitly stated that they conducted a model-based analysis of a stratified screening strategy.292,298,299 Two of these studies were based in the USA292,298 and one was UK based.299 These studies are now described with their key findings and relevance to the UK setting.
Tosteson298 used a discrete event simulation (DES) model with a lifetime horizon, taking a societal and Medicare perspective relevant to the US setting. The model was the Wisconsin breast cancer epidemiology simulation model.311 All-digital mammography was compared with targeted mammography interventions. Mammography screening was targeted using different strategies: age (women < 50 years); and age and density targeted (women < 50 years or women ≥ 50 years with dense breasts). The model structure and data sources were relevant to US women, which limits the relevance of the findings of this study to the UK setting. The authors concluded that all-digital was not a cost-effective option when compared with film mammography. Age-targeted mammography was identified to be a relatively cost-effective use of resources but density-targeted mammography was of uncertain added value, especially in older women (those aged ≥ 65 years). The findings were sensitive to a number of assumptions but, most importantly, the accuracy of the screening modality in older women with non-dense breasts.
Schousboe et al.292 used a five-state Markov model with a lifetime horizon, taking the US payers’ perspective and using data from a US population of women. The study perspective and source of data limit the relevance of the findings from this study to the UK setting. The Markov model was also overly simplistic in terms of capturing the potentially relevant health states and pathways of care likely to be influenced by a stratified screening programme, and was not viewed as a potentially useful starting point for the model-based analysis proposed as part of this programme. This study did not define a single stratified screening intervention per se but rather used observational data to identify what factors influenced relative cost-effectiveness of a breast screening programme. The authors concluded that mammography screening (offered every 3–4 years, biennially or annually) should be stratified based on key criteria: woman’s age; breast density (defined using BI-RADS categories 1–4); history of breast biopsy; family history of breast cancer; and beliefs about the potential benefits and harms of screening. The findings were sensitive to the cost of digital mammography and the assumed prevalence of dense breasts in a population.
Van Dyck et al.299 used four ‘what-if’ scenarios structured around pathways depicted by decision trees and populated with data from the UK population. The study perspective, time horizon, nature and source of model inputs or method of analysis were not reported in the main body of the text. Supplementary materials were provided but did not give additional information, except that reference was made to using a Markov model alongside the decision tree reported in the main paper. The interventions proposed involved stratifying women aged ≥ 50 years by family history and genetic testing using electronic health records, a SNP-based test [BRCA1, BRCA2, TP53, FGFR2, TNRCP, MAP3K1, LSP1, CHECK2, gene(ATM), BRIP1, PALB2] and a breast cancer risk calculator (it was not stated explicitly which one was used). These interventions were combined and used pre screen to create two risk profiles (high and low, but risk levels were not reported). The estimated incremental costs and benefits were not reported and the key drivers of relative cost-effectiveness were not stated. The data inputs and method of analysis were not reported sufficiently for this study to provide a useful starting point for any subsequent analysis.
Discussion
The main aim of an economic evaluation is to examine the available evidence on the costs and effectiveness of health technologies in order to provide decision-makers with the relevant information to assess the technology’s ability to provide value for money to the payer. However, this may require information to be gathered from outside the traditional evidence base of a randomised controlled trial (RCT).316 For example, trial evidence may not examine all the relevant comparators or it may have a short study period which does not examine long-term costs or effects. Models have been exploited in economic evaluation as a means to synthesise the relevant sources of information that are available, extrapolate results into the future and provide estimates of costs and effects in the absence of direct observation.
Broadly, four classifications of modelling approaches are described in the breast screening literature: decision trees, state-transition, mathematical and DES. The MISCAN model was developed by van Oortmarssen et al.317 and is widely reported in the literature. The model is a specifically developed computer simulation package to, first, assess the clinical impact of different screening policies. The model has also been applied to prostate and colorectal cancer screening programmes.
The MISCAN model structure was used as an individual patient sampling model in nine of the identified studies. The natural history of this model is defined in terms of the size of the tumour, where distinction is made between pre-clinical, clinical and screen-detected cancers. This follows closely the general modelling approach for screening programmes presented by Karnon et al.238 The advantage of this modelling approach is that it allows a patient pathway to vary between those who attend screening and those who do not and, where appropriate, account for differences in the patient characteristics, such as disease progression and incidence. Movement from one phase to another is also determined by the treatment an individual is assumed to receive based on the characteristics of the tumour in terms of size.
In contrast, some models, such as the ones used by Carles et al.250 and Salzmann et al.,291 present comparatively simple four-state model structures. Both of the studies fail to explicitly describe and justify the model structures presented. It is questionable if these structures capture the underlying essentials of the breast disease process and screening interventions. Madan et al.273 provided a unique approach in their model of the addition of an extra screening round to the English NHSBSP. Although the authors do not explicitly state the model type, it can be inferred from the model structure that a decision-tree-type model is used, representing the only identified study to take such an approach. The premise of their study is to provide a ‘rapid-response’ economic analysis and argue that decision-makers may be willing to trade-off precision of results estimates for a faster turnaround time with producing and analysing a model.
