This book is distributed under the terms of the Creative Commons Attribution-NonCommercial-NoDerivs License which permits noncommercial use and distribution provided the original author(s) and source are credited. (See https://creativecommons.org/licenses/by-nc-nd/4.0/
NCBI Bookshelf. A service of the National Library of Medicine, National Institutes of Health.
Structured Abstract
Background:
Although patient questionnaires are commonly used to assess health care experiences (eg, satisfaction with care), patient-reported outcome performance measures (PRO-PMs) assessing symptoms and physical functioning have not been part of quality assessment in oncology.
Objective:
To develop and test PRO-PMs for use during chemotherapy.
Methods
- Aim 1: We interviewed 124 stakeholders to identify key symptoms to test as PRO-PMs and potential risk adjustment variables.
- Aim 2: We conducted a structured literature review to identify prevalent symptoms. We combined the results from interviews and the literature review through expert consensus into a final list of symptoms. Then, we evaluated existing PRO-PMs assessing identified symptoms.
- Aim 3: We enrolled patients actively receiving chemotherapy at 6 cancer centers (in California, Connecticut, Florida, Minnesota, North Carolina, and Texas). Each patient completed PRO-PM items at home between 5 and 15 days after initiation of a therapy cycle.
Patients chose to complete the questionnaire online, by an automated telephone system, or on paper, and it was available in English, Spanish, and Mandarin. We defined feasibility as at least 75% of patients completing the PRO questionnaire. We developed practice-level PRO-PM specifications for each symptom individually (eg, proportion of patients at a practice with well-controlled symptom [eg, pain]) and for multisymptom summary measures. To account for variation in case mixes across cancer centers, we risk-adjusted every measure using hierarchical logistic regression models predicting the odds of high symptom burden, controlling for cancer type, age, sex, and race. For the multisymptom measures, optimal cutoffs were identified as those that maximized validity (correlation with physical functioning) and reliability (ability to accurately differentiate performance across providers) while remaining clinically actionable.
Results:
Interviews in aim 1 included patients with cancer in active treatment (n = 56); primary caregivers (n = 21); patient investigators (n = 5); clinicians without clinic administrative responsibilities (n = 11); health care administrators (n = 16, of whom 12 were also clinicians); and national experts, including clinicians (n = 15, of whom 5 were also clinicians). We recruited patients, caregivers, clinicians, and health care administrators from the 6 cancer centers and identified experts nationally. Among patients with cancer, 48% were women, 34% were aged ≥65 years, 14% were Black/African American, 8% were Asian, and 20% had a high school education or less. Common cancer types included genitourinary (32%), gastrointestinal (27%), breast (21%), and lung (20%). Caregiver relationships were typically a spouse, partner, or adult child.
In aim 2, we combined a literature review with interview results from aim 1 to refine a final list of symptoms to test, as follows: gastrointestinal symptoms (nausea, vomiting, constipation, diarrhea), sleep issues, depression, anxiety, pain, neuropathy, and shortness of breath. Although fatigue and appetite loss were acknowledged by the stakeholders as being important, we eliminated these symptoms because they are not sufficiently clinically treatable. Stakeholder recommendations for risk adjustment variables to test empirically included insurance status, cancer type, age, sex, and difficulty paying bills.
In aim 3, a total of 653 patients enrolled, of whom 607 completed the questionnaire (93%). Specifically, 470 of 607 (77%) completed the PRO questionnaire without a reminder call, and another 14% completed it after a staff member called. Most (>95%) participants found the PRO questions to be easy to understand and complete. When questionnaires were aggregated to the cancer center level, 1 cancer center descriptively appeared to perform better than others across measures, and 1 appeared to perform relatively worse, with the other 4 sites grouped similarly. Adjusting for cancer type and age had a modest effect on site-level scores. Adjustment variables for insurance status, sex, and difficulty paying bills were not significant in models and were thus removed. Empirical testing showed that combining the individual PRO-PM items for pain, nausea, and diarrhea was possible, with an optimal cutoff score of 0 to 4 vs 5 to 12 and with higher scores indicating greater symptom burden. The optimal cutoff scores for the 10-item measure were 0 to 14 vs 15 to 40. Summary PRO-PMs numerically differentiated between cancer centers but did not meet the recommended reliability threshold.
Conclusions:
Patients, caregivers, clinicians, national experts, and other stakeholders agree that performance measures based on how patients feel and function would be an important addition for payers to use in evaluating the quality of care provided by health care systems and provider groups. PRO-PMs can be feasibly captured at home during systemic therapy and are acceptable to patients. PRO-PMs may add meaningful information to evaluate quality of care, but more data are needed to establish the reliability and validity of these PRO-PMs before adopting them into performance-based oncology payment models.
Limitations:
Testing occurred at 6 sites among more than 600 patients, and further testing would strengthen generalizability. Additional data are also needed to meet recommended reliability thresholds. Such testing is planned.
Background
Performance measures are standardized measures of clinical performance in health care settings.1 They are widely used in oncology care settings for benchmarking and quality improvement and to guide payments.2-5 Conventional performance measures assess outcomes such as emergency department visits and patient experiences of care (eg, satisfaction with care).3-5 A notable gap is the oncology patient's perspective of symptom burden, quality of life (QOL), and physical function, which are measured with the gold standard of patient-reported outcomes (PROs).6
PRO-performance measures (PRO-PMs) is the standard terminology coined by the National Quality Forum (NQF) in a series of national meetings.7 These meetings were summarized in an article in the Journal of the American Medical Association that the principal investigator for this study and colleagues published in 2013.7 From that work came a book written by several other study team participants8; it noted that although questionnaires exist to measure processes of care, only rarely are PROs assessing symptoms or functioning used as measures of quality.
PRO-PMs7-9 are in use in some medical specialties in the United States (eg, orthopedics)10 but are nascent in oncology.7-9 Organizations that prioritize or endorse quality measures, such as the American Society of Clinical Oncology (ASCO),7,9 Centers for Medicare & Medicaid Services (CMS),10 NQF,2,11 and National Committee for Quality Assurance,12 have signaled their interest in using PRO-PMs in oncology. CMS has also proposed an alternative payment model emphasizing health outcomes assessed with PRO-PMs, called the Oncology Care First Model.13
A natural starting point for developing PRO-PMs is in systemic treatments for cancer, such as chemotherapy, given the high symptom burden14,15 and existing national clinical practice guidelines.16,17 Multiple members of our study team were active in an ASCO workgroup tasked with developing a framework for PRO-PMs. The ASCO PRO-PM framework describes 6 key characteristics: (1) meaningfulness in a given population, (2) prevalence, (3) capability of being measured with PROs, (4) clinical treatability, (5) availability of national clinical practice guidelines for managing the symptom(s) of interest, and (6) heterogeneity across practices in management of the symptom(s).7,18
Table 1 describes each characteristic and gives examples related to 1 potential PRO-PM of interest, cancer-related pain. For instance, pain is already well established as being meaningful to adults with cancer and clinicians.11,12,19-21 Pain is important for outcomes such as survival,22,23 and it has a high prevalence rate19,20 and validated and reliable PROs.24,25 In addition, clinicians can take action to reduce pain in their patients,22,26 and national guidelines exist for treating pain.16,17 Finally, pain management varies across practices and health systems.27,28
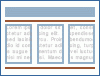
Table 1
Key Characteristics of PRO-PMs and Examples.
In the current study, we built on ASCO's PRO-PM framework7,9 with a mixed-methods study that provided us the opportunity to overcome several extant methodologic challenges. First, it is not well established how best to identify outcomes appropriate for a patient-centered approach to quality assessment based on meaningfulness to patients and clinical treatability.7-9 Second, no standards exist to inform how PRO measurement at the practice level should be adjusted for the differing characteristics of patients (risk adjustment).7-9 Finally, approaches for collecting and reporting performance measure information directly from patients at home has not been established (eg, infrastructure, storage).8 This study addressed all these challenges with qualitative and quantitative work, with a focus on addressing practical questions to support broad implementation and dissemination.
Specific Aims
Our study had 3 specific aims:
- Aim 1: Identify areas of cancer care delivery that are important to patients and other stakeholders when considering quality of care and that are amenable to performance evaluation with PRO measures (eg, symptom burden, physical functioning).
- Aim 2: Identify and evaluate existing PRO questionnaires that assess areas of cancer care delivery identified by stakeholders in aim 1, through a systematic literature search and multidisciplinary review, including the consensus of national stakeholder organizations.
- Aim 3: Conduct testing of identified PRO measures in representative community and academic oncology practice settings to refine the following:
- –
Feasibility of collecting PRO-PMs from patients at home (1-time assessment)
- –
Acceptability of PRO-PMs to patients
- –
Analytic techniques to detect differences at the practice level
- –
Risk adjustment for PRO-PMs
Overall Study Outcomes
The primary study outcome was a set of rigorously selected and tested PRO quality measures for adult oncology that clinical care practices, national quality organizations, and professional societies can all use.
The methodologic outcomes of our study included a discrete, systematic approach to identifying and testing PRO quality measures and risk adjustment variables. Moreover, this work delineated a foundational methodology that can be tested in other chronic health conditions. For instance, we designed our methods for adjusting PRO quality measures (based on patient-level demographic characteristics) to promote fair quality comparisons across oncology practices to be adaptable to other health conditions.
This study had 15 milestones, and all were met (see Appendix A). The results for aim 1 and part of aim 3 were published in JCO Oncology Practice.30 Aim 2 and the remaining results from aim 3 are presented in separate manuscripts in progress.
Participation of Patients and Other Stakeholders
Our multidisciplinary team included patient advocate organizations, clinicians, health care administrators, and state and national stakeholders. The coordinating center was at the University of North Carolina at Chapel Hill (UNC-CH), and the authors of this report comprised the main study team.
The green circle in the middle of Figure 1 represents the 5 patient investigators who served on this study. Of these 5 patient investigators, 2 were in active cancer treatment at the time of the study but have passed away (see the Acknowledgments). Three were former cancer patients from national, nonprofit patient advocate organizations (see author list): (1) the Cancer Information and Support Network (https://cisncancer.org/); (2) Patient Care Partners, LLC (patientcarepartners.org); and (3) Research Advocacy Network (https://researchadvocacy.org/). The patient investigators provided expert input on their lived experiences during cancer treatment and served on the study advisory board.

Figure 1
Multidisciplinary Team and Study Advisory Board.
We engaged the patient investigators throughout the study in a variety of functions that ensured meaningfulness, comprehension, and minimal patient burden of research activities. Patient investigators also aided in interpreting and disseminating the results. The patient investigator roles for each aim are described in Table 2.
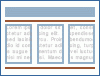
Table 2
Patient Investigator Roles by Aim.
Study Advisory Board
The study advisory board consisted of a multidisciplinary team comprising 18 state and national stakeholders, clinicians, health care administrators, and national experts (see Table 3). We chose study advisory board members for their expertise in quality measure development, clinical care, PRO measures, and lived experiences of cancer treatment and symptoms. We convened the study advisory board quarterly throughout the study via a web-based meeting.
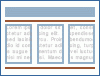
Table 3
Study Advisory Board and Expertise Areas.
Aim 1: Identify Key Areas of Cancer Care Delivery
Overview
In aim 1, we conducted interviews for 2 purposes. The first was to identify areas of cancer care delivery that are important to patients and other stakeholders when considering quality of care. The second was to identify potential risk adjustment variables to test in aim 3. Table 4 describes the overview of methods and results for aim 1.
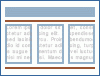
Table 4
Aim 1: Overview of Methods and Results.
Methods
Recruitment Sites
Six cancer centers in California, Connecticut, Florida, Minnesota, North Carolina, and Texas participated. We chose the recruitment sites to represent different regions of the United States, given variation in care quality across the country24,25 and diverse demographic and clinical characteristics of patients with cancer. Three cancer centers were affiliated with academic medical centers, and 3 were community based. The IRBs at each cancer center approved the study. To protect the anonymity of the cancer centers, we report the results for centers identified only by numbers.
Interviews
We conducted 124 interviews to determine priority symptoms and risk adjustment variables for PRO-PMs. Participants were aged ≥21 years and spoke English. We recruited participants via several methods. Site principal investigators sent emails to medical oncologists, nurses, administrators, and national experts at their cancer centers. We also invited national experts in performance measures, PROs, or cancer care delivery to participate in an interview and serve on the study advisory board (see Table 3).
