NCBI Bookshelf. A service of the National Library of Medicine, National Institutes of Health.
National Academies of Sciences, Engineering, and Medicine; Health and Medicine Division; Board on Global Health; Forum on Microbial Threats; Nicholson A, Pavlin J, Buckley G, et al., editors. Exploring the Frontiers of Innovation to Tackle Microbial Threats: Proceedings of a Workshop. Washington (DC): National Academies Press (US); 2020 May 26.
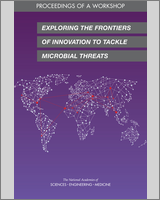
Exploring the Frontiers of Innovation to Tackle Microbial Threats: Proceedings of a Workshop.
Show detailsThe first session of the workshop aimed to harness lessons from emerging scientific, technological, and social innovations. The session’s objective was to present case studies on the lessons learned from approaches that have enabled successful innovations for predictive modeling, big data, mobile health, and diagnostic and detection tools. The session was moderated by Greg Armstrong, director, Office of Advanced Molecular Detection, U.S. Centers for Disease Control and Prevention (CDC). Audrey Lenhart, lead, Insecticide Resistance and Vector Control Team, Center for Global Health/Division of Parasitic Diseases and Malaria Entomology Branch, CDC, explored the role of innovation in the evolution of global vector control response using the example of new vector control tools for malaria.
Caroline Buckee, associate director and associate professor, Center for Communicable Disease Dynamics, Department of Epidemiology at the Harvard T.H. Chan School of Public Health, presented on how new modeling approaches can be applied to inform infectious disease surveillance and outbreak response. Senjuti Saha, scientist, Child Health Research Foundation (CHRF), described the development of laboratory capacity for metagenomics sequencing to counter microbial threats in Bangladesh to explore the challenges and opportunities of developing this type of capacity in a lower-resource setting. Nitika Pant Pai, associate professor in the Department of Medicine, McGill University, examined the effect of digital process innovations for HIV self-testing on community-level health outcomes using evidence from Canada and South Africa.
THE ROLE OF INNOVATION IN THE EVOLUTION OF GLOBAL VECTOR CONTROL RESPONSE
Audrey Lenhart focused on malaria and the Aedes-borne arboviruses to explore the role of innovation in the evolution of global vector control response. These viruses represent the greatest public health burden globally among vector-borne diseases (WHO, 2017a). Malaria is a disease for which there is not currently a licensed vaccine, although chemoprophylaxis is available. As a result, efforts to prevent and control malaria rely heavily on control of the anopheles mosquito vectors. Traditionally, this has been accomplished through the use of insecticide-treated bed nets and the residual spraying of insecticides in houses. She explained that for the Aedes-born arboviruses—primarily dengue, Zika, and chikungunya—no widely licensed vaccines or chemotherapeutics are currently available. For these diseases, vector control is the only tool against both the adult mosquitoes and the aquatic stages of the mosquitoes. For both Aedes and Anopheles mosquito control, most traditional tools have the common denominator of heavy reliance on chemical insecticides. A consequence of this practice has been the widespread emergence of insecticide resistance in these mosquitoes.
Current State of Global Malaria Control Efforts
Insecticide resistance is a major threat to successful control of malaria and other Aedes- and Anopheles-borne diseases, said Lenhart. Despite the progress in malaria control seen in the early 2000s with the advent of large campaigns supported by The Global Fund to Fight AIDS, Tuberculosis and Malaria (The Global Fund) and the President’s Malaria Initiative, progress has stagnated and stalled since 2015 (WHO, 2018a). Concurrently, the prevalence of insecticide resistance has increased in the vectors that transmit malaria. The global expansion of Aedes-borne arboviruses has driven an increase in vector control, with more insecticides being used to try to quell the outbreaks, which is also contributing to the emerging increase in the prevalence of insecticide resistance in these mosquitoes (Dusfour et al., 2019).
Lenhart explained that the World Health Organization (WHO) developed its global vector control response against this backdrop of stalled malaria control progress, continued reliance on insecticide-based interventions, and upward trends in insecticide resistance. Released in 2017, this strategic framework was designed to guide countries in how to address the growing threat of vector-borne diseases through 2030 (WHO, 2017a). She highlighted the framework’s foundation, which calls for increases in basic and applied research, as well as innovations, to enable success in the four pillars of action (see Figure 3-1).

FIGURE 3-1
WHO’s global vector control response framework. SOURCES: Lenhart presentation, December 4, 2019; WHO, 2017a, 2019e.
