NCBI Bookshelf. A service of the National Library of Medicine, National Institutes of Health.
Institute of Medicine (US) Subcommittee on Interpretation and Uses of Dietary Reference Intakes; Institute of Medicine (US) Standing Committee on the Scientific Evaluation of Dietary Reference Intakes. DRI Dietary Reference Intakes: Applications in Dietary Assessment. Washington (DC): National Academies Press (US); 2000.
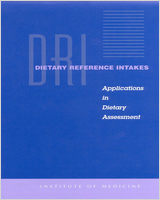
DRI Dietary Reference Intakes: Applications in Dietary Assessment.
Show detailsThis chapter focuses on specific applications of the Dietary Reference Intakes (DRIs) to assess the nutrient adequacy of groups, in particular describing and evaluating dietary survey data. The methodological approaches described in Chapter 4, Chapter 5 and Chapter 6 are applied to some of the specific uses reported in Chapter 2. (Chapter 3 presents an application for assessing the nutrient adequacy of individual diets.) A subsequent report will examine applications of the DRIs for planning nutrient intakes of groups and individuals, which includes many of the other uses presented in Chapter 2.
INTRODUCTION
Assessment of the apparent nutrient adequacy of groups typically has used the former Recommended Dietary Allowances (RDAs) and Recommended Nutrient Intakes (RNIs) because these were the primary dietary standards that were available. In many instances, however, the former RDAs and RNIs were used inappropriately in dietary assessment applications (e.g., RDAs used for dietary assessment of groups, with some arbitrary percentage of the RDA used as a cut-point for determining nutrient adequacy of a group).
The applications considered in this chapter are designed to analyze information about the distribution of average daily intakes over time, referred to as usual nutrient intakes. Typically, though, survey data on nutrient intakes of the same individual are only available for one or two days; sometimes two or more nonconsecutive days of dietary recall data are available for a subsample of individuals, with one day of recall data available for the remainder of the sample.
Thus, to conduct evaluations of dietary survey data, it is usually necessary first to adjust the intake distributions based on at least two nonconsecutive days of dietary recalls to obtain the usual nutrient intake distribution. If these adjustments are not made, outcome variables that rely on any measure other than the group's mean intake are biased (Carriquiry et al., 1997; Nusser et al., 1996). For example, the percentage of individuals in a group with intakes less than a specified cutoff level would be biased (either over- or under-estimated) if determined from unadjusted data on nutrient intakes. See Chapter 4 for methods to adjust intake distributions.
APPLICATION 1: DESCRIBING DIETARY SURVEY DATA
What are the characteristics of the distributions of usual nutrient intake? How variable are usual intakes?
Data available: 24-hour dietary recall data on a nationally representative sample of individuals, with two or more nonconsecutive days of data collected for at least a subsample of individuals.
This discussion assumes that dietary recall data are available from a nationally representative sample of individuals and have been used to estimate the usual nutrient intakes of the population from food and supplements.
The following summary descriptive measures could be examined: mean, median, and other percentiles of the usual nutrient intake distribution. An example of appropriate descriptive statistics is given in Table 7-1.
TABLE 7-1
Describing Nutrient Intakes of Children 4 through 8 Years of Age—Characteristics of Usual Nutrient Intake.
Many researchers have expressed intakes as a percentage of the Recommended Dietary Allowance (RDA) primarily to aid the interpretation of descriptive statistics across life stage and gender groups that have different requirements. Although expressing mean intake as a percentage of the RDA is not incorrect, it is easily misinterpreted. These statistics cannot be used to assess nutrient adequacy.
APPLICATION 2: ASSESSING THE PREVALENCE OF INADEQUATE OR EXCESSIVE INTAKE
What proportion of the population has inadequate nutrient intake? What proportion of the population is at risk of excessive nutrient intake?
Data available: 24-hour dietary recall data on a nationally representative sample of individuals, with two or more nonconsecutive days of data collected for at least a subsample of individuals.