We purposively sampled patients from 6 cancer centers; this approach is a gold standard qualitative research technique involving strategic choices about which individuals to include in a study.31 In qualitative research, the purpose is to maximize the variety of responses rather than to establish generalizable samples as in quantitative research.31 Our goal was to recruit at least 20% of total patients (summed across cancer centers) who were aged ≥65 years, had a racial or ethnic minority heritage, or had a high school education or less. Prior research has shown that at-risk groups may respond in different ways (for example with higher mean scores for pain or other symptoms depending on race or education level) or may have more difficulty understanding health-related questionnaires32,33 and that they are at greater risk of poor cancer outcomes.34
Caregiver inclusion criteria were adults with self-reported primary caregiving responsibilities for a chemotherapy patient receiving care at a recruitment site. Caregivers did not have to be linked to a patient participating in an interview. Patients and caregivers completed standardized items on age, sex, race and ethnicity, education, and cancer type, as tested in prior studies.35,36
Interview guides and procedures
Interview guides were informed by a literature review7,8,11,12,19,20,37-39 and tailored to 3 main groups: (1) patients with cancer in active treatment at a participating cancer center, caregivers, and patient investigators; (2) clinicians; and (3) health care administrators and national experts. Semistructured interview guides (see Appendix B) elicited recommendations for priority symptoms to test as PRO-PMs, optimal timing to administer a PRO questionnaire at home during systemic therapy, and patient characteristics they would recommend using for risk adjustment (eg, patient age). We also asked stakeholders to describe what high-quality care meant to them and the potential barriers and benefits to PRO-PMs.
We conducted these interviews by telephone and audio-recorded them. Consistent with standard methodological best practices,31 we continued interviewing until conceptual saturation was reached within each group (ie, no new ideas emerged). Interviews were transcribed verbatim.
Transcript coding
We coded transcripts in Atlas.ti with 3 coding teams using a common codebook.31 We developed the codebook based on recommendations by the study advisory board, initial readings of transcripts by coders and the research team, and codes for emerging or new themes. Coders pilot tested the initial codebook by independently coding 2 transcripts from interviews with patients and health professionals and comparing them with coding done by research team members. We revised a few concept definitions and applied the enhanced version of the codebook to the remaining transcripts. We reconciled coding discrepancies by consensus. Research team members and study advisory board members reviewed summary reports of the themes discussed by interview participants. This process led to a final set of symptom domains and risk adjustment variables to test.
Results
Description of Interview Participants
As described earlier, we had 3 sets of interview groups. One group included patients in active treatment for cancer and receiving chemotherapy, primary caregivers from participating cancer centers, and patient advocates who were previously treated for cancer. The second group involved clinicians from participating sites, and the third group included health care administrators from participating sites and national experts in performance metrics, PROs, and cancer care delivery from our study advisory board (see Table 3). The health care administrator and national expert groups also included physicians and nurses. Interview participants in aim 1 included patients with cancer in active treatment (n = 56) and primary caregivers (n = 21) at a participating cancer center; patient investigators (n = 5); clinicians without clinic administrative responsibilities (n = 11); health care administrators (n = 16, of whom 12 were also clinicians); and national experts, including clinicians (n = 15, of whom 5 were also clinicians). We present the results for each group below and discuss comparisons between groups at the end of the section.
Patient and Caregiver Recommendations
Our purposive sampling targets for patients with cancer receiving chemotherapy met or exceeded 20% representation for older age (ie, ≥65 years), ethnic and racial minority heritage, and low education (high school or less) (summed across sites). Table 5 shows the demographic characteristics of the patients and caregivers interviewed. The caregiver relationships were typically a partner or an adult child.
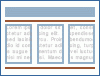
Table 5
Demographic Characteristics of Patient and Caregiver Interviewees.
Table 6 shows that patients and caregivers recommended the inclusion of key symptoms, including emotional distress, physical function, pain, and nausea. They also advised us that cancer centers should continue to collect care experiences, such as communication and information provision.
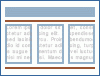
Table 6
Priority Symptom Domains Identified by Patient and Caregiver Interviews.
As documented in Table 7, patients and caregivers reported that PRO-PMs would be helpful to understand symptom burden in relation to the burden that other patients with cancer face. They also discussed the possibility of choosing a treatment or infusion center based on PRO-PM results, but they are limited based on geography or distance and availability in their area, financial constraints, or insurance company guidelines.
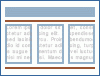
Table 7
Patient and Caregiver Perceptions of Benefits and Barriers to PRO-PMs.
Clinician Recommendations
Clinicians (n = 11) were medical oncologists and nurses at the 6 recruitment sites who did not have administrative responsibilities at their clinic. We separated clinicians with and without administrative responsibilities into separate subgroups because they may have different perspectives on PRO-PMs given their different responsibilities. Table 8 shows the symptom domains recommended by clinicians who did not have administrative responsibilities at their clinic: toxicities, fever, constipation, nausea, neuropathy, depression, and physical function. Clinicians suggested a dual-purpose approach in which individual-level PROs are used at the point of care to improve communication about symptoms among clinicians and patients during visits and then aggregated to the clinic level for use as PRO-PMs. They also expressed interest in assessing patients' preferences for symptom management and whether the care team was meeting those expectations.
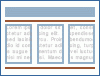
Table 8
Priority Symptom Domains Identified by Clinician Interviews.
Table 9 shows clinician perceptions of benefits from and barriers to PRO-PMs. Clinicians noted that PRO-PMs could help practices learn from the successes of colleagues and reveal when system-wide improvements are needed. For barriers, clinician themes were validity and relevance.
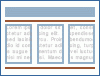
Table 9
Clinician Perceptions of Benefits and Barriers to PRO-PMs.
Health Care Administrator and National Expert Recommendations
Health care administrators (n = 16) reported their roles to be medical directors, nursing leaders, and quality officers. Their educational backgrounds included 7 medical doctors (MDs), 5 registered nurses (RNs; 3 also had PhDs), and 4 bachelor's- or master's-level executives. National experts (n = 15) included 9 with PhDs, 3 MDs, 2 RNs, and 1 master's-level scientist. Table 10 shows the priority symptom domains that these health care administrators and national experts identified.
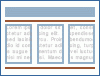
Table 10
Priority Symptom Domains Identified by Health Care Administrator and National Expert Interviews.
Table 11 shows that health care administrators and national experts perceived that PRO-PMs may encourage competition to increase symptom control rates. They also thought that PRO-PMs could enhance their understanding of care costs and help improve care. Perceived barriers included validity and reliability of PRO-PMs and risk adjustment variables, information overload, liability, potential for staff to dismiss PRO-PM data, and lack of funding for implementation and sustainability. National experts discussed topics that were similar to those of the administrators. They expressed concerns, however, about what a meaningful difference between practices would be for PRO-PMs.
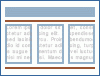
Table 11
Health Care Administrator and National Expert Perceptions of Benefits and Barriers.
Aim 2: Conduct 2 Literature Reviews
Overview
In aim 2, we identified and evaluated existing PRO questionnaires that assess areas of cancer care delivery identified by stakeholders in aim 1 using a systematic literature search and multidisciplinary review. For this aim, we conducted 2 reviews to bolster the findings from the interviews and to ensure that our results were generalizable to the nationwide cancer population and other stakeholders. Table 12 shows the overview for aim 2.
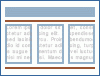
Table 12
Aim 2: Overview of Methods and Results.
Review 1: Aspects of Care Delivery and Quality of Care
Methods
To conduct the first review, we followed typical systematic review methodology, which is consistent with PCORI systematic review standards, Institute of Medicine (now the National Academy of Medicine) standards,47 and Preferred Reporting Items for Systematic Reviews and Meta-Analyses (PRISMA) reporting criteria.48 A health sciences librarian at UNC-CH who has expertise in cancer systematic reviews conducted the systematic searches.
Steps included deciding which databases to search (MEDLINE/PubMed, EMBASE, and the Cochrane Library), identifying search strings that were appropriate for each database, and importing titles and abstracts into a reference manager for coding. The literature search included Medical Subject Headings and Emtree headings and related text and keyword searches when appropriate. The research team and study advisory board reviewed the search strings generated by the health science librarian for potential additions. Searches were limited to English-language studies, with no restrictions for publication year. Appendix C shows the search strings used for the first review.
Studies were eligible for inclusion if they involved any stakeholder group's perception of what constitutes high-quality care for cancer and chemotherapy. Stakeholders included patients with cancer receiving chemotherapy, caregivers, clinicians, health care leaders, and health services researchers. We excluded studies that used pediatric or adolescent populations or that examined the efficacy of treatment regimens or comparative effectiveness without mention of perceptions of high-quality care. Titles and abstracts in a language other than English were included if a translation in English was available.
Coders participated in a training round in which all coders and a senior member of the review team independently coded 20 abstracts and titles. Interrater reliability was calculated with Krippendorff α using SAS statistical software (SAS Institute Inc) with the KALPHA macro49 (distribution obtained with bootstrapping). Krippendorff α is recommended over κ in cases where the codes are nominal (retain or reject abstracts being considered for inclusion in the review) and more than 2 coders are involved.49 Krippendorff α scores exceeded 0.70, the minimum value49,50 (Krippendorff α = .72; 95% CI, 0.59-0.84). In other words, coders agreed nearly three-quarters of the time during the training round. Discrepancies were discussed and resolved by consensus.
Abstract coding was then conducted in stages by 3 coders using Covidence software (https://www.covidence.org/). First, 2 coders independently coded article titles based on predetermined criteria (eg, topic is related to care quality and delivery in cancer). We excluded titles coded as “not relevant” by both coders and retained the other titles. For retained titles, we double-coded the abstracts. Abstracts coded as “not relevant” by both coders were excluded; retained abstracts underwent a full-text review. Articles with disagreements were coded by a third coder, until the retained articles were all coded as relevant. Full-text articles were screened by 2 coders against the eligibility criteria using the PRISMA guidelines.48
Systematic searches and manual searching yielded 1813 unique articles. Of these, 1310 articles were coded as irrelevant during the title and abstract review, and another 432 articles were discarded during full-text review, leaving 36 articles for extraction. Figure 2 shows the flow diagram.

Figure 2
Flow Diagram for Systematic Review.
Results
The patient perspective was represented in nearly all studies. Six studies did not include patients and instead examined the perspectives of administrators, policy experts, oncology social workers, nurses, and researchers. Most studies included cross-sectional interviews, and 2 studies provided longitudinal analyses.
The review articles showed quality-of-care indicators that were important to patients and caregivers included symptom management, psychosocial care (depression, anxiety, distress), and maintaining physical function and daily activities. Key quality-of-care indicators for clinicians and health care administrators included patient psychosocial care (eg, stress, anxiety) and physical symptoms (eg, fatigue, sleep). These findings were consistent with recommendations from patients with cancer, clinicians, and others in aim 1. Table 13 shows the number of articles per domain.
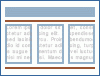
Table 13
Articles Per Symptom Domain.
Barriers to quality care from the patient perspective included lack of psychosocial care, delays in care, and experiences of care (obtaining and understanding health information, lack of coordinated care, and billing issues).51-53 Quality-of-care barrier themes for providers and care teams extracted from review articles included a wide array of issues: workload or administrative burden for reporting performance measures, lack of coordinated care, bureaucracy of managed care, lack of processes to support treatment guidelines, and concerns about the strategies being implemented by managed care to address cancer care quality (eg, decision support tools, pathways, guidelines, and cost reduction strategies).51-54 These issues were also consistent with the interview results from aim 1.
Review 2: Identify and Evaluate Existing PROs for Use in Oncology PRO-PMs
We identified and evaluated existing PRO measures in steps, consistent with standard COSMIN (COnsensus-based Standards for the selection of health Measurement INstruments) methodology.40-42 COSMIN involves 4 steps: (1) addressing conceptual considerations, (2) finding existing PRO instruments, (3) assessing the quality of PRO measures, and (4) making recommendations on the selection of PROs.40-42
In all steps, inclusion criteria for scales to assess the suitability of the PRO questionnaires for our purposes included the following: psychometric properties, evidence of validity and reliability in patients with cancer, PROs developed with patient input, applicability to systemic cancer care, and public availability without licensing fees or permissions. PROs could be specific to cancer care or used across health conditions if they had evidence of reliability and validity in patients with cancer. Two raters independently scored scales using a template, and discrepancies were resolved with consensus with a third reviewer from the study team and the study advisory board. Multisymptom scales or item banks that had been calibrated using item response theory (IRT) were preferred because individual items could be selected not only for this PRO-PM development project, but also by future groups and health systems considering using PRO-PMs. Table 14 shows the systematic reviews we reviewed for scales or item banks assessing multiple symptoms.