None of the identified studies described the process of defining the model structure. The process for defining a model structure as described by Karnon et al.238 may be considered as a second-order issue when presenting higher-level methods and results. If this is the case, and combined with limited amount of space in which studies are reported in academic journals, it is perhaps understandable that this feature is not included in the identified studies. This may also be because some of the models reported were ‘borrowed’ from previous studies and directly applied to the current research question. Therefore, the authors were not involved in the construction phase of the model and hence were not able to define the process of defining the model structure. All of the studies fail to report a justification for the choice of modelling technique employed. Again, this may be a result of the limited space available and the relative importance authors place on including this justification, compared with presenting the detail of the results from a model.
Concluding remarks
This review has identified a substantial number of published economic evaluations relevant to breast screening programmes, the technologies used and modifications to the programme in terms of screening interval and age groups appropriate for screening. None of the identified 52 models provides an ‘off-the-shelf’ model that is fit for the purpose of answering the research question posed by PROCAS. Therefore, it will be necessary to construct a de novo model. The process suggested by Karnon et al.238 will prove useful to inform the general concepts of how to model in the context of breast screening. The MISCAN model cannot be used directly as it is not publicly available, but will inform the approach taken to including cumulative incidence and unobserved parameters. This approach will be supplemented by the modelling proposed by Tan et al.,220 a study which, although not an economic evaluation, provides some useful information.
Study 2: quantifying the impact of a national breast screening programme on women’s out-of-pocket expenses
Background
In the UK, guidance published by NICE41 recommends using the NHS and social services perspective in economic evaluations. The NHS perspective was, therefore, suggested as the relevant viewpoint for the proposed economic evaluation of the risk-stratified breast screening programme. Some published economic evaluations of mammography-based breast screening programmes, such as the one by Brown et al.,246 have used a broader perspective to include the costs incurred by women attending the screening in the UK. The study by Brown et al. used a survey-based approach to identify and quantify the travel and time costs for the women to attend the screening van. The study identified costs for a sample of 132 women who attended screening in 1992 and found that the mean private cost of assessment was £43.53 (SD £30.29), which included a mean travel cost of £10.74 and mean time cost of £32.75. Knowledge of this study might have prompted reviewers of the original PROCAS proposal to suggest that the study design also include an evaluation of the private assessment costs incurred by the women.
Aim
To identify the private assessment cost incurred by women when attending a breast screening programme.
Study objectives
This study has two objectives:
- to estimate the distance travelled by women attending the NHSBSP van
- to identify the self-reported distance travelled and time taken to attend the NHSBSP van.
Method
This study used two approaches to estimate and identify distance travelled and time to attend the screen: (1) survey-based self-report and (2) geographical distance calculation. The methods for these two approaches are now described.
Survey of PROCAS participants
An online survey (available from Ian Jacob on request) was used to identify PROCAS participants’ self-reported travel and time costs by focusing on four key aspects related to attending the breast screening appointment: the journey to the breast screening centre; the time at the breast screening centre; whether or not they had any companions for their visit; and whether or not they were responsible for caring for children or older people at home. The survey was piloted in a sample of women to check the wording of the questions. The final survey was administered using the QUALTRICS online survey tool (www.qualtrics.com).
Sampling frame
This aspect of the study used a stratified purposive sampling approach. At the time of invitation (February 2014), the PROCAS study sample was 53,605. From this eligible sample, women who had attended their screening mammogram within the previous 6 months were identified as potentially eligible survey respondents. Respondents who had died or received a diagnosis of breast cancer were excluded from the eligible sample, which gave a potential sample size of 51,540, of whom 2350 had attended for screening in the last 6 months. The aim was to target a representative sample from each of the 14 active screening sites, and the final target sample size was 600 owing to financial constraints. Not all screening locations are active in the Greater Manchester area at the same time, and 14 screening sites were active at the time of the survey. A weighted sample by number of participants in each of these sites was asked to complete the survey (n = 594). An invitation to participate in the online survey was posted to each of these identified participants. No reminder was sent.
Table 68 shows the total number of women who were eligible for screening, the number recruited to the PROCAS study and the number invited to participate in the survey by screening van location.
TABLE 68
Number of women taking part in PROCAS and invited to take part in the survey
Geographical distance calculation
This method uses data collected from PROCAS study participants’ stated home addresses with postcodes and the known postcode location of the relevant NHSBSP van. Figure 42 shows the location of the screening van sites in Manchester.

FIGURE 42
Screening van sites in Manchester.
The distance between these two postcode-location points was then calculated using Google Maps (Google Inc., Mountain View, CA, USA) and the code ‘ggmap’ in the statistical software package R (The R Foundation for Statistical Computing, Vienna, Austria). The distance calculation involved estimating the distance from the home postcode location to the screening site postcode.
Sampling frame
This aspect of the study used the addresses of all PROCAS participants who attended the van for a screening visit recruited up to March 2013. This equated to a sample size of 50,016 women.
Results
This section reports the results from the two approaches used to generate estimates of travel distance for the study participants to the screening van and the time taken to attend screening.
Survey of PROCAS participants
A total of 175 respondents started to complete the survey and are included in the analysis, which represents a response rate of 29% (175/594). Two of these women stated that they were eligible for free public transport.
Table 69 summarises the responses to the questions included in the survey to identify out-of-pocket expenses.
TABLE 69
Summary of responses to survey on out-of-pocket expenses
The majority (75%, n = 131) of women travelled to the screening van from home and the most common mode of travel was car (81%, n = 141). Twenty-nine per cent (n = 51) did not return to their original starting location after their visit to the screening van but travelled on to a different location, for example they returned to work or went shopping.