Comparing Usual Intakes with the EAR and the UL
Table 7-2 is an example of an evaluation of the intakes of children 4 through 8 years of age. Under certain assumptions an effective estimate of the prevalence of inadequate intake is the percentage of a group with usual nutrient intake less than the Estimated Average Requirement (EAR). Dietary Reference Intakes (DRIs) have not yet been established for all nutrients, and some nutrients have Adequate Intakes (AIs) rather than EARs. As a result the only nutrients to which the probability approach or the EAR cut-point method (described in Chapter 4) can be applied to assess adequacy in this example are vitamin B6, vitamin B12, vitamin C, vitamin E, folate, niacin, riboflavin, thiamin, magnesium, phosphorus, and selenium. Additional nutrients will be added as DRIs are developed for them.
TABLE 7-2
Assessing Nutrient Intakes of Children 4 through 8 Years of Age—What Proportion Has Inadequate Intake? What Proportion Is Potentially at Risk of Excessive Intake?
To estimate the proportion of the population potentially at risk from excessive intake, the percentage of the group with usual nutrient intake exceeding the Tolerable Upper Intake Level (UL) is determined (see Chapter 6). Again, because ULs have not yet been established for all nutrients, the only nutrients for which the proportion at risk for excessive intake can be estimated are niacin, vitamin B6, folate, choline, vitamin C, vitamin D, vitamin E, calcium, phosphorus, magnesium, fluoride, and selenium. Additional nutrients will also be added to this list as DRIs are developed for them. It should be noted however, that even though EARs or ULs are currently available for some nutrients (e.g., vitamin D, fluoride, and choline), assessment of adequacy or potential risk of excess is not possible because these nutrients are not included in the national intake surveys.
Common Mistakes in Evaluating Dietary Survey Data
Some of the most common mistakes in evaluating dietary survey data arise from inappropriate conclusions drawn from comparing mean nutrient intakes with Recommended Dietary Allowances (RDAs). When mean nutrient intake exceeds the RDA, researchers often conclude—inappropriately—that diets meet or even exceed recommended nutritional standards. At one time, when the RDA was defined as the average intake of a population, this mistake was understandable. However, the current definition of the RDA (and the definition implied in the last two revisions [NRC, 1980, 1989]) specifically defines the RDA as a goal for the individual. In fact, as discussed in Chapter 4, because the variance of usual intake typically exceeds the variance of nutrient requirement for most nutrients, the mean usual nutrient intake of a group must exceed the RDA to have a low prevalence of inadequate intakes. Even if mean usual nutrient intake equals or exceeds the RDA, a significant proportion of the population may have inadequate nutrient intake. This is clearly shown in Table 7-1 and Table 7-2, where both the mean and median of usual intake of folate exceed the RDA, yet approximately 35 per cent of children 4 through 8 years of age are estimated to have usual intake less than the requirement.
Mean or median intakes of nutrients with EARs seldom, if ever, can be used to assess adequacy or excessive intake of group diets. The prevalence of inadequacy depends on the shape and variation of the usual intake distribution, not on mean intake. For food energy, however, mean intake relative to the EAR is a possible measure to use in assessing the adequacy of group diets. Because there is a high correlation between energy intake and energy expenditure (requirement), median intake of food energy should be close to the requirement for there to be low risk of inadequate or excessive intake.
Caution also is necessary when interpreting descriptive statistics for nutrients with an AI. When mean usual intake of a group exceeds the AI the expected prevalence of inadequate intake is low. When mean usual nutrient intake of a group is less than the AI, however, nothing can be inferred about the probability of inadequacy (see Chapter 5).
In short, comparing mean intake either to the EAR or RDA or simply looking at mean intake levels should not be used to assess or imply relative nutrient adequacy.
APPLICATION 3: EVALUATING DIFFERENCES IN INTAKE
Do different subgroups of the population (food stamp participants and nonparticipants , for example) differ in their mean nutrient intakes?
- What are the characteristics of the usual nutrient intake distribution for different population subgroups? Do population subgroups have different distributions of usual nutrient intake ?
- Do population subgroups differ in the proportion with inadequate nutrient intake?
- Do population subgroups differ in the proportion at risk of excessive nutrient intake?