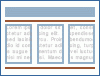
Table 14
Systematic Reviews for Multisymptom Scales in Cancer.
Multisymptom PRO Measures
Instruments varied by psychometric properties, item content, recall period (time frame considered to answer the question, for example, in the past week), dimensionality (whether the scale measured 1 construct or ≥2), and intended study population. Table 15 shows 6 commonly used item banks from the reviews. Table 16 shows the number of PRO measures reviewed per symptom domain.
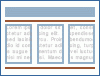
Table 15
Comparison of Common PRO-PM Item Banks Assessing Multiple Symptoms.
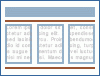
Table 16
Number of PRO Measures Reviewed, by Symptom Domain.
Selected PRO Measures
Following a review of individual PRO measures, 2 multisymptom item libraries met inclusion criteria: the National Cancer Institute's (NCI's) PRO Common Terminology Criteria for Adverse Events (PRO-CTCAE)29,44 and NIH's PROMIS.45,46 The PRO-CTCAE measurement system was developed to capture symptomatic adverse events (AEs) from the patient perspective as an addition to the clinician-reported CTCAE. The PRO-CTCAE was calibrated using IRT,29,44 which provides a statistically rigorous approach for selecting individual items or (fixed-item) instruments that have desirable psychometric characteristics. Users can select up to 124 individual items representing 78 symptom toxicities. The PRO-CTCAE lacks a physical function item, so we supplemented it with the PROMIS Global Health physical function item46 and the physical function item from the Patient-Generated Subjective Global Assessment,62 which is a version of the Eastern Cooperative Oncology Group (ECOG) performance status measurement.
PROMIS is an NIH Roadmap Initiative to develop self-report measures of global, physical, mental, and social health for adults and children in the general population and those living with a chronic condition.45,46 Similar to the PRO-CTCAE described previously, PROMIS item banks are calibrated using IRT, which is a family of modern statistical models enabling the selection of items that have desirable psychometric characteristics. PROMIS item banks have fixed-item short forms assessing more than 50 symptom domains that cut across health conditions. Cancer-specific calibrations and reliability and validity work have been conducted for PROMIS item banks at the patient level.44,45,60 Aim 3 of this project extends this work by testing the reliability and validity of individual and summary items at the practice level for the purposes of comparing PRO-PMs on quality of care.
Performance Measure Specifications
We generated performance measure specifications for each selected item (Table 17). For example, a pain item from the PRO-CTCAE is, “In the last 7 days, what was the severity of your pain at its worst? (none, mild, moderate, severe, very severe).”44 The corresponding PRO-PM specification for high-quality care was “proportion of adult patients in a participating cancer center receiving systemic cancer therapy whose pain severity rating was none or mild during days 5 to 15 of cycle.” Thresholds for high-quality cancer care are noted in Table 17. These thresholds were determined with multistakeholder input from our study advisory board, including patients and clinicians. The thresholds are also consistent with a state-level quality measurement initiative called MN Community Measurement (MNCM).74 The items in Table 17 were tested in aim 3 (described in next section).
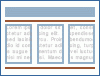
Table 17
Performance Measure Specifications.
Aim 3: Testing at 6 Cancer Centers
Overview
In aim 3, we tested identified PRO-PMs in representative community and academic oncology practice settings to determine (1) the feasibility of collecting a 1-time PRO-PM from patients at home; (2) the acceptability of PRO-PMs to patients with cancer; (3) analytic techniques to detect differences in measure scores across practices; and (4) potential risk adjustment variables for PRO-PMs. Table 18 shows the overview for aim 3.
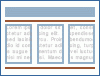
Table 18
Aim 3: Overview of Methods and Results.
Methods
Recruitment Sites
The same 6 cancer centers from aim 1 recruited patients with cancer receiving chemotherapy for aim 3. The cancer centers were located in California, Connecticut, Florida, Minnesota, North Carolina, and Texas. We chose the recruitment sites to represent different regions of the United States, given the variation in care quality across the country24,25 and the diverse demographic and clinical characteristics of patients with cancer. Three cancer centers were affiliated with academic medical centers, and 3 were community based. IRBs at each cancer center approved the study. To protect the anonymity of the cancer centers, we report the results for centers identified only by numbers.
Patient Inclusion and Exclusion Criteria
Adults aged ≥21 years receiving systemic chemotherapy, immunotherapy, or targeted therapy (nonhormonal) for any type of cancer at the 6 recruitment sites gave informed consent. Participants needed to write and speak English, Spanish, or Mandarin. Exclusions were inability to provide consent or ongoing participation in a clinical trial of an investigational drug(s). We purposefully used as few exclusion criteria as possible to increase generalizability to routine care settings.
At enrollment, patients with cancer chose their preferred mode to complete the PRO questionnaire, via either the web or automated phone system (interactive voice response [IVR]); we provided a brief tutorial. Patients also selected their preferred language (English, Spanish, or Mandarin). We administered the PRO questionnaire once and gave participants a $20 gift card.
PRO-PM Items
Items were loaded into an electronic system securely housed at UNC-CH called PRO Core.53 PRO Core enabled patients to complete a PRO questionnaire at home via web or IVR. Patients completed standardized items on demographics, insurance type(s), difficulty paying bills, and computer use as potential risk adjustment variables, as tested in prior studies.35,36 Difficulty paying bills was assessed at baseline with the item, “How difficult is it for you/your family to meet monthly payments on bills?” (not at all, not very, somewhat, very, extremely). Computer use was assessed at baseline with the item, “How often do you use a computer, tablet, or smartphone?” (never, once a week or less, several times a week, daily). Patients also completed acceptability items assessing comprehensibility and ease of use.35,36 The total number of items ranged from 32 to 36, depending on skip patterns.
Completing the PRO Questionnaire at Home
At enrollment, we explained to patients with cancer about completing the PRO questionnaire at home on days 5 to 15 of the treatment cycle. We chose this time frame based on interview recommendations from aim 1 and reviews showing that symptoms are commonly experienced during this time frame.75 For each participant, we identified a treatment cycle after which they would complete their PRO questionnaire. Patients were commonly recruited in infusion centers, and thus their current cycle was typically used. This cycle could be at initiation of a new treatment regimen or during an existing regimen. The timing of the PRO questionnaire was approximately midcycle of a typical chemotherapy regimen when symptoms are common.75
Starting on day 5 following initiation of the cycle, participants received an automated electronic prompt (via either email or IVR) to complete the PRO questionnaire. The email prompt provided a web link to the questionnaire; IVR was an automated call to the patient. For patients who preferred not to complete questions electronically, we offered paper questionnaires. Participants received a daily electronic prompt until either day 9 of the cycle or until the questionnaire was completed. If patients did not self-complete the questionnaire by day 10 posttreatment, they received a staff reminder call (from their treating center) encouraging them to log in or offering to administer the questionnaire verbally by interview.
Medical Record Abstraction
We used medical record abstraction to collect clinical risk adjustment variables: cancer type, comorbid conditions, insurance type(s), oral or intravenous chemotherapy, drug regimen and emetic risk, and whether chemotherapy was curative or palliative. Sites raised concerns that cancer stage information would be difficult to obtain; thus, we collected information from the electronic health record on a variable for curative or palliative chemotherapy as a proxy.
Variables for a Missed PRO, Feasibility, and Acceptability to Patients With Cancer
We defined a “missed PRO” as patients not completing their PRO questionnaire by day 15. We defined feasibility as at least 75% of patients completing their PRO questionnaire. We defined acceptability as at least 75% of patients reporting moderate to high acceptability.87
Development of Individual Item and Summary Measure PRO-PMs
We used PRO-CTCAE29,44 and PROMIS45,46 items to develop individual item PRO-PMs for the following symptoms: pain severity, nausea severity, numbness severity, dyspnea severity, vomiting frequency, diarrhea frequency, fatigue severity, constipation severity, anxiety severity, depression severity, insomnia severity, and loss of appetite frequency. Each item was scored from 0 to 4, with 0 indicating no symptom present and 4 being the most severe or frequent.
We created 3- and 10-item summary measures by combining scores from individual items. The 3-item summary measure combined scores from questions about nausea, diarrhea, and pain, and the scores ranged from 0 to 12. The 3 items were chosen based on stakeholder perceptions of high perceived actionability during chemotherapy. These 3 items are also used by a state-level quality measurement initiative called MNCM.74 The 10-item summary measure included all individual items except loss of appetite and fatigue; scores ranged from 0 to 40. Fatigue and loss of appetite items were excluded because a multistakeholder consensus process determined that they have low clinical treatability and therefore are not appropriate for evaluating the quality of care delivered by a provider or clinic.
Calculation of Site-Level PRO Scores
We calculated site-level PRO scores by dividing the number of patients at a site who reported having the PRO symptom (numerator) by the number of patients at a site who completed a PRO questionnaire (denominator). The criteria for meeting the numerator specification varied by measure.
For the individual items, the cutoff for dichotomizing the measure was between 0 and 1 (none or mild symptoms) and 2 and 4 (moderate to severe symptoms), in concordance with current practice for these items.44 For the summary items, no established clinical threshold is available for dichotomization. Therefore, we used a data-driven approach to identify the cutoffs by comparing scientific measure properties at each possible cutoff.
Risk Adjusting Site-Level PRO Scores
To account for variation in case mix that may influence site rankings irrespective of true care quality at each of the sites, we risk-adjusted the site-level scores. This helps account for factors that varied across sites and were beyond a provider's control. Risk-adjusted site-level scores were estimated using an observed-to-expected ratio approach. These ratios were rescaled using the average site-level performance. This is a practical method commonly used for adjusting measures when evaluating provider performance.76
To calculate observed scores, we summed the number of patients at each site who had high symptom burden and divided by the total number of patients at each site who completed a questionnaire. For expected scores, we calculated the average of the expected probability of high symptom burden for all patients who completed a questionnaire at the site. These expected probabilities were derived from a hierarchical logistic regression model (SAS PROC GLIMMIX), with odds of high symptom burden as the dependent variable. Risk adjustment variables included cancer type, age, sex, and race. Cancer type was defined using a scheme of 5 cancer groupings: (1) breast/gynecologic, (2) gastrointestinal/colorectal, (3) genitourinary, (4) thoracic/head and neck, and (5) other cancers (including solid tumors and hematologic malignancies). Because of the limited number of patients in the data and the relatively large number of dummy variables needed to account for cancer type, we sought to avoid overfitting the model and selected a parsimonious set of predictors. Age was dichotomized at ≥65 years vs <65 years, sex was categorized as female vs male, and race was categorized as non-Hispanic White vs all other races and ethnicities. Although many sites had sufficient numbers of patients of color or patients with Hispanic ethnicity, the lack of diversity in some sites impeded our ability to model variables for race and ethnicity at a more detailed level than White vs all other races and ethnicities. These variables were included in the model as fixed effects. The influence of patient clustering within each site on SEs was accounted for using a site-specific random intercept. To avoid overshrinkage of the expected probabilities, we excluded the random intercept term from our calculation of the probability of high symptom burden (PROG GLIMMIX, option NOBLUP).77
Measure Properties: Reliability and Validity
To evaluate the potential for these risk-adjusted measures to be used as potential PRO-PMs, we evaluated 2 scientific measure properties: reliability and validity. Reliability in this context refers to the ability of a measure to identify the highest- and lowest-performing cancer centers. Validity refers to the ability of a measure to make correct conclusions about provider performance when measured at the group or system level. Optimal measures are both reliable and valid, indicating that they both identify high- and low-performing sites and that the conclusions on performance are accurate.