Importance of Evidence-Based Innovations to Eliminate Malaria
Lenhart emphasized that because current vector control tools are insufficient, innovation is essential if malaria is to be eliminated. A modeling study summarized the fractions in decreases in childhood malaria attributable to insecticide-treated bed nets, indoor residual spraying of insecticides, and combination therapies; they found that though progress has been made, the impacts of these interventions are still below the elimination target (Bhatt et al., 2015). In addition to growing insecticide resistance, major gaps also exist around tools used to control outdoor-biting and day-biting vectors (Aedes), for example. She noted that innovations with the potential to address these gaps are currently under way, including new and repurposed insecticides with novel modes of action to which the mosquitoes are not yet resistant, noninsecticide-based strategies, and genetic modification.
An evidence base needs to be established for an innovation’s effect, said Lenhart, because most large donor organizations will only procure vector control products that have a WHO recommendation. However, for WHO to make a policy recommendation on a vector control tool, it must demonstrate public health impact. WHO’s Vector Control Advisory Group reviews evidence arising from trials with epidemiological endpoints in order to assess the public health impact of these vector control tools, she added. This process runs in parallel with the WHO prequalification process for vector control products, which assesses product efficacy, safety, and quality. The processes of bringing a new innovation into widespread use in donor-funded malaria control programs is challenging because it is lengthy and expensive, she added.
Lenhart described the New Nets Project as a case example of innovation in malaria control. The program is led by the Innovative Vector Control Consortium in partnership with The Global Fund and Unitaid. She described the Innovative Vector Control Consortium as an exemplar of innovation in the vector space because it was specifically created to foster innovation by convening partners from industry, the public sector, and academia in order to spur innovation for vector-borne disease tools. More information about the New Nets Project is provided in Box 3-1.
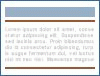
BOX 3-1
Case Example of Innovation in Vector Control: New Nets Project.
Many exciting new tools for vector control are in the pipeline, said Lenhart. She pointed out that controlling mosquitoes is not the sole concern: there must also be an impact on public health. Clinical trials have not traditionally been conducted to evaluate vector control tools. As a result, funding is scarce for generating the evidence base required to show the effect on public health. Procurement of innovative vector control tools is highly donor dependent, so the commercial market for these innovations is limited. This gives rise to the challenge of maintaining industry engagement in continued research and development of these tools. She added that the need for innovation will not dissipate in the future, because as mosquitoes continue to evolve so must the tools to control them.
APPLYING MODELING TO INFORM INFECTIOUS DISEASE SURVEILLANCE AND OUTBREAK RESPONSE
Caroline Buckee discussed the new sources of data being used to model disease outbreaks and the value of new, more complex mapping techniques that use these data in predictive models. She gave several examples of how these techniques and data have been used to model outbreaks in novel ways and direct the flow of resources, then described some of the barriers to broad implementation of these techniques and data. She explained that a new trend emerging in mathematical modeling for infectious diseases is the use of highly complex simulation models that appear to be detailed, accurate, and fit the data well. However, these models should not be embraced unless the data underlying them are accurate. She emphasized that a model is only as good as the data that underlie it.
Risk Mapping and Sources of Data
Buckee and her colleagues are working on the application of risk maps to improve the targeting of resources for infectious disease control. She explained that these risk maps are developed based on the incidence reports of clinical cases from hospitals and clinics around the country, and are used to allocate resources, which is especially important if resources are scarce. One challenge in using risk maps for resource allocation is the global increase in travel, including international travel; internal migration; and large-scale population displacement following natural disasters or conflicts. For example, if the size of a catchment area is assumed based solely on clinic reporting, then it is probably much larger than assumed. Another challenge Buckee noted is that long-term imported infections may appear to be happening in a specific place, but they will actually have a different point of origin. In the context of mathematical modeling, these dynamics need to be understood in order to help infectious disease control programs make decisions based on estimates of the speed at which a disease may spread out of a population at risk and the risk of importation to a population from an endemic area.
Buckee explained that from a modeling standpoint, epidemic numbers—such as the number of cases of Ebola in Sierra Leone—are plotted on a curve, which allows a simple mathematical model to be fit to the epidemic. This is done instead of using a statistical model because of the nonlinear dynamics that drive these types of outbreaks. Understanding that mechanism is necessary for interpreting the model parameter estimates, which are related to the epidemiological factors that underlie the spread, said Buckee. This elucidates the threshold conditions for reducing transmission and allows for evaluating the effectiveness of different interventions.
