NCBI Bookshelf. A service of the National Library of Medicine, National Institutes of Health.
National Academies of Sciences, Engineering, and Medicine; Division on Earth and Life Studies; Health and Medicine Division; Institute for Laboratory Animal Research; Board on Health Sciences Policy; Committee on the State of the Science and Future Needs for Nonhuman Primate Model Systems; Yost OC, Downey A, Ramos KS, editors. Nonhuman Primate Models in Biomedical Research: State of the Science and Future Needs. Washington (DC): National Academies Press (US); 2023 May 4.
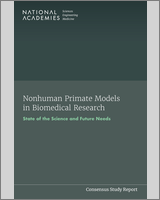
Nonhuman Primate Models in Biomedical Research: State of the Science and Future Needs.
Show detailsThere are numerous motivations—economic, logistical, social, and strategic—for transitioning away from nonhuman primates (NHPs) as research models when doing so is scientifically feasible. The COVID-19 pandemic, for example, demonstrated the importance of NHPs in biomedical research, but also the risks and challenges associated with dependence on NHPs as research models, such as bottlenecks in the development of countermeasures for public health threats that result from finite NHP resources and the lack of suitable alternatives (Hewitt et al., 2020). Accordingly, investigators using NHPs have been clear about their interest in replacing NHPs with other models if the ability of these models to answer the scientific questions under study can be established. New approach methodologies have been used to answer diverse questions of biomedical relevance, and ongoing research efforts continue to explore their potential to
- improve the translatability of nonclinical research by providing data that optimally reproduce the human condition;
- extend current knowledge of human diseases and provide opportunities to gain additional insights, as well as identify knowledge gaps;
- address shortages in the supply of NHPs by reducing the numbers required for biomedical research; and
- replace the use of NHPs in biomedical research.
In the context of this report, the term “new approach methodology” encompasses in vitro and in silico technologies and approaches that can be used to complement NHP studies or reduce reliance on NHPs in biomedical research (see Box 1-2 in Chapter 1). A technology or approach may complement NHP research, for example, if it provides additional insight into human physiology or disease. Complementary approaches may or may not help reduce reliance on NHPs, which can be achieved by substituting alternative models or decreasing the numbers of NHPs used in research. An example is the current practice of screening therapeutic candidates with new approach methodologies before progressing to an NHP model to answer questions that only such a model can answer, thereby directly reducing the number of studies conducted using NHPs and potentially reducing the likelihood that subsequent testing in NHPs will cause harm. The ability to substitute a different model to answer a specific research question does not negate the need for NHP models altogether.
Importantly, the value of in vitro and in silico models is not limited to their ability to reduce reliance on NHPs, as this is rarely the intended purpose for the development of such technologies and approaches. In fact, the committee did not identify any specific examples of studies conducted with the explicit objective of demonstrating that a new approach methodology was an effective substitute for NHPs. While there are examples discussed later in this chapter showing the potential for new approach methodologies to reduce reliance on NHPs, in vitro and in silico models are often used in ways that are complementary to NHP studies and that can help to answer different kinds of scientific questions, including those that cannot be answered using NHP models.
It is important to note that scientists conducting research are always expected to seek out nonanimal or non-NHP methods wherever feasible, and to determine that research using NHPs is the most appropriate (or only) means of answering the scientific question at hand before seeking to use them. Indeed, for proposals responding to NIH funding opportunities that involve the use of NHPs, consideration of alternatives and justification of the use of NHPs are required elements of a separate vertebrate animals section of the grant application. It should also be acknowledged that some technologies and approaches used in NHP research—such as minimally invasive and noninvasive methodologies for the collection of data (e.g., digital biomarkers, including in home enclosure recording) and samples (e.g., laparoscopy, positive reinforcement training of NHPs to voluntarily provide samples) and assays that provide results with samples of decreasing volume/size—can also contribute to reducing the numbers of NHPs used in research. Such technologies and approaches are not considered new approach methodologies for the purposes of this report but are discussed separately in Chapter 5.
As science advances and new technologies and approaches become available, the biomedical research enterprise needs to be prepared to integrate these new approach methodologies into the armamentarium available for addressing research questions related to the nation’s most pressing public health and medical needs. Research investments in exploring opportunities to accelerate the adoption of new approach methodologies are therefore important, and this chapter begins by describing a pathway for adoption of a new technology or approach. The chapter goes on to describe examples of new approach methodologies that can complement or reduce reliance on NHP models for some research questions while explaining why full replacement of NHP models is not yet feasible. The discussion turns next to recent changes in the regulatory landscape that are shaping the use of NHPs and alternatives for studies involving human safety, and then examines the needs and opportunities for facilitating collaboration between NHP researchers and those who develop and use new approach methodologies. The chapter ends with the committee’s conclusions regarding the status of new approach methodologies and the steps needed to further their adoption so as to reduce reliance on NHPs.
A PATHWAY FOR ADOPTION OF NEW APPROACH METHODOLOGIES
Translational relevance1 is the anchor for any nonclinical model system, animal or otherwise, that is intended to help understand, prevent, or treat a human condition. The principles that define translational relevance include replication of human physiology and pathophysiology (organ responses or disease state/pathogenesis), genetics, epigenetics, and/or other aspects of the biology of human cells. New approach methodologies are expected to meet translational expectations if they are to be broadly adopted to decrease reliance on NHPs in biomedical research. Attaining sufficient legitimacy based on its performance (track record) is key to successful adoption of a new approach methodology by scientists and organizations (Eckert et al., 2020; Marx et al., 2020; van der Zalm et al., 2022). Once a new approach methodology has demonstrated that it is equivalent or superior to other models for predicting or explaining human conditions or outcomes, it may be adopted as a model of choice (FDA, 2022b). Ultimately, the performance of a technology or approach determines whether it can be used to answer a specific research question. Although performance uncertainty is inherent in all new technologies and approaches, resource investments that can reduce that uncertainty are required to accelerate their adoption. This requirement is framed in the sections that follow as mapping out a pathway to adoption for a new technology or approach.
Key Terminology
Important terminology used to describe the components of a pathway to adoption for promising new approach methodologies include
- context of use,
- qualification,
- validation, and
- benchmarking.
These terms are defined here to support and provide guidance for those seeking to determine the status and credibility of an emerging technology or innovative approach for an intended use. More detailed discussion of these terms can be found elsewhere (Fabre et al., 2020; FDA, 2017; FDA and NIH, 2016; Patterson et al., 2021; Steger-Hartmann and Raschke, 2020; Tadenev and Burgess, 2019).
Context of Use
Context of use (COU) defines the manner and purpose of use for a technology or approach (how and when it will be used) (Baran et al., 2022; FDA, 2017, 2020). This term can generally be applied for any intended use of a methodology. COU elements include
- what is measured and in what form, and
- the purpose of the technology or approach in the testing of hypotheses or decision making/action.
The term “fit for purpose” is occasionally used synonymously with COU. However, a fit for purpose statement is meant to communicate that the intended use of a technology or approach is supported by validation/qualification information (FDA and NIH, 2016).
Qualification
Qualification is the process of confirming that a methodology is capable of yielding reproducible results that are suitable for the intended purpose based on the defined COU (Kennett, 2010). A technology or approach that is qualified for a specific COU can be relied upon to have a specific interpretation and application (FDA, 2017, 2020; FDA and NIH, 2016). The pathway for qualification of a new approach methodology is depicted in Figure 4-1, which shows that specifying the COU is a first step and defines the needs for the subsequent steps (Parish et al., 2020).

FIGURE 4-1
Pathway for qualification of the performance of a new approach methodology for a specific context of use.
Validation
Validation is the process by which the reliability and relevance of a technology or approach is established for a defined purpose using specific criteria (OECD, 2005). The process includes assessments of the methodology’s sensitivity, specificity, precision, robustness, reproducibility, and stability. Formal validation can involve intensive and costly processes that may not be necessary for all uses of a new approach methodology (FDA, 2017; ICCVAM, 2018); expectations may vary depending on the particular technology or approach.
Qualification versus Validation
Qualification and validation of a methodology denote the means used to demonstrate its suitability for its intended purpose (FDA, 2017; FDA and NIH, 2016). One way to describe the difference between the two terms is that they differ as to the extent and robustness of the parameters evaluated and the number of replicates performed for each. The extent to which a technology or approach needs to be qualified or validated depends on the decisions to be made with the data it generates, including the interpretation of the data and subsequent actions to be taken for a given outcome. For example, are the data intended for regulatory use, or to advance understanding and guide subsequent investigations? COU and assay qualification are intended to help decrease barriers to adoption by highlighting data that confirm a level of validation appropriate for the intended use of the methodology (FDA, 2017).
Benchmarking
Benchmarking entails rigorous comparison of the performance of different technologies or approaches to determine the strengths of each or to provide recommendations regarding their suitability for the purpose at hand (Weber et al., 2019). Benchmarking has several benefits, including increasing awareness of emerging technologies and approaches (NIH, 2023) and understanding how the performance of a new approach methodology compares with that of in vivo approaches. Benchmarking is also appropriate for comparing new approach methodologies relative to the same or different intended uses for a model, allowing for identification of the most appropriate technologies and approaches for specific COUs (Mangul et al., 2019; Wu et al., 2023).
Applying Translational Principles and Qualification/Validation of New Approach Methodologies on a Path to Reducing Reliance on NHPs
When properly qualified or validated, new approach methodologies present great potential to address specific research questions, particularly when intended to decrease reliance on NHPs in biomedical research. In considering the adoption of new approach methodologies to reduce reliance on the use of NHPs in biomedical research, it is reasonable to adopt principles of translational relevance to define qualification and/or validation pathways and to establish a well-defined COU (Low et al., 2021). Qualification schemes for emerging technologies and approaches are highly dependent on the complexity of the questions (or mechanistic hypotheses) of interest and the pathophysiology necessary to answer those questions (Ekert et al., 2020; Hargrove-Grimes et al., 2022; van der Zalm et al., 2022). Even when use of the data derived from a new technology or approach is intended to address a fundamental research question rather than to inform explicit regulatory decision making, there should be confidence that the approach can yield reliable data for its intended purpose. For example, if a new approach methodology is expected to reduce reliance on an NHP model of pulmonary fibrosis, that approach or technology would be expected to recapitulate the underlying molecular and cellular mechanisms that are present and relevant in NHP or human disease. Similarly, the use of new approach methodologies for investigational research in pharma is not defined or restricted by regulatory guidance, and many new approach methodologies are being used now in this context. In contrast, the adoption and integration of a new approach methodology to determine whether a candidate is sufficiently safe to be used in clinical trials needs to be based on proven robustness and a high level of confidence in the technology or approach (Pognan et al., 2023). The level of robustness and confidence required is often afforded by appropriately rigorous qualification and validation and ultimately determined by the intended use of the data.
Specifically, expectations for the stringency of the qualification or validation process relate to the decisions the data will inform and the potential consequences of those decisions for human health. The expectations for stringency may differ when, for example, the COU is the safety evaluation of a novel drug rather than a basic biology application, such as understanding host–pathogen interactions.
NEW APPROACH METHODOLOGIES WITH THE POTENTIAL TO COMPLEMENT OR REDUCE RELIANCE ON NHP MODELS IN BIOMEDICAL RESEARCH
This section describes new approach methodologies with the potential to complement or reduce reliance on NHP models in biomedical research. These examples are intended to be illustrative and do not represent a comprehensive cataloging of all possible new approach methodologies. Although some of these technologies and approaches have shown promise in specific applications, those applications cannot necessarily be generalized to other COUs. Rather, each COU will pose specific requirements that must be addressed when any technology or approach is intended to complement or decrease reliance on NHP models. No data set can support a summary of the validation state of new approach methodologies as a whole.
As discussed in Chapter 1, the committee did not include animal models within its definition of new approach methodologies, but with the advancement of genome editing technologies and cell/tissue transplant approaches, it should be acknowledged that engineered animal models also have the potential to complement and reduce reliance on NHP models in biomedical research.
In Vitro Cell Culture Models
The sections below provide examples of in vitro assays as well as two-dimensional (2D) and three-dimensional (3D) human cell culture models with great potential to complement NHP research and, in some cases, reduce reliance on NHPs.
In Vitro Immunoassays
In vitro immunoassays that use cultured human cells, such as assays that predict the risk of cytokine release syndrome,2 provide a clear example of how in vitro models can address a question related to human safety (Finco et al., 2014). Cytokine release syndrome is an acute systemic inflammatory syndrome that may be triggered by biologic therapeutics, such as monoclonal antibodies (Eastwood et al., 2010), including those used to treat COVID-19. There are several validated methods for assessing the potential risk that immune-modulating drugs or other treatments will trigger exaggerated levels of cytokine release in vivo (Vessillier et al., 2020). Use of in vitro cytokine release assays can aid in the prediction of human physiological responses (Joubert et al., 2016), thus reducing reliance on NHPs for this purpose.
2D and 3D Stem Cell–Based Cell Culture Models
Cell culture models may use immortalized cell lines or primary cells isolated from animals, and both sources have been used to create in vitro models for biomedical research. Given that the use of NHPs in research is driven mainly by their translational relevance to humans, this section focuses primarily on stem cell–based 2D and 3D cellular models with completely human genomes. These 2D and 3D models offer opportunities for translational relevance and may be used in specific contexts to complement or reduce reliance on NHP models, particularly if they are qualified for use and can be relied upon for reproducible results of high quality. While the committee distinguishes between 2D and 3D cell culture models for the descriptions found in this section, appropriate spatial organization and replication of organ functions are important features for both.
The discovery of human induced pluripotent stem cells (iPSCs) in 2006 by Dr. Shinya Yamanaka (2012) transformed cell culture systems and their use to answer critical human health questions. These cells are generated by introducing the Yamanaka factors (Oct3/4, Sox2, Klf4, and c-Myc) into skin fibroblasts or peripheral blood mononuclear cells, resulting in cells that can be differentiated into various cell types (Lam and Wu, 2021). Under specific differentiation conditions, most tissue/organ cell types can now be reliably generated from human iPSCs. Whereas iPSCs have the potential to generate all cell types in a human body, adult human tissue stem cells (ATSCs) have a more limited differentiation capacity (Chang et al., 2019). These tractable and renewable cell systems contain the complete human genome and can be used to study human gene functions, biochemistry, physiology, and molecular mechanisms. Both iPSCs and ATSCs can be derived from individuals of various genetic and ethnic backgrounds, offering unique advantages in understanding genetic and molecular mechanisms governing human cell biology and physiology. Moreover, iPSCs and ATSCs can be generated from patients, providing unprecedented opportunities for revealing disease-related phenotypes; understanding pathology; identifying disease-specific biomarkers; and screening for potential treatments, including personalized treatment strategies (Chang et al., 2019; Moradi et al., 2019; Son et al., 2017).
2D cell cultures are the most simplified cellular model systems. They are composed of a single or a few cell types and lack the molecular and cellular complexity and interactions of human organs under physiological conditions (Jensen and Tang, 2020). In contrast, in vitro systems that contain multiple cell types representing cells from various organs are used in attempts to recapitulate human organs and are being connected to represent multiple organs. However, since an organ is a functional entity with a need for specific structural context, formally defining an in vitro system as an organ requires that functionality and structure be recapitulated to some extent. Multiple cell types have been placed together in elegantly engineered platforms to form “organ-like” structures, and a few have recapitulated functionalities and structural context to some extent (Hofer and Lutolf, 2021; Huh et al., 2011). For example, with the application of tissue-patterning factors and self-organization capabilities, human tissue–specific 3D organoids can be generated from iPSCs (as depicted in Figure 4-2), as well as some ATSCs (Artegiani and Clevers, 2018). Organoids may be phenotypically similar to human tissues/organs in cell-type composition; architecture; and, to a certain degree, function. In general, ATSC-derived organoids specialize in mimicking the physiology of functionally more mature organs, whereas iPSC-derived organoids are useful for modeling organogenesis in a developmental context (Schutgens and Clevers, 2020).

FIGURE 4-2
Generation and differentiation of induced pluripotent stem cells. SOURCE: Image reproduced with permission from Ashutosh Agarwal and created with BioRender.com.
While recreating a whole organ or human body (which contains 78 organs) in vitro is appreciated as a futuristic goal, in the past decade human organoids with endoderm, mesoderm, and ectoderm origins have been generated that represent complex organs, including brain, liver, lung, airway, kidney, intestine, and sensory organs, among others (Artegiani and Clevers, 2018; Ho et al., 2018; Schutgens and Clevers, 2020). Compared with 2D cell culture models, human organoids are in general more complex and, in many cases, more physiologically relevant because they preserve or reconstitute the cell-type diversity of the organs in vivo. In some cases, they also maintain similarity to human organs at the molecular, cellular, and functional levels (Artegiani and Clevers, 2018; Schutgens and Clevers, 2020). Certain cells, such as milk-producing mammary gland cells, function as in vivo counterparts only when cultured under 3D conditions (Barcellos-Hoff et al., 1989). Patterned, self-assembled 3D organoids contain major cell types from the same lineage; cell types originating from different lineages, such as mesenchymal cells, vasculature cells, or immune cells, are usually not present (Ho et al., 2018; Qian et al., 2019).