The reliability score assesses the extent to which a ranking of sites by score is a true indicator of relative performance (eg, the top-ranked site truly being the best performer and the bottom-ranked site being the worst performer). Importantly, reliability does not measure attainment of some optimal rate of care quality, but rather variation between sites. Indeed, reliability would be equally poor if all providers were performing well as if all providers were performing equally poorly. Relative performance is commonly used in alternative payment models, such as the proposed Oncology Care First Model and the existing Oncology Care Model,13 to determine some component of performance-based pay. For example, sites scoring in the top 20% across sites may receive a bonus, whereas sites scoring in the bottom 20% may incur a penalty.
For this project, reliability was estimated using signal-to-noise ratios.78 This method, endorsed by the NQF for measure development,79 calculates the site-to-site variance for a measure and divides the site-to-site variance by the total measure variance (site-to-site variance plus site-specific variance). Each variance component is estimated using the same hierarchical linear modeling technique used to calculate risk-adjusted scores, with the site-to-site variance derived from the variance of the random intercept term and site-specific variance the binomial variance using the risk-adjusted probability of symptom presence and number of attributed patients.78,80 Reliability scores were calculated for both adjusted and unadjusted measures.
The result of this calculation is a reliability score, ranging from 0 to 1, calculated for each site. The average of site-specific reliability scores is the reliability score for the given individual measure or cutoff for the summary measures. If a measure has high reliability scores, a site's ranking reflects that site's “true” performance. Lower reliability scores indicate that a site's ranking is because of random variation. Reliability can vary by measure, and for the summary measures, reliability can vary by cutoff, as this creates differing binary classifications for the dichotomized measure. A score of 0.7 is generally considered the minimum acceptable average score for a measure to be sufficiently reliable for use in provider profiling.79
We determined validity by calculating the Pearson correlation for site-level measure scores and the proportion of patients with low physical function. Low physical function, obtained from the same patient-reported surveys as the PRO measures, was operationalized as a score of ≥2 on the ECOG physical function.43 Clinical reasoning suggests that practices with a higher proportion of patients with burdensome symptoms will have a higher proportion of patients with low physical functioning.
Evaluating Measure Performance
Although reliability and validity are both essential for a measure to perform well in provider profiling, there often exists a tradeoff between the 2 components that should be considered when selecting PRO-PMs. For example, a measure with excellent reliability but poor validity may home in on variation between sites that is not explained by patient-level factors (reliability), but if this variation is not correlated with good clinical care (validity), the measure will serve as a poor indicator of clinical performance. To evaluate these tradeoffs, we developed a graphical method to compare the reliability and validity of our candidate individual items and summary measures. Additionally, we sought to exclude measures with very high or very low percentages of patients with symptoms (eg, close to 100% or 0%), as this pattern would create unstable estimates of symptom burden when measuring performance for sites with small numbers of attributed patients. Additionally, as variance for the binomial distribution shrinks when approaching 0 or 1, symptoms with very high or very low prevalence tend to result in a small range of performance scores across sites, reducing the ability to reliably differentiate between sites.
To compare these 3 dimensions, we created a bubble plot with reliability scores plotted on the x-axis and validity plotted on the y-axis (see “Graphical Comparison of PRO-PM” section). Different-sized bubbles based on the center point are determined by a given measure's reliability and validity, and the size represents the percentage of patients with the symptom. To determine bubble size and screen-out measures with high or low symptom prevalence, we calculated the difference between the percentage of patients with a given symptom and the nearest boundary. For example, if 20% of patients had a symptom, the distance between 20% and 0 is 0.2. If 90% of patients had a given symptom, the distance between 90% and the nearest boundary, 1, is 0.1. Symptoms with 50% prevalence have equal distance from the 2 boundaries. This number was used to adjust the size of bubbles representing distance from a boundary, such that the largest bubbles represented values closest to 50%, and the smallest bubbles represented values closest to either 0% or 100%. The best measures had both high validity and high reliability (coordinates closest to the top-right corner of the graph) as well as large bubbles indicating distance from a boundary. This graphical approach to comparing these 3 measures was used for all individual items as well as for the 3- and 10-item summary measures.
Additionally, for the summary measures, we sought to exclude potential cutoffs where the dichotomization cutoff for combined individual item scores was so low as to make it prone to random error. In aim 1 interviews, clinicians noted that cutoffs prone to random error would discourage health practitioners from trying to control symptoms. If, for example, a cutoff of 1 out of 12 was chosen for the 3-item summary measure, that would mean a patient with a mild symptom on any of the 3 items would be flagged as having a symptom. Additionally, a low cutoff would result in the same score whether a patient had the most severe symptom score across all 3 symptoms or had only 1 mild symptom. These are distinct clinical presentations, and clinical reasoning suggests that having measures that can differentiate between these scenarios is important. As such, we sought cutoffs across candidate summary measures that would capture information from multiple individual symptom items and represent a more holistic view of symptom burden.
Results
Descriptive Statistics: Sample
As shown in Figure 3, we approached 793 patients. Of these, 11 were ineligible and 129 were refusals. We enrolled 653 patients; of those, 607 completed a PRO-PM (93%).

Figure 3
Patient Flow Diagram.
Table 19 gives the demographic characteristics of the patient sample.
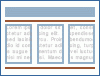
Table 19
Demographic Characteristics for Patients With Cancer by Treatment Site.
Table 20 shows the demographic differences by survey mode.
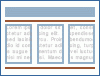
Table 20
Demographic Characteristics by Survey Mode.
Missing Data
Most enrolled patients (607/653 [93%]) completed the PRO-PM, indicating high feasibility for collecting a 1-time PRO questionnaire at home. As shown in Figure 3, 470 of 607 patients (77%) completed the PRO questionnaire without a reminder call. An additional 137 (23%) completed the questionnaire after a call directly from a staff member call (15% logged in and 8% completed questions during the reminder call), which minimized missing data. Patients who did not complete all survey items (n = 6) were dropped from quality measure assessment.
Acceptability to Patients With Cancer
Of these 607 patients, 439 patients (72%) completed PRO-PM items via web, 105 (17%) by paper, 52 (9%) by in-person or telephone interview, and 11 by IVR (2%). Few patients (5%) selected Spanish (n = 27) or Mandarin (n = 3). Patient acceptability was high. Of the 607 patients, 586 (96%) reported that PRO-PM items were easy/very easy to complete; 590 (97%) said PRO-PM items were easy/very easy to understand.
Descriptive Statistics: Individual and Summary Measures
Individual measures were dichotomized at response values 0 or 1 (none or mild symptoms) vs 2 to 4 (moderate to severe symptoms). The 3-item and 10-item summary measures do not have an a priori dichotomization point; therefore, the results include testing of all possible dichotomization points for the 2 summary measures evaluated in this project.
Table 21 shows that the lowest percentage of patients reported vomiting (4%); the symptom with the highest percentage (66%) was fatigue.
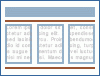
Table 21
Site-to-Site Variation for Unadjusted Symptom Frequency.
In addition to variation in symptom frequency across measures, symptom frequency varied across sites. For the unadjusted individual measures, site 4 descriptively tended to perform best by having the lowest percentage of patients reporting symptoms, whereas site 5 tended to perform worst (Figure 4).

Figure 4
Site-to-Site Differences in Symptom Frequency for Unadjusted Individual Measures.
When we evaluated reliability, defined as the ability to identify the highest and lowest performers (practices), for the individual items pain, fatigue, anxiety, sadness, and appetite loss, reliability varied little from site to site; the model to calculate the site-to-site variance did not converge. The most reliable measure was vomiting (yellow triangles in Figure 4), with a score of 0.774. However, it was the least prevalent symptom, which creates challenges for measure development. The second most reliable measure was insomnia (blue squares in Figure 4), with a prevalence of 42% and a reliability score of 0.485.
We also summed individual items to create 3- and 10-item summary measures. The summary measure scores demonstrated a wide range of scores across patients with cancer (Figure 5a and Figure 5b). For the 3-item summary score, the minimum score was 0 and the maximum score was 10. For the 10-item summary score, the minimum score was 0 and the maximum score was 29.

Figure 5a
Distribution of Patient Scores for the 3-Item Summary Measure.

Figure 5b
Distribution of Patient Scores for the 10-Item Summary Measure.
Graphical Comparison of PRO-PM
Risk adjustment substantially influenced site-level scores for most individual and summary measures (Appendix D). In general, the site-to-site variation in risk-adjusted scores was smaller than the site-to-site variation for the unadjusted scores. This suggests that variation in case mix (patient demographic and clinical characteristics) drives much of the observed variation in site-to-site scores. As a result, the reliability scores for the risk-adjusted measures were smaller than the reliability scores for unadjusted measures. Indeed, for many risk-adjusted measures, the site-to-site variation was so small that the algorithm used to estimate variance of the random intercepts could not converge, and variance was therefore estimated at 0.
Although the graphical comparison method for reliability and validity (Figure 6, Figure 7, and Figure 8) successfully differentiates between measures, the results show low mean reliability and validity scores (ie, close to the origin in the graph) across individual items and summary measures. The center of each bubble is the mean reliability and validity for a given measure, and the size corresponds to the distance between the nearest boundary and the average percentage of patients with the symptom, with larger bubbles indicating values closer to 50%.

Figure 6
Scatter Plot of Reliability and Validity for Risk-Adjusted Individual Symptom Items.

Figure 7
Scatter Plot of Reliability and Validity for the Risk-Adjusted 3-Item Summary Score.

Figure 8
Scatter Plot of Reliability and Validity for the Risk-Adjusted 10-Item Summary Measure.
For Figures 6, 7, and 8, reliability for nearly all measures did not meet the minimum threshold of 0.7, indicating limited ability for the measure to differentiate these sites based on performance. Validity was also low, with Pearson correlations between site-level symptom score and physical function score mostly below 0.5 for individual measures and the 3-item summary measures. The top PRO-PM candidates on each graph have been identified with a label; reliability, validity, and prevalence information is found in Appendix D.
To select optimal PRO-PMs using these graphs, we identified candidate measures with the highest reliability and validity scores and compared these reliability and validity values with the size of the bubble, representing the percentage of patients who had high symptom burden relative to a 0% or 100% boundary where larger bubbles had percentages farther from 0% and 100%. For Figure 6, although the vomiting PRO-PM item had the best reliability, the percentage of patients with a vomiting score of ≥2 was so low (4%) as to cause concerns estimating the expected number of patients with the symptom when counts of attributed patients are small. Additionally, the small percentage of patients creates challenges when differentiating across sites with scores close to the 0% boundary. Therefore, the insomnia measure was identified as the most optimal measure given its relatively high reliability, sufficient validity, and long distance from a 0% or 100% boundary.
The graphical approach also identified candidate measures for the 3-item and 10-item summary measures (Figure 7 and Figure 8). For the 3-item summary measure, 0-4 vs 5-12 was determined to be the best cutoff, with a reliability of 0.53 and a correlation with the physical functioning measure of 0.099. For the 10-item summary score, a cutoff of 0-3 vs 4-40 had greater reliability than did a cutoff of 0-14 vs 15-40, but we thought that the 0-3 vs 4-40 cutoff measure was too low for the measure to be clinically valid. With a cutoff this low, a patient with 4 mild symptoms would be flagged in the numerator as having low-quality care, even if no symptom was severe. Therefore, we identified the 0-14 vs 15-40 cutoff as the optimal cutoff for the 10-item summary measure, having a reliability of 0.497 and correlation with the physical functioning measure of 0.431. To create both a readable figure and allow the reader to explore reliability, validity, and boundary distance for each measure, we have included a full listing of these statistics in Appendix D.
Risk Adjustment Results
Risk adjustment variables (age, cancer type, sex, and race) had a modest effect on site scores for each of the 3 best-performing measures. Among the included risk adjustment variables, age consistently had the most effect on site scores (P values for type III effects were <.05 for all models). Cancer type grouping was significant for the 3-item summary measure (type III effects P = .003) and nearly significant for the 10-item summary measure (P = .0521). Sex and race were not significantly associated with any of the 3 tested PRO-PMs and thus were eliminated as potential risk adjustment variables.
Figure 9, Figure 10, and Figure 11 contain comparisons of observed (unadjusted) scores (blue circles) and risk-adjusted scores (red diamonds). Across these models, better performance is indicated by a risk-adjusted score below the observed score, and worse performance is indicated by the opposite. Appendix E contains the full statistical output for these risk adjustment models.

Figure 9
Observed and Risk-Adjusted Site Scores for Insomnia.

Figure 10
Observed and Risk-Adjusted Site Scores for the 3-Item Summary Measure.