Most frameworks involve one population in an outbreak, but Buckee is interested in looking at spatial dynamics. Until recently, most models used some version of a gravity model for this purpose. Gravity models are developed from transportation theory and hold that large populations attract in a way that is inversely related to distance; that is, cities are attractors and places that are further away from cities have less travel (Haynes and Fotheringham, 1984). Although these models are analytically tractable, they are not validated, they do not have asymmetries in them, and they do not vary over time, Buckee noted.
New Sources of Data to Understand Real-Time Spatial Dynamics
An emerging approach is to work with new data sources to develop a better understanding of spatial dynamics in real time, said Buckee. These data sources can be used within epidemiological models to better understand the flow of disease over space and time. She noted that owing to the ubiquity of mobile phones, they can serve as a useful source of this information. Mobile operators already collect these data routinely, providing scalable information about the location and travel patterns of millions of people in nearly real time. To illustrate, she shared a data visualization demonstration that was developed in collaboration with Telenor, a Norwegian mobile operator, and their business unit in Bangladesh. The visualization tracked the population-wide travel from Dhaka, the capital of Bangladesh, to the countryside and back to Dhaka for the Eid holiday. It provides detailed information about the weekly dynamics of people’s movements during that period. The use of these data raises concerns about privacy and data aggregation, requiring negotiation with national regulatory authorities to ensure that the data are secure. Efforts are under way to standardize these methods, she noted.
Other sources of data can be used to generate useful information about the spread of disease, said Buckee. For instance, Facebook, Google, and other companies are interested in developing their own methods of mapping population displacement and density to release routinely as well as during outbreaks and disasters. She suggested that these organizations should be engaged in a public forum around how privacy is handled in using these data, especially in the context of public health, because public perception will influence the effectiveness and impact of these approaches.
Combining Data Layers to Effectively Generate Useful Information
Modelers are working to bring together different types of data to target resources effectively to stop transmission of disease, Buckee said. Traditional forms of epidemiological data on prevalence and incidence can be complemented by satellite information about vectorial capacity or the distribution in a village, for example, and mobile phone data and other kinds of information can provide more up-to-date population density estimates. Modelers need these data to determine the denominators for the population at risk, Buckee continued. Information about migration rates can be used to assess travel between regions and to inform estimates of importation and exportation in the spread of disease between regions. In the past, modelers were reliant on travel survey data, but they can now draw on other sources of information, such as mobile phone data and biological samples with genomic sequencing. Each of the data sources used in models has biases and problems associated with it, she explained. Therefore, the role of modelers is to bring these data together to make sense of the epidemiological situation.
She highlighted similarities between the emergence and the elimination of outbreaks, as shown in Figure 3-2. Both involve spatial heterogeneity. In emergence, researchers are seeking the early first cases of an outbreak; toward elimination, researchers are seeking the last cases of an outbreak. Some methods for these two efforts can translate between the two, although outbreak emergence calls for simpler modeling and faster communication.

FIGURE 3-2
Optimal use of new approaches depends on epidemiological context. NOTE: CDR = call data record. SOURCES: Buckee presentation, December 4, 2019; Buckee et al., 2018.
Case Examples of Combination Modeling
Buckee presented examples of modeling work in which researchers used a combination of models and various sources of data to generate concrete outcomes. In Pakistan, researchers sought to move beyond a risk map for dengue fever, which shows the vectorial potential for transmission, and toward a targeted risk map that could predict whether Lahore will be at risk of dengue in 1 month, for example (Wesolowski et al., 2015). In Bangladesh, researchers combined models and data sources, including mobile phone and genetic data, to produce a map that estimated the fraction of cases in a particular region that were thought to be imported (Chang et al., 2019). Another project in which Buckee was involved worked with people on the ground in Mozambique just prior to Hurricane Kenneth. In response to concern about the spread of cholera, the project team conducted a simple modeling exercise to rapidly predict the areas at highest risk for cholera outbreak (Kahn et al., 2019). This exercise demonstrated the importance of strong organizational structure in achieving implementation, she said. They had a multisectoral, diverse, and flexible team that spanned the public sector, nongovernmental organizations, and academia; they were in continuous communication about what they needed to know and what data they had available.