Advances in 3D culture technology, microfluidics,3 and bioengineering have led to the generation of more complex microphysiological systems (MPS). MPS are in vitro platforms composed of 3D constructs (including spheroids, organoids, bioprinted tissue constructs, and tissue/organs-on-chips) of human or animal origin that mimic the biochemical, electrical, and/or mechanical properties of organ or tissue function. The design of MPS platforms allows researchers to control the composition of the tissue and its architecture using cellular and extracellular cues—molecular, structural, and mechanical—found within the human organ system (Hargrove-Grimes et al., 2021). Vasculature or immune cells, for example, can be cocultured with various types of organoids (Sun et al., 2022; Trujillo and Muotri, 2018; Yu, 2021). More complex models can be created by fusing different types of organoids generated separately to create assembloids that reconstitute the interaction of multiple tissues (Kanton and Paşca, 2022; Paşca, 2019; Paşca et al., 2022). For example, brain, spinal cord, and muscle organoids have been assembled together to model the motor control from brain to muscle (Andersen et al., 2020). Through the integration of organoids and microfluidic chips, MPS enable the engineering of a more physiologically relevant in vitro model and permit closer control over cell–cell and cell–matrix interactions, biomechanical cues, nutrient delivery, and waste removal (Huh et al., 2011; Ingber, 2022). Box 4-1 describes biomedical applications for tissue-specific organoids and MPS.
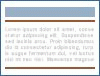
BOX 4-1
BIOMEDICAL APPLICATIONS OF TISSUE-SPECIFIC ORGANOIDS AND MPS.
The real power of MPS technology lies in the capacity of singular organ chips to reveal not only drug effects or biological response on primary human organs but also secondary effects stemming from organ–organ interactions. Therefore, multiorgan MPS are being designed to be as physiologically realistic as possible by connecting individual organ chips in a modular fashion (Ronaldson et al., 2022; Sung et al., 2019); factoring in functional organ scaling in the human body; and replicating those scaling parameters, such as organ volumes, volumetric perfusion rates, and fluid-to-cell ratios (Malik et al., 2021). While multiorgan MPS with as many as 10 organ-specific components have been generated as proof of concept (Novak et al., 2020), MPS models being used in biomedical research are generally less complex. For example, since liver is the primary site for human metabolism, several “liver–other tissue” (e.g., liver–gut, liver–heart, liver–skin, liver–kidney, liver–muscle, liver–bone marrow–tumor tissue) MPS platforms have been developed to study potential side effects of both the parent compound and its metabolites on multiple downstream organs (Chang et al., 2017; Edington et al., 2018; Maschmeyer et al., 2015; McAleer et al., 2019; Sung et al., 2010; Tsamandouras et al., 2017; Vernetti et al., 2017; Wagner et al., 2013). Outside of the drug development space, multiorgan MPS are also being used to better understand human physiology and disease mechanisms. For example, a multiorgan MPS with liver, gut, and cerebral components has been used to gain insight into pathogenic mechanisms for Parkinson’s disease (Trapecar et al., 2021). Another notable example of a complex multiorgan MPS is the EVATAR system, which includes separate organ modules for the ovary, fallopian tube, uterus, cervix, and liver within a microfluidic system to simulate the in vivo female reproductive tract. The system includes endocrine loops with a sustained circulating flow between all tissues and has demonstrated that the human female menstrual cycle can be modeled in vitro (Xiao et al., 2017).
Several scientifically rich hurdles remain. Finding an in vitro blood substitute that could perfuse all organ chips and regulation of the blood surrogate volume to mimic physiological levels are critical to obtain precise concentrations of drug/hormone/chemical metabolites in the blood surrogate volume (Malik et al., 2021; Herland et al., 2020). It is also important to build organ-specific endothelial barriers so that nutrients, oxygen, drugs, and other solutes must circulate through a common vascular conduit (Herland et al., 2020; Ronaldson et al., 2022). Ideally, each chip also requires on-chip analytical technologies so that effective functional readouts (in addition to viability and morphological analyses) can be carried out to assess the cellular responses to a specific challenge (Jalili-Firoozinezhad et al., 2019; Kujala et al., 2016; Odijk et al., 2015; van der Helm et al., 2019). If these technical challenges can be addressed, multiorgan MPS technology is poised to offer useful, cost-effective guidance in the early determination of drug toxicity and efficacy, response to hormones and endogenous metabolites, and modeling of human disease. Ultimately, the goal of multiorgan MPS efforts is not to build a perfect replicate of the human body but to provide a predictive model that is superior to animal models, including NHPs.
Cultured Tissue Slice Models
Cultured human tissue explants,4 such as precision-cut-tissue slices (PCTS), offer some advantages over the 3D stem cell–based MPS models described above in that the natural architecture of the human tissue/organ is retained, all cell types found in the tissue are present and in their original tissue–matrix configuration, and tissue- or organ-specific functions (e.g., metabolic and some aspects of immunologic activity) are preserved to a certain extent (Liu et al., 2019; Majorova et al., 2021). While tissue slices have been used in biomedical research for decades, technological advances over the years that have enabled precision cutting of slices and extended cell viability during culture have increased the utility of this model system for answering specific research questions (Alsafadi et al., 2020). PCTS models can be generated from healthy or diseased human tissues (e.g., liver, kidney, brain, lung), enabling their use in understanding fundamental human anatomy and physiology, as well as disease modeling. Their ability to closely recapitulate in vivo conditions and enable the study of interactions at the molecular, cellular, and extracellular levels has made PCTS useful human-relevant models for research related to drug discovery, toxicology, and host–pathogen interactions, among other purposes (Alsafadi et al., 2020; Majorova et al., 2021). However, finite culture viability and limited accessibility of qualified human tissue constrain the scalability of this approach.
Examples Demonstrating Potential to Complement or Reduce Reliance on NHP Models
This section provides examples of the application of 2D and 3D human cellular models in research areas in which NHPs are commonly used. These examples include research aimed at generating fundamental knowledge regarding normal human biology and translational research focused on understanding disease mechanisms, identifying potential therapeutic targets, and evaluating drug and vaccine candidates. Note that these examples are intended to be illustrative and do not represent a comprehensive cataloging of ways in which these models have complemented NHP research or reduced reliance on NHPs. Note also that, while the examples focus primarily on human cell culture models, animal cell–based models have a role in informing the development of and building confidence in human cell–based MPS, as well as in assisting in the interpretation of existing animal model data and refining the use of future animal models (NASEM, 2021).
As detailed in Chapter 2, NHPs are widely used for research on infectious diseases such as COVID-19, Zika, and malaria to understand pathogen tropism (i.e., the types of cells the pathogen infects), immune responses, and pathological outcomes, as well as for vaccine and drug testing (Doritchamou et al., 2017; Galinski, 2022; Haese et al., 2021; Liang et al., 2021; Miner and Diamond, 2017; Osuna and Whitney, 2017; Rutkai et al., 2022). With rapid advances in human stem cell research, many 2D and 3D human cell culture systems have been applied to address similar questions.
The COVID-19 pandemic in particular spurred collaborative efforts to develop and apply human cellular models to address the urgent need to understand SARS-CoV-2 pathogenesis and to develop effective therapeutic and prophylactic products (Kleinstreuer and Holmes, 2021). In the past 3 years, many studies have reported on the use of such models to elucidate cellular tropism, to study immune responses to SARS-CoV-2 and the pathology in different tissue and organs, and to facilitate drug development for COVID-19 (Busquet et al., 2020; Clevers, 2020; Monteil et al., 2020). Examples include cardiac cells recapitulating patient cardiac cytopathic features (Perez-Bermejo et al., 2021); examining immune responses in lung lineage cells (Huang et al., 2020; Youk et al., 2020); demonstrating infection and viral replication within the intestinal epithelium using intestinal organoids (Lamers et al., 2020); and studying neurotropism identified in brain organoids (Jacob et al., 2020; Samudyata et al., 2022; Wang et al., 2021). Si and colleagues (2021) demonstrated the utility of an MPS model (human airway chip) for screening antiviral therapeutics for SARS-CoV-2 and the potential to accelerate the identification of drugs previously approved for other indications that have repurposing potential.
In research on Zika virus, human brain organoids were used to suggest the causality of infection and microcephaly (Qian et al., 2016). Human iPSC–derived 2D neural cultures and 3D brain organoids were used to identify the neurotropism and pathogenesis of Zika virus infection (Qian et al., 2016; Tang et al., 2016) and to screen protective treatments (Xu et al., 2016). The Centers for Disease Control and Prevention used human stem cell–based in vitro findings, along with evidence from animal models and clinical and epidemiological evidence in humans, to conclude that Zika virus causes microcephaly (Rasmussen et al., 2016). Importantly, neural progenitor cells were found to be one of the main cellular targets in vitro (Tang et al., 2016), a finding that has been replicated in many animal models, including NHP models.
Human brain organoids are also being applied to study human immunodeficiency virus (HIV) infection of the central nervous system, including neuropathology and the establishment of viral reservoirs (dos Reis et al., 2020; Premeaux et al., 2021). Understanding of latent reservoirs is important to the development of effective curative strategies, which have remained elusive despite successes in the development of HIV therapeutics, as discussed in Chapter 2 (see Box 2-3). Findings from such in vitro human model systems can be used to better inform the design of experiments in NHP models for questions that cannot be addressed in vitro.
The complementarity of in vitro and NHP models has also been a critical component of the study of neurological disorders. The brain is the most evolutionarily advanced organ and is difficult to model in vitro because of its complex circuitry and ongoing development in response to experience. Compared with other conventional animal models, NHPs exhibit greater similarity to humans in brain structure and complexity, and as discussed in Chapter 2, research using NHP models therefore provides valuable insights into brain function and neurological disorders such as Parkinson’s disease, Alzheimer’s disease, Huntington’s disease, and stroke, many of which remain untreatable. To date, many iPSC lines have been established from patients with neuropsychiatric (Levy and Pasca, 2023; Zhang et al., 2023) and neurodegenerative disorders. Brain cells or organoids have been generated from these lines to examine the molecular and cellular changes potentially linked to disease (Mertens et al., 2018) (see also Box 4-2) and to enable “initial rapid, high-throughput screens (genetic or pharmacological) to define and characterize putative targets” (Outeiro et al., 2021, p. 835).
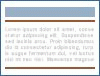
BOX 4-2
POTENTIAL OF HUMAN CELLULAR MODELS TO YIELD INSIGHTS INTO THE PATHOGENESIS AND TREATMENT OF ALZHEIMER’S DISEASE.
While brain organoids are enabling improved understanding of neurodevelopmental processes and diseases (Andrews and Kriegstein, 2022), these models currently have limitedapplicability to the study of experience-related refinement of neural circuitry (e.g., changes in response to visual cues or other postnatal stimuli) and neurological conditions that are emergent from the activity of neural networks (Andrews and Kriegstein, 2022; Jalink and Caiazzo, 2021; Trujillo and Muotri, 2018). Brain organoids cannot yet recapitulate the full complexity of the spatial organization of a human brain, have limited cellular diversity and connectivity between different brain regions (Arlotta and Gage, 2023), and demonstrate several shortcomings with respect to studying the development of neurodegenerative disorders that develop over long periods of time (Jalink and Caiazzo, 2021; Kim et al., 2021; Qian et al., 2019; Shou et al., 2020; Sun et al., 2021; Wang, 2018). Animal models, including NHPs, are needed to test mechanistic hypotheses for the development of typical symptoms and to test therapeutic strategies at the organism level (Outeiro et al., 2021). From these studies, it is clear that certain pathologic features are conserved in both in vitro and NHP models, which thus complement each other.
Given their similarities to humans, NHPs are important models in the area of reproductive biology and have contributed to improved understanding of endometriosis, as discussed in Chapter 2. Recently, complex, multicellular endometrial organoids have been developed that provide an in vitro model for the study of endometrial physiology (e.g., molecular and cellular regulation of dynamic cyclic tissue remodeling) and pathology (e.g., endometriosis), highlighting the opportunities for organoid models to contribute to both basic and translational research (Song and Fazleabas, 2021).
NHP research plays an important role in the development of safe and effective treatments that are translatable to humans. Given the relatively low cost and high throughput of in vitro culture systems, initial drug screening using disease-relevant human cellular models may significantly reduce the number of NHPs required for drug development research by advancing only promising candidates to NHP studies (Prior et al., 2017; SCHEER, 2017). The Comprehensive In Vitro Proarrhythmia Assay (CiPA) initiative, for example, which began following a 2013 workshop hosted by the Food and Drug Administration (FDA) (CiPA Initiative, 2019), resulted in validation of the use of human iPSC–derived cardiomyocytes (hiPSC-CM) to determine the risk of drugs inducing torsade des pointes tachyarrhythmias in a clinical setting (Blinova et al., 2018). The use of iPSC-derived cardiac myocytes in the CiPA initiative is one example, specifically in toxicology, of a successful approach to clearly demonstrating the utility of a 2D hiPSC-CM system for detecting drug-induced proarrhythmic effects and reducing the use of animals, including NHPs, in such research (Pang et al., 2019). In another example, Kerns and colleagues (2021) showed that a MPS could recapitulate antibody-mediated lung toxicities observed in cynomolgus macaques, demonstrating the value of the model for evaluating translation of animal findings to human cellular models, and raising the potential for a reduction in reliance on NHP-based safety assessments.
Other Applications of In Vitro Cell Culture Models
As emphasized earlier in this chapter, the usefulness of new approach methodologies extends beyond opportunities to reduce reliance on NHPs; 2D and 3D cell culture models have many valuable applications, particularly when good animal models for human disease are lacking. For example, iPSCs derived from patients suffering from familial cancers can be used to identify the mechanisms underlying the onset of pediatric cancer and to detect some familial mutations, making it possible for these cells to serve as a valuable prognostic tool (Marin Navarro et al., 2018). Disease causality for several genetic polymorphisms in long QT syndrome and aortic valve disease were demonstrated by combining the use of cardiomyocytes differentiated from iPSCs with multiomics technologies (Evans et al., 2022). Similarly, causality was established between a specific gene mutation and disease-associated phenotype using cortical neurons differentiated from iPSCs from patients with major mental disorders and genome editing (Wen et al., 2014).
A notable area of application for in vitro cell culture models is personalized medicine. In the past decade, innovative 3D culture approaches have resulted in successful derivation and cultivation of tumor organoids, including colon, breast, liver, lung, pancreas, ovary, bladder, esophagus, and brain cancer organoids. These tumor organoids have shown some similarity in drug responses to the responses of patients, making them a valuable tool for studying personalized disease progression and therapies (Veninga and Voest, 2021). In a recent example, Schuster and colleagues (2020) developed a microfluidic platform to study pancreatic tumor organoids. Using this system, hundreds of patient-derived cultures were treated with combinations of drug treatments, and an automated, high-throughput analysis of the microfluidic 3D organoids enabled the identification of potentially effective treatments for individual patients, demonstrating the potential of these culture systems to support personalized precision medicine. Importantly, the results showed significant differences in drug response among different patients, a feature that cannot be studied using NHPs. In another example, human rectal organoids from cystic fibrosis patients were used to predict drug response, enabling the identification of responders to CFTR-modulating drug therapy even for those patients with very rare genetic mutations in the CFTR gene (Dekkers et al., 2016).
Advantages and Limitations of In Vitro Models
Significant advances have been made in deriving and culturing human cells, tissues, and organs from stem cells to study basic biology and certain disease-related cellular pathologies. For example, the molecular mechanisms underlying cortical layer formation were delineated using brain organoids involving Wnt signaling, which is disrupted by a psychiatric disorder–associated genetic mutation in brain organoids derived from patient iPSCs (Qian et al., 2020). In another example, human iPSC–derived neural cells were used to identify the fundamental molecular and cellular mechanisms underlying human neoteny (Iwata et al., 2023; Linker et al., 2022).