Research studies often focus either on differences in nutrient intake for population subgroups or on the relationship between certain factors and nutrient intakes. Such studies are simply extensions of the dietary survey applications discussed above. They typically use both descriptive and multiple regression analyses to examine differences in nutrient intakes across population subgroups. Descriptive analyses compare differences across subgroups in means, medians, and percentages with intake less than the Estimated Average Requirement (EAR) or exceeding the Tolerable Upper Intake Level (UL). Multiple regression analyses use individual data on nutrient intakes to estimate the effects of various factors on nutrient intake. The results can be used to present regression-adjusted differences in measures among the subgroups.
As an example of this application, consider an evaluation of the Food Stamp Program (FSP) that involves estimating the relationship between FSP participation and nutrient intakes. In this application, 24-hour dietary recall data are available on a nationally representative sample of individuals eligible for the FSP. This sample includes both FSP participants and low-income nonparticipants.
Descriptive Analyses of Nutrient Intakes
Descriptive analyses would examine the mean, median, and other selected percentiles of the usual nutrient intake distribution.
Statistical tests can be conducted to determine whether FSP participation is associated with differences in nutrient intake. In this case, if comparison of the means is all that is wanted (although of limited value), no adjustments for usual intake are necessary and a t-test can be used. However, before performing these tests, it is important to consider survey weights and survey design effects. If sampled individuals have different survey weights attached to them (see Chapter 4), the mean and the standard error of the mean need to be computed using these weights. If the survey design is clustered, the variance can be artificially reduced and thus needs to be adjusted. Various software programs can be used for this purpose. 1
However, if interest is on information at the tails of the distributions (i.e., percentiles), adjustment of the intake distributions to obtain the usual nutrient intake distributions from the observed nutrient intake distributions is needed to more accurately reflect the individual-to-individual variation in intake. For example, one might wish to determine whether the proportion of individuals with inadequate intakes is different among FSP participants and low-income nonparticipants.
To describe differences in the prevalence of apparently inadequate nutrient intakes between subgroups, the percentages of FSP participants and low-income nonparticipants with usual nutrient intake less than the EAR (for nutrients with an EAR) should be calculated and compared. Similarly, to describe differences in the percentage potentially at risk from excessive nutrient intakes by subgroup, the percentages of FSP participants and low-income nonparticipants with usual nutrient intake greater than the UL (for nutrients with a UL) should be calculated and compared. Tests such as t-tests can then be conducted to determine whether these differences are statistically significant.
Multiple Regression Analyses of Nutrient Intake
One important objective of multiple regression analysis is to correct the simple difference in group mean intake discussed above for other differences between subgroups. For example, suppose FSP participants and low-income nonparticipants differ in their characteristics (such as household income or family size) and that these differences also affect nutrient intake. Multiple regression analyses (straightforward analyses of covariance) can adjust the simple difference in mean nutrient intake between FSP participants and nonparticipants for differences attributed to household income and family size. The results of these analyses can be used to calculate regression-adjusted differences in nutrient intake for different population subgroups.
In multiple regression analysis, the dependent variable refers to an individual, not to a group. As noted previously, individual nutrient intake observed on one day is not the same as usual nutrient intake for that individual. Although adjustments can be made to the intake distribution of a group to estimate the usual intake distribution (see Chapter 4), adjustments cannot usually be made to individual values to estimate usual individual intake. The discussion below focuses on using observed nutrient intake data for individuals to define dependent variables for multiple regression analyses, how to interpret the results from the regression analyses, and how to use the results of these analyses to assess differences in nutrient adequacy across subgroups.
Regression-Adjusted Differences in Mean Nutrient Intakes
For a multiple regression analysis of nutrient intakes, the dependent variable is usually the observed individual nutrient intake. In the context of the FSP, the dependent variable would be observed nutrient intakes while predictor variables might include—in addition to food stamp participation—household income, family size, education, region of residence, and other important characteristics influencing nutrient intake. This type of multiple regression analysis typically produces a set of regression coefficients and their standard deviations. On the basis of the estimated coefficient for FSP participation, regression-adjusted differences in mean nutrient intake can be calculated between FSP participants and low-income nonparticipants, controlling for other differences between participants and nonparticipants that may also influence nutrient intake. In addition, just as the mean of observed nutrient intake is an unbiased estimate of mean usual nutrient intake, these regression-adjusted differences in mean observed intakes are unbiased estimates of regression-adjusted mean usual nutrient intake.