Eleven (6%) women reported paying a fare to travel to the screening van, which was a mean of £4.00 (range £2.10 to £8.00).
The mean time taken to travel to the screening van was 18 minutes (range 1–90 minutes). One woman did not answer this question.
The mean distance travelled to the screening van was 4.08 miles (range 0–17 miles). Seven of the women reported a travel distance of zero miles.
The mean time that women reported spending at the screening van, including waiting and screening time, was 36 minutes (range 10–240 minutes). Two women did not answer this question.
The majority of women (61%, n = 106) reported that they would have been at work had they not been at the screening van. For the women who reported taking time off work, the mean reported time away from work was 2 hours and 23 minutes (range 3 minutes to 8 hours and 30 minutes). Four women did not answer this question. Seven per cent of women (n = 12) reported that they had lost earnings as a result of being away from work.
Some women (17%, n = 29) reported they had a companion with them when they visited the screening van. Just one woman reported that this person accompanied them because of their poor health. A very small percentage of women (2%, n = 4) needed to get someone to look after children/dependants while they were at the screening visit, which took a mean of 1 hour and 55 minutes (range 40 minutes to 4 hours). No one had to pay for this care.
A small percentage of women, 13% (n = 22), reported that they needed to pay other costs while attending the screening visit. Two women did not answer this question. The reported additional expense was parking, with the exception of one woman who reported paying for petrol. The mean reported additional cost was £2.83 (range £1.20 to £5.00).
Attaching unit costs
The cost of women’s time was estimated using the median gross weekly earnings for women (including full-time and part-time workers) for 2013, which is £327.5318 for an assumed 37-hour week. This equated to a cost of £2.66 (range 15 pence to £13.28) for travel time and £5.31 (range £1.48 to £35.41) for screening time.
Using the government-advised mileage rate for a 2000cc petrol car, which is 24 pence per mile,319 the distance travelled equates to 98 pence (range £0 to £4.08).
Geographical distance calculation
This study used data for 50,016 women recruited to PROCAS. The mean estimated distance travelled was 1.48 miles, which would take a mean of 5.57 minutes to travel.
Discussion
This study has identified relatively low travel costs and travel time for women to attend the breast screening programme, which is not surprising given that the service is available in screening vans close to women’s homes. There was a large variation in the reported time it took for women to be screened, including the waiting time.
There is no agreed method for estimating patients’ assessment costs for attending a screening programme. The two approaches used here are not likely to produce the same estimates of travel distance because the self-report survey asked women to use the actual starting location on the day they attended the screening van. However, the geographical distance travelled method assumed that the women travelled from their home to the screening van.
Limitations
This study has a number of limitations. The self-report survey was limited by the relatively low response rate and subsequent small sample size. However, this sample size was still larger than the sample used in the study previously reported by Brown et al.246 The survey could also have been biased as a result of the use of self-reporting and relying on reliable recall of the actual day of visiting the screening van. We tried to minimise the impact of poor recall by asking women to complete the survey within 6 months of their screening visit.
The screening van locations rotate around the Greater Manchester area, and hence it was not possible to sample from every location in the area. We also had to limit the sampling frame to exclude women without a subsequent diagnosis of cancer. The potential bias from limiting the sampling frame in these two ways is not known.
Concluding remarks
This study has shown that it was valid to exclude patient assessment costs from the eocnomic evaluation and use the NHS perspective for identifying and quantifying resource use and costs.
Study 3: preliminary model-based cost-effectiveness analysis of a risk-based screening strategy for breast cancer as part of the NHS Breast Screening Programme
Background
There is still considerable uncertainty about who should receive mammographic screening, at what age and at what interval. When it was first introduced, the existing NHSBSP was informed by economic evidence.320 In theory, any changes to existing programmes should also be informed by economic evidence, as modifications are likely to alter the balance of relative costs and patient benefits. The risk-based screening programme developed as part of PROCAS will ideally save money by concentrating resources where they are needed most while also avoiding unnecessary screening in women at low risk of developing cancer. It is, however, necessary to identify the incremental costs and benefits associated with the proposed risk-based screening programme. Generating this evidence base will help decision-makers to understand what factors will help to facilitate an effective use of the finite health-care budget allocated to the provision of a national screening programme.
Owing to the timing of the work in the programme grant, the proposed risk-based screening intervention developed as part of PROCAS was still in its initial stages when the work on the economic modelling was started. It was decided to develop a preliminary economic model, rather than produce a definitive model, to identify the potentially relevant costs and patient benefits for a risk-based strategy compared with the existing screening programme. The draft model would be structured in such a way to allow it to be repopulated as and when data from the PROCAS cohort become available over time.
Aim
This study aimed to identify the incremental costs and patient benefits associated with using risk-based screening strategies for breast cancer.
Method
A preliminary model-based cost-effectiveness analysis was conducted. A systematic review did not identify any relevant model-based evaluations. Therefore, a de novo decision-analytic model, taking the NHS perspective over a lifetime horizon, was structured to compare risk-based breast screening with the current screening programme. The risk stratification was assumed to be provided using a risk prediction algorithm (developed during projects 1 and 2).