Barriers to Translating Modeling Innovations to the Field
Buckee explored several barriers to translating innovations in modeling to the field. The first is that academic incentives are misaligned with translational goals. The incentives to write publications and secure grants are at odds with the translational goals of local capacity building, navigating regulatory and political issues, long-term engagement with infection control programs, and continuous methodological refinement. Regulatory and political issues are also restricting the feasibility of moving toward the use of private data for modeling. Academics are not well suited for the challenging and time-consuming process of negotiating data access, breaking down barriers between public and private stakeholders, and obtaining buy-in from relevant parties on the ground. She noted that even the best modeling approach will not be used to guide decision making if there is no demand for it or if the epidemiological data are weak. The quality of a model depends on quality reporting of epidemiological surveillance and response data, which is not prioritized in many cases, and on receiving feedback about which questions are most important to address.
Finally, she said that current funding mechanisms do not allow rapid response. Epidemic response requires responsive, flexible cross-sector teams that are in constant communication, rather than centralized hubs. These teams should be able to respond quickly, have domain area expertise, and be in close contact with epidemiologists on the ground—this is not typically possible for academics working within the constraints of large federal grants, for example. She added that surveillance work, including “peace time” methodological development, should be better integrated with teams that respond to crises.
UNBIASED METAGENOMICS SEQUENCING TO COUNTER MICROBIAL THREATS: LESSONS FROM BANGLADESH
Senjuti Saha described the development of a laboratory for metagenomic sequencing to counter microbial threats in Bangladesh to demonstrate the value of building capacity on site in countries that typically outsource this type of work. She explained that CHRF’s mission is to improve the evidence needed to inform policy decisions that would improve child health in Bangladesh and around the world. To aid policy makers in making data-driven decisions, CHRF established a pathogen surveillance program in Bangladesh that is carried out in microbiology laboratories in four hospitals across the country, with support from WHO Rotavirus and Invasive Bacterial Vaccine Preventable Diseases Sentinel hospital sites.
The objectives of the pathogen surveillance program in Bangladesh are (1) to describe the etiology, the epidemiology, and the burden of diseases, including meningitis, sepsis, pneumonia, and enteric fever; (2) to establish a platform to measure vaccine needs and impact; and (3) to characterize circulating pathogen types, including antimicrobial resistance and serotypes. CHRF has maintained a focus on four priority pathogens: Neisseria meningitidis, Streptococcus pneumoniae, Haemophilus influenzae type b (Hib), and group B Streptococcus.
Collaborating to Develop Local Capacity for Metagenomic Sequencing
Saha’s presentation focused on meningitis sequencing in Dhaka Shishu Hospital, the largest pediatric hospital in the country. In 1993, CHRF began surveillance using culture to detect the etiology of meningitis in cerebrospinal fluid, gradually adding serology and antigen testing and progressing from conventional polymerase chain reaction (PCR) to quantitative PCR (qPCR) in 2015. In spite of their extensive efforts, the etiology of many meningitis cases remains unknown. Between 2003 and 2016, Saha remarked that the hospital collected 24,000 samples of cerebral spinal fluid, including 8,000 cases of suspected infectious meningitis based on ≥ 10 white blood cells per cubic millimeter, a biomarker of clinical meningitis. Even among cases where the white blood cell count was more than 500, researchers were unable to determine the etiology in more than half of those cases. This lack of evidence hampered efforts to institute data-driven policies for prevention or treatment, she noted.
The group at CHRF hypothesized that RNA metagenomics could solve the mystery, but they had no evidence to show that they could conduct even the simplest sequencing on site in the hospital’s small laboratory. Typically, this kind of research is done remotely by a country in the Global North, which does not build capacity in Bangladesh. In 2018, CHRF was able to break this cycle by developing capacity for metagenomic sequencing through a collaboration with the Chan Zuckerberg Biohub. The Biohub had expertise in solving cases of meningitis with unknown etiology, and the hospital had years of samples with case-based electronic data. To ensure that the collaboration was bilaterally beneficial, they ensured that all standard operating procedures were open access, all technologies and pipelines resulting from the collaboration were transferrable and sustainable, local scientists would be trained through the collaboration, and the local group would own the data produced through the collaboration.
Elucidating the Causative Agents of Meningitis Using Metagenomics
Saha provided an overview of the collaboration to elucidate the causative agents of meningitis using metagenomics. During the first phase, 96 samples were collected and sent to the Biohub laboratories in San Francisco: 36 positive controls that were etiology-confirmed using standard laboratory techniques in Bangladesh; 36 negative controls that were noninfectious cases of meningitis and water samples; and 25 “mystery” idiopathic cases, in which etiology could not be attributed using standard laboratory techniques. They extracted RNA from all of the samples, prepared a library, and then sequenced and analyzed the samples.