Multiple regression analysis of nutrient intakes has been used to assess the relationship between program participation and nutrient intakes in FSP eligible individuals (Gordon et al., 1995; Oliveira and Gunderson, 2000; Rose et al., 1998). Specifically, the regression-adjusted differences in mean intake between program participants and a comparison group of nonparticipants were interpreted, with certain caveats, as the estimated effects of program participation. However, as noted previously, mean intakes cannot be used to assess nutrient adequacy. Similarly, differences in mean intakes between subgroups cannot be used to draw conclusions about the effects of program participation on nutrient adequacy. They can be used only to make inferences about differences in mean intakes between program participants and nonparticipants. The approach described below provides a method of estimating the effect of FSP participation on nutrient adequacy.
Comparison of the Prevalence of Inadequate Nutrient Intakes
As discussed above, multiple regression analysis can be used to estimate differences in mean intakes between two subgroups such as FSP participants and eligible nonparticipants, while controlling for other factors that affect nutrient intake. A more difficult research question, however, is testing the difference between subgroups in the prevalence of apparent nutrient inadequacy, after controlling for other factors that affect nutrient intake. This analysis involves comparing changes to the tail of the intake distributions. In the context of the FSP, the question is whether the proportion of individuals with usual intakes below the EAR is different between FSP participants and nonparticipants, after controlling for other factors that affect nutrient intake.
A proposed approach that enables users to control for effects of potentially confounding variables through regression analysis is outlined below, using the FSP as an example. The required data include:
- one day of intake data for each person
- two independent days of intake for at least a subsample of each group (however, one day of intake data on each individual suffices if only the difference in group mean intake is of interest)
- each person's values for each of the potentially confounding variables (e.g., income, education, age, etc.), or at least a reliably imputed value, as well as an indicator for FSP participation status (e.g., participant, non-participant).
Step 1. First, a regression equation is fitted to the observed intake data. Variables in the regression model would include FSP participation (coded as 0 or 1) and any other variables thought to affect intakes. For example, if age were the only other variable considered relevant, the equation would be:
Observed intake (Y) = constant + B 1 (age) + B 2 (FSP participation) + error.
The fitted regression equation would contain estimated values for the constant and the regression coefficients for FSP participation and for any other variable that was included in the model. These estimated values are denoted as b 1 , b 2 , b 3 , etc.
Step 2. Given the estimated regression coefficients from the first step, a standard predicted intake value is generated for each individual by inserting the values of the covariates for the individual, appropriately centered, into the fitted regression equation. The modifier “standard” is used because in this step, one standardizes individual intakes to those that would be observed if everyone in the sample had been, for example, the same age and had the same income. Suppose that the sample consisted of all women aged 20 to 50. A good centering or standardizing age would be 35, the midpoint of the sample age range. This step therefore, standardizes all intakes to values that would have been observed had all sampled individuals differed only in the FSP participation status. If age were the only other covariate, the standardized predicted intakes would be calculated as:
Standardized predicted intake = observed intake (Y) − b1 (age − 35),
where b 1 is the estimated regression coefficient associated with age.
If age is the only covariate (other than FSP participation) believed to be associated with intake, the standard predicted intakes above would correspond to intake values adjusted to a standard age (in this case 35). In essence, step 2 removes the effect of the covariates other than FSP participation on intakes. If the effect of age is to increase intake (i.e., if b 1 is positive), then the standard predicted intakes for individuals who are younger than 35 will be larger than the observed intake for those individuals. On the other hand, the standard predicted intakes for individuals who are older than 35 will be smaller than the intakes observed.
Step 3. Next, the effect of day-to-day variability is removed from the standardized predicted intakes to produce an adjusted usual intake distribution. This step, described previously in Chapter 4, would be done separately for the two groups. Once an adjusted usual intake distribution has been obtained (standardized, for example, to age 35) for each group of individuals, the proportion of each group with intakes below the EAR can then be determined and compared using a simple t-test.