Model structure
A range of mathematical and simulation modelling techniques has been used to evaluate breast cancer screening policies. These models include simple decision trees, cohort Markov models and more complex modelling approaches that aim to overcome the Markovian assumption of exponential transition rates between health states. These decision-analytic models provide a structure for ordering and synthesising information from a wide range of sources, which may include primary and secondary data analysis alongside less robust evidence such as expert opinion. The ultimate aim of which is to explore the impacts of screening policies in terms of effectiveness (incidence and mortality) and costs over the lifetime of a screening cohort.
Before constructing a model, it is important to be clear about the nature of the problem and define the objectives, scope and context of the study. Table 70 describes the objectives and scope of this preliminary model-based evaluation.
TABLE 70
Objectives and scope of the model
Model approach
The selected modelling approach used in this preliminary analysis was informed by a review of existing model-based evaluations of breast screening programmes and also current methods and approaches informed by the more general literature on how to conduct economic analyses of cancer screening programmes. A general cancer screening model structure, as presented by Karnon et al.,238 formed the framework for identifying the relevant modelling structure and approach (Figure 43). In this description there is a distinction made between the model structure and the modelling approaches (or techniques) used to inform the final structure described in the following section. The framework proposed by Karnon et al. defined two broad cancer states, non-invasive and invasive, which interact with screening. Moving from this general framework to a breast cancer-specific model requires representation of disease progression. Karnon et al. also suggested natural history of disease modelling as the preferred analytic approach, where the disease is described from the point of progression, at which it becomes detectable, and subsequent death. It was argued that this approach permits the evaluation of screening options that have not been observed in primary research where only estimates of test sensitivity and specificity are required to assess these options. The systematic review (see study 1) identified two broad approaches when representing the natural history of breast cancer: (1) tumour size and (2) tumour stage.

FIGURE 43
General cancer screening model (Karnon et al.).
Several modelling approaches (or techniques) are available to evaluate breast cancer screening. Broadly, three classifications of models are described in the general modelling literature including decision trees, state-transition models (Markov) and individual sampling models (DES). In reviewing modelling studies of screening programmes, Karnon et al.238 also distinguished an additional classification of ‘complex mathematical models’. Decision tree models are not useful as a single approach in this context, as they are overly simplistic and restrictive when evaluating screening programmes, but they may be combined with other structures to represent the complexity of the process being modelled.
The cohort Markov model is the most commonly employed technique when modelling the economic impact of health-care interventions over time. This also appears to be the most common approach taken when examining screening programmes. This is a state-transition approach that requires the definition and representation of disease and screening programme in terms of mutually exclusive (health) states. The time horizon of the model is split into cycles of equal length, at the end of which a cohort of individuals may move to another health state and remain in the same state. This process continues until the entire cohort reaches an absorbing state (e.g. death). Movement between these states is determined by transition probabilities, which are conditional on the current health state but may also vary according to the overall time in the model (Figure 44).

FIGURE 44
An example of a state-transition model.
Individual event-based models provide an alternative and perhaps more flexible modelling technique, an example of which is DES. This type of model asks what and when is the next event for the participant at the point in which they experience their current event. This approach is distinguished from a state-transition model in which this question is asked at regularly occurring intervals. The participants in a DES model structure can retain a memory of previous events and individual characteristics which then can influence their pathway through the model. Barton et al.292 catalogue four different DES modelling techniques which can be used to determine which event occurs and the timing of the events and discuss the advantages and disadvantages of (1) the time-slice approach; (2) determining first event and then time; (3) determining first time and then event; and (4) sampling times for each possible event and taking the minimum.
State-transition models require transition probabilities between each of the allowed subsequent states. For example, the rate of transition between DCIS to clinically invasive breast cancer per cycle length or the transition probability of ‘other cause mortality’ (from any health state), which may be age dependent. This is distinguished from time-to-event parameters in an event-based model, which are typically derived from fitting parametric survival curves to observed data (e.g. Kaplan–Meier curves).
The natural history of breast cancer can be described in more complex mathematical models, such as those presented by Baker241 and updates to the family of MISCAN models.317 Using this method, the authors describe input parameters as continuous variables that change over time, the parameters of which are specified in mathematical functions which may be solved either analytically or numerically (by simulation). The type of data required by these more complex models are similar to those of event-based models, but are generally used in order to better describe the pre-clinical phases of the disease.
Evaluating an individual risk-based screening policy means that there is a need to incorporate an element of within-cohort heterogeneity in terms of breast cancer risk and also to understand how to set the defined screening intervals based on estimated risk. Increased risk for subgroups of the cohort would, in turn, mean that each defined risk category faces differing breast cancer rates. Previously published models assumed that all women face the same risk of developing disease; incorporating heterogenity based on risk in other model structures would result in cumbersome structures. The MISCAN model may have proved a useful starting point for this preliminary economic analysis of a risk stratified screening programme. However, three factors meant that using the MISCAN model was not practical or feasible. The MISCAN model is not publicy available and would have required collaboration with the model developers. This was explored but did not prove to be a practical solution. The MISCAN model is populated from US data (the Surveilance, Epidemiology and End Results program), which makes it of limited direct relevance to the UK population without further intepretation of the relevance of these data. In addition, the MISCAN model would have still required extensive modification to make it relevant to evaluate a risk-based screening strategy for the UK context.