She noted that the most challenging process was analyzing the vast amount of data culled from each sample. To assist with analysis, the collaborators used the free, open source software IDSeq that has useful embedded software called “Background,” which helps to control for environmental RNA contaminants in different settings. They used the negative control samples to establish the microbial distribution that is normally present everywhere. Using that as a background, they gave every pathogen in the positive and idiopathic samples a Z score. Based on the Z score, they were able to model any pathogens that were found.
Her team found high concordance among the positive and negative controls, said Saha (Saha et al., 2019a). When pathogens were found using metagenomics and their model, there was a high likelihood that it was a true pathogen. However, pathogens that were found using qPCR with very high cycle threshold values tended to be missed by metagenomics. They did not find any significant pathogens in their negative control samples. Idiopathic samples were found to contain a diverse range of pathogens, she reported. Out of 25 idiopathic samples tested, 10 were solved by the metagenomic analysis (Saha et al., 2019a). Saha pointed out that these samples were not collected or stored with the intention of analyzing them with a sensitive method such as metagenomics. Clinical follow-up of the 10 cases that were solved by metagenomic analysis revealed three cases of chikungunya, which is a relatively new virus in Bangladesh (Saha et al., 2019a). They were aware that the virus causes fevers, but they did not realize that it was crossing the blood–brain barrier and causing chikungunya meningitis.
Further investigation revealed that these three cases originated during the first large chikungunya outbreak in Bangladesh in summer 2017. The researchers suspected that there were more cases of chikungunya that had been missed, but they could not afford to conduct unbiased metagenomics on hundreds of samples. Instead, they optimized a low-cost qPCR method to test almost 500 stored cerebral spinal fluid samples from 2017. Using this method, they discovered that 12 percent of all meningitis cases that came into the hospital that summer were positive for chikungunya. By comparing these findings with cases of chikungunya fever recorded during the same time period, Saha and her colleagues showed that there had been an undocumented chikungunya meningitis outbreak that overlapped with the chikungunya fever outbreak in summer 2017 (Saha et al., 2019a). The success of this effort allowed the researchers to secure funding for the second phase of the project, during which they transferred the technology to Bangladesh and set up a small metagenomics laboratory.
Developing Local Sequencing Capacity: Barriers and Opportunities
Saha described some of the challenges involved in setting up a sequencing platform laboratory in a low- and middle-income country (LMIC) such as Bangladesh. For instance, it is difficult to obtain supplies because distributors and manufacturers do not have local offices in Bangladesh, which requires laboratories to work with resellers or local distributors to obtain reagents. These distributors tend to charge higher prices for local laboratories, she noted. In this case, Saha’s colleagues in San Francisco were able to negotiate on behalf of the laboratory in Bangladesh to help them obtain supplies at a lower cost. However, without such intervention, small laboratories in lower-income settings lack the power or influence to negotiate for fair pricing. Laboratories in LMICs must also deal with infrastructure issues, she added. For example, Dhaka is a very hot city with poor air quality, which requires setting up equipment to control temperature, humidity, and dust content.
Saha maintained that these challenges are all surmountable with the help of global collaboration and the enthusiasm of local scientists in the laboratory to participate in this research (Saha et al., 2018, 2019b). Her team has been able to empower, educate, and engage local scientists on the front lines of public health. Furthermore, this work built the capacity in Dhaka to use metagenomics to investigate the severe outbreak of dengue fever in 2019, which affected more than 100,000 people with high rates of mortality, by sequencing cerebral spinal fluid and serum samples in real time. The lab is also using metagenomics for nationwide mosquito surveillance for dengue fever, chikungunya, and Zika in order to help predict outbreaks and outbreak severity.
Much progress is being made, Saha noted, but this is just the beginning of a long-term process that is being undertaken by CHRF. In 2018, CHRF staff performed 3,000 cultures on blood from children who were suspected of having sepsis. At that time, researchers were able to attribute etiology to only 17 percent of these samples, and all of those patients were treated with antibiotics—which probably included third-generation cephalosporins. This speaks to the magnitude of the “unknowns” that Saha and her colleagues at CHRF are working to address. She closed by encouraging a more holistic approach to outbreak investigation. Rather than using an etiology-by-etiology approach, she suggested using methods between endemics to build landscapes or maps of known pathogens in order to detect emerging and reemerging outbreaks.