The ability to derive ATSCs and iPSCs from patients, combined with targeted differentiation into disease-relevant cell types, enables use of these cellular models to reveal disease-related phenotypes, understand pathology, and identify potential biomarkers. The more complex cell models, such as organoids and MPS, allow better understanding of disease pathology and mechanisms using relevant human cell and tissue types, as well as physiologic niches important for disease development and progression, especially in hereditary diseases. ATSCs and iPSCs also are amenable to CRISPR/Cas-based genetic editing, thus making it possible to understand the functional contribution of specific genes involved in normal function or under diseased conditions, and to establish a causal relationship between genotype and phenotype (Evans et al., 2022; Wen et al., 2014).
However, not all human cell types can be isolated or generated from ATSCs or iPSCs, and the integrity of the cells (e.g., low rate of genotype discordance between parent cell and iPSC cell DNA, genomic structural integrity, and transcriptomic similarity between the derived cell type and the cells that reside in tissues in vivo) must be confirmed before the cells can be used reliably (Assou et al., 2018; Kammers et al., 2017; Kanchan et al., 2020). As with all models, predictivity and validation principles must be established. For translational purposes, qualification and a well-defined COU for these model systems are essential (Hargrove-Grimes et al., 2021, 2022; Ingber, 2022; Low et al., 2021) and can significantly expand the types of critical human health–related questions they can be used to explore.
While organoids and MPS have successfully mimicked many aspects of the complex physiology of human organs, 3D culture systems in their current state cannot replicate all of the complex interactions that occur in vivo (Low et al., 2021; Marx et al., 2020), nor can they be used to study processes that require systemic regulation. Therefore, for human health and safety questions that require this level of complexity, these in vitro systems cannot replace NHPs. The use of in vitro systems to study surgical interventions is limited as well (Ruspi et al., 2019). Furthermore, certain outcomes, such as behavior, can be studied only in the full context that is present in vivo. However, results from studies using in vitro models can inform improvements in the design of experiments using NHPs to reduce reliance on these animal models, such as with drug screening, as discussed earlier in this chapter.
In Silico Models
Over the years, researchers have attempted to use a wide array of quantitative and computational methods to model the properties of biological systems, including their response to perturbations, with greater or lesser degrees of success. Statistical modeling can be used to associate a relatively small number of variables with defined endpoints and can provide robust estimation of the effects of a number of parameters in inferring properties of a system. Differential equations can be fit to temporal data sets to model the response of a biological system to a perturbation and to predict responses to related challenges (Daun et al., 2008). Bayesian and Boolean networks and Markov models can be used to capture the complex interactions among parameters that characterize a biological system and to predict the responses to stimuli that alter those parameters (Chen et al., 2016; Li et al., 2007; Trairatphisan et al., 2013; Yoon, 2009). While there are many examples of the use of these and other quantitative methods in the analysis of data from biological systems, each has limitations for applications involving capturing the complexity of behavioral, biochemical, or other responses of NHPs to external stimuli.
For example, statistical inference requires that one start with a set of “outcomes” for the system under study. An underlying model can be created that is based on the variables believed to influence the outcomes, and sampling of a population can be used to estimate parameters that relate the variables to the observed outcomes; the parameterized model can then be used to infer the outcome state for new measurements on the system under study. Indeed, statistical inference can take advantage of scientists’ ability to control variability and measure a more comprehensive set of relevant parameters in NHPs as compared with other systems. The resulting models can be used to make accurate predictions across a number of readouts. However, the small number of NHPs available for use in a given study often prevents a sufficient number of measurements for the creation of statistical models with more than a few parameters, and these models would likely carry a great deal of uncertainty. Further, even if data were available for larger numbers of animals, it is unlikely that a statistical model would be able to estimate response to a system-perturbing stimulus that would be sufficiently different from responses already observed. Similarly, a differential equations model requires that one start with a predefined collection of supposed interactions that govern the evolution of the system and sufficient time-course measurements to estimate what can quickly become a large number of model parameters (Daun et al., 2008). Consequently, most models cannot capture the full complexity of NHP models and extend predictions to human systems.
Machine learning (ML), a subset of artificial intelligence (AI), has been recognized as having the potential to overcome some of the limitations of other computational modeling tools. AI/ML methods are generally capable of finding patterns in large, complex data sets that can be used to draw inferences about the system being studied (Bzdok et al., 2017; Paul et al., 2021). Improvements in both AI/ML methodology and computational speed and power have led to dramatic increases in performance capabilities (by some estimates, performance doubles every 3 months [Saran, 2019]). Over the last decade, AI/ML has been a major contributor to improvements in the speed and efficiency of in silico drug development (Jayatunga et al., 2022; Patel and Shah, 2022; Vo et al., 2020; Walden et al., 2021), with applications including molecule design (Paul et al., 2021) and the application of knowledge graphs to understand target biology (Alshahrani et al., 2022). As documented by Jayatunga and colleagues (2022), several companies have advanced molecules to clinical trials based on AI drug discovery. AI-enabled programs have been able to complete the discovery and preclinical phases within 4 years, compared with historical timelines of 5–6 years (Jayatunga et al., 2022). In particular, AI/ML has shown utility in prescreening large compound libraries to identify high-priority candidates (Dreiman et al., 2021). Although these compounds must still be tested in a model system such as NHPs, narrowing the field of drug candidates will naturally reduce the use of NHPs in validation experiments.
AI/ML methods rely on having large bodies of “training data” that can enable AI/ML models to learn rules for predicting outcomes of interest (Vo et al., 2020). For example, one might use the chemical structures of small molecules and a particular response of cell lines treated with those compounds, such as growth arrest, to train AI/ML models to recognize structural features that are predictive of the response and its magnitude. The AI/ML models thus trained could be used to evaluate thousands of structurally defined compounds in order to identify candidates that might be more effective than existing compounds or might have fewer side effects. Yet while this methodology can speed drug development by guiding the search for new agents and help design and reduce reliance on NHP studies, it does not obviate the need for in vitro or in vivo testing.
AI/ML has also seen increased applications in many areas of health care and pharmaceutical and chemical research by enabling predictions (Luechtefeld and Hartung, 2017; Luechtefeld et al., 2018), the creation of synthetic data to fill data gaps, and the extraction of complex and nonlinear relationships between input data and desired outcomes (Walden et al., 2021). With access to ever-greater amounts of digitized historical experimental data, data-driven supervised AI/ML algorithms have proven effective in a number of applications (Maharao et al., 2020; Walden et al., 2021). To improve model performance with smaller or relatively limited (sparse) data sets, ML researchers have developed new computational methods that include AI/transfer learning; one-shot, zero-shot learning; and Bayesian-based optimization methods (Walden et al., 2021). They have also developed hybrid methods that integrate mechanistic modeling with more conventional ML as a way of increasing the interpretability of AI/ML models (Antontsev et al., 2021)—something that is necessary if these models are to be used to derive biologic insight into the systems being modeled.
Potential to Complement and Reduce Reliance on NHPs
As touched on briefly in the previous section, the ability of AI/ML methods to learn from data depends on both the quantity and appropriateness of the training and validation data employed. In many instances, AI/ML tools have the potential to learn from historical NHP data and could provide insights beyond those extracted using conventional tools (Steger-Hartmann et al., 2020). Doing so with appropriate data could allow the creation of virtual NHP control groups. These arms would be modeled using previously collected data and used in place of NHPs receiving a placebo, thereby reducing the number of NHPs used in nonclinical studies—an outcome that would both increase the utility of NHP models and make better use of these models at a time when NHP availability is severely limited. This approach would require careful design of the control and treatment arms in the relevant studies to ensure that robust conclusions can be drawn (Steger-Hartmann et al., 2020; Wright et al., 2023). A hybrid approach, combining mechanistic modeling and ML (Antontsev et al., 2021), also provides an opportunity to develop virtual NHP tissue and organ models to inform formulation, design, and dosing regimens and predict toxicological and efficacy endpoints, thus enabling the execution of more informed, refined NHP studies with the potential to reduce the numbers of NHPs required. Efforts to develop virtual dog organs and tissues to model toxicologic endpoints for new drugs are currently under way (NC3RS, 2021) and if successful, could guide similar approaches for NHPs. Such efforts rely on the accumulation of mechanistic knowledge through animal studies. In many instances, however, the mechanistic models of drug metabolism and drug effects are incomplete, so the implementation of such a strategy would require additional methodologic development and validation.
It has been suggested that combining systems biology with AI/ML can help better translate data from animal (including NHP) studies to clinical research (Brubaker and Lauffenburger, 2020). This approach would overcome some of the limitations inherent in the assumption that orthologs (evolutionarily related genes in different species that carry out the same basic function) influence cellular or organismal phenotypes in the same way. Instead, the hope is that by incorporating higher-order associations among genes, RNAs, proteins, and metabolites, together with mechanistic models, AI/ML models can be developed to better predict human responses relative to those in animal models, thus helping to improve the translational relevance of data from NHP studies. Such an approach is being used in vaccine development for HIV. Building on efforts to identify correlates of protection against HIV and simian immunodeficiency virus infection (Ehrenberg et al., 2019; Fourati et al., 2019), ML models are aiding in the discovery of latent variables from NHP vaccine data that are most predictive of efficacy in human trials. By identifying biologic pathways predictive of clinical trial outcomes, such data can guide the development and evaluation of future vaccine candidates (Lauffenburger, 2022). In the area of drug development, Singh and Shah (2017) demonstrate the impact of NHP pharmacokinetic (PK) and efficacy data using multiscale PK/pharmacodynamic (PD) modeling to predict the efficacy of an antibody–drug conjugate, trastuzumab emtansine, for breast cancer in humans.
Even in these advanced settings, however, AI/ML is limited by the data available to train the models, as it cannot learn far beyond the realm of the data inputs. For example, with enough data on the observed mutations of the SARS-CoV-2 virus spike protein and information about transmissibility or disease severity, AI/ML models might be able to predict the severity of a new variant. Such models, however, would likely not be of value in predicting the transmissibility of another virus for which the structure of the capsid proteins was radically different or one with distinct modes of infection or transmission. Nor would models trained on data relevant to modeling transmission of the virus likely be of use in solving a different problem, such as the development of a new antiviral agent to fight COVID-19. The use of NHP models adds another layer of complexity to considering both how AI/ML models will be trained and validated (given the small numbers of animals available) and how those models will be used. While these problems are not insurmountable, their consideration is essential if AI/ML methods are to affect the use and utility of NHP models. Consequently, AI/ML methods are viewed as complementary to the use of NHPs and other in vitro and animal models, and in this context are recognized primarily as tools with the potential to extract additional insights from NHP studies. The application of AI/ML methods to extract insights from NHP studies is discussed further in Chapter 5.
Challenges for Adoption of AI/ML Models
A number of limitations have slowed the adoption of AI/ML models. Any computational model—and indeed any analytical model—will be limited by the ability to characterize the biological or pathophysiological phenotype of the model systems under study and to relate this information to the relevant human phenotype. While it may be easy to distinguish tumor from normal tissue, even cancers have continuous phenotypes with respect to molecular characteristics and the gross phenotype, and may have different effects in different species; most chronic diseases are even more difficult to characterize and translate between models.
Scientists conduct experiments to answer a specific question, such as what factors explain the properties of the biologic system under study. Although AI/ML methods can do an outstanding job of identifying experimental groups or classifying new samples into known groups, they often fail to provide a clear picture of how particular assignments are made. AI/ML methods work by iteratively combining multiple data elements and weighting them at each stage to arrive at the prediction of some “outcome” that is captured in the data. The result is a model that is highly nonlinear, meaning that the data elements being combined and their respective weights in the final model are often obscured from the user and impossible to extract, which in turn leads to difficulties in explaining and interpreting AI outcomes and gives rise to the “black box” character of these methods (Mathews, 2019). Consequently, some scientists remain skeptical of the results derived with these models (Baran and Henstock, 2023).
Research into creating explainable AI models is ongoing, and good results have been obtained with hybrid methods that integrate AI/ML and mechanistic modeling (Antontsev et al., 2021), but the work done in this area thus far has not made it possible to explain every output and identify sources of error. Lack of repeatability, replicability, and reproducibility may still be observed during AI/ML learning because of different software versions, implementation variations, hardware differences, and unrecognized biases in the data (Haibe-Kains et al., 2020). However, these challenges can be addressed by the identification of appropriate COUs and the application of appropriate qualification, validation, and benchmarking criteria (Baran and Henstock, 2023; FDA, 2017; Kennett, 2010; NIH, 2023; OECD, 2014; Wu et al., 2023). Also important is having both representative training and validation data sets and appropriate design for the training and validation phases of model development. There are countless examples in the literature of computational methods trained and validated using data from, for example, largely Caucasian populations when the intended application is in a population that is more diverse (Parikh et al., 2019). Other studies have mixed training and validation data sets in ways that bias the results and ultimately limit their applicability. This challenge has been widely recognized, and many publications address it, describing validation criteria and approaches for identifying and selecting training and validation data (Lin and Chou, 2022; Lu et al., 2018; Maharao et al., 2020; OECD, 2014; Sprous et al., 2010; Tropsha, 2010; Vo et al., 2020).
Lack of AI/ML expertise, training, and education has led to nonspecific use of these methods and false expectations about their applicability. Further, users often fail to recognize critical aspects of AI/ML model development, including the importance of representative, high-quality training and validation data (de Hond et al., 2022). In NHP research, a fragmented knowledge landscape, lack of data harmonization, lack of data digitization and digitalization, and limited access to NHP and human data of sufficient quality and quantity can result in poor system training and overfitting, which in turn leads to poor-quality model performance. Moreover, inadequate reporting of experimental conditions from NHP research can make it difficult to identify comparable data sets with which to train or validate AI/ML models. Advances in ML, such as those based on continuous learning techniques, along with focusing of the questions being asked, can aid in filling data gaps with accurate synthetic data in a specific COU.
Effective use of AI/ML requires careful planning of experiments to generate appropriate data. With AI/ML tools, one must identify a specific and focused question and a design that will allow collection of the appropriate data. Thus there may be many investigations that could be conducted in NHPs that would not feasibly be replaced by AI/ML modeling. In some experiments, for example, NHPs are exposed to a particular compound or agent, and a readout is obtained from various organs and systems. Such a broad question with an unknown agent whose mechanism of action is unknown would not be amenable to AI/ML analyses.
It is important to note that AI/ML has the potential to introduce new research cybersecurity requirements. At a time when life science organizations are increasingly targeted by cyberattacks (Guttieres et al., 2019), effective AI/ML development and use requires that data remain secure against unauthorized access while remaining readily accessible to peers and collaborators. The potential for obtaining NHP study data, such as video, through the Freedom of Information Act and the subsequent potential for misrepresentation also needs to be considered and addressed.
Finally, advancing the use of AI/ML models to complement and reduce reliance on NHP models will require a strong commitment to open science and data sharing—including the sharing of training data sets, computational models, and software code. The value of any such AI/ML model will be strongly driven by the data used to train and validate it, while the community’s confidence in the model’s results will depend on the transparency and understanding of the model so it can be tested and validated (Haibe-Kains et al., 2020).
REGULATORY GUIDANCE ON TECHNOLOGIES AND APPROACHES TO COMPLEMENT OR REDUCE RELIANCE ON NHP MODELS
The National Institutes of Health (NIH) has supported a few programmatic efforts with the expressed intent of enabling investigational new drug applications (INDs) (see, for example, the Bridging Interventional Development Gaps program supported by the National Center for Advancing Translational Sciences [NIH, 2022]). These efforts represent a very small percentage of the total resource allocation of the NIH institutes and centers, and do not necessarily involve research conducted with NHPs. With this in mind, this section provides some relevant information about the regulatory landscape for new approach methodologies. Notably, the regulatory views included in this section are not relevant to the use of NHPs in basic disease research and are focused on informing human safety.
In general practice, NHPs are used to answer questions about human safety when it is scientifically demonstrated and clear that NHPs are the most relevant species for human translation, and no other approach is appropriate for the purpose of the study (SCHEER, 2017). Scientific demonstration “may include metabolic, pharmacokinetic, and pharmacologic similarities to humans as well as sensitivity to particular types of toxicity, among other factors” (FDA, 2022c, p.3). The committee found evidence that the FDA and other regulatory agencies are supportive of new approach methodologies and have communicated strategies that are being considered to put this acceptance into practice (FDA, 2021a, 2022a). To this end, the FDA formed an Alternative Methods Working Group5 focused on the advancement of both new and existing models to support regulatory decision making in toxicology. The working group also facilitates interactions with global regulatory bodies that are considering the application of alternative methodologies in toxicology (FDA, 2022a). This effort and others are focused on ensuring that the data generated will be comparable and of the highest quality, and that studies using these models and the data generated from them will be robust, reproducible, relevant, and fit for purpose. In response to the 21st Century Cures Act of 2016,6 the FDA also launched its Innovative Science and Technology Approaches for New Drugs (ISTAND) pilot program, which is designed to support the development and, in some cases, qualification of novel approaches to drug development—including MPS and AI/ML-based algorithms—for use in regulatory decision making (FDA, 2021c). All of these efforts have culminated in the FDA Modernization Act 2.0,7 enacted in December 2022, which amended the Federal Food, Drug, and Cosmetic Act to explicitly permit the use of new approach methodologies, including in vitro and in silico methods, as alternatives to in vivo nonclinical testing. Taken together with the efforts already implemented at the FDA, this new law encourages the application of new approach methodologies and documents policy efforts to facilitate their use as they are qualified and/or validated.