A de novo model was, therefore, developed [hereafter termed the Manchester Breast Screening Model (MBSM)]. The MBSM developed for this takes into account the advantages and disadvantages of each published model type (see the systematic review in study 1) and the policy question under evaluation. The MSBM needed to be able to incorporate four components:
- a decision-tree based structure to represent the choice between a risk-based screening programme and current practice (Figure 45)
- a DES to represent the care pathway in the screening programmes (Figure 46; box C)
- a state-transition structure to represent development of a tumour (see Figure 46; boxes A and B)
- a survival analysis approach to represent subsequent progression to death.

FIGURE 45
The intervention and comparator pathways included in the MBSM.

FIGURE 46
The MBSM.
Table 71 summarises the key events represented in the model.
TABLE 71
Events in the MBSM
Figure 45 also illustrates how the risk categories informed by the PROCAS-developed risk-based algorithm were linked with the relevant defined screening interval. There were no published data on the relevant risk categories or associated screening interval. Therefore, expert judgement was used to define the relevant risk categories identified in PROCAS and the suggested relevant screening interval. Two clinicians (a geneticist and a breast oncologist) and an imaging scientist provided their expert opinion collectively to produce the six risk categories and screening intervals.
The face validity of the MBSM was confirmed using input from all members of the programme grant research team and also supplemented with expert opion elicited during a separate 1-day (December 2013) workshop (funded by Genesis). The expert workshop was attended by 12 experts representing academics, clinicians and imaging scientists with an interest in breast screening programmes. This included individuals from the national screening committees and academics with previous experience of evaluating screening programmes.
Informing model input parameters
The approaches to informing the model input parameters are now described.
Ten-year and lifetime risk of invasive breast cancer
Incorporating risk of being diagnosed with breast cancer is a key parameter input. There were no published data reporting the proportion of women falling into each risk category (10-year or lifetime) following screening. The estimated 10-year risk was taken from the application of the IBIS-I breast cancer risk evaluation tool11 for the PROCAS study population who were aged 50 years at the time of enrolment (Figure 47). The x-axis on Figure 47 shows the estimated probability associated with each risk category. This was the assumed age at which their risk of breast cancer was estimated. The average 10-year risk is lower for this sample age when compared with the entire PROCAS study population which comprised a range of ages (Table 72).

FIGURE 47
Empirical distribution of estimated 10-year breast cancer risk.
TABLE 72
Description of estimated 10-year risk of developing breast cancer
To incorporate individual risk into the MBSM, the estimated 10-year risk for each individual was adjusted to reflect lifetime risk. Owing to lack of data, it was necessary to assume that the RR at 10 years for each individual in comparison with the average population risk as estimated by the IBIS-I tool remained constant throughout the lifetime of an individual.
A range of parametric distributions were fitted to the adjusted lifetime risk of the PROCAS study population, the aim of which was to identify the most appropriate distribution, and its associated parameters, which we could use as a direct input parameter.
Two goodness-of-fit statistics were used to select the most appropriate distribution. The Kolmogorov–Smirnov test measures the fitted cumulative distribution to the input data and is calculated as the largest absolute difference between the cumulative distributions for the input data and fitted distribution. A small p-value indicated that the sample is highly unlikely, and, therefore, the fit should be rejected. Conversely, a high p-value indicated the sample is very likely and would be repeated and, therefore, the fit should not be rejected. The Anderson–Darling statistics can be interpreted in the same way. The best-fitting distribution is selected based on the highest estimated value. For the observed data, the Pearson type V distribution was identified as having the highest p-value and, therefore, this distribution was selected for the model (Table 73).
TABLE 73
Goodness-of-fit statistics for lifetime risk
For comparative purposes, the worst (Weibull) and best (Pearson type V) distributions for the probability density function, cumulative density function, quantile–quantile plot, probability–probability plot and box plots are shown in Appendix 5.
As part of the goodness-of-fit procedure, the estimated parameters for the Pearson type V distribution were estimated, which were then used in the model.
Incidence of invasive and in situ breast cancer
The incidences of invasive (ICD-1065 C50) and in situ (ICD-1065 D05) breast cancer were obtained from the Office for National Statistics for the years 2009–11.321 These rates represent observed breast cancer dignosis incorporating the effectiveness of the current univeral screening programme (3-year interval, age range 47–73 years). An empirical distribution was formed (Figure 48), representing the age at which a diagnosis is likely to occur. The combined incidence rates for both invasive and in situ carcinoma were used to provide a single age at which clinical diagnosis will occur. This allows specification of the type of DCIS (indolent or aggressive), taking account of the assumption in the model structure that all breast cancer tumours onset in a DCIS phase.

FIGURE 48
Incidence of invasive and in situ breast cancer UK.
In situ carcinoma and invasive carcinoma
To reflect that a certain number of in situ carcinomas (DCIS) will never progress to invasive disease, two types of DCIS, indolent and aggressive, were defined. It was assumed that indolent DCIS will never progress but aggressive DCIS will progress through a defined natural history model. The probability of aggressive DCIS was informed by Tan et al.310
Breast cancer stage
Breast cancer stage was based on the tumour node metastasis (TNM) staging system and matched the tumour size at diagnosis to stage.
Onset lag period
Current breast cancer incidence represents the age at which diagnosis occurs. However, it is not possible to directly observe the age at which the tumour is initiated in an individual. In the model, a tumour onset lag parameter was defined to adjust observed breast cancer incidence to reflect the onset of a tumour. This parameter was informed from a published breast cancer natural history model.322 To reflect the uncertainty with this variable, a uniform distribution was assumed based on the reported range for the estimated lag period.
