Included under terms of UK Non-commercial Government License.
NCBI Bookshelf. A service of the National Library of Medicine, National Institutes of Health.
Raine R, Fitzpatrick R, Barratt H, et al. Challenges, solutions and future directions in the evaluation of service innovations in health care and public health. Southampton (UK): NIHR Journals Library; 2016 May. (Health Services and Delivery Research, No. 4.16.) doi: 10.3310/hsdr04160-37
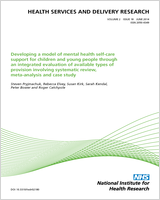
Challenges, solutions and future directions in the evaluation of service innovations in health care and public health.
Show detailsAbstract
An understanding of the impact of health and care interventions and policy is essential for decisions about which to fund. In this essay we discuss quantitative approaches in providing evaluative evidence. Experimental approaches allow the use of ‘gold-standard’ methods such as randomised controlled trials to produce results with high internal validity. However, the findings may be limited with regard to generalisation: that is, feature reduced externality validity. Observational quantitative approaches, including matching, synthetic control and instrumental variables, use administrative, survey and other forms of ‘observational’ data, and produce results with good generalisability. These methods have been developing in the literature and are better able to address core challenges such as selection bias, and so improve internal validity. Evaluators have a range of quantitative methods available, both experimental and observational. It is perhaps a combination of these approaches that is most suited to evaluating complex interventions.
Scientific summary
An understanding of the impact of health and care interventions and policy is essential to inform decisions about which to fund. Quantitative approaches can provide robust evaluative evidence about the causal effects of intervention choices.
Randomised controlled trials (RCTs) are well established. They have good internal validity: that is, they produce accurate estimates of causal effects for the study participants, minimising selection bias (confounding by indication). The findings may, however, be limited with regard to generalisation: that is, feature reduced externality validity.
Observational quantitative approaches, which use data on actual practice, can produce results with good generalisability. These methods have been developing in the literature and are better able to address core challenges such as selection bias, and so improve internal validity.
This essay aims to summarise a range of established and new approaches, discussing the implications for improving internal and external validity in evaluations of complex interventions.
Randomised controlled trials can provide unbiased estimates of the relative effectiveness of different interventions within the study sample. However, treatment protocols and interventions can differ from those used in routine practice, and this can limit the generalisability of RCT results. To address this issue, trial samples can be reweighted using observational data about the characteristics of people in routine practice, comparing the outcomes of people in the trial with those in practice settings. Evidence for similarity of outcomes can be assessed using ‘placebo tests’.
Observational studies may provide effect estimates confounded by indication (i.e. exhibit treatment-selection bias) because the factors that determine actual treatment options for individuals are also likely to affect their treatment outcomes. Observational studies seek to address selection by trying to remove the consequences of the selection process. This can be done by using data on all relevant selection factors, applying matching methods, including recently developed ‘genetic’ matching, or using regression control.
When selection is likely to be influence by unobserved factors, alternative methods are available that exploit the existence of particular circumstances that structure the problem and data. These include instrumental variables, regression discontinuity and the difference-in-difference.
There a growing need to demonstrate effectiveness and cost-effectiveness of complex interventions. This essay has shown that evaluators have a range of quantitative methods, both experimental and observational. However, it is perhaps the use of a combination of these approaches that might be most suited to evaluating complex interventions.
Introduction
An understanding of the impact of different health and care interventions is essential in helping us to make the best choices when deciding which interventions and treatments to fund. Quantitative approaches can provide robust evaluative evidence about the causal effects of intervention choices. Randomised controlled trials (RCTs) are well established, but recently there have been significant advances and refinements in observational approaches, allowing complex interventions to be assessed in more pragmatic settings.
There are significant challenges in the evaluation of complex interventions. Complexity can be taken to mean complicated in the sense of interventions with many interdependency components but, more pertinently, complexity can also refer to systems that adapt to context and exhibit non-linear responses.1 Many health and care policy ‘interventions’ can be regarded as complex in the latter sense, for example policies to create closer integration across the care system or to support person-centred approaches to management of chronic disease.
Observational or non-experimental methods, though having their own limitations, are an alternative way to produce estimates of the causal effects of complex interventions. They rely on ‘natural’ variation in a population regarding the characteristics of the intervention, and the factors that affect its outcomes. As with experimental approaches such as RCTs, establishing the counterfactual outcome is the key to evaluation. In general, treatment choice is according to anticipated prognosis or performance. Hence a study that makes unadjusted comparisons of the outcomes of those units (e.g. patients or hospitals) is liable to provide estimates of the effect of treatment that are biased owing to selection into treatment (also known as confounding by indication). On their own, the observed outcomes of non-recipients of the intervention will not be good indicators of the counterfactual outcomes that would have been experienced by people who did use the intervention.