Figure 11
Observed and Risk-Adjusted Site Scores for the 10-item Summary Measure.
When evaluating differences between observed and risk-adjusted scores for insomnia, we found that the scores for sites 2 and 4 worsened when risk-adjusted (2.6% and 1.7%, respectively), whereas the scores for sites 5 and 6 improved by decreasing their measured percentage of patients with symptoms (−2.7% and −2.1%, respectively). The scores for sites 1 and 3 remained virtually unchanged after risk adjustment (0% and −0.4%, respectively). Overall average scores changed by less than a percentage point.
The results were similar for the 3-item summary measure, with score increases for sites 2 and 4; decreases for sites 3, 5, and 6; and little change for site 1. For the 10-item long-form summary measure, site 6 had little change for risk adjustment, but scores for sites 1, 3, and 5 improved substantially (−1%, −2%, and −1.1%, respectively), whereas scores for sites 2 and 4 worsened (2.7% and 1.4%, respectively). Of note, before adjustment, site 2 scored 4% better than site 3 on the 10-item summary measure, but after adjustment, site 3 outperformed site 2 by 0.6%.
Discussion
Recap of Main Results
In aim 1, we conducted 124 interviews to identify key symptoms to test as PRO-PMs and identify potential risk adjustment variables. Interview participants included patients with cancer receiving chemotherapy, caregivers, clinicians, and health care administrators from participating sites; former patients with cancer who are now patient advocates; and national experts. Interview themes indicated that these groups perceived PRO-PMs to be acceptable, with benefits and barriers noted.
In aim 2, we conducted a structured literature review to identify prevalent symptoms. We combined the results from interviews and the literature review through expert consensus into a final list of symptoms. Prioritized symptoms related to gastrointestinal function (diarrhea, constipation, nausea, vomiting), depression, anxiety, pain, insomnia, fatigue, dyspnea, physical function, and neuropathy. These symptoms are prevalent, meaningful to patients with cancer, and clinically treatable. Although fatigue and loss of appetite were acknowledged by the stakeholders as important, we eliminated them because they are not sufficiently clinically treatable.
Then, we conducted a review and evaluation of existing PRO-PMs assessing identified symptoms. Preference was given to PRO-PMs that are publicly available multisymptom item libraries with reliability and validity evidence in patients with cancer. This standardized review yielded 2 multisymptom item banks to test in aim 3: namely, the PRO-CTCAE, developed by the NCI, and the PROMIS instrument, developed by the NIH.
In aim 3, across the 6 cancer centers, more than 600 patients completed the questionnaire (93% adherence). This finding shows that a 1-time PRO-PM can be feasibly captured at home during systemic therapy. Specifically, 470 of 607 patients (77%) completed the questionnaire without a reminder call and 14% completed it after a call from a staff member. On the survey, more than 95% of participants found the PRO questions to be easy to understand and complete.
Our results demonstrate the ability to score sites using PRO-PMs collected from individual patients with cancer and to aggregate results to the site level. Also feasible is combining individual measures to create summary PRO-PM items, to evaluate the reliability and validity of individual and summary items, and to apply risk adjustment to individual and summary items.
When questionnaires were aggregated to the cancer center level, 1 cancer center appeared descriptively to perform better than others across measures, and 1 appeared to perform relatively worse, with the other 4 sites grouped similarly. Although this is true for this time period and these cancer centers, the generalizability of these findings is uncertain. Adjusting for cancer type and age had a modest impact on site-level scores and site ordering and reduced reliability scores compared with unadjusted measures. Adjustment variables for insurance status, sex, and difficulty paying bills were not significant in models and were thus removed. Empirical testing showed that combining the individual PRO-PMs for pain, nausea, and diarrhea was possible, with an optimal cutoff score of 0-4 vs 5-12. When the 10 symptoms were combined into 1 composite PRO-PM, the optimal cutoff score was 0-14 vs 15-40. Summary PRO-PMs differentiated between cancer centers, but they did not meet the recommended reliability threshold, and the extent to which identified cutoffs can be generalized into a measure that could be considered for endorsement is unknown. Additionally, these cutoffs do not necessarily reflect clinically meaningful thresholds. More work is needed to validate these measures at the site level.
Results Related to Evidence in the Literature
This study adds to the literature in 3 main ways. First, using stakeholder engagement to prioritize domains for PRO-PMs in systemic therapy is an important advancement. Second, showing that a PRO-PM can be feasibly collected at home during a treatment cycle for the development of PRO-PMs documents welcome progress in the field. Finally, we conducted foundational work to develop and test PRO-PMs in oncology.
Stakeholder priority symptoms were pain, mental health, sleep, gastrointestinal symptoms, neuropathy, dyspnea, and physical function, which mostly overlap the NCI recommended symptoms to assess in clinical trials81 and a growing literature on recommended symptom sets.82,83 Physical function is not on NCI's list but was mentioned by interview participants, albeit with some reservations. Study advisory board members and interviewees raised concerns that physical function may not be a “fair” performance measure because it may be influenced by factors beyond treatment.84,85 Interview participants also recommended that cancer centers continue to collect patient experiences of the visit (eg, Consumer Assessment of Healthcare Providers and Symptoms [CAHPS] Cancer Care51), which is already common in quality programs.2,8 Thus, more than 1 type of outcome measure may be needed to adequately capture the patient's perception of the quality of care. Future research should determine optimal burden acceptable to patients for assessing their quality of care with PRO and patient experience measures.
Interview participants noted the potential benefits of PRO-PMs, mostly consistent with their health care role. Clinicians and administrators described how PRO-PMs could help practices learn what they are doing well and what improvements are needed for symptom control. Administrators thought that PRO-PMs might encourage competition and could enhance their understanding of care costs. Patients with cancer and their caregivers thought that understanding symptom burden in relation to other patients would be helpful. However, patients and caregivers speculated that they may not be able to choose a treatment center based on PRO-PM site scores because of insurance, geographic, or financial constraints.
Clinicians, administrators, and national experts had noted similar barriers: the validity and relevance of PRO-PMs. Clinicians at all sites mentioned that their patients with cancer are more at risk than patients at other institutions, and thus, training on how risk adjustment variables were empirically chosen and their function might increase transparency. Barriers unique to administrators were liability and lack of funding for implementing PRO questionnaires and PRO-PMs. National experts speculated that only a few benchmarks may show meaningful differences between practices.
Interview participants recommended collecting PRO data 5 to 15 days after the start of a treatment cycle when some symptoms related to therapy, such as nausea, might peak. Patients with cancer completed a 1-time PRO questionnaire so we could gauge initial acceptability and feasibility. Collecting PRO questionnaires at 1 time point is consistent with methodology used by MNCM.74 Future research is warranted to examine whether PRO questionnaires administered at more than 1 time point (specifically for the purpose of quality assessment) are feasible and acceptable to patients, clinicians, and other stakeholders.
Potential risk adjustment variables were also identified through interviews and a literature search.8,9,18,86-88 Five were variables commonly used as risk adjusters: age, sex, race and ethnicity, insurance type, and cancer type.86-88 Additional risk adjustment variables mentioned were education, working, being married/partnered, having difficulty paying bills, palliative vs curative care, regimen and emetic risk, and comorbid conditions. The final set of parsimonious risk adjustment variables used across all models consisted of age, sex, race and ethnicity, and cancer type. The influence of these variables on provider rank was modest, and the inclusion of further predictors did not meaningfully change the results.
Clinicians, health care administrators, and national experts felt strongly that PRO-PMs should be part of an overall approach to patient-centered care. In such an initiative, PROs would be used at the point of care to improve communication about symptoms among clinicians and patients with cancer, and then used as aggregated PRO-PMs at the clinic level. Clinicians wanted to be informed of PROs at visits so that they can intervene, which may increase the acceptability of PRO-PMs.
In feasibility testing at 6 cancer centers, 470 of 607 patients with cancer (77%) self-reported, and reports from another 14% were obtained with reminder calls from staff (research coordinator or nurse based at the clinic). In busy cancer centers, reminder calls from staff to complete a PRO-PM may not be possible. Patients were given a $20 gift card to complete the PRO questionnaire because the research was not part of their usual care. We are conducting a follow-up wave of testing at the same cancer centers to determine the adherence percentage when patients are not compensated.
Although interviews suggested that both web and IVR should be available to patients for reporting PROs, only a small proportion of patients ultimately used IVR, suggesting that web with paper and human backup may be sufficient in this context. The potential benefit of IVR is that patients do not need to have internet access or computer experience. Broadband access in the United States is highly variable,89 and some at-risk groups (eg, older, rural) are less likely than other groups to have internet access.90,91 In a large pragmatic PRO intervention trial in community oncology practices (PCORI IHS-1511-33392),36 more than a third of chemotherapy patients chose IVR to complete their weekly PRO questionnaire, and these patients represented at-risk groups.92 Depending on available resources and priorities, cancer centers may want to include an option to contact patients to recover otherwise-missing data, especially considering the low response rates typically observed for CAHPS satisfaction with care questionnaires when used in routine care settings.93
We were also able to evaluate the potential of candidate PRO-PMs to be reliable and valid indicators of site performance. The graphical approach used in this aim is novel; it can be applied to a variety of settings when comparing a range of potential performance measures is desired. Feasibility testing also showed that individual PRO-PM items can be combined to create summary items and that risk adjustment can be applied to individual items and summary items. Using summary items is novel, and the approach could be beneficial as payers consider expanding programs that reward providers for high-quality care.
The relative descriptive performance across sites on unadjusted measures was generally consistent: 1 site appeared to perform better than other sites across measures, 1 site appeared to perform relatively worse, and the other 4 sites grouped in the middle. For some individual items and composite measures, variation across sites was not sufficient to calculate the necessary variance components for reliability assessment. This is a result of both the number of sites included in this study and the small variation between sites on some measures. Additionally, once case mix (patient demographic and clinical characteristics) was accounted for through risk adjustment, the variation between sites was further reduced, and even fewer models had site-to-site variance components that could be estimated. This finding suggests that the rankings of the 6 sites in this study may not reliably reflect the underlying relative performance across sites, particularly when variation in case mix is considered. Additionally, Pearson correlation for many measures was relatively low, owing in part to the limited ability to measure correlation between site-level low physical functioning and symptom burden for the 6 sites.
Although this exploratory work did not identify an individual item or composite item with optimal measure properties, the methods successfully differentiated between candidate PRO-PMs with the data collected for this project, and the method could be used in the future to test additional measures once more data are collected. Additionally, the testing process used to differentiate between measures fulfills many of the scientific measure testing requirements from the NQF. Thus, this information could be used to support a future application for endorsement if optimally performing PRO-PMs are identified or if the measures in this work were tested to generate sufficient data to tabulate reliability.
Lessons Learned and Potential for Generalizability
We learned that engagement with stakeholders, patients, caregivers, patient investigators, and our study advisory board had a notable impact on study phases and operations; these elements of our project helped us conduct the study in a way that was meaningful to patients with cancer. Our patient investigators reviewed and piloted patient-facing materials. The study advisory board helped develop and guide the analysis and interpretation of results, next steps, and dissemination. Furthermore, the patients provided their perspectives through interviews and questionnaire completion. Because we engaged multiple stakeholder groups, our results may generalize to the national population of patients with cancer and treatment centers. Finally, although the method used to differentiate between candidate PRO-PMs appeared to be successful using the data collected for this study, the generalizability of the specific conclusions on reliability, validity, and optimal cutoffs for summary measures may not be. However, the graphical method developed for this project is likely broadly applicable and could be generalized to expanded oncology PRO-PM data sets as well as other settings where measure development is desired.
Impact of Results on Health Care Decision-making
Symptom management is a cornerstone of clinical practice. Prior qualitative and quantitative work in oncology consistently indicates that symptoms and QOL are among the highest priorities to patients with cancer and caregivers. In aim 1 interviews, patients and caregivers discussed how understanding their symptom burden in relation to other patients would be helpful to them. Patients and caregivers also speculated about the possibility of choosing a treatment center based on average symptom scores at cancer centers, but they also noted that their choices might be limited because of insurance, geographic, or financial constraints. Clinicians suggested a dual-purpose approach where individual-level PROs are used at the point of care to improve communication about symptoms among clinicians and patients during visits and then used as adjusted PRO-PMs at the clinic level to assess quality.