Tumour growth
The rates of growth of preclinical cancers is also an unobservable parameter. The approach presented in Tan et al.310 was taken to estimate this parameter in which tumour growth is defined as a series of events classified by the size in millimeters. Each of the sojourn times was taken from Tan et al.310 and used to estimate individual growth rates for each participant. Each sojourn time was assumed to follow an exponential distribution.
Mortality
The model incorporates two events for mortality: death due to other causes and death due to breast cancer. The probability of dying from other causes was modelled as an age-dependent probability distribution which was informed by Office for National Statistics death registrations for England and Wales by single year of age for females.323 To reflect more recent mortality rates, we use the period 2003–12. These data were used to construct an empirical distribution which allows the sampling of age-conditional rates. Figure 49 shows the comparison between all-cause mortality for the entire period (1963–2012; see black line) and the period used to inform our model (green line). Figure 50 shows the same comparison of data plotted as a cumulative probability mortality distribution.

FIGURE 49
Smoothed empirical probability mortality distribution.

FIGURE 50
Smoothed empirical cumulative probability mortality distribution.
The relative survival of screening participants who are subsequently diagnosed with breast cancer based on tumour diameter was modelled, using data reported by the National Cancer Intelligence Network;324 these data are matched to the tumour size at diagnosis within the model (Table 74).
TABLE 74
Relative survival following treatment for breast cancer
Mammography screening test performance
The performance of mammography screening is defined in terms of sensitivity and specificity. The sensitivity of mammography screening depends on the size of the tumour (Table 75). This parameter was informed by the study by Tan et al.310
TABLE 75
Mammography screening test performance
Costs associated with the screening, diagnosis and treatment of breast cancer in the UK
The associated costs for screening were estimated from the reported programme cost from the NHSBSP. The programme screened 1.94 million women aged ≥ 45 years, which equated to approximately £49 per woman screened. However, the year for these cost data was not reported in the original data source. Once a diagnosis of breast cancer has occurred, it is classified according to TNM stage. The UK relevant costs by stage were taken from Dolan et al.325 and inflated to current prices. These were the only available data and, ideally, more recent treatment costs would make the estimates more up to date.
Health-related quality of life
To simplify the calculation of QALYs between events in the model, age-dependent utility values were not incorporated. Instead, an average breast cancer free utility value between the ages of 45 and 100 years was defined as 0.79. This was informed by age-specific UK general population utility values, which came from the EQ-5D.326
Utility values associated with breast cancer were informed by a published literature review and synthesised meta-analysis.327 The review defined utilities in terms of early breast cancer and metastatic breast cancer. These were then matched based on the stage at which the breast cancer is diagnosed (Table 76). Peasgood et al.327 identified a large number of utility values. A total of 13 databases were searched, and 49 papers providing 476 unique utility values were identified. A variety of methods were used to elicit the utility values, and these were found to vary significantly between valuation methods and depending on who conducted the valuation. The identified utility values were grouped into six categories: screening-related states; preventative states; adverse events in breast cancer and its treatment; non-specific breast cancer; metastatic breast cancer states; and early breast cancer states. The large number of values identified for metastatic breast cancer and early breast cancer states enabled data to be synthesised using metaregression. This method used utility as the dependent variables with study characteristics as independent variables allowing the estimation of pooled utility values. The authors used two separate models for early and metastatic breast cancer as these health states differ considerably, which requires different control variables to be used in the metaregression. Table 77 shows three models together with the overall mean estimated utility value. Model 1 was weighted by the inverse of the SD, model 2 was weighted by sample size using all available utility values and model 3 was weighted by sample size but drops any values that may not be recognised as utility scores.
TABLE 76
Summary of model input parameters
TABLE 77
Breast cancer utility values
Discounting
To present costs and benefits in present values, it is necessary to apply a discount rate. In line with current recommendations published by NICE, all costs and benefits were discounted at 3.5% per year.328
Two approaches were taken to calculate discounted costs and discounted QALYs because the analysis aims to identify the incremental costs and benefits of risk-based screening intervals over the lifetime of a population of women. Approach 1 takes account of the instantaneous events in the screening programme and approach 2 takes account of discounting between these events.
The first approach deals with costs or QALYs accrued at a single time point, such as the cost of attending subsequent screening mammograms. The standard discounting formula is applied (see Equation 1):
To calculate the discounted costs or QALYs which were accrued between two events, for example the QALYs between two screening mammograms, an alternative discounting formula was applied (see Equation 2):
where Vab is the value of costs or QALYs accrued at a constant rate between the times of events a and b. The instantaneous discount rate (IDR) is equivalent to the discount rate (DR) from Equation 1, given by Equation 3:
Summary of model input parameters
Table 76 summarises the model input parameters.
Modelling assumptions
The key assumptions taken in our model are:
- Death attributable to breast cancer occurs only following the initiation of breast cancer onset in the model.
- Following a test positive mammogram, screen recall assessment happens immediately.
- Following a confirmed diagnosis of breast cancer, treatment follows immediately.
- Only women who have a progressing preclinical tumour will present symptomatically [i.e. women without a breast tumour will not present symptomatically outside a screening programme (e.g. ‘worried well’ women)].
- Once women reach the maximum age range for the screening programme, no further screening mammograms take place (i.e. once women reach the maximum screening age they will not request a screening appointment)
- Women with indolent DCIS will not progress on to subsequent breast cancer natural history states.