Observational approaches seek to address this problem by trying to identify and account for the consequences of this selection process. In RCTs, people are randomly assigned between intervention and (counterfactual) control groups. In most RCTs there is a selection process for including study participants, for example according to eligibility criteria, which may include requiring informed consent. By contrast, in observational studies the inclusion criteria tend to be less stringent, which allows these studies to observe outcomes for those patients and treatments that are relevant for routine practice.
These contrasting features of RCTs and observational approaches give rise to different properties when they are used in evaluations. Two important properties are those of internal and external validity. The former, that of internal validity, concerns the extent to which the contrast we are making allows accurate estimates of causal effects for the study participants: that is, the study avoids selection bias due to treatment selection (confounding by indication). The second, that of external validity, is about the extent to which the results of a study are generalisable and applicable to routine practice: that is, the study avoids selection bias due to sample selection.
Randomised controlled trials have far greater internal validity owing to the randomisation process which removes the effects of selection into treatment, but often have lower external validity owing to the restrictions an experimental study places on how an intervention can be delivered and who can be included: that is, sample selection. Other essays in this volume delineate the repertoire of randomised designs now available in health services research (e.g. Essay 2).
Observational studies, on the other hand, should provide results that are representative of what may be achieved in practice, and therefore may have greater external validity. However, the internal validity of observational studies is compromised because the assignment of the intervention is conditional on certain (unobserved) characteristics (not the play of chance) that also affect outcomes, and this introduces bias due to treatment selection.
In other words, we would expect RCTs to provide unbiased estimates of sample average treatment effects, but potentially biased estimates of the treatment effects of people receiving the intervention in routine practice: that is, of the population average treatment effects of the treated. Box 3.1 provides details.
When evaluating complex interventions, both of these validity requirements can be hard to achieve. With regard internal validity, there are particular challenges in identifying and distinguishing the ‘intervention’, and precluding cross-contamination between intervention and control groups. Turning to external validity, because complex interventions tend to be highly context specific in their effects, generalising the results for policy and practice requires more nuanced analyses of why effects occur, not just an estimate of the magnitude of the effect within the RCT setting.
This essay aims to summarise a range of both established and new approaches aimed at assessing and improving internal and external validity for both observational and experimental evaluations of complex interventions.
Improving external validity of randomised controlled trials
Why external validity is an issue
Randomised controlled trials, when carried out to a high standard, can provide unbiased estimates of the relative effectiveness of different interventions within the study sample. Although much time and attention have been given to ensuring maximisation of internal validity through high-quality design and conduct of RCTs, problems of external validity have been less rigorously addressed. In RCTs, treatment protocols and interventions can differ from those used in routine practice, and therefore results from a RCT may be unrepresentative of what would happen in practice. Moreover, regarding complex interventions, the effects of the intervention might depend closely on factors outside the study, making the results highly context-specific. For example, the impact of telehealth could also be affected by local policies regarding health and social care integration.
Assumptions are being made when directly generalising the results of a RCT to target populations: first, that the RCT participants have similar characteristics to the target population and that the control intervention in the RCT equates to what is provided as usual care in routine practice; and, second, that the intervention in the RCT is delivered as it would be if rolled out in practice.
Using observational data to improve external validity of randomised controlled trials
There have been recent developments in using observational approaches and data to address the issue of external validity. These involve comparing the characteristics of RCT study samples with data from the population of people using (or eligible for) the intervention. Trial samples can be reweighted in line with the characteristics of people in routine practice, and then the outcomes of the people in the trial can be compared with those of people in routine practice. Placebo tests can then be used to assess the evidence for similarity of outcomes.
Recent work by Hartman et al.2 has built on previous approaches which have considered the external validity and generalisability of results from RCTs. The work illustrates how to extrapolate from the sample average treatment effect estimated from a RCT to a population average treatment effect for the population of interest, by combining results from experimental studies with observational studies. In particular, they specify the assumptions that would be required for a reweighting of the treatment group population of a RCT – using observational data on a target population of treated individuals – to produce population-treatment effects of the treated.
As well as formally defining the assumptions required for estimating population treatment effects from RCT data, Hartman et al.2 also recommend that future randomised trials should consider how the results may be combined with observational data, and consider this when designing the trial.
Placebo tests
Placebo tests are an approach for assessing model validity by testing for an effect where none should exist. Placebo tests can be used to assess whether or not the assumptions required for generalising RCTs to routine practice are likely to be met. In Hartman et al.,2 the placebo tests contrast the outcomes for patients who receive the treatment in routine practice with those who receive that same treatment within the trial setting, after adjusting for differences in observed patient characteristics between the RCT and the routine practice settings. The placebo tests are formulated such that the null hypothesis is that the adjusted outcomes from the treatment group in the RCT are ‘not equivalent’ to the outcomes following treatment in routine practice.2 If the null is not rejected, then this is an indication that the results of the RCT are not generalisable because of differences in the patients or the treatments between the RCT and routine practice settings, or that there is insufficient statistical power to reject the null hypothesis (with reference to Box 3.1, this is essentially a test of where are the observed characteristics of the treated population and are the reweighted RCT outcomes for the treatment group). Placebo tests can also be used to compare control groups in a similar way between sample and target populations.
