NCBI Bookshelf. A service of the National Library of Medicine, National Institutes of Health.
National Academies of Sciences, Engineering, and Medicine; Health and Medicine Division; Board on Health Care Services; National Cancer Policy Forum; Nass SJ, Patlak M, Zevon E, editors. Improving Cancer Diagnosis and Care: Clinical Application of Computational Methods in Precision Oncology: Proceedings of a Workshop. Washington (DC): National Academies Press (US); 2019 Jun 20.

Improving Cancer Diagnosis and Care: Clinical Application of Computational Methods in Precision Oncology: Proceedings of a Workshop.
Show detailsWORKSHOP OVERVIEW1
A hallmark of high-quality cancer care is the delivery of the right treatment to the right patient at the right time. Precision oncology therapies, which target specific genetic changes in a patient’s cancer, are changing the nature of cancer treatment by allowing clinicians to select therapies that are most likely to benefit individual patients. In current clinical practice, oncologists are increasingly formulating cancer treatment plans using results from complex laboratory and imaging tests that characterize the molecular underpinnings of an individual patient’s cancer. These molecular fingerprints can be quite complex and heterogeneous, even within a single patient. To enable these molecular tumor characterizations to effectively and safely inform cancer care, the cancer community is working to develop and validate multiparameter omics tests and imaging tests as well as software and computational methods for interpretation of the resulting datasets.
To examine opportunities to improve cancer diagnosis and care in the new precision oncology era, the National Cancer Policy Forum developed a two-workshop series. The first workshop focused on patient access to expertise and technologies in oncologic imaging and pathology and was held in February 2018.2 The second workshop, conducted in collaboration with the Board on Mathematical Sciences and Analytics, was held in October 2018 to examine the use of multidimensional data derived from patients with cancer, and the computational methods that analyze these data to inform cancer treatment decisions.
The workshop convened diverse stakeholders and experts, including clinicians, researchers and statisticians, and patient advocates, as well as representatives of health care organizations, academic medical centers, insurers, and federal agencies. The workshop included presentations and panel discussions on the current state of computational precision oncology and its opportunities, challenges, and limitations. Topics explored included
- Data quality, completeness, sharing, and privacy;
- Preclinical and clinical validation of the reliability, safety, and effectiveness of diagnostic tests and clinical decision support tools;
- Regulatory oversight and reimbursement;
- Communication of omics findings to clinicians and patients; and
- Lessons from the use of computational precision oncology in clinical practice.
This workshop proceedings highlights suggestions from individual participants regarding potential ways to improve the translation of computational precision oncology into clinical practice. These suggestions are discussed throughout the proceedings and are summarized in Box 1. Appendix A includes the Statement of Task for the workshop. The agenda is provided in Appendix B. Speakers’ presentations and the webcast have been archived online.3 A brief summary of the first workshop in the series can be found in Box 2.
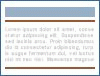
BOX 1
Suggestions from Individual Workshop Participants to Improve the Translation of Computational Precision Oncology into Clinical Practice.
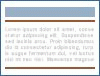
BOX 2
Overview of the First Workshop on Improving Cancer Diagnosis and Care.
NEW PARADIGM IN CANCER DIAGNOSIS AND CARE
Several speakers described the evolving paradigm in precision cancer diagnosis and care due to the advent of new techniques for the molecular characterization of patients’ tumors, as well as advanced computational methods for interpretation of these complex data.
Christopher Cogle, professor of medicine at the University of Florida College of Medicine, compared computational modeling with the more traditional approaches for preclinical assessment of oncology drugs: in vitro and in vivo studies. For in vitro studies, tumor cells are removed from patients or animals and tested in the laboratory with a potential drug or drug combination. However, he said in vitro results are not strongly correlated with clinical efficacy (Burstein et al., 2011). For in vivo testing, potential drugs are administered to animals with tumors. In vivo testing may be more predictive of clinical efficacy, but this has not been shown conclusively, according to Cogle.
By contrast, computational modeling uses large sets of patient and tumor data to predict efficacy of specific therapies for different tumor subtypes. Computational modeling can consider multiple tumor characteristics and drug options simultaneously, and findings can be updated with advancing knowledge. Lisa McShane, acting associate director for the Division of Cancer Treatment and Diagnosis and chief of the Biometric Research Program at the National Cancer Institute (NCI), said that computationally intensive methods are best suited to answering complex questions in which there are many variables to consider and data from a large number of patients. She said that a major challenge in the development of complex computational algorithms in oncology is the lack of sufficiently large datasets; when computational algorithms are derived from an inadequate number of patients, they often fail when tested in independent datasets.
Cogle stressed that the quality of computational modeling output depends on the quality and completeness of the data used. McShane agreed and added that there is a misconception that powerful computational methods can compensate for poor-quality data. However, the application of computational methods on large quantities of poor-quality data typically lead to poorly performing algorithms (see section on Data Quality and Completeness).
Cogle noted that early precision oncology efforts used diagnostic tests to assess the activity of a single gene (e.g., human epidermal growth factor receptor 2 [HER2] in breast cancer) and to match patients to a single drug targeting that activity. Current precision oncology diagnostic testing is more complex, including multiplex testing to identify the tumor’s genetic sequence (genome), active genes (exome), and proteins (proteome). This complex information has the potential to enable more precise targeting of therapies. However, Cogle stressed that “from a regulatory perspective, there is not much guidance on how to use a more complex system other than single-gene–single-drug matching, and our technology is way beyond that.”