- Only one diagnosis can occur in the lifetime of a woman.
- Following the initial screening appointment where screening interval based on risk is determined, no further adjustments are made to this risk-based interval.
- The TC estimated risk is a perfect measure of risk in our population.
- The risk of being diagnosed with breast cancer does not change over time [e.g. when women change lifestyle factors that may effect this risk (i.e. smoking cessation)].
- All women enter the model at the age of 45 years rather than 50 years. This was necessary to allow time for the tumour to progress to a detectable size at the age of 50 years, which would mirror current evidence. In addition, to account for prevalence of breast cancer at the initial screening appointment; tumour onset may have occurred subsequent to 45 years of age.
- There is a lag period, taken from the literature, which shifts the observed incidence of breast cancer under the current screening policy to younger ages. The age distribution of onset remains the same.
- There is independence between the risk of developing breast cancer and the rate at which breast cancer progresses.
Results
Results from the preliminary economic model are now presented. These results are indicative only and represent an initial analysis of the data.
Costs
The estimated total costs and discounted cost per screening participant are shown in Table 78.
TABLE 78
Total costs and discounted cost per screening participant
Life-years
The estimated total life-years per screening participant are shown in Table 79.
TABLE 79
Total life-years per screening participant
Discussion
This study conducted a preliminary economic analysis model to represent a risk-based breast screening strategy compared with the current NHSBSP. The model was populated to show some indicative results from introducing a risk-based screeing programme with subsequent targeted screening intervals. The model-based evaluation was, as anticipated, limited by the availability of key data inputs. Key data are needed before a sufficiently robust base case analysis can be conducted.
In the absence of these data, it was not logical to perform sensitivity analysis on a base case that was not sufficiently advanced. Conducting a sensitivity analysis at this stage of the model development would only indicate what we already know, which is that there are key parameter inputs that were not sufficiently informed. Ideally, we would want to use a combination of probabilistic and one-way sensitivity analyses to understand joint uncertainty in the model parameters together with key structural assumptions that are driving relative cost-effectiveness. Three remaining analyses are in progress: model calibration to confirm whether or not the estimated unobservable natural history parameters accurately reflect observed incidence of in situ and invasive breast cancer; (2) probabilistic sensitivity analysis to identify the joint parameter uncertainty in the model; and (3) expected value of perfect information will be calculated to quantify the value of future research.
Concluding remarks
This preliminary model-based cost-effectiveness analysis study has identified the relevant costs and patient benefits associated with introducing a risk-based stratified screening programme. Using the available data, the risk-based screening programme appears to be a potentially cost-effective option, but this result is uncertain because of the limitations in the available data. The current level of uncertainty would suggest that it is not rational to recommend the introduction of a risk-based breast screening programme at a national level.
General discussion
A substantial body of economic evidence has been developed to inform the inception and modification of breast screening programmes in the UK and globally. This study conducted a systematic review, which confirmed this extensive evidence base but failed to identify definitive evidence to support the cost-effectiveness of existing screening programmes. Subsequent modifications to a breast screening programme should also be informed by economic evidence. This study started to look at the implications of introducing a risk-based stratified screening programme by structuring a preliminary model-based cost-effectiveness analysis to identify the incremental costs and benefits compared with the current national breast screening programme.
The model-based analysis assumed the NHS perspective. A survey to understand the patient assessment costs of attending a screening programme was conducted, and confirmed that the patient costs incurred were relatively low. The results from this survey were consistent with those reported by Brown et al.246 This finding provided reassurance that taking the NHS perspective for the economic analysis was reasonable. The NHS viewpoint is also suggested to be most appropriate given the lack of agreement on the methods to incorporate a broader perspective, and current national guidelines for economic analysis suggest that the NHS perspective is appropriate.328
The economic analysis suggested that if the assumptions and model inputs used in the preliminary analysis are correct then risk-based stratified screening could be a cost-effective use of health-care resources. The estimated cost per QALY was below the nationally agreed threshold range of £20,000 per QALY used by NICE to guide whether or not interventions are likely to be cost-effective. However, this finding should be interpreted with extreme caution. The availability of data necessary to understand the impact of introducing a risk-based screening programme was low in terms of relevance to the research question. In particular, the identified key data requirements included information on the predictive value of the risk-based algorithm; empirical data to support the identified relevant risk categories and associated screening interval; estimated lifetime risk for each individual is needed to replace the current extrapolation of 10-year risk data to incorporate individual risk into the MBSM; whether or not the duration of the preclinical screen-detectable period (sojourn time) is linked with the algorithm predicted risk category; and the cost of treatment linked with identified risk category.
A number of key assumptions were made to generate an estimate of cost-effectiveness, which are likely to drive the observed incremental costs and benefits of the intervention compared with current practice. One key assumption that needs further evaluation using a prospective follow-up study is that the method used to create the risk stratifications, the TC risk algorithm, generates a perfectly sensitive and specific measure of risk in the population of women. There were no data to support or refute this assumption available for this economic analysis but expert opinion suggested that this was a valid approach to take. Generating robust evidence to identify the predictive value of risk-scoring algorithms will be a common challenge as health-care systems embrace the move towards personalised medicine.329
A further key assumption was the assumed independence between the estimated risk of developing breast cancer and subsequent rate at which breast cancer progresses. This has implications for the treatment strategies used by clinicians and the overall survival of women. There are an increasing number of personalised medicines in the area of breast cancer emerging into practice. However, these currently focus on the genetic variation or the presence of specific biomarkers in the breast tumour.330 It is likely that, as risk-scoring algorithms become used in practice, clinicians will evolve their prescribing behaviour to personalise the treatment regimens and more aggressively treat women at a high risk of breast cancer. This is an area for future research.