DIGITAL PROCESS INNOVATIONS FOR HIV SELF-TESTING
Nitika Pant Pai presented on how digital process innovations for HIV self-testing can affect health outcomes at the community level, drawing on experiences in South Africa and Canada. She explained that HIV self-testing is a self-screening process whereby end users perform the test on their own by collecting their own blood or oral samples, interpreting and recording their test results, and proactively seeking linkages to counseling and care. The U.S. Food and Drug Administration (FDA) approved the first in-home, oral HIV self-test in 2012 (FDA, 2014). Four years later, WHO released guidelines on HIV self-testing and partner notification stating that self-testing could complement conventional testing strategies, particularly in the last mile of care (WHO, 2016). As of 2019, more than 70 countries had HIV self-testing guidelines or policies in place (WHO, 2019d). HIV self-testing has been implemented as part of the effort to end the HIV epidemic, she noted.
Overview of the HIVSmart! App
HIVSmart! is an app-based program designed with support from the McGill University Health Centre and the Government of South Africa to help people in LMICs self-test their own HIV status, said Pant Pai. The program addresses several gaps in efforts to achieve the targets set forth in 2014 by the Joint United Nations Programme on HIV/AIDS to end the HIV epidemic, which are known as the 90-90-90 targets. These targets call for 90 percent of all people living with HIV to know their HIV status by 2020, ensuring that 90 percent of those diagnosed with HIV receive treatment by 2020, and viral suppression of 90 percent of all people receiving antiretroviral therapy by 2020 (UNAIDS, 2014). Although HIV self-testing has expanded access, she noted, it is unclear how well the self-tests are being administered. Other concerns relate to interpreting and storing the test results, ensuring timely linkages from self-testing to counseling and care, and keeping patients in care (Pant Pai et al., 2013a). Pant Pai explained that HIVSmart! has evolved from an app to a complete program of care that provides an efficient method of screening, counseling, and treatment for patients and providers. The program offers the following multiple services delivered in tandem:
- Evidence-based HIV information;
- A user-friendly, language tailored, mobile app;
- Step-by-step instructions for self-test conduct;
- Pretest risk assessment and counseling;
- Rapid self-test results;
- Secure and confidential cloud storage of data;
- Geolocations of clinics for confirmatory testing;
- 24/7 counseling in multiple forms; and
- Rapid linkages to antiretroviral therapy and retention in care.
Evidence for App-Based HIV Self-Testing
Pant Pai presented some of the evidence that has accrued to support app-based HIV self-testing with HIVSmart! The program was initially a web-based HIV self-testing strategy, piloted by university students in Canada in 2009 (Pant Pai et al., 2014) and by health care workers in South Africa (Pant Pai et al., 2013b); the app-based version of HIVSmart! was initially tested in Montreal with a cross-sectional study (Pant Pai et al., 2018). Between 2016 and 2018, the app-based HIVSmart! program was evaluated among 3,000 people in South African townships using an observational cohort study design to see if it would help people actually conduct self-testing, seek linkages to care, and receive treatment (Janssen et al., 2020).
Pant Pai explained that in process innovation, cohort study designs have advantages over randomized controlled trials in that they can capture how participants receive the innovation as well as the dimension of choice and other conceptual underpinnings that determine how participants integrate the innovation into their lives. Participants in the study were given a choice between two popular self-testing strategies: (1) supervised self-testing, whereby individuals conduct the self-test in the presence of a health care professional in a clinic setting, and (2) unsupervised self-testing, whereby individuals obtain self-tests from a pharmacy and conduct the self-test at home (Janssen et al., 2020). The second strategy is more common in the United States, she noted.
The study investigated how people chose between these two strategies and how they integrated the self-tests into their lives. It also explored the effects of these strategies on linkages to care, on the detection of new infections, and on the expansion of access to care. The study provided participants with the HIVSmart! app on their phones; half of the 1,500 participants chose the supervised strategy and the others chose the unsupervised strategy, she reported. During the rollout of this program, some participants reported that they did not have enough space in their homes to safely conduct the self-testing strategy and requested that a kiosk be set up near the clinic where they could safely conduct the self-test without supervision. She noted that this is an example of how an innovation can be tailored to setting-specific needs.
Almost all of the participants returned for care; some participants returned to the clinic setting while others wanted to return to clinics outside of the setting. The geolocation feature of the HIVSmart! app allowed researchers to monitor where participants were seeking linkages to care and to connect with participants and ensure that almost all of them receive care. The intervention also expanded access to care, she added. HIV self-testing through the app became so popular among the community that they were able to discontinue the social media campaign advertising its benefits.