Health care administrators perceived that PRO-PMs may encourage competition among health care systems to increase symptom control rates. They also thought that PRO-PMs could enhance their understanding of care costs and help improve care. Perceived barriers included the reliability and validity of PRO-PMs and risk adjustment variables, information overload, liability, potential for staff to dismiss PRO-PM data, and lack of funding for implementation and sustainability. National experts discussed similar topics; they added concerns about what a meaningful difference between practices would be for PRO-PMs.
Subpopulation Considerations
We identified adjustment variables for patient characteristics (age, insurance type, and cancer type) that were significant in models. Future research may need to confirm that these risk adjustment variables are robust for oncology PRO-PMs. Additionally, consideration could be given to other patient-specific clinical (eg, health status, comorbidities) and social determinants of health variables that could influence the ranking of sites observed in this work.
Study Limitations and Recommendations for Future Research
The findings from our study should be interpreted with an eye toward limitations and recommendations for future research. First, patients with cancer, clinicians, and other stakeholders were recruited from 6 cancer centers. Whether the recommended PRO-PMs for systemic therapy and risk adjustment variables will be similar in other cancer centers, treatment types (eg, radiation therapy), disease stages, or other health conditions is unknown. Additional PRO-PMs may need to be developed for systemic therapy for both cancer and other health conditions. Although there were limited numbers of patient participants with hematologic malignancies, these findings likely extend across ambulatory populations with cancer, although future research could focus on those with lymphoma or indolent leukemias.
In the aim 1 interviews, the sample of some stakeholder groups may not be representative, although we took care to purposefully sample whenever possible. Patient and caregiver stakeholder groups included patients with cancer in active treatment (n = 56) and primary caregivers (n = 21) at a participating cancer center, as well as patient investigators who previously were treated for cancer (n = 5). Patients were purposefully sampled so that underserved groups were represented (48% were women, 34% were aged ≥65 years, 14% were Black/African American, 8% were Asian, and 20% had a high school education or less). Cancer types included genitourinary (32%), gastrointestinal (27%), breast (21%), and lung (20%). Future research should endeavor to recruit diverse patient groups, especially those treated at community cancer centers and in rural areas.
We were not able to purposefully sample primary caregivers because they typically cannot be identified by cancer center databases. Thus, when patients were approached, for example, during infusions, primary caregivers may also have been present. It was not necessary for primary caregivers to be linked to a participating patient, however. Thus, the sample we obtained for caregivers was 71% female, and 24% were aged ≥65 years, 76% were non-Hispanic White, and 14% had a high school education or less. Caregiver relationships were typically spouse, partner, or an adult child. These demographics are typical for primary caregivers for cancer, as 65% to 75% are usually women with an average age of 69 years.94 Future research should attempt purposeful sampling to make sure diverse voices are heard. Caregiver perspectives on important PRO-PMs were similar to those of patients with cancer. Caregivers are an important part of the cancer care team and may be able to assist patients with cancer in completing PRO-PMs at home. In this study, caregivers were key stakeholders for input but did not directly provide reports related to patient symptoms. Future research could consider including caregiver or proxy reporting.
Professional stakeholder groups included clinicians without clinic administrative responsibilities (n = 11), health care administrators (n = 16, of whom 12 were also clinicians), and national experts, including clinicians (n = 15, of whom 5 were also clinicians). Clinicians and health care administrators were recruited from the 6 cancer centers, and experts were identified nationally. A total of 28 clinicians were included. We separated clinicians with administrative responsibilities into a separate subgroup because they may have a different perspective given their additional responsibilities. Nonetheless, additional validity work with clinicians, administrators, and national experts at more cancer centers is needed to confirm our interview findings.
We recommend engaging patients who are undergoing active treatment, patient advocates, caregivers, clinicians, health care administrators, and national experts to inform the development of PRO-PMs. This engagement will increase transparency of the process for professional groups and highlight the patient voice. Future research should consider adding payers as a stakeholder group.
Second, patients with cancer completed a 1-time PRO questionnaire at home and were compensated with a $20 gift card so we could examine acceptability and feasibility. Collecting PRO-PMs at 1 time point is consistent with the methodology used by MNCM.74 Future research is warranted to examine whether PRO questionnaires administered at more than 1 time point (for the purpose of quality assessment) are feasible and acceptable to patients, clinicians, and other stakeholders. Future research is also needed to determine the adherence percentage when no compensation is offered to patients with cancer (such testing is underway).
Third, our interpretation of what is feasible for a PRO-PM completed at home in this study (for the sole purpose of assessing quality of care) may not generalize to routine care. Of 793 patients approached, 16% refused to participate. This refusal rate is an excellent achievement because observational research typically has refusal rates around 30%. Refusals or nonresponders to PRO-PMs in routine care would likely be much higher. For example, refusal rates for commonly used patient-reported quality assessments, such as CAHPS Cancer Care,51 can be as high as 80% refusals for mailed surveys and 60% for emailed surveys.93 Future research will need to determine the minimum percentage of patients in a clinic necessary for reliable and valid PRO-PMs.
Feasibility metrics should also be interpreted with respect to different denominators. Feasibility was defined as completion rates that exceed an a priori threshold of 75%. When using a denominator of 607 for the number of patients who completed a PRO-PM at home without a reminder call, the feasibility percentage is 77% (470/607). When using a denominator of the 653 patients enrolled, however, the feasibility percentage is 72% (470/653). Reminder calls from staff to complete a PRO-PM may not be possible in many cancer centers. Research is warranted to determine typical completion rates in routine care and whether completion rates vary by demographic characteristics (ie, missing not at random).
Fourth, benchmarks for meaningful differences between practices for PRO-PMs have not been established. Thus, our results warrant additional assessment in research studies before moving to routine care settings. Our methods for assessing the reliability and validity of PRO-PMs, and those for identifying appropriate threshold scores for composite PRO-PMs, may be useful in future PRO-PM development. However, given the limited number of sites included in this study, it is not certain whether the cutoffs identified for the summary measures are generalizable to other sites as optimal performance measures or if these identified cutoffs are specific to this context and would change if additional data are gathered. The specific performance measures and graphical process for differentiating between them that we developed warrant future testing of reliability and validity at a larger number of cancer centers. More data are needed across additional sites to establish the reliability and validity of these measures for use as PRO-PMs. If these data can be obtained and analysis finds measures that meet scientifically acceptable criteria for reliability and validity, consideration can be given to submitting individual and summary items as PRO-PM concepts for endorsement by the NQF and subsequent inclusion in performance-based oncology payment models.
Conclusions
Patients, patient advocates, caregivers, clinicians, and national experts agree that performance measures based on how patients feel and function will be an important addition to quality measurement. PRO-PMs can be feasibly captured at home during systemic therapy; moreover, they are acceptable to patients. Testing at 6 cancer centers showed that aggregating individuals' PRO responses to the clinical practice level and applying risk adjustment variables are both possible. Individual PRO-PM items can be combined to create summary PRO-PMs. PRO-PMs may add meaningful information to evaluating quality of care. Additional data are needed to meet the recommended reliability threshold for the tested measures as well as to test the generalizability of cutoffs for summary measures developed for this study.
The main scientific strengths of this study include internal validity, stakeholder engagement, foundational empirical work, and relevance to patient care. Limitations include a relatively small number of cancer centers (6) and need for future reliability and validity testing. The methods developed and tested in this study will advance the field of patient-centered performance measurement.
References
- 1.
- Vital Signs: Core Metrics for Health and Health Care Progress. Mil Med. 2016;181(6):505-506. [PubMed: 27244056]
- 2.
- Hassett MJ, McNiff KK, Dicker AP, et al. High-priority topics for cancer quality measure development: results of the 2012 American Society of Clinical Oncology Collaborative Cancer Measure Summit. J Oncol Pract. 2014;10(3):e160-e166. doi:10.1200/JOP.2013.001240 [PubMed: 24549319] [CrossRef]
- 3.
- Desch CE, McNiff KK, Schneider EC, et al. American Society of Clinical Oncology/National Comprehensive Cancer Network quality measures. J Clin Oncol. 2008;26(21):3631-3637. [PubMed: 18640941]
- 4.
- Neuss MN, Malin JL, Chan S, et al. Measuring the improving quality of outpatient care in medical oncology practices in the United States. J Clin Oncol. 2013;31(11):1471-1477. [PubMed: 23478057]
- 5.
- Gilbert E, Sherry V, McGettigan S, Berkowitz A. Health-care metrics in oncology. J Adv Pract Oncol. 2015;6(1):57-61. [PMC free article: PMC4577034] [PubMed: 26413375]
- 6.
- Center for Drug Evaluation and Research. Patient-Focused Drug Development: Methods to Identify What Is Important to Patients Guidance for Industry, Food and Drug Administration Staff, and Other Stakeholders. US Food and Drug Administration. Published April 27, 2020. Accessed March 13, 2021. https://www
.fda.gov/media /131230/download - 7.
- Basch E, Torda P, Adams K. Standards for patient-reported outcome-based performance measures. JAMA. 2013;310(2):139-140. [PubMed: 23839744]
- 8.
- Cella D, Hahn EA, Jensen SE, et al. Patient-Reported Outcomes in Performance Measurement. RTI Press; 2015. Accessed August 9, 2020. http://www
.ncbi.nlm.nih .gov/books/NBK424378/ [PubMed: 28211667] - 9.
- Basch E, Spertus J, Dudley RA, et al. Methods for developing patient-reported outcome-based performance measures (PRO-PMs). Value Health. 2015;18(4):493-504. [PubMed: 26091604]
- 10.
- Center for Medicare & Medicaid Services (CMS). CMS Measures Management System Blueprint. Accessed August 9, 2020. https://www
.cms.gov/Medicare /Quality-Initiatives-Patient-Assessment-Instruments /MMS/MMS-Blueprint - 11.
- National Quality Forum. Measuring What Matters to Patients; Innovations in Integrating the Patient Experience into Development of Meaningful Performance Measures. Published August 28, 2017. Accessed September 10, 2021. https://www
.qualityforum .org/Publications /2017/08/Measuring_What _Matters_to_Patients __Innovations_in _Integrating_the_Patient _Experience_into _Development_of_Meaningful _Performance_Measures.aspx - 12.
- National Committee for Quality Assurance. Improving the health care experience: measuring what matters to people. Published June 6, 2018. Accessed September 10, 2021. https://www
.ncqa.org /news/improving-the-health-care-experience-measuring-what-matters-to-people/ - 13.
- Centers for Medicare & Medicaid Services Innovation Center. Oncology Care First Model: Informal Request for Information. Centers for Medicare & Medicaid Services (CMS). Accessed September 10, 2021. https://innovation
.cms .gov/files/x/ocf-informalrfi.pdf - 14.
- Amdal CD, Jacobsen A-B, Guren MG, Bjordal K. Patient-reported outcomes evaluating palliative radiotherapy and chemotherapy in patients with oesophageal cancer: a systematic review. Acta Oncol. 2013;52(4):679-690. [PubMed: 23190360]
- 15.
- Huang C-Y, Ju D-T, Chang C-F, Muralidhar Reddy P, Velmurugan BK. A review on the effects of current chemotherapy drugs and natural agents in treating non-small cell lung cancer. Biomedicine (Taipei). 2017;7(4):23. doi:10.1051/bmdcn/2017070423 [PMC free article: PMC5682982] [PubMed: 29130448] [CrossRef]
- 16.
- Zech DF, Grond S, Lynch J, Hertel D, Lehmann KA. Validation of World Health Organization Guidelines for cancer pain relief: a 10-year prospective study. Pain. 1995;63(1):65-76. [PubMed: 8577492]
- 17.
- Dy SM, Asch SM, Naeim A, Sanati H, Walling A, Lorenz KA. Evidence-based standards for cancer pain management. J Clin Oncol. 2008;26(23):3879-3885. [PubMed: 18688056]
- 18.
- Basch E, Snyder C, McNiff K, et al. Patient-reported outcome performance measures in oncology. J Oncol Pract. 2014;10(3):209-211. [PMC free article: PMC5527827] [PubMed: 24756142]
- 19.
- Reilly CM, Bruner DW, Mitchell SA, et al. A literature synthesis of symptom prevalence and severity in persons receiving active cancer treatment. Support Care Cancer. 2013;21(6):1525-1550. [PMC free article: PMC4299699] [PubMed: 23314601]
- 20.