As an example, the use of placebo tests can be discussed using the Whole Systems Demonstrator (WSD) cluster randomised trial as an example.3 This was a large RCT that evaluated telehealth against standard care. The trial randomised 3230 adults with chronic conditions to either telehealth or usual care, where the telehealth arm received home-based technology to record medical information, such as blood glucose level and weight, and to answer symptom questions. Information from patients was then transmitted automatically to monitoring centres staffed by employees from local health-care organisations. Published results were cautiously optimistic and suggested that telehealth patients experienced fewer emergency hospital admissions than controls over 12 months [incidence rate ratio 0.81, 95% confidence interval (CI) 0.65 to 1.00; p = 0.046].4
Concerns about the generalisability of the WSD trial were raised for several reasons, but in particular because emergency admission rates increased among control patients shortly after their recruitment, suggesting that these patients may not have received usual care. It was deemed unlikely that this increase in admissions represented a normal evolution in service use, and instead it was suggested that health-care professionals may have identified unmet needs while recruiting patients to the control group and changed the management of this group from usual care or, alternatively, the trial recruitment processes might have led to changes in behaviour among patients.4
To assess whether or not the control group in the WSD RCT was representative of usual care, placebo tests were carried out.4 A target population of patients who met the RCT inclusion criteria and who received usual care (n = 88,830) was identified from observational data. Of these, 1293 individuals were matched with the RCT controls on 65 identified baseline covariates (e.g. demographics, blood pressure, medications). The placebo test then contrasted outcomes from the RCT between the matched target control population and the control group of the WSD trial. This process is described in greater detail in Steventon et al.4
A comparison of the RCT control arm versus the matched controls from the observational data gave an incidence rate ratio of 1.22 (95% CI 1.05 to 1.43) for emergency admissions. In other words, emergency admissions were significantly higher in the control arm of the WSD trial than in a similar group of patients who did not participate in the trial. Consequently, the trial results may have shown an inflated beneficial effect of telehealth interventions. This example highlights the importance of assessing the generalisability of RCT results to a target population. For those settings in which placebo tests fail, Steventon et al.5 propose sensitivity analyses.
Improving internal validity of observational studies
Addressing the selection problem
Observational studies, no matter how large, may provide effectiveness estimates confounded by indication: that is, exhibit treatment-selection bias. Where treatment or intervention assignment uses a decision rule that accounts for the characteristics of the individual – for example, the treatment may be more likely to be given to the patients in the poorest health – observed outcomes will provide a biased estimate of the intervention if these characteristics also directly impact on outcomes. In such an example, the intervention group will have lower observed outcomes as a result of their poor health, irrespective of the effects of the treatment.
Observational studies seek to address this selection problem by trying to remove the consequences of the selection process. The basis of this approach is to account as far as possible for the difference in characteristics between people who are getting the intervention and those who are not. This method generally relies on being able to observe all relevant characteristics, which in practice is never entirely possible. Where we anticipate having data on all relevant confounders, adjustment can be made using a number of well-documented methods, including regression and matching (e.g. propensity and prognostic scoring). More recent developments in this regard include ‘genetic’ matching.
When we do not anticipate being able to identify all confounders, adjustment is more complex. Where selection is on unobserved characteristics, methods include the use of instrumental variables (IVs), regression discontinuity and the difference-in-difference approach. These methods exploit the existence of particular circumstances that structure the problem and data to address the selection problem. There have been a number of significant refinements of these approaches in recent years, including the use of synthetic controls and improved diagnostics.
The difference-in-difference method
The difference-in-difference approach addresses the selection problem by comparing the experiences of a control group with the intervention group before and after the intervention. The idea is that, under certain assumptions, selection bias can be controlled for by removing any difference in the outcome indicator between groups before the intervention from differences after the intervention.
From Figure 3.1, β4 is the unadjusted difference in outcome indicators between the two groups after the intervention. By contrast, β3 is the adjusted difference, and provides a (less) biased estimate of the intervention effect as it takes into account differences in the outcome measure between the two groups that were present at the start of the evaluation period. In this linear example, β1 is the size of the selection bias. The unbiased estimate β3 in this example is found by subtracting the difference β1 at baseline (time t = 0) from the difference β4 at time t = 1.

FIGURE 3.1
The difference-in-difference approach.