It is important to note that:
- The estimates of prevalence of inadequacy in each of the two groups obtained using the adjusted standardized intakes will be biased, and perhaps severely so. This is because the adjusted standardized intakes have a variability that is too small. When using the standardized intakes in the adjustment procedure, one proceeds as if the regression coefficient b 1 was a known, fixed value. In reality, b 1 is an estimate, and as such has a variance that is not “added” to the variance of observed intakes. However, the difference between the prevalence estimates for the two groups will still be approximately unbiased, as long as the distribution of ages among the two participation groups is approximately similar, or as long as individuals in one group tend to be younger than individuals in the other group. If, however, all individuals in one group have ages clustered around the centering age value, while all individuals in the other group have ages that are either much lower or much higher than the centering value, then the adjustment above will lead to biased inferences about the effect of FSP participation on the prevalence of inadequacy.
- Only one covariate has been included in this example. The approach above extends naturally to the case of more than one covariate, and the same centering principle would hold. If, for example, income was a second covariate and if the range of incomes in the sample went from $10,000 to $40,000, then the appropriate centering value for income would be the midpoint ($40,000 − $10,000)/2 + $10,000 = $25,000. In this case, one would be adjusting observed intakes to look like the intakes that would have been observed if all individuals had been 35 years of age and earned $25,000.
- The adjustment above relies on the ability to accurately specify a regression model for intake. The model needs to contain all covariates thought to be associated with intake, particularly if they are also thought to be correlated with FSP participation. The estimated regression coefficients will have better statistical properties when intakes are approximately normally distributed.
The hypothetical example below (see also Table 7-3) illustrates the first four steps of this approach to assess whether FSP participation affects the mean intake of the group or the prevalence of inadequacy of nutrient A. In this example, it is suspected that age may influence intake of nutrient A and may also be associated with FSP participation. For each of a large group of individuals, 2 days of intake data are available, and the age of each individual is known. Some are FSP participants (FSP = 1) and others are not (FSP = 0). The overall group mean intake of nutrient A is 772 units. Table 7-3 shows data for six of these individuals.
TABLE 7-3
Data for Six Individuals from a (Hypothetical) Large Survey of Food Stamp Program (FSP) Participants and Nonparticipants.
Step 1. In the first step, a regression model is fitted to the intake data (column 4 of the table). The resulting prediction equation is:
Observed intake = −9 + 21.7 × age + 68.7 × FSP
Step 2. Next, standard predicted intakes are calculated for each individual for each day of intake. The regression coefficient associated with age generated from the intake data is used, but the coefficient for FSP participation and the intercept are not included. The centering value chosen for age is 35, the midpoint of the range of ages among individuals. Thus, the equation used is:
Standardized predicted intake = observed intake (Y) − 21.7 × (age − 35),
these intakes are shown in the last column of the table.
Step 3. Age-standardized intakes are then used in transformations to remove the effect of day-to-day variability, leading to age-standardized usual intake distributions for FSP participants and FSP nonparticipants (see Chapter 4). Note that these distributions will have the incorrect spread relative to the distribution of usual intakes that would be obtained if individuals had not been standardized to have the same age.
Step 4. Finally, the proportion of individuals with intakes below the EAR in each age-adjusted usual intake distribution can be compared to determine whether FSP participation affects the prevalence of nutrient inadequacy. The actual estimates of inadequacy in each group are meaningless; only the difference between the two prevalence estimates is approximately unbiased.
Cautions Regarding the Use of Binary Variables for Inadequacy
In an analysis of the probability of inadequacy, researchers might be tempted to determine differences in nutrient adequacy between two groups by obtaining an estimate of each individual's usual intake (perhaps by using the observed mean intake as the estimate) and then determining whether the individual is consuming adequate amounts of the nutrient by comparing the intake to the EAR. In this way, a categorical variable with two values (0 for inadequate, 1 for adequate) can be created and used as a response variable in a regression.