New FDA guidance with direct relevance to the reliance on NHPs for conducting nonclinical toxicity assessments is included in Nonclinical Considerations for Mitigating Nonhuman Primate Supply Constraints Arising from the COVID-19 Pandemic (FDA, 2022c). The FDA provided this guidance to address challenges related to the limited NHP supply resulting from the pandemic. The guidance states: “FDA supports the principles of the 3Rs, to reduce, refine, and replace animal use in testing when feasible. We encourage sponsors to consult with us if they wish to use a nonanimal testing method they believe is suitable, adequate, validated, and feasible. We will consider if such an alternative method is adequate to meet the regulatory need.” Although this guidance was prompted by the COVID-19 pandemic, the core issue was the reduction in the already limited NHP supply available for nonclinical toxicity assessments, together with the simultaneous increase in demand for NHPs for evaluating candidate COVID-19 treatments and vaccines. In response, the FDA acknowledged the restricted availability of NHPs for other pharmaceutical development programs and potential delays in the development of new medications for diseases that currently lack any effective treatment options. Specifically, this guidance discourages “the use of NHPs for the general toxicology assessment of small molecule drugs unless the sponsor can provide a scientifically compelling reason why NHPs must be used.” The guidance also states:
- “If the biological product is active in other nonrodent species, the sponsor should conduct any warranted general toxicity studies in a nonrodent species other than the NHP, whenever scientifically justified.”
- “On a case-by-case basis, if the biological product is active in a rodent and acts on a well-characterized target (e.g., vascular endothelial growth factor, or its receptor), it may be scientifically appropriate for sponsors to conduct warranted general toxicity studies only in the rodent.”
- “Sponsors should not use sexually mature NHPs in toxicity studies designed specifically to assess fertility by histopathological examination when fertility parameters can be assessed in rodents.”
- “Consistent with current FDA guidance on the assessment of developmental and reproductive toxicity (DART), FDA considers NHPs to be a nonroutine test species for the DART assessment of small molecule drugs. FDA strongly discourages sponsors from using NHPs for assessing DART endpoints for their small molecule drug development programs.”
- “Consistent with current FDA guidance, sponsors should only use NHPs for the DART assessment of biological products if they are the only relevant species. For the duration of the COVID-19-related disruption in the supply of NHPs, sponsors should also consider the following when planning approaches to address DART for biological products that are pharmacologically inactive in non-primates: […] while the supply of NHPs is disrupted, we strongly encourage the use of appropriate alternative models for assessing DART endpoints (e.g., species-specific surrogates in rodents, genetically modified rodents) when scientifically justified.” (FDA, 2022c).
In general, this guidance provides many approaches that can be considered to reduce reliance on NHPs even after the end of the COVID-19 public health crisis.
Another relevant FDA source is Advancing Regulatory Science at FDA: Focus Areas of Regulatory Science (FDA, 2021b). The section of this document on “Novel Technologies to Improve Predictivity of Non-clinical Studies and Replace, Reduce, and Refine Reliance on Animal Testing” (p. 31) describes the FDA’s intention “to replace, reduce, and refine (the 3 Rs) dependence on animal studies by advancing development of, and evaluating new, fit-for-purpose nonclinical tools, standards, and approaches that may someday improve predictability.” In the document, it is recognized that in silico modeling, such as using “available information in computational science approaches to predict safety issues, can be used to supplement and may potentially replace some risk analyses that are currently based on animal data.” Of note, the document also expresses that these advances will depend on the development of performance criteria for the realistic assessment of the potential use of these new tools for safety and efficacy testing, and the conduct of “large multilaboratory studies to assess the reliability, sensitivity, specificity, and reproducibility of in vitro alternatives to in vivo assays (e.g., to assess the potency of certain vaccines).”
NEED FOR COLLABORATION BETWEEN NHP RESEARCHERS AND THOSE DEVELOPING AND USING NEW APPROACH METHODOLOGIES
The establishment of collaborations between developers of new approach methodologies and those who currently use NHPs may reduce barriers to the adoption of new approach methodologies (ICCVAM, 2018). These collaborations might entail training NHP researchers to implement new technologies and approaches in their own laboratories or creating a partnership whereby research with different model systems would be conducted in different laboratories. Effective collaborations involving multiple laboratories with expertise in different model systems are facilitated by supportive funding mechanisms. For example, the Collaborative Research in Computational Neuroscience program—a joint program of the National Science Foundation and NIH—supports collaborative research projects in theoretical and experimental neuroscience that involve multiple investigators using both animal models (including NHPs) and computational models (Flanders, 2022). Coordination within NIH—for example, between programs supporting NHP research and those developing MPS for Alzheimer’s disease research—could facilitate the pursuit of promising research goals in this space. Additionally, the inclusion of experts in new approach methodologies in the review process for NIH research proposals involving NHP models (including review of the consideration of alternatives in the vertebrate animals section of the proposal) could help identify opportunities for collaborative research that might complement the proposed NHP study or reduce the need for NHPs.
At present, interactions between research groups developing and using new approach methodologies and those using NHP models are limited. Such interactions could help raise awareness among NHP researchers regarding the evolving capabilities of in vitro and in silico systems, and could educate investigators developing new approach methodologies on the needs and priorities of NHP researchers, thereby enabling improvements in the design of in vitro systems such that they would be better suited to answering research questions for which NHPs are currently used. For example, this approach could be used to define COUs to ensure that systems would be designed to be fit for purpose. Accordingly, there is an urgent need for mechanisms that can facilitate such direct interaction and collaboration, including
- multilaboratory funding opportunities, such as those supported by the Collaborative Research in Computational Neuroscience program;
- challenge programs that encourage cross-sector collaborations, such as that supporting the application of data from experimental dog studies to the development of a virtual “second species” for toxicology studies;
- cross-training programs; and
- conferences, symposiums, and other events designed to provide opportunities for interaction between those investigators with expertise in the evolving capabilities of new approach methodologies and those using animal models, and to catalyze collaborations.
CONCLUSIONS
In its examination of new approach methodologies, the committee identified numerous ways in which in vitro and in silico models are being used to complement NHP research, but few concrete examples of a demonstrated role for those models in reducing reliance on NHPs. While references to replacement of NHPs are aspirational at this time, the committee envisions the potential for new approach methodologies to reduce reliance on NHPs in the future, especially as technology and science continue to advance, and as investments are made in the development of fit-for-purpose model systems and in their validation and qualification. In the absence of validation, enthusiasm for new technologies and approaches must be tempered to avoid overpromising their capabilities as valid replacements for necessary and proven experimental systems. Based on its evaluation of the research and development status of new approach methodologies, the committee reached the following conclusions:
Conclusion 4-1: Based on the current state of the science, there are no alternative approaches that can replace nonhuman primate (NHP) models to answer research questions that require complete multiorgan interactions and integrated biology. Thus, NHPs continue to be essential for the conduct of National Institutes of Health–supported biomedical research.
Conclusion 4-2: Select new approach methodologies (in vitro and in silico models) can replicate certain complex cellular interactions and functions. As such, these new approach methodologies may be used to answer specific research questions that contribute to understanding human biology to prevent and treat human disease. Although there currently exist no alternatives that can fully replace nonhuman primates, it is reasonable to be optimistic that this may change in the years ahead as new approach methodologies continue to advance.
Conclusion 4-3: Furthering the adoption of new approach methodologies (including in vitro and in silico model systems and approaches) with the intent of reducing reliance on nonhuman primate models will require planning and support for studies that can demonstrate adequate performance for specific contexts of use or intended purposes. Expectations for qualification or validation of new approach methodologies depend on the decisions to be made using the data derived from their use and the potential human health consequences of those decisions.
Conclusion 4-4: While nonhuman primates have been regarded as preeminent models for the evaluation of human safety and efficacy, recent guidance demonstrates that the Food and Drug Administration and other regulatory agencies are supportive of the use of new technologies and approaches for regulatory decision making once they have been adequately qualified or validated.
Conclusion 4-5: Efforts to reduce reliance on nonhuman primates (NHPs) in biomedical research will require investment in opportunities to facilitate direct interaction and collaborative research among investigators using NHP models and those developing in vitro and in silico approaches to expand the applicability of new approach methodologies to research questions for which NHPs are currently needed. At present, however, few mechanisms for fostering such interaction and collaborative research are available.
REFERENCES
- Alsafadi HN, Uhl F, Pineda R, Bailey K, Rojas M, Wagner D, Königshoff M. Applications and approaches for three-dimensional precision-cut lung slices. Disease modeling and drug discovery. American Journal of Respiratory Cell and Molecular Biology. 2020;62(6):681–691. [PMC free article: PMC7401444] [PubMed: 31991090]
- Alshahrani M, Almansour A, Alkhaldi A, Thafar MA, Uludag M, Essack M, Hoehndorf R. Combining biomedical knowledge graphs and text to improve predictions for drug-target interactions and drug-indications. PeerJ. 2022;10:e13061. [PMC free article: PMC8988936] [PubMed: 35402106]
- Alzheimer A. About a peculiar disease of the cerebral cortex. By Alois Alzheimer, 1907 (translated by L. Jarvik and H. Greenson). Alzheimer Disease and Associated Disorders. 1987;1(1):3–8. [PubMed: 3331112]
- Andersen J, Revah O, Miura Y, Thom N, Amin ND, Kelley KW, Singh M, Chen X, Thete MV, Walczak EM, Vogel H, Fan HC, Paşca SP. Generation of functional human 3D cortico-motor assembloids. Cell. 2020;183(7):1913–1929.e1926. [PMC free article: PMC8711252] [PubMed: 33333020]
- Andrews MG, Kriegstein AR. Challenges of organoid research. Annual Review of Neuroscience. 2022;45:23–39. [PMC free article: PMC10559943] [PubMed: 34985918]
- Antontsev V, Jagarapu A, Bundey Y, Hou H, Khotimchenko M, Walsh J, Varshney J. A hybrid modeling approach for assessing mechanistic models of small molecule partitioning in vivo using a machine learning-integrated modeling platform. Scientific Reports. 2021;11(1):11143. [PMC free article: PMC8160209] [PubMed: 34045592]
- Arlotta P, Gage FH. Neural organoids and the quest to understand and treat psychiatric disease. Biological Psychiatry. 2023;93(7):588–589. [PubMed: 36889858]
- Artegiani B, Clevers H. Use and application of 3D-organoid technology. Human Molecular Genetics. 2018;27(R2):R99–R107. [PubMed: 29796608]
- Assou S, Bouckenheimer J, De Vos J. Concise review: Assessing the genome integrity of human induced pluripotent stem cells: What quality control metrics? Stem Cells. 2018;36(6):814–821. [PubMed: 29441649]
- Bali J, Gheinani AH, Zurbriggen S, Rajendran L. Role of genes linked to sporadic Alzheimer’s disease risk in the production of β-amyloid peptides. Proceedings of the National Academy of Sciences. 2012;109(38):15307–15311. [PMC free article: PMC3458335] [PubMed: 22949636]
- Baran S, Henstock P. SSRN. 2022. Pathways for successful AI adoption in drug. http://dx
.doi.org/10.2139/ssrn.4305068 . - Baran SW, Brown PC, Baudy AR, Fitzpatrick SC, Frantz C, Fullerton A, Gan J, Hardwick RN, Hillgren KM, Kopec AK, Liras JL, Medrick DL, Nagao R, Proctor WR, Ramsden D, Ribeiro AJS, Stresser D, Sung KE, Sura R, Wagoner CW, Wang Q, Arslan SY, Yoder G, Ekert JE. Perspectives on the evaluation and adoption of complex in vitro models in drug development: Workshop with the FDA and the pharmaceutical industry (IQ MPS affiliate). ALTEX. 2022;39(2) [PubMed: 35064273]
- Barcellos-Hoff MH, Aggeler J, Ram TG, Bissell MJ. Functional differentiation and alveolar morphogenesis of primary mammary cultures on reconstituted basement membrane. Development. 1989;105(2):223–235. [PMC free article: PMC2948482] [PubMed: 2806122]
- Berthier E, Young E, Beebe D. Engineers are from PDMS-land, biologists are from polystyrenia. Lab on a Chip. 2012;12:1224–1237. https://doi
.org/10.1039/C2LC20982A . [PubMed: 22318426] - Blinova K, Dang Q, Millard D, Smith G, Pierson J, Guo L, Brock M, Lu HR, Kraushaar U, Zeng H, Shi H, Zhang X, Sawada K, Osada T, Kanda Y, Sekino Y, Pang L, Feaster TK, Kettenhofen R, Stockbridge N, Strauss DG, Gintant G. International multisite study of human-induced pluripotent stem cell-derived cardiomyocytes for drug proarrhythmic potential assessment. Cell Reports. 2018;24(13):3582–3592. [PMC free article: PMC6226030] [PubMed: 30257217]
- Bowles KR, Silva MC, Whitney K, Bertucci T, Berlind JE, Lai JD, Garza JC, Boles NC, Mahali S, Strang KH, Marsh JA, Chen C, Pugh DA, Liu Y, Gordon RE, Goderie SK, Chowdhury R, Lotz S, Lane K, Crary JF, Haggarty SJ, Karch CM, Ichida JK, Goate AM, Temple S. ELAVL4, splicing, and glutamatergic dysfunction precede neuron loss in MAPT mutation cerebral organoids. Cell. 2021;184(17):4547–4563.e4517. [PMC free article: PMC8635409] [PubMed: 34314701]
- Brubaker DK, Lauffenburger DA. Translating preclinical models to humans. Science. 2020;367(6479):742–743. [PubMed: 32054749]
- Busquet F, Hartung T, Pallocca G, Rovida C, Leist M. Harnessing the power of novel animal-free test methods for the development of COVID-19 drugs and vaccines. Archives of Toxicology. 2020;94(6):2263–2272. [PMC free article: PMC7245508] [PubMed: 32447523]
- Bzdok D, Krzywinski M, Altman N. Machine learning: a primer. Nature Methods. 2017;14:1119–1120. [PMC free article: PMC5905345] [PubMed: 29664466]
- CDC (Centers for Disease Control and Prevention). Common eye disorders and diseases. 2022. [February 21, 2023]. https://www
.cdc.gov/visionhealth /basics/ced . - Chang EA, Jin SW, Nam MH, Kim SD. Human induced pluripotent stem cells: Clinical significance and applications in neurologic diseases. Journal of Korean Neurosurgical Society. 2019;62(5):493–501. [PMC free article: PMC6732359] [PubMed: 31392877]
- Chang SY, Weber EJ, Sidorenko VS, Chapron A, Yeung CK, Gao C, Mao Q, Shen D, Wang J, Rosenquist TA, Dickman KG, Neumann T, Grollman AP, Kelly EJ, Himmelfarb J, Eaton DL. Human liver-kidney model elucidates the mechanisms of aristolochic acid nephrotoxicity. JCI Insight. 2017;2(22):e95978. [PMC free article: PMC5752374] [PubMed: 29202460]
- Chen P, Liu R, Li Y, Chen L. Detecting critical state before phase transition of complex biological systems by hidden Markov model. Bioinformatics (Oxford, England). 2016;32(14):2143–2150. [PubMed: 27153710]
- Chow VW, Mattson MP, Wong PC, Gleichmann M. An overview of APP processing enzymes and products. NeuroMolecular Medicine. 2010;12(1):1–12. [PMC free article: PMC2889200] [PubMed: 20232515]
- CiPA (Comprehensive in vitro Proarrhythmia Assay) Initiative. CIPA Initiative. 2019. [January 30, 2023]. https://cipaproject
.org . - Clevers H. COVID-19: Organoids go viral. Nature Reviews Molecular Cell Biology. 2020;21(7):355–356. [PMC free article: PMC7262486] [PubMed: 32483314]
- Cross N, van Steen C, Zegaoui Y, Satherley A, Angelillo L. Retinitis pigmentosa: Burden of disease and current unmet needs. Clinical Ophthalmology. 2022;16:1993–2010. [PMC free article: PMC9232096] [PubMed: 35757022]
- Daun S, Rubin J, Vodovotz Y, Clermont G. Equation-based models of dynamic biological systems. Journal of Critical Care. 2008;23(4):585–594. [PMC free article: PMC6698907] [PubMed: 19056027]
- de Hond AAH, Leeuwenberg AM, Hooft L, Kant IMJ, Nijman SWJ, van Os HJA, Aardoom JJ, Debray TPA, Schuit E, van Smeden M, Reitsma JB, Steyerberg EW, Chavannes NH, Moons KGM. Guidelines and quality criteria for artificial intelligence-based prediction models in healthcare: A scoping review. NPJ Digital Medicine. 2022;5(1):2. [PMC free article: PMC8748878] [PubMed: 35013569]
- Dekkers JF, Berkers G, Kruisselbrink E, Vonk A, de Jonge HR, Janssens HM, Bronsveld I, van de Graaf EA, Nieuwenhuis EE, Houwen RH, Vleggaar FP, Escher JC, de Rijke YB, Majoor CJ, Heijerman HG, de Winter-de Groot KM, Clevers H, van der Ent CK, Beekman JM. Characterizing responses to CFTR-modulating drugs using rectal organoids derived from subjects with cystic fibrosis. Science Translational Medicine. 2016;8(344):344ra384. [PubMed: 27334259]
- Donkers JM, Eslami Amirabadi H, van de Steeg E. Intestine-on-a-chip: Next level in vitro research model of the human intestine. Current Opinion in Toxicology. 2021;25:6–14.