Challenges and Opportunities in HIV Self-Testing Strategies
Pant Pai described some of the challenges encountered in implementing HIV self-testing strategies. Connectivity issues were common, and some participants had smartphones that were not able to fully use the functionality of the HIVSmart! app. This was resolved by allowing these participants to either use the supervised strategy or to implement the unsupervised strategy in one of the offsite kiosks. It was also challenging to mobilize care seeking outside of clinics. Health Insurance Portability and Accountability Act compliance required the use of firewalls that were challenging to address. The app was also met with resistance based on skepticism of new technology. Some people rejected the app, for example, because they thought the digital innovation would be detrimental by undermining peer navigators that were already in place in the health care system. Pant Pai worked to build confidence in the system among health care providers and patients, who eventually requested that the program be rolled out community-wide.
To address other challenges at the outset, Pant Pai’s team customized the app to the context and made it culturally adaptable, so they were able to work around language issues. To address ethical considerations, the qualitative component of the cohort study explored the tension between autonomy and risk mitigation—that is, between wanting privacy and negotiating presence (Janssen et al., 2020). The participants reported that they most appreciated the ability to negotiate their space, their level of support, and the testing process itself. They also liked the fact that the process was simple and confidential and that they could self-determine where, with whom, and when to self-test. In the context of future opportunities, Pant Pai noted that the HIVSmart! strategy has been adopted by Fast-Track Cities with support from the International Association of Providers of AIDS Care. Pant Pai’s team is working to customize the strategy for use in Canada and hopes to scale up this strategy for other key populations and hotspots to help eliminate HIV.
DISCUSSION
Carolyn Carroll asked how Buckee was able to persuade Telenor to provide data, given existing privacy concerns. Buckee responded that she has worked with multiple mobile operators in this space of research for some time, and Telenor has taken the lead in the industry in terms of finding ways to use their data for public health and other socially beneficial projects. She explained that Telenor has its own research group working on how to use its data for these projects within its multilevel privacy and security process that works with business units, regulators, and governments to ensure that data stay within the operator in-country. Aggregated matrices are sent to the research group in Oslo, which then shares the data with select academic researchers.
Buckee pointed out that the data are provided at such a large scale that the information is memoryless and aggregated to many towers at once; it does not reveal information about individual trajectories, and reidentification of individuals is not possible after the aggregate data are shared. She noted that the creation of these types of aggregations for different purposes has been a matter of concern, but Telenor has been at the forefront of making sure it is done safely. Buckee confirmed that reidentification of individuals is impossible through Telenor’s processes prior to providing the data.
Eva Harris, director, Center for Global Public Health, University of California, Berkeley, asked Lenhart about community involvement in the New Nets Project. Lenhart explained that she is not part of the New Nets Project, but the communities receiving nets through the program are not doing so for the first time; they are receiving innovative replacement nets treated with dual insecticide. She added that within any of these large initiatives, there are community engagement components, particularly around the potential misuse of bed nets.
Harris asked Buckee about issues with data sharing—such as funder requirements to make the deidentified data available for public analysis—that might dissuade local stakeholders from putting in time to collect the data for other people to analyze. She also flagged the work required to harmonize and apply standards to data analysis, noting a tension between the Global North, where people may like data to be publicly available for analysis, and the people collecting the data in the Global South, where people collecting the data may want to control how they are used or analyzed. Buckee remarked that the incentive structures within academia can be pernicious in that people collecting data often fail to receive the authorship and credit they deserve, for example. She noted that there is a similar glamorization of the analytics and artificial intelligence (AI) in modeling, while the work of actually collecting highly detailed epidemiological data is less appreciated. She called for a shift in the academic world to frame global health research as unlike other branches of science. In terms of data sharing, another issue is the pressure for countries to share all of their epidemiological data. Although this is framed as being in their best interests regionally and globally, some countries may not be eager to do so.
Marcos Espinal, director, Department of Communicable Diseases and Environmental Determinants of Health, Pan American Health Organization, applauded Buckee’s call for people to be put first and accounted for by policy makers in order for modeling to be helpful. Espinal put modeling in the context of human movement and migration, which is often politicized as being damaging rather than beneficial. He noted that policy makers sometimes use modeling results for predicting an outbreak in a certain place as an excuse to deport migrants.
