NCBI Bookshelf. A service of the National Library of Medicine, National Institutes of Health.
Evans I, Thornton H, Chalmers I, et al. Testing Treatments: Better Research for Better Healthcare. 2nd edition. London: Pinter & Martin; 2011.
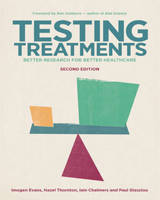
Testing Treatments: Better Research for Better Healthcare. 2nd edition.
Show detailsTHE PLAY OF CHANCE AND THE LAW OF LARGE NUMBERS
Trustworthy evidence about the effects of treatments relies on preventing biases (and of dealing with those that have not been prevented). Unless these characteristics of fair tests have been achieved, no amount of manipulation of the results of research can solve the problems that will remain, and their dangerous – sometimes lethal – consequences (see Chapters 1 and 2). Even when the steps taken to reduce biases have been successful, however, one can still be misled by the play of chance.
Everyone realizes that if you toss a coin repeatedly it is not all that uncommon to see ‘runs’ of five or more heads or tails, one after the other. And everyone realizes that the more times you toss a coin, the more likely it is that you will end up with similar numbers of heads and tails.
When comparing two treatments, any differences in results may simply reflect this play of chance. Say 40% of patients die after Treatment A compared with 60% of similar patients who die after receiving Treatment B. Table 1 shows what you would expect if 10 patients received each of the two treatments. The difference in the number of deaths between the two treatments is expressed as a ‘risk ratio’. The risk ratio in this example is 0.67.
Table 1
Does this small study provide a reliable estimate of the difference between Treatment A and Treatment B?
Based on these small numbers, would it be reasonable to conclude that Treatment A was better than Treatment B? Probably not. Chance might be the reason that some people got better in one group rather than the other. If the comparison was repeated in other small groups of patients, the numbers who died in each group might be reversed (6 against 4), or come out the same (5 against 5), or in some other ratio – just by chance.
But what would you expect to see if exactly the same proportion of patients in each treatment group (40% and 60%) died after 100 patients had received each of the treatments (Table 2)? Although the measure of difference (the risk ratio) is exactly the same (0.67) as in the comparison shown in Table 1, 40 deaths compared with 60 deaths is a more impressive difference than 4 compared with 6, and less likely to reflect the play of chance. So, the way to avoid being misled by the play of chance in treatment comparisons is to base conclusions on studying sufficiently large numbers of patients who die, deteriorate or improve, or stay the same. This is sometimes referred to as ‘the law of large numbers’.
Table 2
Does this moderate-sized study provide a reliable estimate of the difference between Treatment A and Treatment B?
ASSESSING THE ROLE THAT CHANCE MAY HAVE PLAYED IN FAIR TESTS
The role of chance can lead us to make two types of mistakes when interpreting the results of fair treatment comparisons: we may either mistakenly conclude that there are real differences in treatment outcomes when there are not, or that there are no differences when there are. The larger the number of treatment outcomes of interest observed, the lower the likelihood that we will be misled in these ways.
Because treatment comparisons cannot include everyone who has had or will have the condition being treated, it will never be possible definitively to find the ‘true differences’ between treatments. Instead, studies have to produce best guesses of what the true differences are likely to be.
The reliability of estimated differences will often be indicated by ‘Confidence Intervals’ (CI). These give the range within which the true differences are likely to lie. Most people will already be familiar with the concept of confidence intervals, even if not by that name. For example, in the run-up to an election, an opinion poll may report that Party A is 10 percentage points ahead of Party B; but the report will then often note that the difference between the parties could be as little as 5 points or as large as 15 points. This ‘confidence interval’ indicates that the true difference between the parties is likely to lie somewhere between 5 and 15 percentage points. The larger the number of people polled, the less the uncertainty there will be about the results, and therefore the narrower will be the confidence interval associated with the estimate of the difference.

The 95% Confidence Interval (CI) for the difference between Party A and Party B narrows as the number of people polled increases
Just as one can assess the degree of uncertainty around an estimated difference in the proportions of voters supporting two political parties, so also one can assess the degree of uncertainty around an estimated difference in the proportions of patients improving or deteriorating after two treatments. And here again, the greater the number of the treatment outcomes observed – say, recovery after a heart attack – in a comparison of two treatments, the narrower will be the confidence intervals surrounding estimates of treatment differences. With confidence intervals, ‘the narrower the better’.
A confidence interval is usually accompanied by an indication of how confident we can be that the true value lies within the range of estimates presented. A ‘95% confidence interval’, for example, means that we can be 95% confident that the true value of whatever it is that is being estimated lies within the confidence interval’s range. This means that there is a 5 in 100 (5%) chance that, actually, the ‘true’ value lies outside the range.
WHAT DOES A ‘SIGNIFICANT DIFFERENCE’ BETWEEN TREATMENTS MEAN?