- Doritchamou J, Teo A, Fried M, Duffy P. Malaria in pregnancy: The relevance of animal models for vaccine development. Lab Animal. 2017;46(10):388–398. [PMC free article: PMC6771290] [PubMed: 28984865]
- Dos Reis RS, Sant S, Keeney H, Wagner MCE, Ayyavoo V. Modeling HIV-1 neuropathogenesis using three-dimensional human brain organoids (HBOrgs) with HIV-1 infected microglia. Scientific Reports. 2020;10(1):15209. [PMC free article: PMC7494890] [PubMed: 32938988]
- Dreiman GHS, Bictash M, Fish PV, Griffin L, Svensson F. Changing the HTS paradigm: AI-driven iterative screening for hit finding. SLAS Discovery. 2021;26(2):257–262. [PMC free article: PMC7838329] [PubMed: 32808550]
- Eastwood D, Findlay L, Poole S, Bird C, Wadhwa M, Moore M, Burns C, Thorpe R, Stebbings R. Monoclonal antibody TGN1412 trial failure explained by species differences in CD28 expression on CD4+ effector memory T-cells. British Journal of Pharmacology. 2010;161(3):512–526. [PMC free article: PMC2990151] [PubMed: 20880392]
- Edington CD, Chen WLK, Geishecker E, Kassis T, Soenksen LR, Bhushan BM, Freake D, Kirschner J, Maass C, Tsamandouras N, Valdez J, Cook CD, Parent T, Snyder S, Yu J, Suter E, Shockley M, Velazquez J, Velazquez JJ, Stockdale L, Papps JP, Lee I, Vann N, Gamboa M, LaBarge ME, Zhong Z, Wang X, Boyer LA, Lauffenburger DA, Carrier RL, Communal C, Tannenbaum SR, Stokes CL, Hughes DJ, Rohatgi G, Trumper DL, Cirit M, Griffith LG. Interconnected microphysiological systems for quantitative biology and pharmacology studies. Scientific Reports. 2018;8(1):4530. [PMC free article: PMC5852083] [PubMed: 29540740]
- Ekert JE, Deakyne J, Pribul-Allen P, Terry R, Schofield C, Jeong CG, Storey J, Mohamet L, Francis J, Naidoo A, Amador A, Klein JL, Rowan W. Recommended guidelines for developing, qualifying, and implementing complex in vitro models (CIVMs) for drug discovery. SLAS Discovery. 2020;25(10):1174–1190. [PubMed: 32495689]
- Ehrenberg PK, Shangguan S, Issac B, Alter G, Geretz A, Izumi T, Bryant C, Eller MA, Wegmann F, Apps R, Creegan M, Bolton DL, Sekaly RP, Robb ML, Gramzinski RA, Pau MG, Schuitemaker H, Barouch DH, Michael NL, Thomas R. A vaccine-induced gene expression signature correlates with protection against SIV and HIV in multiple trials. Science Translational Medicine. 2019;11(507) [PMC free article: PMC7383941] [PubMed: 31462510]
- Evans PC, Davidson SM, Wojta J, Bäck M, Bollini S, Brittan M, Catapano AL, Chaudhry B, Cluitmans M, Gnecchi M, Guzik TJ, Hoefer I, Madonna R, Monteiro JP, Morawietz H, Osto E, Padró T, Sluimer JC, Tocchetti CG, Van der Heiden K, Vilahur G, Waltenberger J, Weber C. From novel discovery tools and biomarkers to precision medicine-basic cardiovascular science highlights of 2021/22. Cardiovascular Research. 2022;118(13):2754–2767.
- Fabre K, Berridge B, Proctor WR, Ralston S, Will Y, Baran SW, Yoder G, Van Vleet TR. Introduction to a manuscript series on the characterization and use of microphysiological systems (MPS) in pharmaceutical safety and ADME applications. Lab on a Chip. 2020;20(6):1049–1057. [PubMed: 32073020]
- Fan L, Mao C, Hu X, Zhang S, Yang Z, Hu Z, Sun H, Fan Y, Dong Y, Yang J, Shi C, Xu Y. New insights into the pathogenesis of Alzheimer’s disease. Frontiers in Neurology. 2019;10:1312. [PMC free article: PMC6965067] [PubMed: 31998208]
- FDA (Food and Drug Administration). FDA’ s predictive toxicology roadmap. 2017. [February 27, 2023]. https://www
.fda.gov/media /109634/download . - FDA. Qualification process for drug development tools: Guidance for industry and FDA staff. 2020. [March 23, 2023]. https://www
.fda.gov/media /133511/download . - FDA. Advancing new alternative methodologies at FDA. 2021a. [February 27, 2023]. https://www
.fda.gov/media /144891/download . - FDA. Advancing regulatory science at FDA: Focus areas of regulatory sciences (FARS). 2021b. [February 27, 2023]. https://www
.fda.gov/media /145001/download . - FDA. Innovative science and technology approaches for new drugs (ISTAND) pilot program. 2021c. [February 27, 2023]. https://www
.fda.gov/drugs /drug-development-tool-ddt-qualification-programs /innovative-science-and-technologyapproaches-new-drugs-istand-pilot-program . - FDA. Advancing alternative methods at FDA. 2022a. [February 27, 2023]. https://www
.fda.gov/science-research /about-science-researchfda /advancing-alternative-methods-fda . - FDA. New Approach Methods (NAMs). 2022b. [February 17, 2023]. https://www
.fda.gov/food /toxicology-research /new-approach-methods-nams . - FDA. Nonclinical considerations for mitigating nonhuman primate supply constraints arising from the COVID-19 pandemic. 2022c. [February 27, 2023]. https://www
.fda.gov/media /155950/download . - FDA and NIH. BEST (Biomarkers, EndpointS, and other Tools) Resource. 2016. [March 23, 2023]. https://www
.ncbi.nlm .nih.gov/books/NBK326791/ [PubMed: 27010052] - Finco D, Grimaldi C, Fort M, Walker M, Kiessling A, Wolf B, Salcedo T, Faggioni R, Schneider A, Ibraghimov A, Scesney S, Serna D, Prell R, Stebbings R, Narayanan PK. Cytokine release assays: Current practices and future directions. Cytokine. 2014;66(2):143–155. [PubMed: 24412476]
- Flanders M. Document provided to the Committee on the State of the Science and Future Needs for Nonhuman Primate Model Systems on November 21, 2022. 2022. NHP Panel written response. Available by request through the National Academies’ Public Access Records Office.
- Forro C, Caron D, Angotzi GN, Gallo V, Berdondini L, Santoro F, Palazzolo G, Panuccio G. Electrophysiology read-out tools for brain-on-chip biotechnology. Micromachines (Basel). 2021;12(2) [PMC free article: PMC7912435] [PubMed: 33498905]
- Fourati S, Ribeiro SP, Blasco Tavares Pereira Lopes F, Talla A, Lefebvre F, Cameron M, Kaewkungwal J, Pitisuttithum P, Nitayaphan S, Rerks-Ngarm S, Kim JH, Thomas R, Gilbert PB, Tomaras GD, Koup RA, Michael NL, McElrath MJ, Gottardo R, Sékaly R-P. Integrated systems approach defines the antiviral pathways conferring protection by the RV144 HIV vaccine. Nature Communications. 2019;10(1):863. [PMC free article: PMC6382801] [PubMed: 30787294]
- Galinski MR. Systems biology of malaria explored with nonhuman primates. Malaria Journal. 2022;21(1) [PMC free article: PMC9172618] [PubMed: 35672852]
- Glenner GG, Wong CW. Alzheimer’s disease: Initial report of the purification and characterization of a novel cerebrovascular amyloid protein. Biochemical and Biophysical Research Communications. 1984;120(3):885–890. [PubMed: 6375662]
- Gough A, Soto-Gutierrez A, Vernetti L, Ebrahimkhani MR, Stern AM, Taylor DL. Human biomimetic liver microphysiology systems in drug development and precision medicine. Nature Reviews Gastroenterology and Hepatology. 2021;18(4):252–268. [PMC free article: PMC9106093] [PubMed: 33335282]
- Guttieres D, Stewart S, Wolfrum J, Springs S. Cyberbiosecurity in advanced manufacturing models. Frontiers in Bioengineering and Biotechnology. 2019;7 [PMC free article: PMC6737271] [PubMed: 31552236]
- Habibey R, Rojo Arias JE, Striebel J, Busskamp V. Microfluidics for neuronal cell and circuit engineering. Chemical Reviews. 2022;122(18):14842–14880. [PMC free article: PMC9523714] [PubMed: 36070858]
- Haese NN, Roberts VHJ, Chen A, Streblow DN, Morgan TK, Hirsch AJ. Nonhuman primate models of Zika virus infection and disease during pregnancy. Viruses. 2021;13(10) [PMC free article: PMC8539636] [PubMed: 34696518]
- Haibe-Kains B, Adam GA, Hosny A, Khodakarami F, Waldron L, Wang B, McIntosh C, Goldenberg A, Kundaje A, Greene CS, Broderick T, Hoffman MM, Leek JT, Korthauer K, Huber W, Brazma A, Pineau J, Tibshirani R, Hastie T, Ioannidis JPA, Quackenbush J, Aerts H. Transparency and reproducibility in artificial intelligence. Nature. 2020;586(7829):E14–e16. [PMC free article: PMC8144864] [PubMed: 33057217]
- Hargrove-Grimes P, Low LA, Tagle DA. Microphysiological systems: What it takes for community adoption. Experimental Biology and Medicine. 2021;246(12):1435–1446. [PMC free article: PMC8243212] [PubMed: 33899539]
- Hargrove-Grimes P, Low LA, Tagle DA. Microphysiological systems: Stakeholder challenges to adoption in drug development. Cells, Tissues, Organs. 2022;211(3):269–281. [PMC free article: PMC8831652] [PubMed: 34380142]
- Harrison SP, Baumgarten SF, Verma R, Lunov O, Dejneka A, Sullivan GJ. Liver organoids: Recent developments, limitations and potential. Frontiers in Medicine. 2021;8:574047. [PMC free article: PMC8131532] [PubMed: 34026769]
- Herland A, Maoz BM, Das D, Somayaji MR, Prantil-Baun R, Novak R, Cronce M, Huffstater T, Jeanty SSF, Ingram M, Chalkiadaki A, Benson Chou D, Marquez S, Delahanty A, Jalili-Firoozinezhad S, Milton Y, Sontheimer-Phelps A, Swenor B, Levy O, Parker KK, Przekwas A, Ingber DE. Quantitative prediction of human pharmacokinetic responses to drugs via fluidically coupled vascularized organ chips. Nature Biomedical Engineering. 2020;4(4):421–436. [PMC free article: PMC8011576] [PubMed: 31988459]
- Hewitt JA, Lutz C, Florence WC, Pitt MLM, Rao S, Rappaport J, Haigwood NL. ACTIVating resources for the COVID-19 pandemic: In vivo models for vaccines and therapeutics. Cell Host and Microbe. 2020;28(5):646–659. [PMC free article: PMC7528903] [PubMed: 33152279]
- Ho BX, Pek NMQ, Soh BS. Disease modeling using 3D organoids derived from human induced pluripotent stem cells. International Journal of Molecular Sciences. 2018;19(4) [PMC free article: PMC5979503] [PubMed: 29561796]
- Hofer M, Lutolf MP. Engineering organoids. Nature Reviews Materials. 2021;6(5):402–420. [PMC free article: PMC7893133] [PubMed: 33623712]
- Holder GE. Pattern electroretinography (PERG) and an integrated approach to visual pathway diagnosis. Progress in Retinal and Eye Research. 2001;20(4):531–561. [PubMed: 11390258]
- Homan KA, Kolesky DB, Skylar-Scott MA, Herrmann J, Obuobi H, Moisan A, Lewis JA. Bioprinting of 3D convoluted renal proximal tubules on perfusable chips. Scientific Reports. 2016;6(1):34845. [PMC free article: PMC5057112] [PubMed: 27725720]
- Homan KA, Gupta N, Kroll KT, Kolesky DB, Skylar-Scott M, Miyoshi T, Mau D, Valerius MT, Ferrante T, Bonventre JV, Lewis JA, Morizane R. Flow-enhanced vascularization and maturation of kidney organoids in vitro. Nature Methods. 2019;16(3):255–262. [PMC free article: PMC6488032] [PubMed: 30742039]
- Huang J, Hume AJ, Abo KM, Werder RB, Villacorta-Martin C, Alysandratos KD, Beermann ML, Simone-Roach C, Lindstrom-Vautrin J, Olejnik J, Suder EL, Bullitt E, Hinds A, Sharma A, Bosmann M, Wang R, Hawkins F, Burks EJ, Saeed M, Wilson AA, Mühlberger E, Kotton DN. SARS-CoV-2 infection of pluripotent stem cell-derived human lung alveolar type 2 cells elicits a rapid epithelial-intrinsic inflammatory response. Cell Stem Cell. 2020;27(6):962–973.e967. [PMC free article: PMC7500949] [PubMed: 32979316]
- Huh D, Hamilton GA, Ingber DE. From 3D cell culture to organs-on-chips. Trends in Cell Biology. 2011;21(12):745–754. [PMC free article: PMC4386065] [PubMed: 22033488]
- Hutton M, Lendon CL, Rizzu P, Baker M, Froelich S, Houlden H, Pickering-Brown S, Chakraverty S, Isaacs A, Grover A, Hackett J, Adamson J, Lincoln S, Dickson D, Davies P, Petersen RC, Stevens M, de Graaff E, Wauters E, van Baren J, Hillebrand M, Joosse M, Kwon JM, Nowotny P, Che LK, Norton J, Morris JC, Reed LA, Trojanowski J, Basun H, Lannfelt L, Neystat M, Fahn S, Dark F, Tannenberg T, Dodd PR, Hayward N, Kwok JBJ, Schofield PR, Andreadis A, Snowden J, Craufurd D, Neary D, Owen F, Oostra BA, Hardy J, Goate A, van Swieten J, Mann D, Lynch T, Heutink P. Association of missense and 5’-splice-site mutations in tau with the inherited dementia FTDP-17. Nature. 1998;393(6686):702–705. [PubMed: 9641683]
- ICCVAM. A strategic roadmap for establishing new approaches to evaluate the safety of chemicals and medical products in the United States. 2018. [March 23, 2023]. https://ntp
.niehs.nih .gov/iccvam/docs/roadmap /iccvam_strategicroadmap _january2018_document_508.pdf . - Ingber DE. Human organs-on-chips for disease modelling, drug development and personalized medicine. Nature Reviews Genetics. 2022;23:467–491. [PMC free article: PMC8951665] [PubMed: 35338360]