Overdiagnosis is a key topic for continued debate when evaluating the impact of national breast screening programmes. Overdiagnosis describes cancers picked up and treated as a result of screening which would not have been diagnosed in a woman’s lifetime had screening not taken place. Duffy et al.331 estimated the absolute benefits of a national breast screening programme to be 8.8 and 5.7 breast cancer deaths prevented per 1000 women screened for 20 years starting at age 50 years, from the Swedish Two-County Trial and screening programme in England, respectively. The corresponding estimated numbers of cases overdiagnosed per 1000 women screened for 20 years were, respectively, 4.3 and 2.3 per 1000. The issue of overdiagnosis is an important caveat for any screening programme and it is not clear whether using a risk-based strategy will inflate or decrease the number of overdiagnosed cases of breast cancer or the associated impact on relative cost-effectiveness.
There are a number of limitations with the assumed cost of the screening programme. The component costs of the assumed £96M cost per year is not known. It is likely that the number of women screened is increasing over time, which would mean that £96M per year will be an underestimate of the current costs. Furthermore, this figure does not include non-attenders, which may affect the total cost. Overall, the published figure is likely to be an underestimate of the total NHSBSP cost, but there are no other data sources to verify this figure. This is an area for future research.
Implications for policy
This study has developed a preliminary economic model, which is indicative of the relative cost-effectiveness of the risk-based stratified screening programme compared with the current UK screening programme. The model has indicated some key parameters and assumptions that drive the observed estimate of cost-effectiveness. Based on the current uncertainties in the evidence base, it is necessary for further work to be carried out. This study was designed from a UK perspective and it is outside its scope to try and draw comparison across other EU countries. The model provides a conceptual basis for identifying the relative costs and benefits of a risk-based strategy, but further data, and subsequent sensitivity analysis, are required before definitive conclusions can be made.
Future research
The model-based analysis has identified some key uncertainties in the evidence base needed to generate robust estimates of the relative cost-effectiveness of a risk-based breast screening programme.
- The accuracy and predictive value of the algorithm (TC) used to generate the risk subgroups in the population of women.
- Information on the link between the estimated risk of developing breast cancer and the rate at which breast cancer progresses.
- The observed uptake rate of a risk-based screening programme together with an understanding of the factors driving the observed uptake rate and relationship with women’s perception of their perceived risk of developing breast cancer.
- The actual cost of the current national breast screening programme.
- The cost of treatment for breast cancer, taking into account current treatment modalities, and the potential link between risk category and selection of treatment.
- The resources and costs used, for a national programme, to inform women of their individual risk of developing cancer and the mechanism needed to target them towards the appropriate screening interval. Subsequent modifications to the risk-based algorithm and associated screening interval could be evaluated using the proposed MBSM.
Conclusion
This preliminary model-based cost-effectiveness analysis has shown that using a risk-based stratified breast screening programme may potentially be a cost-effective use of resources. This result should be interpreted with extreme caution, as there were limitations in the data available to populate the model inputs and the estimated relative cost-effectiveness is associated with extensive uncertainty. Additional research is needed to reduce the current uncertainty in the evidence base to produce a reliable base-case analysis, and then subsequent sensitivity analyses are needed to identify the key drivers of the relative cost-effectiveness of a risk-based breast screening programme.
- Aim
- Objectives
- Study 1: systematic review of economic evaluations of breast screening programmes and screening modalities
- Study 2: quantifying the impact of a national breast screening programme on women’s out-of-pocket expenses
- Study 3: preliminary model-based cost-effectiveness analysis of a risk-based screening strategy for breast cancer as part of the NHS Breast Screening Programme
- General discussion
- Implications for policy
- Future research
- Conclusion
- Cost-effectiveness - Improvement in risk prediction, early detection and prevent...Cost-effectiveness - Improvement in risk prediction, early detection and prevention of breast cancer in the NHS Breast Screening Programme and family history clinics: a dual cohort study
- 602428955F1 NIH_MGC_16 Homo sapiens cDNA clone IMAGE:4558668 5', mRNA sequence602428955F1 NIH_MGC_16 Homo sapiens cDNA clone IMAGE:4558668 5', mRNA sequencegi|13136514|gnl|dbEST|7994952|gb|BG 6.1|Nucleotide
- Systrophia helicycloides isolate C6_Ink-Pal-cP90-j cytochrome c oxidase subunit ...Systrophia helicycloides isolate C6_Ink-Pal-cP90-j cytochrome c oxidase subunit I (COI) gene, partial cds; mitochondrialgi|328671891|gb|JF414814.1|Nucleotide
- Homo sapiens replication protein A4, 34kDa, mRNA (cDNA clone MGC:97361 IMAGE:726...Homo sapiens replication protein A4, 34kDa, mRNA (cDNA clone MGC:97361 IMAGE:7262622), complete cdsgi|46854954|gb|BC069824.1|Nucleotide
Your browsing activity is empty.
Activity recording is turned off.
See more...