Dependent variables should not be binary variables for inadequacy, defined on the basis of nutrient intake below the EAR or below any other threshold value. This is because an individual's true requirement is unknown. Individuals whose usual nutrient intake is below the EAR may still be meeting their own nutrient requirement; while individuals whose usual nutrient intake is above the EAR may not be satisfying their individual nutrient requirement. As a result, a binary variable denoting whether an individual's usual nutrient intake is less than the EAR will misclassify some individuals. 2
A second problem associated with using a binary variable to denote nutrient inadequacy is that observed nutrient intake for an individual differs from usual nutrient intake. Therefore, some individuals will be classified as below the EAR on the basis of observed nutrient intake although their usual nutrient intake would put them above the EAR, and vice versa. In general, because of underreporting, using observed nutrient intake data overstates the proportion of individuals with usual nutrient intakes less than the EAR.
As a result of both of these considerations, a logistic regression for multivariate analysis in which the response variable is a binary variable constructed by comparing the individual's intake to the EAR will lead to biased estimates of the effects of the covariates on the probability of inadequacy.
SUMMARY
Table 7-4 summarizes these applications of the Dietary Reference Intakes (DRIs) to assess nutrient intakes of groups. Answers to many of the descriptive questions—such as those regarding the characteristics of the distribution of usual nutrient intake and differences in mean nutrient intakes between population subgroups—do not depend on the DRIs. However, determining the proportion of a group with inadequate usual nutrient intake is only possible for nutrients with Estimated Average Requirements (EARs). Determining the proportion of a group potentially at risk of adverse effects due to excessive usual nutrient intake is only possible for nutrients with Tolerable Upper Intake Levels (ULs). DRIs have not yet been established for many important nutrients and either an EAR or a UL has not yet been determined for others. An important issue, therefore, is what to do until the DRIs are established for these other nutrients. Descriptive applications (such as the example in Table 7-1) might combine information for nutrients with DRIs along with nutrients for which only the older Recommended Dietary Allowances (RDAs) or Recommended Nutrient Intakes (RNIs) are available. However, for evaluation measures (such as the example summarized in Table 7-2), nutrients or food components which do not yet have EARs and ULs under the DRI process should be omitted from applications that assess the prevalence of inadequate intakes or those at potential risk of adverse effects due to excessive intakes.
TABLE 7-4
Applications: Evaluating Dietary Survey Data.
Footnotes
- 1
Software programs exist to calculate t-tests of the differences between means when sample individuals have different survey weights and the survey has a cluster design. Software programs that can be used include SUDAAN (Software for the Statistical Analysis of Correlated Data, Research Triangle Institute, 3040 Cornwallis Road, PO Box 12194, Research Triangle Park, NC 27709-2194), WESVAR (Westat Variance, Westat, 1650 Research Blvd., Rockville, MD 20850), and PC-CARP (Personal Computer Cluster Analysis and Regression Program, Statistical Laboratory, Iowa State University, Ames, IA 50011-1210).
- 2
For a group, the percentage with usual intake less than the EAR is a good estimate of the proportion with inadequate usual nutrient intake because those individuals who are misclassified cancel each other out. That is, the individuals with usual nutrient intake less than the EAR who are still meeting their requirement are offset by the individuals with usual nutrient intake above the EAR who are not meeting their requirement (triangles A and B of Figure 4-8).
- Specific Applications: Assessing Nutrient Intakes of Groups Using the Dietary Re...Specific Applications: Assessing Nutrient Intakes of Groups Using the Dietary Reference Intakes - DRI Dietary Reference Intakes
- Hereditary Angioedema Types I and IIHereditary Angioedema Types I and IIMedGen
- C2717905[conceptid] (1)MedGen
- PREDICTED: Gracilinanus agilis diacylglycerol kinase iota (DGKI), mRNAPREDICTED: Gracilinanus agilis diacylglycerol kinase iota (DGKI), mRNAgi|2119513918|ref|XM_044677460.1|Nucleotide
- PREDICTED: Spodoptera frugiperda venom dipeptidyl peptidase 4 (LOC118263265), tr...PREDICTED: Spodoptera frugiperda venom dipeptidyl peptidase 4 (LOC118263265), transcript variant X2, mRNAgi|2306755292|ref|XM_050705198.1|Nucleotide
Your browsing activity is empty.
Activity recording is turned off.
See more...