- Iwata R, Casimir P, Erkol E, Boubakar L, Planque M, Gallego López I, Ditkowsky M, Gaspariunaite V, Beckers S, Remans D, Vints K, Vandekeer A, Poovathingal S, Bird M, Vlaeminck I, Creemers E, Wierda K, Corthout N, Vermeersch P, Carpentier S, Davie K, Mazzone M, Gounko N, Aerts S, Ghesquière B, Fendt S, Vanderhaeghen P. Mitochondria metabolism sets the species-specific tempo of neuronal development. Science. 2023;379(6632):eabn4705. https://doi
.org/10.1126/science.abn4705 . [PubMed: 36705539] - Jacob F, Pather SR, Huang WK, Zhang F, Wong SZH, Zhou H, Cubitt B, Fan W, Chen CZ, Xu M, Pradhan M, Zhang DY, Zheng W, Bang AG, Song H, Carlos de la Torre J, Ming GL. Human pluripotent stem cell-derived neural cells and brain organoids reveal SARS-CoV-2 neurotropism predominates in choroid plexus epithelium. Cell Stem Cell. 2020;27(6):937–950.e939. [PMC free article: PMC7505550] [PubMed: 33010822]
- Jalili-Firoozinezhad S, Gazzaniga FS, Calamari EL, Camacho DM, Fadel CW, Bein A, Swenor B, Nestor B, Cronce MJ, Tovaglieri A, Levy O, Gregory KE, Breault DT, Cabral JMS, Kasper DL, Novak R, Ingber DE. A complex human gut microbiome cultured in an anaerobic intestine-on-a-chip. Nature Biomedical Engineering. 2019;3(7):520–531. [PMC free article: PMC6658209] [PubMed: 31086325]
- Jalink P, Caiazzo M. Brain organoids: Filling the need for a human model of neurological disorder. Biology. 2021;10(8) [PMC free article: PMC8389592] [PubMed: 34439972]
- Janardhanan, Jang KJ, Joshipura SR, Kadam MM, Kanellias M, Kujala VJ, Kulkarni G, Le CY, Lucchesi C, Manatakis DV, Maniar KK, Quinn ME, Ravan JS, Rizos AC, Sauld JFK, Sliz JD, Tien-Street W, Trinidad DR, Velez J, Wendell M, Irrechukwu O, Mahalingaiah PK, Ingber DE, Scannell JW, Levner D. Performance assessment and economic analysis of a human liver-chip for predictive toxicology. Communications Medicine. 2022;2(1):154. [PMC free article: PMC9727064] [PubMed: 36473994]
- Jang KJ, Otieno MA, Ronxhi J, Lim H-K, Ewart L, Kodella KR, Petropolis DB, Kulkarni G, Rubins JE, Conegliano D, Nawroth J, Simic D, Lam W, Singer M, Barale E, Singh B, Sonee M, Streeter AJ, Man-they C, Jones B, Srivastava A, Andersson LC, Williams D, Park H, Barrile R, Sliz J, Herland A, Haney S, Karalis K, Ingber DE, Hamilton GA. Reproducing human and cross-species drug toxicities using a liver-chip. Science Translational Medicine. 2019;11(517):eaax5516. [PubMed: 31694927]
- Jayatunga MKP, Xie W, Ruder L, Schulze U, Meier C. Nature Reviews. 2022. [March 10, 2023]. AI in small-molecule drug discovery: A coming wave? https://www
.nature.com /articles/d41573-022-00025-1 . [PubMed: 35132242] - Jensen C, Teng Y. Is it time to start transitioning from 2D to 3D cell culture? Frontiers in Molecular Biosciences. 2020;7 [PMC free article: PMC7067892] [PubMed: 32211418]
- Joubert MK, Deshpande M, Yang J, Reynolds H, Bryson C, Fogg M, Baker MP, Herskovitz J, Goletz TJ, Zhou L, Moxness M, Flynn GC, Narhi LO, Jawa V. Use of in vitro assays to assess immunogenicity risk of antibody-based biotherapeutics. PLoS One. 2016;11(8):e0159328. [PMC free article: PMC4975389] [PubMed: 27494246]
- Kammers K, Taub MA, Ruczinski I, Martin J, Yanek LR, Frazee A, Gao Y, Hoyle D, Faraday N, Becker DM, Cheng L, Wang ZZ, Leek JT, Becker LC, Mathias RA. Integrity of induced pluripotent stem cell (iPSC) derived megakaryocytes as assessed by genetic and transcriptomic analysis. PLoS One. 2017;12(1):e0167794. [PMC free article: PMC5249236] [PubMed: 28107356]
- Kanchan K, Iyer K, Yanek LR, Carcamo-Orive I, Taub MA, Malley C, Baldwin K, Becker LC, Broeckel U, Cheng L, Cowan C, D’Antonio M, Frazer KA, Quertermous T, Mostoslavsky G, Murphy G, Rabinovitch M, Rader DJ, Steinberg MH, Topol E, Yang W, Knowles JW, Jaquish CE, Ruczinski I, Mathias RA. Genomic integrity of human induced pluripotent stem cells across nine studies in the NHLBI NextGen program. Stem Cell Research. 2020;46:101803. [PMC free article: PMC7575060] [PubMed: 32442913]
- Kanton S, Paşca SP. Human assembloids. Development. 2022;149(20) [PubMed: 36317797]
- Karran E, Mercken M, Strooper BD. The amyloid cascade hypothesis for Alzheimer’s disease: An appraisal for the development of therapeutics. Nature Reviews Drug Discovery. 2011;10(9):698–712. [PubMed: 21852788]
- Kasendra M, Tovaglieri A, Sontheimer-Phelps A, Jalili-Firoozinezhad S, Bein A, Chalkiadaki A, Scholl W, Zhang C, Rickner H, Richmond CA, Li H, Breault DT, Ingber DE. Development of a primary human small intestine-on-a-chip using biopsy-derived organoids. Scientific Reports. 2018;8(1):2871. [PMC free article: PMC5811607] [PubMed: 29440725]
- Kennett S. 2010. [February 27, 2023]. Assay qualification/validation—A reviewer’s expectations.https://cdn
.ymaws.com/sites/casss .siteym .com/resource/resmgr /CE_Pharm_Speaker_Slides /2010_CE_KennettSarah.pdf - Kerns SJ, Belgur C, Petropolis D, Kanellias M, Barrile R, Sam J, Weinzierl T, Fauti T, Freimoser-Grundschober A, Eckmann J, Hage C, Geiger M, Ng PR, Tien-Street W, Manatakis DV, Micallef V, Gerard R, Bscheider M, Breous-Nystrom E, Schneider A, Cabon L. Human immunocompetent Organ-on-Chip platforms allow safety profiling of tumor-targeted T-cell bispecific antibodies. eLife. 2021;10:e67106. [PMC free article: PMC8373379] [PubMed: 34378534]
- Kim HJ, Huh D, Hamilton G, Ingber DE. Human gut-on-a-chip inhabited by microbial flora that experiences intestinal peristalsis-like motions and flow. Lab on a Chip. 2012;12(12):2165–2174. [PubMed: 22434367]
- Kim J, Koo BK, Knoblich JA. Human organoids: Model systems for human biology and medicine. Nature Reviews Molecular Cell Biology. 2020;21(10):571–584. [PMC free article: PMC7339799] [PubMed: 32636524]
- Kim J, Sullivan GJ, Park IH. How well do brain organoids capture your brain? iScience. 2021;24(2):102063. [PMC free article: PMC7856464] [PubMed: 33554067]
- Kleinstreuer N, Holmes A. Harnessing the power of microphysiological systems for COVID-19 research. Drug Discovery Today. 2021;26(11):2496–2501. [PMC free article: PMC8317448] [PubMed: 34332095]
- Kujala VJ, Pasqualini FS, Goss JA, Nawroth JC, Parker KK. Laminar ventricular myocardium on a microelectrode array-based chip. Journal of Materials Chemistry B. 2016;20:3534–3543. [PubMed: 32263387]
- Lam CK, Wu JC. Clinical trial in a dish. Arteriosclerosis, Thrombosis, and Vascular Biology. 2021;41(3):1019–1031. [PubMed: 33472401]
- Lamers MM, Beumer J, van der Vaart J, Knoops K, Puschhof J, Breugem TI, Ravelli RBG, Paul van Schayck J, Mykytyn AZ, Duimel HQ, van Donselaar E, Riesebosch S, Kuijpers HJH, Schipper D, van de Wetering WJ, de Graaf M, Koopmans M, Cuppen E, Peters PJ, Haagmans BL, Clevers H. SARS-CoV-2 productively infects human gut enterocytes. Science. 2020;369(6499):50–54. [PMC free article: PMC7199907] [PubMed: 32358202]
- Lauffenburger DA. Presentation at Committee on the State of the Science and Future Needs for Nonhuman Primate Model Systems—Public Meeting, Virtual. Nov 21, 2022. Nonhuman primate model systems: State of the science and future needs.
- Leinonen H, Tanila H. Vision in laboratory rodents-tools to measure it and implications for behavioral research. Behavioural Brain Research. 2018;352:172–182. [PubMed: 28760697]
- Levy R, Paşca S. What have organoids and assembloids taught us about the pathophysiology of neuropsychiatric disorders? Biological Psychiatry. 2023;93(7):632–641. https://doi
.org/10.1016/j .biopsych.2022.11.017 . [PubMed: 36739210] - Li P, Zhang C, Perkins EJ, Gong P, Deng Y. Comparison of probabilistic boolean network and dynamic bayesian network approaches for inferring gene regulatory networks. BMC Bioinformatics. 2007;8(Suppl 7):S13. [PMC free article: PMC2099481] [PubMed: 18047712]
- Li Q, Nan K, Le Floch P, Lin Z, Sheng H, Blum TS, Liu J. Cyborg organoids: Implantation of nano-electronics via organogenesis for tissue-wide electrophysiology. Nano Letters. 2019;19(8):5781–5789. [PubMed: 31347851]
- Li Z, Su W, Zhu Y, Tao T, Li D, Peng X, Qin J. Drug absorption related nephrotoxicity assessment on an intestine-kidney chip. Biomicrofluidics. 2017;11(3):034114. [PMC free article: PMC5453794] [PubMed: 28652884]
- Liang JG, Su D, Song TZ, Zeng Y, Huang W, Wu J, Xu R, Luo P, Yang X, Zhang X, Luo S, Liang Y, Li X, Huang J, Wang Q, Huang X, Xu Q, Luo M, Huang A, Luo D, Zhao C, Yang F, Han JB, Zheng YT, Liang P. S-Trimer, a COVID-19 subunit vaccine candidate, induces protective immunity in nonhuman primates. Nature Communications. 2021;12(1):1346. [PMC free article: PMC7921634] [PubMed: 33649323]
- Lin Z, Chou WC. Machine learning and artificial intelligence in toxicological sciences. Toxciological Sciences. 2022;189(1):7–19. [PMC free article: PMC9609874] [PubMed: 35861448]
- Linker S, Narvaiza I, Hsu J, Wang M, Qiu F, Mendes A, Oefner R, Kottilil K, Sharma A, Randolph-Moore L, Mejia E, Santos R, Marchetto M, Gage F. Human-specific regulation of neural maturation identified by cross-primate transcriptomics. Current Biology. 2022;32(22):4797–4807. https://doi
.org/10.1016/j .cub.2022.09.028 . [PubMed: 36228612] - Liu G, Betts C, Cunoosamy D, Aberg P, Hornberg J, Balogh Sivars K, Cohen T. Use of precision cut lung slices as a translational model for the study of lung biology. Respiratory Research. 2019;20(1):162. [PMC free article: PMC6642541] [PubMed: 31324219]
- Low LA, Mummery C, Berridge BR, Austin CP, Tagle DA. Organs-on-chips: into the next decade. Nature reviews. Drug Discovery. 2021;20(5):345–361. [PubMed: 32913334]
- Luechtefeld T, Hartung T. Computational approaches to chemical hazard assessment. ALTEX. 2017;34(4):459–478. [PMC free article: PMC5848496] [PubMed: 29101769]
- Luechtefeld T, Rowlands C, Hartung T. Big-data and machine learning to revamp computational toxicology and its use in risk assessment. Toxicology Research. 2018;7(5):732–744. [PMC free article: PMC6116175] [PubMed: 30310652]
- Lu J, Lu D, Fu Z, Zheng M, Luo X. Machine learning-based modeling of drug toxicity. Methods in Molecular Biology. 2018;1754:247–264. [PubMed: 29536448]
- Maharao N, Antontsev V, Wright M, Varshney J. Entering the era of computationally driven drug development. Drug Metabolism Reviews. 2020;52(2):283–298. [PubMed: 32083960]
- Majorova D, Atkins E, Martineau H, Vokral I, Oosterhuis D, Olinga P, Wren B, Cuccui J, Werling D. Use of precision-cut tissue slices as a translational model to study host-pathogen interaction. Frontiers in Veterinary Science. 2021;8:686088. [PMC free article: PMC8212980] [PubMed: 34150901]
- Malik M, Yang Y, Fathi P, Mahler GJ, Esch MB. Critical considerations for the design of multi-organ microphysiological systems (MPS). Frontiers in Cell and Developmental Biology. 2021;9 [PMC free article: PMC8459628] [PubMed: 34568333]
- Mangul S, Martin LS, Hill BL, Lam AK, Distler MG, Zelikovsky A, Eskin E, Flint J. Systematic benchmarking of omics computational tools. Nature Communications. 2019;10(1):1393. [PMC free article: PMC6437167] [PubMed: 30918265]
- Marin Navarro A, Susanto E, Falk A, Wilhelm M. Modeling cancer using patient-derived induced pluripotent stem cells to understand development of childhood malignancies. Cell Death Discovery. 2018;4(1):7. [PMC free article: PMC5841293] [PubMed: 29531804]
- Marx U, Akabane T, Andersson TB, Baker E, Beilmann M, Beken S, Brendler-Schwaab S, Cirit M, David R, Dehne EM, Durieux I, Ewart L, Fitzpatrick SC, Frey O, Fuchs F, Griffith LG, Hamilton GA, Hartung T, Hoeng J, Hogberg H, Hughes DJ, Ingber DE, Iskandar A, Kanamori T, Kojima H, Kuehnl J, Leist M, Li B, Loskill P, Mendrick DL, Neumann T, Pallocca G, Rusyn I, Smirnova L, Steger-Hartmann T, Tagle DA, Tonevitsky A, Tsyb S, Trapecar M, Van de Water B, Van den Eijnden-van Raaij J, Vulto P, Watanabe K, Wolf A, Zhou X, Roth A. Biology-inspired microphysiological systems to advance patient benefit and animal welfare in drug development. ALTEX. 2020;37(3):365–394. [PMC free article: PMC7863570] [PubMed: 32113184]
- Maschmeyer I, Hasenberg T, Jaenicke A, Lindner M, Lorenz AK, Zech J, Garbe LA, Sonntag F, Hayden P, Ayehunie S, Lauster R, Marx U, Materne EM. Chip-based human liver-intestine and liver-skin co-cultures--a first step toward systemic repeated dose substance testing in vitro. European Journal of Pharmaceutics and Biopharmaceutics. 2015;95(Pt A):77–87. [PMC free article: PMC6574126] [PubMed: 25857839]
- Mathews SM. Intelligent Computing: Proceedings of the Computing Conference. Cham: Springer International Publishing; 2019. Explainable artificial intelligence applications in NLP, biomedical, and malware classification: A literature review; pp. 1269–1292.