The difference-in-difference approach was utilised in an evaluation of personal health budgets (PHBs), which was a policy piloted in 2009 whereby patients were given control over their own budgets to meet their care needs.6 A fully RCT proved unfeasible, so a pragmatic design was chosen whereby some sites were randomised while others selected participants. The study comprised 2000 participants covering a range of health conditions. For illustration, one of the main outcome measures was care-related quality of life (Adult Social Care Outcomes Toolkit; ASCOT). At baseline the PHB group had (significantly) lower ASCOT scores than the control group. When measured at follow-up, the PHB group still had lower ASCOT scores than the control group, but the gap had closed. Subtracting the larger negative difference at baseline in the scores produced a result whereby the PHB group had significantly higher (better) scores than the control group at follow-up. Without accounting for the poorer quality of life of the PHB group prior to the intervention, this selection bias might have led to opposite conclusions about the effectiveness of PHBs.
A range of assumptions is required for the difference-in-difference approach to give unbiased estimates. It can be applied where there is only a partial uptake to a new intervention, allowing for a control group, and where there is a defined before-and-after time, recorded in the data. Although it can also control for persistent differences between groups that are not related to the intervention, it is a less appropriate method where baseline factors may influence the rate of change of outcomes during the follow-up period, that is, when there are unobserved pretreatment differences between the groups whose effects change over the follow-up period. For this, the synthetic control method may be a more appropriate approach.
Synthetic controls
The synthetic control method also aims to control for treatment selection bias by using covariates and outcomes of the intervention and control groups prior to implementation to adjust outcomes after the intervention. The method exploits the availability of multiple time points and control units (e.g. different localities) prior to baseline to try and adjust for those unobserved characteristics whose effects may differ over time. A ‘synthetic’ control sample is constructed by weighting together multiple control units in such a way that the expected outcomes in the pre-implementation period are similar for the control and intervention groups, in order to minimise the treatment selection bias because of unobserved factors whose effects vary over time.
The synthetic control method estimates treatment effects by using this weighted synthetic control group to represent the counterfactual outcomes for the treated group after implmentation.7 The idea behind synthetic controls is that a combination of units (where units may be hospitals, general practices, an area or region, etc.) often provide a better comparison for the unit exposed to the intervention of interest than any single unit alone. Furthermore, as the choice of synthetic control does not require access to post-intervention outcomes, this method allows researchers to decide on study design without knowing how these decisions will affect the resulting conclusions: an important issue for reducing potential research bias in observational studies.7
Kreif et al.8 carried out an analysis to compare and contrast both the difference-in-difference method and synthetic controls for an evaluation of the Advance Quality (AQ) programme, which is a pay-for-performance initiative linking health-care provider income to quality measures. The initiative was first introduced into 24 hospitals in the North West of England, with the other nine regions in England providing a potential comparison group of 132 hospitals. Figure 3.2 shows the trajectory of the difference from expected mortality rates before and after the implementation of the AQ programme, in both the North West and the rest of England. For control regions, the size of the difference from the expected mortality rate is relatively constant and fluctuates around zero for the period of observation. For the North West, the mortality rate is higher than would be expected before AQ, but this improves after the introduction of the AQ programme. What is clear from the graph is that there is little evidence to support the assumption of parallel trends required for a (standard) difference-in-difference approach.

FIGURE 3.2
The observed risk adjusted mortality in pneumonia patients for the North West vs. rest of England before and after the introduction of AQ. Adapted from Kreif et al., © 2015, John Wiley & Sons Ltd., under the terms of the Creative Commons (more...)
For the synthetic control method, a control group was selected from the potential pool of 132 hospitals according to pre-intervention characteristics of hospital type, size and scale, and hospital quality measures. The controls were weighted according to the pretreatment trajectory of the intervention hospitals (Figure 3.3) and hence the synthetic control is more representative of the counterfactual outcome for the intervention hospitals.

FIGURE 3.3
Comparison of the intervention hospitals with the weighted synthetic control group, pre and post intervention. Adapted from Kreif et al., © 2015, John Wiley & Sons Ltd., under the terms of the Creative Commons Attribution International (more...)
For this particular example, when using a synthetic control comparator it was found that the AQ initiative did not significantly reduce 28-day hospital mortality. This is in contrast to the original analysis that found a significant reduction in mortality in the AQ group. This highlights the need to consider how well analysis assumptions are met when choosing an appropriate method, and also the importance of considering alternative approaches and contrasting the results. In this example, the difference-in-difference approach appeared to be flawed in that the parallel trends assumption was poorly met. The synthetic control method should be considered as an alternative approach if parallel trends are not present, although this method does require data on a sufficiently long pretreatment period to allow for the selection and weighting of an appropriate control group. More details on this analysis and other alternatives are provided in the paper by Kreif et al.8
Instrumental variables
The concept of IVs has been around for over half a century, and they are widely used in economics because of the difficulty of doing controlled experiments in this field.9 Despite their popularity in economics, they have been little used in the biostatistical literature, but their popularity is growing as they may provide a useful methodology for addressing the selection problem posed by observational studies when relevant instruments can be identified.