Espinal offered the example of Venezuelan migrants to Colombia, Ecuador, and Peru being blamed for bringing in diseases such as malaria, giving rise to serious human rights issues. Buckee added that policy makers’ responses often depend on which level of government they are in; for example, in a national malaria control program, policy makers may be less interested in blaming people and more interested in trying to control the disease. She noted that public perception of new modeling approaches at the community level, which are often shaped by Facebook and other social media platforms, can be the most hostile.
In terms of higher levels of government, Buckee said, one of the things modeling has shown is that shutting a border down to keep out migrants will not stop an epidemic. This is an outdated idea based on migration patterns from 100 years ago, Buckee said. She suggested that models and data demonstrate that the world is globally connected, but scientists have not sufficiently demonstrated the value of that connectedness. In infectious disease outbreaks, notions of national identity are blurred and inconsequential with respect to pathogen transmission. She pointed out that feedback from policy makers also depends on their level in a government. For instance, policy makers at lower levels may not wish to be perceived as tied up with corporate interests. For policy makers at higher levels of government, Buckee proposed treating epidemics as a global issue. She suggested focusing on building lab capacity in LMICs and helping governments and ministries of health build the capacity to manage epidemics.
Tom Scott, director and professor, Department of Entomology, University of California, Davis, asked about the development and application of innovation in two areas: the first phase of coming up with an idea and carrying it through to the proof of principle to build up an evidence base, and the second phase of scaling it up to delivery of appropriate coverage in order to achieve the desired public health outcome. Scott asked about the challenges and barriers encountered in each respective phase and about issues related to delivering at scale that occur despite the innovation of ideas and work to complete proof of principle.
Pant Pai responded that universities and granting agencies are supporting innovation, but the support systems needed to scale an innovation are not in place. Pant Pai said that training in science, epidemiology, and medicine equips researchers to prove that an innovation works scientifically; however, businesses use the approach of releasing innovations and allowing the market to improve on it through an iterative approach of failing and learning. She noted that scaling up requires liaising with different stakeholders who need to share the intent of ensuring that innovations reach the people in need and suggested setting up a coalition among agencies that can support academics, scientists, and innovators in scaling interventions for the greater good. Pant Pai remarked that the current academic system’s focus on publishing makes universities less likely to support innovations that are for the greater good, which will need to be addressed in order to make progress.
Saha added that the discussion around innovation and scaling interventions highlights the issue of power imbalance, because many innovations driven by donors and research agendas are meaningless if they are not implemented where they are needed most. However, better coordination will be needed to scale up innovations, she said. For example, WHO’s Invasive Bacterial Vaccine-Preventable Diseases Laboratory Network, which spans approximately 150 laboratories in more than 50 countries and operates alongside WHO networks for the flu and rotavirus, do not communicate with each another (WHO, 2017b). Saha called for these actors to coordinate with one another to scale up interventions by leveraging existing infrastructure, rather than building parallel systems.
Buckee added that there is too much emphasis on innovation and pilot projects that only last for 1 year, which is not long enough to achieve meaningful results. She suggested moving away from rewarding innovation and focusing on scaling and implementation, which is more difficult and requires creative thinking. She noted that innovation in public health requires constant investment and evaluation, while innovation in the private sector tends to take off when the market drives it. Pant Pai said focusing on implementation and scale up will require aligning funds and bringing together cross-sector expertise to scale up innovations.
Daniel Berman, lead, Longitude Prize, remarked that Pant Pai made a strong case from the user perspective that HIVSmart! was making a difference in people seeking to know their HIV status. He asked for more information about the number of patients who have undetectable levels of the virus, the percentage of patients who say that they are staying on treatment, and transmission rates. Berman also stated that the system in South Africa is stronger than in other settings, but there are still disruptions to the supply of antiretrovirals that lead to increases in transmission rates and patients getting sick again because drugs are not available. Berman asked if issues such as medicine disruptions or clinic closures could be tracked through the app to help the system to serve its patients better.
Pant Pai said that she only focused on linkage and retention, but the app could potentially be expanded to connect geolocation hotspots and use machine learning to predict outcomes. Pant Pai said that these types of innovations seem in line with the South African government’s focus on simple solutions and the use of technology. Berman added that the process of scaling up extends beyond academic institutions, and that private companies or nonprofit organizations could possibly use surveillance data that expose flaws in health systems that cannot be used by governments, owing to their potential political volatility.
- Harnessing Lessons from Emerging Scientific, Technological, and Social Innovatio...Harnessing Lessons from Emerging Scientific, Technological, and Social Innovations - Exploring the Frontiers of Innovation to Tackle Microbial Threats
Your browsing activity is empty.
Activity recording is turned off.
See more...