- McAleer CW, Pointon A, Long CJ, Brighton RL, Wilkin BD, Bridges LR, Narasimhan Sriram N, Fabre K, McDougall R, Muse VP, Mettetal JT, Srivastava A, Williams D, Schnepper MT, Roles JL, Shuler ML, Hickman JJ, Ewart L. On the potential of in vitro organ-chip models to define temporal pharmacokinetic-pharmacodynamic relationships. Scientific Reports. 2019;9(1):9619. [PMC free article: PMC6610665] [PubMed: 31270362]
- Mertens J, Reid D, Lau S, Kim Y, Gage FH. Aging in a dish: iPSC-derived and directly induced neurons for studying brain aging and age-related neurodegenerative diseases. Annual Review of Genetics. 2018;52:271–293. [PMC free article: PMC6415910] [PubMed: 30208291]
- Miner JJ, Diamond MS. Zika virus pathogenesis and tissue tropism. Cell Host Microbe. 2017;21(2):134–142. [PMC free article: PMC5328190] [PubMed: 28182948]
- Monteil V, Kwon H, Prado P, Hagelkrüys A, Wimmer RA, Stahl M, Leopoldi A, Garreta E, Hurtado Del Pozo C, Prosper F, Romero JP, Wirnsberger G, Zhang H, Slutsky AS, Conder R, Montserrat N, Mirazimi A, Penninger JM. Inhibition of SARS-CoV-2 infections in engineered human tissues using clinical-grade soluble human ACE2. Cell. 2020;181(4):905–913.e907. [PMC free article: PMC7181998] [PubMed: 32333836]
- Moradi S, Mahdizadeh H, Šarić T, Kim J, Harati J, Shahsavarani H, Greber B, Moore JB. Research and therapy with induced pluripotent stem cells (iPSCs): Social, legal, and ethical considerations. Stem Cell Research & Therapy. 2019;10(1):341. [PMC free article: PMC6873767] [PubMed: 31753034]
- Musah S, Mammoto A, Ferrante TC, Jeanty SSF, Hirano-Kobayashi M, Mammoto T, Roberts K, Chung S, Novak R, Ingram M, Fatanat-Didar T, Koshy S, Weaver JC, Church GM, Ingber DE. Mature induced-pluripotent-stem-cell-derived human podocytes reconstitute kidney glomerular-capillary-wall function on a chip. Nature Biomedical Engineering. 2017;1 [PMC free article: PMC5639718] [PubMed: 29038743]
- NASEM (National Academies of Sciences, Engineering, and Medicine). Microphysiological systems: Bridging human and animal research: Proceedings of a workshop— in brief. Washington, DC: The National Academies Press; 2021. [PubMed: 34554662]
- Natarajan A, Sethumadhavan A, Krishnan UM. Toward building the neuromuscular junction: In vitro models to study synaptogenesis and neurodegeneration. ACS Omega. 2019;4(7):12969–12977. [PMC free article: PMC6682064] [PubMed: 31460423]
- NC3RS (National Center for the Replacement, Refinement, and Reduction of Animals in Research). Towards a virtual second species in toxicology for drug development. 2021. [January 30, 2023]. https://nc3rs
.org.uk /crackit/sites/innovation /files/2021-07/Virtual %20Second%20Species %20Final%20Brief%20_0.pdf . - NEI (National Eye Institute). Age-related macular degeneration (AMD). 2021. [February 21, 2023]. https://www
.nei.nih.gov /learn-abouteye-health /eye-conditions-and-diseases /age-related-macular-degeneration . - NIA (National Institute on Aging). Division of Neuroscience. Virtual workshop, July 19–20, 2022. 2022. [March 31, 2023]. Microphysiological systems to advance precision medicine for Alzheimer’ s disease (AD) and AD-related dementias (ADRD) treatment and prevention. https://www
.nia.nih.gov /sites/default/files /2022-09/nia-mps-workshop-summary.pdf . - NIH (National Institutes of Health). Bridging Interventional Development Gaps (BrIDGs). 2022. [March 23, 2023]. https://ncats
.nih.gov/bridgs . - NIH. Benchmarking. 2023. [January 12, 2023]. https://ors
.od.nih.gov /OD/OQM/benchmarking /Pages/benchmarking.aspx . - Novak R, Ingram M, Marquez S, Das D, Delahanty A, Herland A, Maoz BM, Jeanty SSF, Somayaji MR, Burt M, Calamari E, Chalkiadaki A, Cho A, Choe Y, Chou DB, Cronce M, Dauth S, Divic T, Fernandez-Alcon J, Ferrante T, Ferrier J, FitzGerald EA, Fleming R, Jalili-Firoozinezhad S, Grevesse T, Goss JA, Hamkins-Indik T, Henry O, Hinojosa C, Huffstater T, Jang KJ, Kujala V, Leng L, Mannix R, Milton Y, Nawroth J, Nestor BA, Ng CF, O’Connor B, Park TE, Sanchez H, Sliz J, Sontheimer-Phelps A, Swenor B, Thompson G 2nd, Touloumes GJ, Tranchemontagne Z, Wen N, Yadid M, Bahinski A, Hamilton GA, Levner D, Levy O, Przekwas A, Prantil-Baun R, Parker KK, Ingber DE. Robotic fluidic coupling and interrogation of multiple vascularized organ chips. Nature Biomedical Engineering. 2020;4(4):407–420. [PMC free article: PMC8057865] [PubMed: 31988458]
- Odijk M, van der Meer AD, Levner D, Kim HJ, van der Helm MW, Segerink LI, Frimat JP, Hamilton GA, Ingber DE, van den Berg A. Measuring direct current trans-epithelial electrical resistance in organ-on-a-chip microsystems. Lab on a Chip. 2015;15(3):745–752. [PubMed: 25427650]
- OECD (Organisation for Economic Co-operation and Development). Joint meeting of the Chemicals Committee and the Working Party on Chemicals, Pesticides, and Biotechnology. 2005. [February 27, 2023]. https://ntp
.niehs.nih .gov/iccvam/suppdocs /feddocs/oecd/oecd-gd34.pdf . - OECD. Guidance document on the validation of (quantitative) structure-activity relationship [(q)SAR] models. 2014. [March 31, 2023]. https://www
.oecd.org /env/guidance-document-on-the-validation-of-quantitative-structure-activity-relationshipq-sar-models-9789264085442-en .htm . - Osuna CE, Whitney JB. Nonhuman primate models of Zika virus infection, immunity, and therapeutic development. Journal of Infectious Diseases. 2017;216(suppl_10):S928–S934. [PMC free article: PMC5853426] [PubMed: 29267926]
- Outeiro TF, Heutink P, Bezard E, Cenci AM. From IPS cells to rodents and nonhuman primates: Filling gaps in modeling Parkinson’s disease. Movement Disorders. 2021;36(4):832–841. [PubMed: 33200446]
- Pang L, Sager P, Yang X, Shi H, Sannajust F, Brock M, Wu JC, Abi-Gerges N, Lyn-Cook B, Berridge BR, Stockbridge N. Workshop report: FDA workshop on improving cardiotoxicity assessment with human-relevant platforms. Circulation Research. 2019;125(9):855–867. [PMC free article: PMC6788760] [PubMed: 31600125]
- Parikh RB, Teeple S, Navathe AS. Addressing bias in artificial intelligence in health care. JAMA. 2019;322(24):2377–2378. [PubMed: 31755905]
- Parish ST, Aschner M, Casey W, Corvaro M, Embry MR, Fitzpatrick S, Kidd D, Kleinstreuer NC, Lima BS, Settivari RS, Wolf DC, Yamazaki D, Boobis A. An evaluation framework for new approach methodologies (NAMs) for human health safety assessment. Regulatory Toxicology and Pharmacology. 2020;112:104592. [PubMed: 32017962]
- Paşca SP. Assembling human brain organoids. Science. 2019;363(6423):126–127. [PubMed: 30630918]
- Paşca SP, Arlotta P, Bateup H, Camp JG, Cappello S, Gage F, Knoblich J, Kriegstein A, Lancaster M, Ming G, Muotri A, Park I, Reiner O, Song H, Studer L, Temple S, Testa G, Treutlein B, Vaccarino F. A nomenclature consensus for nervous system organoids and assembloids. Nature. 2022;609(7929):907–910. [PMC free article: PMC10571504] [PubMed: 36171373]
- Patel V, Shah M. Artificial intelligence and machine learning in drug discovery and development. Intelligent Medicine. 2022;2(3):134–140.
- Patterson EA, Whelan MP, Worth AP. The role of validation in establishing the scientific credibility of predictive toxicology approaches intended for regulatory application. Computational Toxicology. 2021;17:100144. [PMC free article: PMC7903516] [PubMed: 33681540]
- Paul D, Sanap G, Shenoy S, Kalyane D, Kalia K, Tekade RK. Artificial intelligence in drug discovery and development. Drug Discovery Today. 2021;26(1):80–93. [PMC free article: PMC7577280] [PubMed: 33099022]
- Perez-Bermejo JA, Kang S, Rockwood SJ, Simoneau CR, Joy DA, Silva AC, Ramadoss GN, Flanigan WR, Fozouni P, Li H, Chen PY, Nakamura K, Whitman JD, Hanson PJ, McManus BM, Ott M, Conklin BR, McDevitt TC. SARS-CoV-2 infection of human iPSC-derived cardiac cells reflects cytopathic features in hearts of patients with COVID-19. Science Translational Medicine. 2021;13(590) [PMC free article: PMC8128284] [PubMed: 33723017]
- Pognan F, Beilmann M, Boonen HCM, Czich A, Dear G, Hewitt P, Mow T, Oinonen T, Roth A, Steger-Hartmann T, Valentin JP, Van Goethem F, Weaver RJ, Newham P. The evolving role of investigative toxicology in the pharmaceutical industry. Nature Reviews. Drug Discovery. 2023;1-19 Advance online publication. [PMC free article: PMC9924869] [PubMed: 36781957]
- Premeaux TA, Mediouni S, Leda A, Furler RL, Valente ST, Fine HA, Nixon DF, Ndhlovu LC. Next-generation human cerebral organoids as powerful tools to advance neuroHIV research. mBio. 2021;12(4):e0068021. [PMC free article: PMC8406283] [PubMed: 34253056]
- Prior H, Sewell F, Stewart J. Overview of 3Rs opportunities in drug discovery and development using non-human primates. Drug Discovery Today: Disease Models. 2017;23:11–16.
- Qian X, Nguyen HN, Song MM, Hadiono C, Ogden SC, Hammack C, Yao B, Hamersky GR, Jacob F, Zhong C, Yoon KJ, Jeang W, Lin L, Li Y, Thakor J, Berg DA, Zhang C, Kang E, Chickering M, Nauen D, Ho CY, Wen Z, Christian KM, Shi PY, Maher BJ, Wu H, Jin P, Tang H, Song H, Ming GL. Brain-region-specific organoids using mini-bioreactors for modeling Zika exposure. Cell. 2016;165(5):1238–1254. [PMC free article: PMC4900885] [PubMed: 27118425]
- Qian X, Song H, Ming GL. Brain organoids: Advances, applications and challenges. Development. 2019;146(8) [PMC free article: PMC6503989] [PubMed: 30992274]
- Qian X, Yijing S, Adam C, Deutschmann A, Pather S, Goldberg E, Su K, Li S, Lu L, Jacob F, Nguyen P, Huh S, Hoke A, Swinford-Jackson S, Wen Z, Gu X, Pierce RC, Wu H, Briand L, Chen HI, Wolf J, Song H, Ming G. Sliced human cortical organoids for modeling distinct cortical layer formation. Cell Stem Cell. 2020;26(5):766–781. https://doi
.org10.1016/j .stem.2020.02.002 . [PMC free article: PMC7366517] [PubMed: 32142682] - Rasmussen SA, Jamieson DJ, Honein MA, Petersen LR. Zika virus and birth defects—Reviewing the evidence for causality. New England Journal of Medicine. 2016;374(20):1981–1987. [PubMed: 27074377]
- Ringman JM, Goate A, Masters CL, Cairns NJ, Danek A, Graff-Radford N, Ghetti B, Morris JC., Dominantly Inherited Alzheimer Network. Genetic heterogeneity in Alzheimer disease and implications for treatment strategies. Current Neurology and Neuroscience Reports. 2014;14(11):499. [PMC free article: PMC4162987] [PubMed: 25217249]
- Ronaldson-Bouchard K, Teles D, Yeager K, Tavakol DN, Zhao Y, Chramiec A, Tagore S, Summers M, Stylianos S, Tamargo M, Lee BM, Halligan SP, Abaci EH, Guo Z, Jacków J, Pappalardo A, Shih J, Soni RK, Sonar S, German C, Christiano AM, Califano A, Hirschi KK, Chen CS, Przekwas A, Vunjak-Novakovic G. A multi-organ chip with matured tissue niches linked by vascular flow. Nature Biomedical Engineering. 2022;6(4):351–371. [PMC free article: PMC9250010] [PubMed: 35478225]
- Ruspi ML, Chehrassan M, Faldini C, Cristofolini L. In vitro experimental studies and numerical modeling to investigate the biomechanical effects of surgical interventions on the spine. Critical Reviews in Biomedical Engineering. 2019;47(4):295–322. [PubMed: 31679261]
- Rutkai I, Mayer MG, Hellmers LM, Ning B, Huang Z, Monjure CJ, Coyne C, Silvestri R, Golden N, Hensley K, Chandler K, Lehmicke G, Bix GJ, Maness NJ, Russell-Lodrigue K, Hu TY, Roy CJ, Blair RV, Bohm R, Doyle-Meyers LA, Rappaport J, Fischer T. Neuropathology and virus in brain of SARS-CoV-2 infected non-human primates. Nature Communications. 2022;13(1):1745. [PMC free article: PMC8975902] [PubMed: 35365631]
- Samudyata A, Oliveira AO, Malwade S, Rufino de Sousa N, Goparaju SK, Gracias J, Orhan F, Steponaviciute L, Schalling M, Sheridan SD, Perlis RH, Rothfuchs AG, Sellgren CM. SARS-CoV-2 promotes microglial synapse elimination in human brain organoids. Molecular Psychiatry. 2022;27(10):3939–3950. [PMC free article: PMC9533278] [PubMed: 36198765]
- Saran C. Stanford University finds that AI is outpacing Moore’s Law. 2019. [March 28, 2023]. https://www
.computerweekly .com/news/252475371 /Stanford-University-finds-that-AI-is-outpacing-Moores-Law . - SCHEER (Scientific Committee on Health Environmental and Emerging Risks). Final opinion on the need for non-human primates in biomedical research, production and testing of products and devices. 2017. [March 31, 2023]. https://ec
.europa.eu /environment/chemicals /lab_animals/pdf/Scheer_may2017.pdf . [PubMed: 31280699] - Schepers A, Li C, Chhabra A, Seney BT, Bhatia S. Engineering a perfusable 3D human liver platform from IPS cells. Lab on a Chip. 2016;16(14):2644–2653. [PMC free article: PMC5318999] [PubMed: 27296616]
- Schuster B, Junkin M, Kashaf SS, Romero-Calvo I, Kirby K, Matthews J, Weber CR, Rzhetsky A, White KP, Tay S. Automated microfluidic platform for dynamic and combinatorial drug screening of tumor organoids. Nature Communications. 2020;11(1):5271. [PMC free article: PMC7573629] [PubMed: 33077832]
- Schutgens F, Clevers H. Human organoids: Tools for understanding biology and treating diseases. Annual Review of Pathology. 2020;15:211–234. [PubMed: 31550983]
- Shou Y, Liang F, Xu S, Li X. The application of brain organoids: From neuronal development to neurological diseases. Frontiers in Cell and Developmental Biology. 2020;8:579659. [PMC free article: PMC7642488] [PubMed: 33195219]
- Si L, Bai H, Rodas M, Cao W, Oh CY, Jiang A, Moller R, Hoagland D, Oishi K, Horiuchi S, Uhl S, Blanco-Melo D, Albrecht RA, Liu WC, Jordan T, Nilsson-Payant BE, Golynker I, Frere J, Logue J, Haupt R, McGrath M, Weston S, Zhang T, Plebani R, Soong M, Nurani A, Kim SM, Zhu DY, Benam KH, Goyal G, Gilpin SE, Prantil-Baun R, Gygi SP, Powers RK, Carlson KE, Frieman M, tenOever BR, Ingber DE. A human-airway-on-a-chip for the rapid identification of candidate antiviral therapeutics and prophylactics. Nature Biomedical Engineering. 2021;5(8):815–829. [PMC free article: PMC8387338] [PubMed: 33941899]
- Singh AP, Shah DK. Application of a PK-PD modeling and simulation-based strategy for clinical translation of antibody-drug conjugates: A case study with trastuzumab emtansine (T-DM1). American Association of Pharmaceutical Scientists Journal. 2017;19(4):1054–1070. [PMC free article: PMC5849468] [PubMed: 28374319]
- Singh R, Cuzzani O, Binette F, Sternberg H, West MD, Nasonkin IO. Pluripotent stem cells for retinal tissue engineering: Current status and future prospects. Stem Cell Reviews and Reports. 2018;14(4):463–483. [PMC free article: PMC6013538] [PubMed: 29675776]
- Smirnova L, Caffo BS, Gracias DH, Huang Q, Morales Pantoja IE, Tang B, Zack DJ, Berlinicke CA, Boyd JL, Harris TD, Johnson EC, Kagan BJ, Kahn J, Muotri AR, Paulhamus BL, Schwamborn JC, Plotkin J, Szalay AS, Vogelstein JT, Worley PF, Hartung T. Organoid intelligence (OI): The new frontier in biocomputing and intelligence-in-a-dish. Frontiers in Science. 2023;1:1017235.
- Son MY, Kim YD, Seol B, Lee MO, Na HJ, Yoo B, Chang JS, Cho YS. Biomarker discovery by modeling Behçet’s disease with patient-specific human induced pluripotent stem cells. Stem Cells and Development. 2017;26(2):133–145. [PubMed: 27733093]
- Song Y, Fazleabas A. Endometrial organoids: A rising star for research on endometrial development an associated diseases. Reproductive Sciences (Thousand Oaks, Calif.). 2021;28:12. [PubMed: 33533008]
- Sprous DG, Palmer RK, Swanson JT, Lawless M. QSAR in the pharmaceutical research setting: QSAR models for broad, large problems. Current Topics in Medicinal Chemistry. 2010;10(6):619–637. [PubMed: 20337590]
- Steger-Hartmann T, Raschke M. Translating in vitro to in vivo and animal to human. Current Opinion in Toxicology. 2020;23-24:6–10.