The essence of an IV approach is to find an indicator that is highly related to the treatment or intervention being assessed but does not independently affect the outcome of interest.10 There is an analogy with the RCT approach in that the random allocator can be regarded as a perfect instrument.11 In an IV analysis, the intervention variable is therefore replaced by the chosen IV, to remove the dependence of the intervention variable (or assignment) on the unobserved confounders which might influence outcomes other than through the intervention. This reduces, or in the case of very large samples eliminates, the selection bias. The use of IVs will be illustrated with an example.
A 2009 survey of service care utilisation by the elderly was carried out, with the aim of assessing the cost-effectiveness of the services provided.12 In this case, the intensity of service utilisation was expected to be directly related to a patient’s level of frailty and ill-health, as these factors are key elements in the patient’s assessment of need. Therefore, methods had to be considered which distinguished the variation in care-related quality of life owing to service use as opposed to other factors.
Data were collected on a range of outcome measures, including a measure of social care-related quality of life (SCRQOL), using the ASCOT measure.13 As is common with health and care utilisation data, the observed relationship between the intensity of service utilisation and the outcome (care-related quality of life) was negatively sloped. In other words, unadjusted data would suggest a negative effect of care utilisation on care-related quality of life. However, this observed result was probably attributable to selection bias because the amount of service a person was offered in the care system was related to their baseline level of need (e.g. severity of health condition), which was in turn negatively correlated with their quality of life. Observed confounders – for example indicators of need or poor health – can be used to control for selection, but there may be many other unobserved factors that influence both selection (i.e. in this case the amount of service) and outcomes.
To address these, an IV analysis was carried out. An IV was needed that was related to the amount of service a person received but not their current SCRQOL score. As different local authorities used different service eligibility policies, this characteristic could be used as an instrument. With the use of the IV controlling for both known and unknown confounders, a significant positive association between intensity of service use and care-related quality of life was found.
Instrumental variables have a number of applications and have been used in recent studies assessing NHS expenditure on mortality and quality of life,14 impact of social care use on hospital utilisation15 and impact of care services on hospital delayed discharge rates,16 to name but a few. They can be an effective way to deal with unobservables, and are useful for adjusting for treatment selection problems that occur in non-randomised studies, but, as with all methods, there are limitations.11,17 The key challenge is to find suitable instruments and, once chosen, to demonstrate that the choice of IV was appropriate. A crucial assumption for the correct use of IVs is that the IV is (strongly) associated with the intervention of interest but not directly to the outcome. This condition cannot be directly evaluated, although a range of diagnostic tests may help to inform instrument specification. Nonetheless, the choice of the IV is often largely based on prior judgement. Poor or ‘weak’ instruments will lead to significant bias, potentially offsetting reductions in selection bias. IV analyses need a large sample, and results from an IV analysis may still be biased. Recommendations and guidance around the use of IVs for the evaluation of complex interventions would be a useful step for taking these methods forward.
Propensity scores
The above sections discuss methods that are suitable when not all of the selection characteristics are known. When it is believed that all the factors on which selection of the intervention group has been based are known and observed, propensity scores can be used. Propensity scores were first described in the early 1980s.18 A propensity score is developed by using a statistical model to estimate the individual likelihood that patients will receive treatment, using known explanatory variables such as demographic and medical history, prescribed drugs and consultation behaviour. This enables a propensity score to be calculated for each patient: that is, the likelihood that they will receive treatment or ‘fitted value’. Propensity scores can then be used either for adjustment in statistical models or to create matched groups by selecting treatment and control groups with similar propensity scores.19 Propensity score approaches have been used extensively in applied health research.
Although propensity scores go some way towards adjusting for selection bias in observational studies, they do not assure internal validity. Propensity scores can only be based on known and observed patient characteristics.
There is evidence of how propensity score approaches can fail to adequately address bias. The Randomised Aldactone Evaluation Study (RALES)20 evaluated spironolactone (Aldactone, G.D. Searle LLC), an aldosterone inhibitor, compared with placebo, and found that it reduced mortality in patients with severe heart failure (hazard ratio 0.70, 95% CI 0.60 to 0.82; p < 0.001). The results of this RCT were subsequently confirmed in two further trials.21,22
Freemantle et al.19 attempted to replicate the results of the RALES and subsequent trials using data from the Health Improvement Network, with the ultimate objective of bridging from the trial population to a real-world population of people with heart failure. As it was believed that a number of factors would influence the prescribing of spironolactone to patients, a complex propensity score was developed to account for this, which included information on patient demographics, comorbidities and current drug treatments. Two groups (n = 4412) treated and not treated with spironolactone, tightly matched on propensity score, were then selected for the analysis. An initial comparison of the two matched groups appeared to show results contradictory to those from the RALES trial, in that patients treated with spironolactone had an increased mortality risk (hazard ratio 1.32, 95% CI 1.18 to 1.47; p < 0.001).