- Henry DH, Viswanathan HN, Elkin EP, Traina S, Wade S, Cella D. Symptoms and treatment burden associated with cancer treatment: results from a cross-sectional national survey in the U.S. Support Care Cancer. 2008;16(7):791-801. [PubMed: 18204940]
- 21.
- Cleeland CS. The measurement of pain from metastatic bone disease: capturing the patient’s experience. Clin Cancer Res. 2006;12(20 Pt 2):6236s-6242s. doi:10.1158/1078-0432.CCR-06-0988 [PubMed: 17062707] [CrossRef]
- 22.
- Howell D, Molloy S, Wilkinson K, et al. Patient-reported outcomes in routine cancer clinical practice: a scoping review of use, impact on health outcomes, and implementation factors. Ann Oncol. 2015;26(9):1846-1858. [PubMed: 25888610]
- 23.
- Halabi S, Vogelzang NJ, Kornblith AB, et al. Pain predicts overall survival in men with metastatic castration-refractory prostate cancer. J Clin Oncol. 2008;26(15):2544-2549. [PubMed: 18487572]
- 24.
- Kirkova J, Davis MP, Walsh D, et al. Cancer symptom assessment instruments: a systematic review. J Clin Oncol. 2006;24(9):1459-1473. [PubMed: 16549841]
- 25.
- Amtmann D, Cook KF, Jensen MP, et al. Development of a PROMIS item bank to measure pain interference. Pain. 2010;150(1):173-182. [PMC free article: PMC2916053] [PubMed: 20554116]
- 26.
- Cleeland CS, Body J-J, Stopeck A, et al. Pain outcomes in patients with advanced breast cancer and bone metastases: results from a randomized, double-blind study of denosumab and zoledronic acid. Cancer. 2013;119(4):832-838. [PubMed: 22951813]
- 27.
- Deandrea S, Montanari M, Moja L, Apolone G. Prevalence of undertreatment in cancer pain. A review of published literature. Ann Oncol. 2008;19(12):1985-1991. [PMC free article: PMC2733110] [PubMed: 18632721]
- 28.
- Davis MP, Walsh D. Epidemiology of cancer pain and factors influencing poor pain control. Am J Hosp Palliat Care. 2004;21(2):137-142. [PubMed: 15055515]
- 29.
- Basch E, Reeve BB, Mitchell SA, et al. Development of the National Cancer Institute’s patient-reported outcomes version of the common terminology criteria for adverse events (PRO-CTCAE). J Natl Cancer Inst. 2014;106(9):dju244. doi:10.1093/jnci/dju244 [PMC free article: PMC4200059] [PubMed: 25265940] [CrossRef]
- 30.
- Stover AM, Urick BY, Deal AM, et al. Performance measures based on how adults with cancer feel and function: stakeholder recommendations and feasibility testing in six cancer centers. JCO Oncol Pract. 2020;16(3):e234-e250. doi:10.1200/JOP.19.00784 [PMC free article: PMC7069703] [PubMed: 32074014] [CrossRef]
- 31.
- Patton MQ. Qualitative Research & Evaluation Methods. 3rd ed. Sage Publications; 2002.
- 32.
- Moy B, Polite BN, Halpern MT, et al. American Society of Clinical Oncology policy statement: opportunities in the patient protection and affordable care act to reduce cancer care disparities. J Clin Oncol. 2011;29(28):3816-3824. [PubMed: 21810680]
- 33.
- Hahn EA, Cella D. Health outcomes assessment in vulnerable populations: measurement challenges and recommendations. Arch Phys Med Rehabil. 2003;84(4 Suppl 2):S35-S42. doi:10.1053/apmr.2003.50245 [PubMed: 12692770] [CrossRef]
- 34.
- Morris AM, Rhoads KF, Stain SC, Birkmeyer JD. Understanding racial disparities in cancer treatment and outcomes. J Am Coll Surg. 2010;211(1):105-113. [PubMed: 20610256]
- 35.
- Stover A, Irwin DE, Chen RC, et al. Integrating patient-reported measures into routine cancer care: cancer patients’ and clinicians’ perceptions of acceptability and value. EGEMS (Wash DC). 2015;3(1):1169. doi:10.13063/2327-9214.1169 [PMC free article: PMC4636110] [PubMed: 26557724] [CrossRef]
- 36.
- Stover AM, Tompkins Stricker C, Hammelef K, et al. Using stakeholder engagement to overcome barriers to implementing patient-reported outcomes (PROs) in cancer care delivery: approaches from 3 prospective studies. Med Care. 2019;57:S92-S99. doi:10.1097/MLR.0000000000001103 [PubMed: 30985602] [CrossRef]
- 37.
- Atkinson TM, Li Y, Coffey CW, et al. Reliability of adverse symptom event reporting by clinicians. Qual Life Res. 2012;21(7):1159-1164. [PMC free article: PMC3633532] [PubMed: 21984468]
- 38.
- Basch E, Jia X, Heller G, et al. Adverse symptom event reporting by patients vs clinicians: relationships with clinical outcomes. J Natl Cancer Inst. 2009;101(23):1624-1632. [PMC free article: PMC2786917] [PubMed: 19920223]
- 39.
- Butt Z, Rosenbloom SK, Abernethy AP, et al. Fatigue is the most important symptom for advanced cancer patients who have had chemotherapy. J Natl Compr Cancer Netw. 2008;6(5):448-455. [PMC free article: PMC5089809] [PubMed: 18492460]
- 40.
- Prinsen CAC, Vohra S, Rose MR, et al. How to select outcome measurement instruments for outcomes included in a “Core Outcome Set” – a practical guideline. Trials. 2016;17(1):449. doi:10.1186/s13063-016-1555-2 [PMC free article: PMC5020549] [PubMed: 27618914] [CrossRef]
- 41.
- Mokkink LB, Prinsen CAC, Bouter LM, de Vet HCW, Terwee CB. The COnsensus-based Standards for the selection of health Measurement INstruments (COSMIN) and how to select an outcome measurement instrument. Braz J Phys Ther. 2016;20(2):105-113. [PMC free article: PMC4900032] [PubMed: 26786084]
- 42.
- Gorst SL, Prinsen CAC, Salcher-Konrad M, Matvienko-Sikar K, Williamson PR, Terwee CB. Methods used in the selection of instruments for outcomes included in core outcome sets have improved since the publication of the COSMIN/COMET guideline. J Clin Epidemiol. 2020;125:64-75. [PubMed: 32470621]
- 43.
- Ottery FD. Definition of standardized nutritional assessment and interventional pathways in oncology. Nutr. 1996;12(1 Suppl):S15-S19. [PubMed: 8850213]
- 44.
- Dueck AC, Mendoza TR, Mitchell SA, et al. Validity and reliability of the US National Cancer Institute’s Patient-Reported Outcomes Version of the Common Terminology Criteria for Adverse Events (PRO-CTCAE). JAMA Oncol. 2015;1(8):1051-1059. [PMC free article: PMC4857599] [PubMed: 26270597]
- 45.
- Cella D, Yount S, Rothrock N, et al. The Patient-Reported Outcomes Measurement Information System (PROMIS): progress of an NIH Roadmap cooperative group during its first two years. Med Care. 2007;45(5 Suppl 1):S3-S11. doi:10.1097/01.mlr.0000258615.42478.55 [PMC free article: PMC2829758] [PubMed: 17443116] [CrossRef]
- 46.
- Hays RD, Bjorner JB, Revicki DA, Spritzer KL, Cella D. Development of physical and mental health summary scores from the Patient-Reported Outcomes Measurement Information System (PROMIS) global items. Qual Life Res. 2009;18(7):873-880. [PMC free article: PMC2724630] [PubMed: 19543809]
- 47.
- Institute of Medicine. Finding What Works in Health Care: Standards for Systematic Reviews. National Academies Press; 2011. [PubMed: 24983062]
- 48.
- Liberati A, Altman DG, Tetzlaff J, et al. The PRISMA statement for reporting systematic reviews and meta-analyses of studies that evaluate healthcare interventions: explanation and elaboration. BMJ. 2009;339. doi:10.1136/bmj.b2700 [PMC free article: PMC2714672] [PubMed: 19622552] [CrossRef]
- 49.
- Hayes AF, Krippendorff K. Answering the call for a standard reliability measure for coding data. Commun Methods Meas. 2007;1(1):77-989.
- 50.
- Krippendorff K. Computing Krippendorff’s alpha-reliability. University of Pennsylvania; 2011. Accessed March 13, 2021. http://repository
.upenn .edu/asc_papers/43 - 51.
- Hess LM, Pohl G. Perspectives of quality care in cancer treatment: a review of the literature. Am Health Drug Benefits. 2013;6(6):321-329. [PMC free article: PMC4031722] [PubMed: 24991367]
- 52.
- Colosia AD, Peltz G, Pohl G, et al. A review and characterization of the various perceptions of quality cancer care. Cancer. 2011;117(5):884-896. [PMC free article: PMC3073118] [PubMed: 20939015]
- 53.
- Patel MI, Periyakoil VS, Blayney DW, et al. Redesigning cancer care delivery: views from patients and caregivers. J Oncol Pract. 2017;13(4):e291-e302. doi:10.1200/JOP.2016.017327 [PMC free article: PMC5455153] [PubMed: 28399387] [CrossRef]
- 54.
- Jagsi R, Chiang A, Polite BN, et al. Qualitative analysis of practicing oncologists’ attitudes and experiences regarding collection of patient-reported outcomes. J Oncol Pract. 2013;9(6):e290-e297. doi:10.1200/JOP.2012.000823 [PubMed: 23943890] [CrossRef]
- 55.
- Aktas A, Walsh D, Kirkova J. The psychometric properties of cancer multisymptom assessment instruments: a clinical review. Support Care Cancer. 2015;23(7):2189-2202. [PubMed: 25894883]
- 56.
- Catt S, Starkings R, Shilling V, Fallowfield L. Patient-reported outcome measures of the impact of cancer on patients’ everyday lives: a systematic review. J Cancer Surviv. 2017;11(2):211-232. [PMC free article: PMC5357497] [PubMed: 27834041]
- 57.
- Brédart A, Kop J-L, Efficace F, et al. Quality of care in the oncology outpatient setting from patients’ perspective: a systematic review of questionnaires’ content and psychometric performance. Psychooncology. 2015;24(4):382-394. [PubMed: 25196048]
- 58.
- Howell D, Fitch M, Bakker D, et al. Core domains for a person-focused outcome measurement system in cancer (PROMS-Cancer Core) for routine care: a scoping review and Canadian Delphi Consensus. Value Health. 2013;16(1):76-87. [PubMed: 23337218]
- 59.
- Jensen RE, Moinpour CM, Potosky AL, et al. Responsiveness of 8 Patient-Reported Outcomes Measurement Information System (PROMIS) measures in a large, community-based cancer study cohort. Cancer. 2017;123(2):327-335. [PMC free article: PMC5222745] [PubMed: 27696377]
- 60.
- van Roij J, Fransen H, van de Poll-Franse L, Zijlstra M, Raijmakers N. Measuring health-related quality of life in patients with advanced cancer: a systematic review of self-administered measurement instruments. Qual Life Res. 2018;27(8):1937-1955. [PubMed: 29427216]
- 61.
- Jordhoy MS, Inger Ringdal G, Helbostad JL, Oldervoll L, Loge JH, Kaasa S. Assessing physical functioning: a systematic review of quality of life measures developed for use in palliative care. Palliat Med. 2007;21(8):673-682. [PubMed: 18073253]
- 62.
- Zijlema WL, Stolk RP, Löwe B, et al. How to assess common somatic symptoms in large-scale studies: a systematic review of questionnaires. J Psychosom Res. 2013;74(6):459-468. [PubMed: 23731742]
- 63.
- Pullmer R, Linden W, Rnic K, Vodermaier A. Measuring symptoms in gastrointestinal cancer: a systematic review of assessment instruments. Support Care Cancer. 2014;22(11):2941-2955. [PubMed: 24865875]
- 64.
- King MT, Winters ZE, Olivotto IA, et al. Patient-reported outcomes in ductal carcinoma in situ: a systematic review. Eur J Cancer. 2017;71:95-108. [PubMed: 27987454]
- 65.