- Steger-Hartmann T, Kreuchwig A, Vaas L, Wichard J, Bringezu F, Amberg A, Muster W, Pognan F, Barber C. Introducing the concept of virtual control groups into preclinical toxicology testing. ALTEX. 2020;37(3):343–349. [PubMed: 32242633]
- Strassnig M, Ganguli M. About a peculiar disease of the cerebral cortex: Alzheimer’s original case revisited. Psychiatry (Edgmont). 2005;2(9):30–33. [PMC free article: PMC2993534] [PubMed: 21120106]
- Sun N, Meng X, Liu Y, Song D, Jiang C, Cai J. Applications of brain organoids in neurodevelopment and neurological diseases. Journal of Biomedical Science. 2021;28(1):30. [PMC free article: PMC8063318] [PubMed: 33888112]
- Sun XY, Ju XC, Li Y, Zeng PM, Wu J, Zhou YY, Shen LB, Dong J, Chen YJ, Luo ZG. Generation of vascularized brain organoids to study neurovascular interactions. eLife. 2022;11 [PMC free article: PMC9246368] [PubMed: 35506651]
- Sung JH, Wang YI, Narasimhan Sriram N, Jackson M, Long C, Hickman JJ, Shuler ML. Recent advances in body-on-a-chip systems. Analytical Chemistry. 2019;91(1):330–351. [PMC free article: PMC6687466] [PubMed: 30472828]
- Sung JH, Kam C, Shuler ML. A microfluidic device for a pharmacokinetic–pharmacodynamic (PK–PD) model on a chip. Lab on a Chip. 2010;10(4):446–455. [PubMed: 20126684]
- Tadenev ALD, Burgess RW. Model validity for preclinical studies in precision medicine: Precisely how precise do we need to be? Mammalian Genome. 2019;30(5):111–122. [PMC free article: PMC6606658] [PubMed: 30953144]
- Tang H, Hammack C, Ogden SC, Wen Z, Qian X, Li Y, Yao B, Shin J, Zhang F, Lee EM, Christian KM, Didier RA, Jin P, Song H, Ming GL. Zika virus infects human cortical neural progenitors and attenuates their growth. Cell Stem Cell. 2016;18(5):587–590. [PMC free article: PMC5299540] [PubMed: 26952870]
- Trairatphisan P, Mizera A, Pang J, Tantar AA, Schneider J, Sauter T. Recent development and biomedical applications of probabilistic boolean networks. Cell Communication and Signaling. 2013;11(1):46. [PMC free article: PMC3726340] [PubMed: 23815817]
- Trapecar M, Wogram E, Svoboda D, Communal C, Omer A, Lungjangwa T, Sphabmixay P, Velazquez J, Schneider K, Wright CW, Mildrum S, Hendricks A, Levine S, Muffat J, Lee MJ, Lauffenburger DA, Trumper D, Jaenisch R, Griffith LG. Human physiomimetic model integrating microphysiological systems of the gut, liver, and brain for studies of neurodegenerative diseases. Science Advances. 2021;7(5):eabd1707. [PMC free article: PMC7846169] [PubMed: 33514545]
- Tropsha A. Best practices for QSAR model development, validation, and exploitation. Molecular Informatics. 2010;29(6-7):476–488. [PubMed: 27463326]
- Trujillo CA, Muotri AR. Brain organoids and the study of neurodevelopment. Trends in Molecular Medicine. 2018;24(12):982–990. [PMC free article: PMC6289846] [PubMed: 30377071]
- Tsamandouras N, Chen WLK, Edington CD, Stokes CL, Griffith LG, Cirit M. Integrated gut and liver microphysiological systems for quantitative in vitro pharmacokinetic studies. The AAPS Journal. 2017;19(5):1499–1512. [PMC free article: PMC6540747] [PubMed: 28752430]
- van der Helm MW, Henry OYF, Bein A, Hamkins-Indik T, Cronce MJ, Leineweber WD, Odijk M, van der Meer AD, Eijkel JCT, Ingber DE, van den Berg A, Segerink LI. Non-invasive sensing of transepithelial barrier function and tissue differentiation in organs-on-chips using impedance spectroscopy. Lab on a Chip. 2019;19(3):452–463. [PubMed: 30632575]
- van der Zalm AJ, Barroso J, Browne P, Casey W, Gordon J, Henry TR, Kleinstreuer NC, Lowit AB, Perron M, Clippinger AJ. A framework for establishing scientific confidence in new approach methodologies. Archives of Toxicology. 2022;96(11):2865–2879. [PMC free article: PMC9525335] [PubMed: 35987941]
- Veening-Griffioen DH, Ferreira GS, van Meer PJK, Boon WPC, Gispen-de Wied CC, Moors EHM, Schellekens H. Are some animal models more equal than others? A case study on the translational value of animal models of efficacy for Alzheimer’s disease. European Journal of Pharmacology. 2019;859:172524. [PubMed: 31291566]
- Veninga V, Voest EE. Tumor organoids: Opportunities and challenges to guide precision medicine. Cancer Cell. 2021;39(9):1190–1201. [PubMed: 34416168]
- Vernetti L, Gough A, Baetz N, Blutt S, Broughman JR, Brown JA, Foulke-Abel J, Hasan N, In J, Kelly E, Kovbasnjuk O, Repper J, Senutovitch N, Stabb J, Yeung C, Zachos NC, Donowitz M, Estes M, Himmelfarb J, Truskey G, Wikswo JP, Taylor DL. Functional coupling of human microphysiology systems: Intestine, liver, kidney proximal tubule, blood-brain barrier and skeletal muscle. Scientific Reports. 2017;7(1):42296. [PMC free article: PMC5296733] [PubMed: 28176881]
- Vessillier S, Fort M, O’Donnell L, Hinton H, Nadwodny K, Piccotti J, Rigsby P, Staflin K, Stebbings R, Mekala D, Willingham A, Wolf B. Development of the first reference antibody panel for qualification and validation of cytokine release assay platforms—Report of an international collaborative study. Cytokine: X. 2020;2(4):100042. [PMC free article: PMC7789043] [PubMed: 33458650]
- Vila OF, Qu Y, Vunjak-Novakovic G. In vitro models of neuromuscular junctions and their potential for novel drug discovery and development. Expert Opinion on Drug Discovery. 2020;15(3):307–317. [PMC free article: PMC7044056] [PubMed: 31846349]
- Vo AH, Van Vleet TR, Gupta RR, Liguori MJ, Rao MS. An overview of machine learning and big data for drug toxicity evaluation. Chemical Research in Toxicology. 2020;33(1):20–37. [PubMed: 31625725]
- Wagner I, Materne EM, Brincker S, Süssbier U, Frädrich C, Busek M, Sonntag F, Sakharov DA, Trushkin EV, Tonevitsky AG, Lauster R, Marx U. A dynamic multi-organ-chip for long-term cultivation and substance testing proven by 3D human liver and skin tissue co-culture. Lab on a Chip. 2013;13(18):3538–3547. [PubMed: 23648632]
- Walden DM, Bundey Y, Jagarapu A, Antontsev V, Chakravarty K, Varshney J. Molecular simulation and statistical learning methods toward predicting drug-polymer amorphous solid dispersion miscibility, stability, and formulation design. Molecules. 2021;26(1) [PMC free article: PMC7794704] [PubMed: 33401494]
- Wang C, Zhang M, Garcia G Jr., Tian E, Cui Q, Chen X, Sun G, Wang J, Arumugaswami V, Shi Y. ApoE-isoform-dependent SARS-CoV-2 neurotropism and cellular response. Cell Stem Cell. 2021;28(2):331–342.e335. [PMC free article: PMC7832490] [PubMed: 33450186]
- Wang H. Modeling neurological diseases with human brain organoids. Frontiers in Synaptic Neuroscience. 2018;10:15. [PMC free article: PMC6002496] [PubMed: 29937727]
- Wang Y, Wang H, Deng P, Tao T, Liu H, Wu S, Chen W, Qin J. Modeling human nonalcoholic fatty liver disease (NAFLD) with an organoids-on-a-chip system. ACS Biomaterials Science & Engineering. 2020;6(10):5734–5743. [PubMed: 33320545]
- Weber EJ, Chapron A, Chapron BD, Voellinger JL, Lidberg KA, Yeung CK, Wang Z, Yamaura Y, Hailey DW, Neumann T, Shen DD, Thummel KE, Muczynski KA, Himmelfarb J, Kelly EJ. Development of a microphysiological model of human kidney proximal tubule function. Kidney International. 2016;90(3):627–637. [PMC free article: PMC4987715] [PubMed: 27521113]
- Weber LM, Saelens W, Cannoodt R, Soneson C, Hapfelmeier A, Gardner PP, Boulesteix AL, Saeys Y, Robinson MD. Essential guidelines for computational method benchmarking. Genome Biology. 2019;20(1):125. [PMC free article: PMC6584985] [PubMed: 31221194]
- Wen Z, Nguyen HN, Guo Z, Lalli MA, Wang X, Su Y, Kim NS, Yoon KJ, Shin J, Zhang C, Makri G, Nauen D, Yu H, Guzman E, Chiang CH, Yoritomo N, Kaibuchi K, Zou J, Christian KM, Cheng L, Ross CA, Margolis RL, Chen G, Kosik KS, Song H, Ming GL. Synaptic dysregulation in a human IPS cell model of mental disorders. Nature. 2014;515(7527):414–418. [PMC free article: PMC4501856] [PubMed: 25132547]
- Wolfe MS. The role of tau in neurodegenerative diseases and its potential as a therapeutic target. Scientifica (Cairo). 2012;2012:796024. [PMC free article: PMC3820460] [PubMed: 24278740]
- Workman MJ, Gleeson JP, Troisi EJ, Estrada HQ, Kerns SJ, Hinojosa CD, Hamilton GA, Targan SR, Svendsen CN, Barrett RJ. Enhanced utilization of induced pluripotent stem cell-derived human intestinal organoids using microengineered chips. Cellular and Molecular Gastroenterology and Hepatology. 2018;5(4):669–677.e662. [PMC free article: PMC6009013] [PubMed: 29930984]
- Wright PSR, Smith GF, Briggs KA, Thomas R, Maglennon G, Mikulskis P, Chapman M, Greene N, Phillips BU, Bender A. Retrospective analysis of the potential use of virtual control groups in preclinical toxicity assessment using the eTOX database. Regulatory Toxicology and Pharmacology. 2023;138:105309. [PubMed: 36481280]
- Wu L, Yan B, Han J, Li R, Xiao J, He S, Bo X. Toxric: A comprehensive database of toxicological data and benchmarks. Nucleic Acids Research. 2023;51(D1):D1432–d1445. [PMC free article: PMC9825425] [PubMed: 36400569]
- Xiao S, Coppeta JR, Rogers HB, Isenberg BC, Zhu J, Olalekan SA, McKinnon KE, Dokic D, Rashedi AS, Haisenleder DJ, Malpani SS, Arnold-Murray CA, Chen K, Jiang M, Bai L, Nguyen CT, Zhang J, Laronda MM, Hope TJ, Maniar KP, Pavone ME, Avram MJ, Sefton EC, Getsios S, Burdette JE, Kim JJ, Borenstein JT, Woodruff TK. A microfluidic culture model of the human reproductive tract and 28-day menstrual cycle. Nature Communciations. 2017;8:14584. [PMC free article: PMC5379057] [PubMed: 28350383]
- Xu M, Lee EM, Wen Z, Cheng Y, Huang WK, Qian X, Tcw J, Kouznetsova J, Ogden SC, Hammack C, Jacob F, Nguyen HN, Itkin M, Hanna C, Shinn P, Allen C, Michael SG, Simeonov A, Huang W, Christian KM, Goate A, Brennand KJ, Huang R, Xia M, Ming GL, Zheng W, Song H, Tang H. Identification of small-molecule inhibitors of Zika virus infection and induced neural cell death via a drug repurposing screen. Nature Medicine. 2016;22(10):1101–1107. [PMC free article: PMC5386783] [PubMed: 27571349]
- Yamamoto K, Yamaoka N, Imaizumi Y, Nagashima T, Furutani T, Ito T, Okada Y, Honda H, Shimizu K. Development of a human neuromuscular tissue-on-a-chip model on a 24-well-plate-format compartmentalized microfluidic device. Lab on a Chip. 2021;21(10):1897–1907. [PubMed: 34008665]
- Yamanaka S. Induced pluripotent stem cells: Past, present, and future. Cell Stem Cell. 2012;10(6):678–684. [PubMed: 22704507]
- Yin F, Zhang X, Wang L, Wang Y, Zhu Y, Li Z, Tao T, Chen W, Yu H, Qin J. HiPSC-derived multiorganoids-on-chip system for safety assessment of antidepressant drugs. Lab on a Chip. 2021;21(3):571–581. [PubMed: 33319899]
- Yoon BJ. Hidden markov models and their applications in biological sequence analysis. Current Genomics. 2009;10(6):402–415. [PMC free article: PMC2766791] [PubMed: 20190955]
- Youk J, Kim T, Evans KV, Jeong YI, Hur Y, Hong SP, Kim JH, Yi K, Kim SY, Na KJ, Bleazard T, Kim HM, Fellows M, Mahbubani KT, Saeb-Parsy K, Kim SY, Kim YT, Koh GY, Choi BS, Ju YS, Lee JH. Three-dimensional human alveolar stem cell culture models reveal infection response to SARS-CoV-2. Cell Stem Cell. 2020;27(6):905–919.e910. [PMC free article: PMC7577700] [PubMed: 33142113]
- Yu J. Vascularized organoids: A more complete model. International Journal of Stem Cells. 2021;14(2):127–137. [PMC free article: PMC8138664] [PubMed: 33377457]
- Zhang Z, Wang X, Park S, Song H, Ming G. Development and application of brain region–specific organoids for investigating psychiatric disorders. Biological Psychiatry. 2023;93(7):594–605. https://doi
.org/10.1016/j .biopsych.2022.12.015 . [PMC free article: PMC9998354] [PubMed: 36759261]
Footnotes
- 1
- 2
Of note, not all in vitro cytokine release assays involve the culturing of human cells.
- 3
One of the technological advances driving the development of MPS platforms, particularly for drug-testing applications, is the effort to use inert plastic- or glass-based materials for fabricating chips. Materials that have traditionally been used for microfluidic devices (such as polydimethylsiloxane [PDMS]) suffer from drug and drug metabolite losses due to adsorption to device surfaces and absorption into the device material (Berthier et al., 2012; Hargrove-Grimes et al., 2022).
- 4
While some may refer to tissue slices as ex vivo models, for the purposes of this report, tissue slices that are cultured are classified as in vitro systems.
- 5
A recent and detailed source for the views of the FDA on alternative methods can be found here: https://www
.fda.gov/science-research /about-science-research-fda /advancing-alternative-methods-fda (accessed January 16, 2023). A current list of FDA guidance that includes use of alternative methods can be found here: https://www .fda.gov/science-research /about-science-research-fda /regulatory-guidances-list-alternative-methods (accessed January 16, 2023). - 6
P.L. 114–255.
- 7
FDA Modernization Act 2.0, 117th Congress (December 29, 2022).
- A PATHWAY FOR ADOPTION OF NEW APPROACH METHODOLOGIES
- NEW APPROACH METHODOLOGIES WITH THE POTENTIAL TO COMPLEMENT OR REDUCE RELIANCE ON NHP MODELS IN BIOMEDICAL RESEARCH
- REGULATORY GUIDANCE ON TECHNOLOGIES AND APPROACHES TO COMPLEMENT OR REDUCE RELIANCE ON NHP MODELS
- NEED FOR COLLABORATION BETWEEN NHP RESEARCHERS AND THOSE DEVELOPING AND USING NEW APPROACH METHODOLOGIES
- CONCLUSIONS
- REFERENCES
- The Landscape of New Approach Methodologies - Nonhuman Primate Models in Biomedi...The Landscape of New Approach Methodologies - Nonhuman Primate Models in Biomedical Research
- Preface - Nonhuman Primate Models in Biomedical ResearchPreface - Nonhuman Primate Models in Biomedical Research
- Mord2 modifier of retinal degeneration 2 [Mus musculus]Mord2 modifier of retinal degeneration 2 [Mus musculus]Gene ID:100035175Gene
- Mord2 AND (alive[prop]) (1)Gene
- CELF5 CUGBP Elav-like family member 5 [Homo sapiens]CELF5 CUGBP Elav-like family member 5 [Homo sapiens]Gene ID:60680Gene
Your browsing activity is empty.
Activity recording is turned off.
See more...