The performance of a propensity score may be evaluated by assessing its homogeneity at different points on the scale. Figure 3.4 shows that, for different values of the propensity score (divided into quartiles), different estimates of the effect of spironolactone on mortality risk were estimated. If the propensity score had worked well, one would expect to see similar treatment effects across all strata. For this example, it appears that the prescriber making the clinical decision to treat with spironolactone used additional information on severity of heart failure that was not captured by the propensity score. Therefore, the matching of the two groups for the analysis was not appropriate, resulting in individuals with too low a risk of mortality being matched to the spironolactone patients. In particular, this affects the results of the apparently low-propensity subjects: that is, the lowest two quartiles of the propensity score (see Figure 3.4), where the hazard ratio for spironolactone is particularly high, although a bias is present across the entire range of the score.

FIGURE 3.4
Results of the propensity score analysis (overall and in quartiles) compared with the results of the RALES trial. HR, hazard ratio. Adapted by permission from BMJ Publishing Group Limited. Making interferences on treatment effects from real world data: (more...)
Propensity scores assume that all important variables have been included and that there are no additional processes related to disease severity that are associated with who receives the treatment of interest and who does not.
This analysis highlights the risks of using propensity scores where they do not adequately adjust for confounding by indication. Propensity scores, like all observational methods, need to be developed and used with caution, and results from propensity analyses need to be interpreted with caution. A useful starting point, as was taken in this example, is to start with the replication of a known treatment effect, and bridge from there to consider further unanswered questions. Considering the interaction between the propensity score and treatment outcome may also be a useful step to aid understanding of the score.
More recent studies have suggested that alternative approaches to generating a matched control group may perform better. An approach that may hold particular promise is ‘genetic matching’, which does not assume that there is a propensity score which is correctly specified but rather uses a multivariate matching (genetic) algorithm to produce a matched control group to maximise the balance of observed covariates between the treatment and control groups.23
Moving forward with quantitative methods for evaluating complex interventions
The growth in the availability of administrative, survey and other forms of ‘observational’ data offers huge potential for improved and more comprehensive evaluation of health and care interventions. Experimental trials, although allowing the use of ‘gold-standard’ methods such as RCTs, are often very expensive and time-consuming. Moreover, as outlined in this paper, the findings of RCTs may be limited with regard to generalisation: that is, feature reduced externality validity. Observational quantitative approaches can offer alternative methods of evaluation, particularly for complex interventions, by helping to both address issues of generalisability of RCT results and provide direct estimates of treatment effects. Methods for observational or non-randomisation studies have been advancing substantially in the literature, with many innovations now available to better address core challenges such as selection bias, and therefore to better improve internal validity Other essays in this volume (particularly Essays 6 and 7) describe other features of optimal study designs to evaluate complex features of health and care systems.
Observational studies will always be subject to treatment selection bias to some extent, and so have reduced internal validity compared with RCTs. Nonetheless, methods such as matching, synthetic control and IVs may mitigate the problem, if not completely eradicate any biases, especially when there are unknown confounding factors. Observational studies, therefore, can be used in place of RCTs to estimate treatment effects if careful consideration is given to the analysis and it is accepted that some bias will remain.24,25 Observational methods are also being used alongside RCTs to directly tackle the issue of representativeness of experimental studies.
The growing availability of routine data allows the use of observational methods. Although the quality, availability and completeness of routine data could always be improved to allow its easier use for the assessment of complex interventions, routine data can be used at relatively low cost and often have large scale and breadth (if not depth). Arguably, routine data sets are an underutilised resource, and greater investment to improve routine data sets and make them more research-friendly would increase their usage. Of course, routine data have their limitations: primary outcomes of interest may not be recorded, and there may be issues with the completeness and compatibility of data sets. In general, though, they have high external validity as they draw on data about everyday operation, and they can accommodate a high degree of subgroup analysis to explore why and when an intervention works. More pragmatically, observational studies based on routine data are generally low cost and have high feasibility.
Quantitative methods for estimating the causal effects of complex intervention inevitably make strong assumptions which must be critically examined. Future studies should report effectiveness estimates according to approaches that make different but plausible underlying assumptions. Where new methods are being utilised, it would be useful to compare the results with those from standard or appropriate alternative methodologies, and implications of the chosen analysis should also be explored through sensitivity analyses.
There is a growing need to demonstrate effectiveness and cost-effectiveness of complex interventions. Although just scratching the surface, this essay has shown that evaluators have a range of quantitative methods available, which include those in both the experimental and the observational toolboxes. However, it is perhaps the use of a combination of these approaches that might be most suited to evaluating complex interventions.