- Chopra I, Kamal KM. A systematic review of quality of life instruments in long-term breast cancer survivors. Health Qual Life Outcomes. 2012;10:14. doi:10.1186/1477-7525-10-14 [PMC free article: PMC3280928] [PubMed: 22289425] [CrossRef]
- 66.
- Donovan KA, Donovan HS, Cella D, et al. Recommended patient-reported core set of symptoms and quality-of-life domains to measure in ovarian cancer treatment trials. J Natl Cancer Inst. 2014;106(7):dju128. doi:10.1093/jnci/dju128 [PMC free article: PMC4110471] [PubMed: 25006190] [CrossRef]
- 67.
- Ojo B, Genden EM, Teng MS, Milbury K, Misiukiewicz KJ, Badr H. A systematic review of head and neck cancer quality of life assessment instruments. Oral Oncol. 2012;48(10):923-937. [PMC free article: PMC3406264] [PubMed: 22525604]
- 68.
- Bouazza YB, Chiairi I, El Kharbouchi O, et al. Patient-reported outcome measures (PROMs) in the management of lung cancer: a systematic review. Lung Cancer. 2017;113:140-151. [PubMed: 29110842]
- 69.
- Sandler KA, Mitchell SA, Basch E, et al. Content validity of anatomic site-specific Patient-Reported Outcomes Version of the Common Terminology Criteria for Adverse Events (PRO-CTCAE) item sets for assessment of acute symptomatic toxicities in radiation oncology. Int J Radiat Oncol Biol Phys. 2018;102(1):44-52. [PubMed: 30102201]
- 70.
- Kemmler G, Holzner B, Kopp M, et al. Comparison of two quality-of-life instruments for cancer patients: the functional assessment of cancer therapy-general and the European Organization for Research and Treatment of Cancer Quality of Life Questionnaire-C30. J Clin Oncol. 1999;17(9):2932-2940. [PubMed: 10561373]
- 71.
- Cleeland CS, Mendoza TR, Wang XS, et al. Assessing symptom distress in cancer patients: the M.D. Anderson Symptom Inventory. Cancer. 2000;89(7):1634-1646. [PubMed: 11013380]
- 72.
- Ware JE, Kosinski M, Keller SD. SF-36 Physical and Mental Health Summary Scales: A Users’ Manual. Health Assessment Lab. Published December 1994.
- 73.
- Herdman M, Gudex C, Lloyd A, et al. Development and preliminary testing of the new five-level version of EQ-5D (EQ-5D-5L). Qual Life Res. 2011;20(10):1727-1736. [PMC free article: PMC3220807] [PubMed: 21479777]
- 74.
- MN Community Measurement. 2018 Annual Report. MN Community Measurement; 2018. Accessed March 13, 2021. https://mncm
.org/wp-content /uploads/2020 /01/MNCM_Annual_Report_2018.pdf - 75.
- Kristensen A, Solheim TS, Amundsen T, et al. Measurement of health-related quality of life during chemotherapy - the importance of timing. Acta Oncol. 2017;56(5):737-745. [PubMed: 28117614]
- 76.
- Ash AS, Shwartz M, Pekoz EA, Hanchate AD. Chapter 12. Comparing outcomes across providers. In: Risk Adjustment for Measuring Health Care Outcomes. 4th ed. Health Administration Press; 2012.
- 77.
- Urick BY, Urmie JM. Framework for assessing pharmacy value. Res Soc Adm Pharm. 2019;15(11):1326-1337. [PubMed: 30630670]
- 78.
- Adams JL. The Reliability of Provider Profiling: A Tutorial. RAND; 2009.
- 79.
- Reliability: NQF’s Current Definition of Reliability and Related Concepts. National Quality Forum. Published May 16, 2018. [Link no longer active.] https://www
.qualityforum .org/Measuring_Performance /Scientific_Methods_Panel /Meetings /2018_Scientific _Methods_Panel_Meetings.aspx - 80.
- Lawson EH, Ko CY, Adams JL, Chow WB, Hall BL. Reliability of evaluating hospital quality by colorectal surgical site infection type. Ann Surg. 2013;258(6):994-1000. [PubMed: 23657082]
- 81.
- Reeve BB, Mitchell SA, Dueck AC, et al. Recommended patient-reported core set of symptoms to measure in adult cancer treatment trials. J Natl Cancer Inst. 2014;106(7):dju129. doi:10.1093/jnci/dju129 [PMC free article: PMC4110472] [PubMed: 25006191] [CrossRef]
- 82.
- Chera BS, Eisbruch A, Murphy BA, et al. Recommended patient-reported core set of symptoms to measure in head and neck cancer treatment trials. J Natl Cancer Inst. 2014;106(7):dju127. doi:10.1093/jnci/dju127 [PMC free article: PMC4192043] [PubMed: 25006189] [CrossRef]
- 83.
- Chen RC, Chang P, Vetter RJ, et al. Recommended patient-reported core set of symptoms to measure in prostate cancer treatment trials. J Natl Cancer Inst. 2014;106(7):dju132. doi:10.1093/jnci/dju132 [PMC free article: PMC4192044] [PubMed: 25006192] [CrossRef]
- 84.
- Ness KK, Wall MM, Oakes JM, Robison LL, Gurney JG. Physical performance limitations and participation restrictions among cancer survivors: a population-based study. Ann Epidemiol. 2006;16(3):197-205. [PubMed: 16137893]
- 85.
- Salakari MRJ, Surakka T, Nurminen R, Pylkkänen L. Effects of rehabilitation among patients with advances cancer: a systematic review. Acta Oncol. 2015;54(5):618-628. [PubMed: 25752965]
- 86.
- Nuttall D, Parkin D, Devlin N. Inter-provider comparison of patient-reported outcomes: developing an adjustment to account for differences in patient case mix. Health Econ. 2015;24(1):41-54. [PubMed: 24115397]
- 87.
- National Quality Forum. Risk Adjustment for Socioeconomic Status or Other Sociodemographic Factors. Published August 2014. Accessed September 10, 2021. https://www
.qualityforum .org/Publications /2014/08/Risk_Adjustment _for_Socioeconomic _Status_or_Other_Sociodemographic _Factors.aspx - 88.
- Krumholz HM, Chen J, Wang Y, Radford MJ, Chen YT, Marciniak TA. Comparing AMI mortality among hospitals in patients 65 years of age and older: evaluating methods of risk adjustment. Circulation. 1999;99(23):2986-2992. [PubMed: 10368115]
- 89.
- Federal Communications Commission. 2018 Broadband Deployment Report. Published February 5, 2018. Accessed August 9, 2020. https://www
.fcc.gov/reports-research /reports /broadband-progress-reports /2018-broadband-deployment-report - 90.
- Perzynski AT, Roach MJ, Shick S, et al. Patient portals and broadband internet inequality. J Am Med Inform Assoc. 2017;24(5):927-932. [PMC free article: PMC6259664] [PubMed: 28371853]
- 91.
- Douthit N, Kiv S, Dwolatzky T, Biswas S. Exposing some important barriers to health care access in the rural USA. Public Health. 2015;129(6):611-620. [PubMed: 26025176]
- 92.
- Stover AM, Henson S, Jensen J, et al. Demographic and symptom differences in PRO-TECT trial (AFT-39) cancer patients electing to complete weekly home patient-reported outcome measures (PROMs) via an automated phone call vs. email: implications for implementing PROs into routine care. Qual Life Res. 2019;28(Suppl 1):S1.
- 93.
- Fowler FJ, Cosenza C, Cripps LA, Edgman-Levitan S, Cleary PD. The effect of administration mode on CAHPS survey response rates and results: a comparison of mail and web-based approaches. Health Serv Res. 2019;54(3):714-721. [PMC free article: PMC6505419] [PubMed: 30656646]
- 94.
- Carlson LE, Waller A, Mitchell AJ. Screening for distress and unmet needs in patients with cancer: review and recommendations. J Clin Oncol. 2012;30(11):1160-1177. [PubMed: 22412146]
Related Publications
- •.
- Stover AM, Kurtzman R, Walker Bissram J, et al. Stakeholder perceptions of key aspects of high-quality cancer care to assess with patient reported outcome measures: a systematic review. Cancers (Basel). 2021;13(14):3628. doi:10.3390/cancers13143628 [PMC free article: PMC8306432] [PubMed: 34298841] [CrossRef]
- •.
- Stover AM, Urick BY, Deal AM, et al. Performance measures based on how adults with cancer feel and function: stakeholder recommendations and feasibility testing in six cancer centers. JCO Oncol Pract. 2020;16(3):e234-e250. doi:10.1200/JOP.19.00784 [PMC free article: PMC7069703] [PubMed: 32074014] [CrossRef]
- •.
- Stover AM, Basch EM. Using patient-reported outcome measures as quality indicators in routine cancer care. Cancer. 2016;122(3):355-357. doi:10.1002/cncr.29768 [PMC free article: PMC5501295] [PubMed: 26619153] [CrossRef]
Acknowledgments
We would like to acknowledge Lucy Burgess Austin of Roanoke Rapids, North Carolina, and Kendall Johnson of Graham, North Carolina, who served as patient investigators on this work.
Funding sources included PCORI ME-1507-32079, 1UL1TR00111, KL2TR001109, DK056350, P30-DK56350, P30-CA16086, and P30-CA008748. The research reported in this publication was partially funded through a PCORI award (ME-1507-32079). This work is solely the responsibility of the authors and does not necessarily represent the views of PCORI, its Board of Governors, or its Methodology Committee.
Portions of this work were presented at the 2017 International Society for Quality of Life research and 2019 ASCO quality-of-care symposium.
This project made use of systems and services provided by the Patient-Reported Outcomes Core (PRO Core; https://pro.unc.edu/) at the Lineberger Comprehensive Cancer Center (LCCC) of UNC. PRO Core is funded in part by an NCI Cancer Center Core Support Grant (5-P30-CA016086) and the University Cancer Research Fund of North Carolina. The LCCC Bioinformatics Core provided the computational infrastructure for the project.
This study made use of resources funded through the Gillings School of Global Public Health Nutrition Obesity Research Center (National Institute of Diabetes and Digestive and Kidney Diseases; P30 DK56350) and the LCCC (NCI funded; P30-CA16086): the Communication for Health Applications and Interventions Core.
Research reported in this report was funded through a Patient-Centered Outcomes Research Institute® (PCORI®) Award (ME-1507-32079). Further information available at: https://www.pcori.org/research-results/2016/developing-patient-reported-outcome-measures-assess-side-effects-cancer
Appendices
Appendix A.
Study Milestones (PDF, 134K)
Appendix B.
Interview Questions (PDF, 125K)
Appendix C.
Search Strings for Systematic Review (PDF, 132K)
Appendix D.
Adjusted and Unadjusted Site-Level Scores by Measure (PDF, 135K)
Appendix E.
Statistical Results From Risk Adjustment Models for Candidate PRO-PMs (PDF, 186K)
Appendix F.
Abbreviations and Acronyms (PDF, 87K)
Suggested citation:
Stover AM, Urick BY, Jansen J, et al. (2022). Developing Patient-Reported Outcome Measures to Assess Side Effects of Cancer Treatment. Patient-Centered Outcomes Research Institute (PCORI). https://doi.org/10.25302/09.2021.ME.150732079
Disclaimer
The [views, statements, opinions] presented in this report are solely the responsibility of the author(s) and do not necessarily represent the views of the Patient-Centered Outcomes Research Institute® (PCORI®), its Board of Governors or Methodology Committee.
- NLM CatalogRelated NLM Catalog Entries
- PMCPubMed Central citations
- PubMedLinks to PubMed
- Developing Patient-Reported Outcome Measures to Assess Side Effects of Cancer Tr...Developing Patient-Reported Outcome Measures to Assess Side Effects of Cancer Treatment
- condensin-2 complex subunit G2 isoform X1 [Homo sapiens]condensin-2 complex subunit G2 isoform X1 [Homo sapiens]gi|2462615024|ref|XP_054214494.1|Protein
- condensin-2 complex subunit G2 isoform X9 [Homo sapiens]condensin-2 complex subunit G2 isoform X9 [Homo sapiens]gi|767948169|ref|XP_011514664.1|Protein
- chst6 [Ictalurus punctatus]chst6 [Ictalurus punctatus]Gene ID:108268591Gene
- nssv1449992 (1)dbVar
Your browsing activity is empty.
Activity recording is turned off.
See more...