Well, this is a trick question, because ‘significant difference’ can have several meanings. First, it can mean a difference that is actually important to the patient. However, when the authors of research reports state that there is a ‘significant difference’ they are often referring to ‘statistical significance’. And ‘statistically significant differences’ are not necessarily ‘significant’ in the everyday sense of the word. A difference between treatments which is very unlikely to be due to chance – ‘a statistically significant difference’ – may have little or no practical importance.
Take the example of a systematic review of randomized trials comparing the experiences of tens of thousands of healthy men who took an aspirin a day with the experiences of tens of thousands of other healthy men who did not take aspirin. This review found a lower rate of heart attacks among the aspirin takers and the difference was ‘statistically significant’ – that is, it was unlikely to be explained by the play of chance. But that doesn’t mean that it is necessarily of practical importance. If a healthy man’s chance of having a heart attack is already very low, taking a drug to make it even lower may be unjustified, particularly since aspirin has side-effects, some of which – bleeding, for example – are occasionally lethal.1 On the basis of the evidence from the systematic review we can estimate that, if 1,000 men took an aspirin a day for ten years, five of them would avoid a heart attack during that time, but three of them would have a major haemorrhage.
WHAT DOES ‘STATISTICALLY SIGNIFICANT’ MEAN?
‘To be honest, it’s a tricky idea. It can tell us if the difference between a drug and a placebo or between the life expectancies of two groups of people, for example, could be just down to chance . . . It means that a difference as large as the one observed is unlikely to have occurred by chance alone.
Statisticians use standard levels of “unlikely”. Commonly they use significant at the 5% level (sometimes written as p=0.05). In this case a difference is said to be ‘significant’ because it has a less than 1 in 20 probability of occurring if all that is going on is chance.’
Spiegelhalter D, quoted in: Making Sense of Statistics. 2010. www.senseaboutscience.org
OBTAINING LARGE ENOUGH NUMBERS IN FAIR TESTS OF TREATMENTS
Sometimes in tests of treatments it is possible to obtain large enough numbers from research done in one or two centres. However, to assess the impact of treatments on rare outcomes like death, it is usually necessary to invite patients in many centres, and often in many countries, to participate in research to obtain reliable evidence. For example, participation by 10,000 patients in 13 countries showed that steroid drugs given to people with serious brain injuries – a treatment which had been in use for over three decades – was lethal.2 In another fair test organized by the same research team, participation by 20,000 patients in 40 countries showed that an inexpensive drug called tranexamic acid reduces death from bleeding after injury.3 Because these studies had been designed to reduce biases as well as uncertainties resulting from the play of chance, they are exemplary fair tests, and provide good-quality evidence of great relevance to healthcare worldwide. Indeed, in a poll organized by the BMJ, the second of these randomized trials was voted the most important study of 2010.
The Figure below is based on data kindly provided by the award-winning team to illustrate how, to reduce the risks of being misled by the play of chance, it is important to base estimates of treatment effects on as much information as possible. The diamond at the bottom of the Figure represents the overall result of the trial of tranexamic acid. It shows that the drug reduces death from bleeding by nearly 30% (risk ratio just above 0.7). This overall result provides the most reliable estimate of the effect of this drug, even though the estimate from centres in Continent A suggests a less striking effect (which is not statistically significant, and likely to be an underestimate of the true effect) and the estimate from centres in the ‘Other continents’ category suggests a more striking effect (which is likely to be an overestimate).

Effects of tranexamic acid on death among trauma patients with significant haemorrhage, overall and by continent of participants (unpublished data from CRASH-2: Lancet 2010;376:23–32).
In rather the same way that the play of chance can be reduced by combining data from many centres in a multinational trial, the results from similar but separate studies can sometimes be combined statistically – a process known as ‘meta-analysis’ (see also Chapter 8). Although methods for meta-analysis were developed by statisticians over many years, it was not until the 1970s that they began to be applied more extensively, initially by social scientists in the USA and then by medical researchers. By the end of the 20th century, meta-analysis had become widely accepted as an important element of fair tests of treatments.
For example, five studies in five different countries were organized and funded separately to address an unanswered, 60-year-old question: in premature babies ‘What blood level of oxygen gives the greatest likelihood that babies will survive with no major disabilities?’ If the blood oxygen levels are too high, babies may be blinded; if too low, they may die or develop cerebral palsy. Because, even in these frail babies, the differences resulting from different levels of oxygen are likely to be modest, large numbers are required to detect them. So the research teams responsible for each of the five studies agreed to combine the evidence from their respective studies to provide a more reliable estimate than any one of their studies could provide individually.4
KEY POINT
- Account must be taken of ‘the play of chance’ by assessing the confidence that can be placed in the quality and quantity of evidence available
- Taking account of the play of chance - Testing TreatmentsTaking account of the play of chance - Testing Treatments
Your browsing activity is empty.
Activity recording is turned off.
See more...