Acknowledgements
The authors would like to thank the editors; co-authors of some of the paper cited in this essay, including Noemi Kreif, Matt Sutton, Erin Hartmann and Adam Steventon; and participants at the Evaluation London 2015 workshop. We would also like to thank Jane Dennett for her help in copy-editing and preparing this manuscript. Any errors and omissions are the responsibility of the authors.
Contributions of authors
Clare Gillies (Medical Statistician) synthesised the evidence and literature, and produced the first draft of the essay.
Nick Freemantle (Epidemiologist and Biostatistician) provided expert input (particularly on the validity of RCTs and matching) and helped to edit the essay.
Richard Grieve (Health Economics Methodologist) provided expert input (particularly on observational methods) and helped to edit the essay.
Jasjeet Sekhon (Political Scientist and Statistician) provided expert input (particularly on RCT design and observational methods) and helped to edit the essay
Julien Forder (Economist) edited the essay, added further material, consolidated the various inputs and produced the final draft.
References
- 1.
- Shiell A, Hawe P, Gold L. Complex interventions or complex systems? Implications for health economic evaluation. BMJ 2008;336:1281–3. 10.1136/bmj.39569.510521.AD. [PMC free article: PMC2413333] [PubMed: 18535071] [CrossRef]
- 2.
- Hartman E, Grieve R, Ramsahai R, Sekhon JS. From sample average treatment effect to population average treatment effect on the treated: combining experimental with observational studies to estimate population treatment effects. J Roy Stat Soc A Sta 2015;178:757–78. 10.1111/rssa.12094. [CrossRef]
- 3.
- Bower P, Cartwright M, Hirani SP, Barlow J, Hendy J, Knapp M, et al. A comprehensive evaluation of the impact of telemonitoring in patients with long-term conditions and social care needs: protocol for the Whole Systems Demonstrator cluster randomised trial. BMC Health Serv Res 2011;11:184. 10.1186/1472-6963-11-184. [PMC free article: PMC3169462] [PubMed: 21819569] [CrossRef]
- 4.
- Steventon A, Bardsley M, Billings J, Dixon J, Doll H, Hirani S, et al. Effect of telehealth on use of secondary care and mortality: findings from the Whole System Demonstrator cluster randomised trial. BMJ 2012;344:e3874. 10.1136/bmj.e3874. [PMC free article: PMC3381047] [PubMed: 22723612] [CrossRef]
- 5.
- Steventon A, Grieve R, Bardsley M. An approach to assess generalizability in comparative effectiveness research: a case study of the whole systems demonstrator cluster randomized trial comparing telehealth with usual care for patients with chronic health conditions. Med Decis Mak 2015;35:1023–36. 10.1177/0272989X15585131. [PMC free article: PMC4592957] [PubMed: 25986472] [CrossRef]
- 6.
- Jones K, Forder J, Caiels J, Welch E, Glendinning C, Windle K. Personalization in the health care system: do personal health budgets have an impact on outcomes and cost? J Health Serv Res Policy 2013;18(Suppl. 2):59–67. 10.1177/1355819613503152. [PubMed: 24048694] [CrossRef]
- 7.
- Abadie A, Diamond A, Hainmueller J. Synthetic control methods for comparative case studies: estimating the effect of California’s tobacco control program. J Am Med Assoc 2010;105:493–505. 10.1198/jasa.2009.ap08746. [CrossRef]
- 8.
- Kreif N, Grieve HD, Hangartner D, Turner AJ, Nikolova S, Sutton M. Examination of the synthetic control method for evaluating health policies with multiple treated units [published online ahead of print 7 October 2015]. Health Econ 2015. [PMC free article: PMC5111584] [PubMed: 26443693]
- 9.
- Newhouse JP, McClellan M. Econometrics in outcomes research: the use of instrumental variables. Ann Rev Public Health 1998;19:17–34. 10.1146/annurev.publhealth.19.1.17. [PubMed: 9611610] [CrossRef]
- 10.
- Stukel TA, Fisher ES, Wennberg DE, Alter DA, Gottlieb DJ, Vermeulen MJ. Analysis of observational studies in the presence of treatment selection bias: effects of invasive cardiac management on ami survival using propensity score and instrumental variable methods. J Am Med Assoc 2007;297:278–85. 10.1001/jama.297.3.278. [PMC free article: PMC2170524] [PubMed: 17227979] [CrossRef]
- 11.
- Jones AM, Rice N. Econometric Evaluation of Health Policies. In Glied S, Smith PC, editors. The Oxford Handbook of Health Economics. Oxford: Oxford University Press; 2011. 10.1093/oxfordhb/9780199238828.013.0037. [CrossRef]
- 12.
- Forder J, Malley J, Towers AM, Netten A. Using cost-effectiveness estimates from survey data to guide commissioning: an application to home care. Health Econ 2014;23:979–92. 10.1002/hec.2973. [PubMed: 24038337] [CrossRef]
- 13.
- Netten A, Burge P, Malley J, Potoglou D, Towers A. Outcomes of social care for adults: developing a preference weighted measure. Health Technol Assess 2012;16(16). 10.3310/hta16160. [PubMed: 22459668] [CrossRef]
- 14.
- Claxton K, Martin S, Soares M, Rice N, Spackman E, Hinde S, et al. Methods for the estimation of the National Institute for Health and Care Excellence cost-effectiveness threshold. Health Technol Assess 2015;19(14). 10.3310/hta19140. [PMC free article: PMC4781395] [PubMed: 25692211] [CrossRef]
- 15.
- Forder J. Long-term care and hospital utilisation by older people: an analysis of substitution rates. Health Econ 2009;18:1322–38. 10.1002/hec.1438. [PubMed: 19206085] [CrossRef]
- 16.
- Gaughan J, Gravelle H, Siciliani L. Testing the bed-blocking hypothesis: does nursing and care home supply reduce delayed hospital discharges? Health Econ 2015;24:32–44. 10.1002/hec.3150. [PMC free article: PMC4406135] [PubMed: 25760581] [CrossRef]
- 17.
- Angrist JD, Pischke J-S. Mostly Harmless Econometrics: An Empiricist’s Companion. Princeton, NJ: Princeton University Press; 2009.
- 18.
- Rosenbaum PR, Rubin DB. The central role of the propensity score in observational studies for causal effects. Biometrika 1983;70:41–55. 10.1093/biomet/70.1.41. [CrossRef]
- 19.
- Freemantle N, Marston L, Walters K, Wood J, Reynolds MR, Petersen I. Making inferences on treatment effects from real world data: propensity scores, confounding by indication, and other perils for the unwary in observational research. BMJ 2013;347:f6904. 10.1136/bmj.f6409. [PubMed: 24217206] [CrossRef]
- 20.
- Pitt B, Zannad F, Remme WJ, Cody R, Castaigne A, Perez A, et al. The effect of spironolactone on morbidity and mortality in patients with severe heart failure. N Engl J Med 1999;341:709–17. 10.1056/NEJM199909023411001. [PubMed: 10471456] [CrossRef]
- 21.
- Pitt B, Remme W, Zannad F, Neaton J, Martinez F, Roniker B, et al. Eplerenone, a selective aldosterone blocker, in patients with left ventricular dysfunction after myocardial infarction. N Engl J Med 2003;348:1309–21. 10.1056/NEJMoa030207. [PubMed: 12668699] [CrossRef]
- 22.
- Zannad F, McMurray JJV, Krum H, van Veldhuisen DJ, Swedberg K, Shi H, et al. Eplerenone in patients with systolic heart failure and mild symptoms. N Engl J Med 2011;364:11–21. 10.1056/NEJMoa1009492. [PubMed: 21073363] [CrossRef]
- 23.
- Diamond A, Sekhon JS. Genetic matching for estimating causal effects: a general multivariate matching method for achieving balance in observational studies. Rev Econ Stat 2013;95:932–45. 10.1162/REST_a_00318. [CrossRef]
- 24.
- Freemantle N, Richardson M, Wood J, Ray D, Khosla S, Shahian D, et al. Weekend hospitalization and additional risk of death: an analysis of inpatient data. J Roy Soc Med 2012;105:74–84. 10.1258/jrsm.2012.120009. [PMC free article: PMC3284293] [PubMed: 22307037] [CrossRef]
- 25.
- Lester W, Freemantle N, Begaj I, Ray D, Wood J, Pagano D. Fatal venous thromboembolism associated with hospital admission: a cohort study to assess the impact of a national risk assessment target. Heart 2013;99:1734–9. 10.1136/heartjnl-2013-304479. [PubMed: 24038168] [CrossRef]
List of abbreviations
- AQ
Advance Quality
- ASCOT
Adult Social Care Outcomes Toolkit
- CI
confidence interval
- IV
instrumental variable
- PHB
personal health budget
- RALES
Randomized Aldactone Evalution Study
- RCT
randomised controlled trial
- SCRQOL
social care-related quality of life
- WSD
Whole Systems Demonstrator
- Declared competing interests of authors: None
- This essay should be referenced as follows: Gillies C, Freemantle N, Grieve R, Sekhon J, Forder J. Advancing quantitative methods for the evaluation of complex interventions. In Raine R, Fitzpatrick R, Barratt H, Bevan G, Black N, Boaden R, et al. Challenges, solutions and future directions in the evaluation of service innovations in health care and public health. Health Serv Deliv Res 2016;4(16). pp. 37–54.
- Advancing quantitative methods for the evaluation of complex interventions - Cha...Advancing quantitative methods for the evaluation of complex interventions - Challenges, solutions and future directions in the evaluation of service innovations in health care and public health
Your browsing activity is empty.
Activity recording is turned off.
See more...