Atul Butte, director of the Bakar Computational Health Sciences Institute at the University of California, San Francisco, noted that molecular characterizations of patient tumors show that although tumors often share several common gene aberrations, many also have rare genetic flaws, with some unique to a single patient. For example, he said there may be nearly 1,000 different subtypes of lung cancer, defined by their unique genetic signatures. Some of these subtypes may include highly specific targets for drug therapy that will be effective in only a small number of patients. Keith Flaherty, director of the Henri and Belinda Termeer Center for Targeted Therapy, said, “We need a strategy, both diagnostic and therapeutic, that is going to allow us to make more rapid inroads into potentially very small patient subpopulations.” He also noted that treatment-salient features can vary across tumors, even within an individual patient. If most of a patient’s tumors have a molecular feature targeted effectively by a drug, their cancer will still eventually progress because of the subpopulation of tumor cells that can resist treatment.
Hedvig Hricak, chair of the department of radiology at the Memorial Sloan Kettering Cancer Center, showed how tumor heterogeneity in an individual patient can be revealed with molecular imaging. This imaging can facilitate appropriate treatment selection and is also used in drug development to optimize target engagement and determine appropriate dosage, she noted. “Molecular imaging is essential in patient- and value-driven care, and imaging in drug development is now slowly being accepted around the Western world,” she said.
Howard McLeod, medical director of the DeBartolo Family Personalized Medicine Institute, said genetic testing of a patient’s tumor and germline genome will be pivotal to the success of precision medicine. He noted that genetic information provides diagnostic, prognostic, treatment, and toxicity risk information, and can also suggest appropriate clinical trials for patients. However, genetic reports on individual patients can be as long as 50 pages, with listings of hundreds of variants that may not have clinical significance. McLeod noted that it is difficult to interpret this genetic information, even for clinicians trained in molecular oncology. Lincoln Nadauld, executive director of precision medicine and precision genomics at Intermountain Healthcare, said that when his organization first launched their program of tumor genetic testing, clinicians used the test results less than 20 percent of the time, often because they were uncomfortable interpreting the data.
Mia Levy, director of the Rush University Cancer Center, also emphasized the growth of genomic data, with complex tests now able to detect hundreds of genetic changes. She said clinicians need decision support tools that address which tests to order, how to interpret and report test results, and how to combine genetic features with other patient features to determine an appropriate treatment. Lee Newcomer, principal of Lee N. Newcomer Consulting, agreed, saying, “We can’t take the multiple variables coming in now from oncology patients about the microenvironment, genomics, etc., and process those in a human brain. We need computational methods to drive us in the direction of making more subtle clinical decisions.” Hricak stressed, “Only by proper predictive modeling are we going to have personalized treatment.” Levy added, “We’re at this precipice of change in how we think about clinical decision support in medicine, which has evolved from the evidence-driven paradigm, to protocol-driven care, to data-driven approaches.”
Computational Technologies
Machine Learning
Pratik Shah, principal research scientist and a principal investigator at the Massachusetts Institute of Technology (MIT) Media Lab who leads the Health 0.0 research program, classified computational technologies used in precision oncology in a hierarchy of three major types. At the bottom level is automation. An example of automation is a machine learning computer algorithm trained with thousands of photographs that uses prior data to identify specific features in new images. “This low-level intelligence depends on large datasets and is subject to bias and invalid causal inferences,” he said. The middle level is knowledge creation, such as using machine learning to create new knowledge from data that humans might perceive, but do not understand how to use. The highest level is artificial intelligence (AI), in which the algorithm uses computational processes that are beyond human cognitive capacity.
According to Shah, most machine learning applications are in the form of automation. There are few examples of knowledge creation, he said, and even fewer examples of true AI. McLeod noted that fully AI-driven care may be achievable, but is still a distant goal because “there hasn’t been that hard work done to build the knowledge to have the I in AI.” He noted that such intelligence needs to be able to consider the full patient context, such as kidney function, body weight, comorbidities, patient preferences, and cost.
Shah provided several examples of how computational technologies are being used for knowledge creation to support clinical decisions. In response to a request from clinicians at Brigham and Women’s Hospital, he and his colleagues devised a method to computationally “stain” prostate biopsy tissue slides without physically staining them (Rana et al., 2018). Traditionally, such slides are stained with a dye such as hematoxylin and eosin (H&E) that makes certain diagnostic features more prominent and facilitates cancer diagnosis. Shah and colleagues used a machine learning program to detect those features in tissue samples without staining, and then digitally altered the images akin to what a physical H&E stain would do when applied to the tissue slices. Shah and colleagues also created a neural network system that could digitally remove the stains on old stored samples and digitally re-stain them to identify different features. He is now testing this digital staining with clinicians, most of whom cannot distinguish between the digitally stained and physically stained tissues, and are able to diagnose tumors equally well in both types.
Shah also reported on a project in which he and colleagues used archived clinical trial data to train a computational algorithm to identify novel patterns in treatment toxicity and to use that information to optimize dosing regimens (Yauney and Shah, 2018). The program applies a technique called “reinforcement learning,” which teaches the algorithm to prioritize the goal of tumor reduction while minimizing adverse effects. The computer-driven regimens provided doses at different amounts and intervals and skipped more doses compared to dosing regimens devised by clinicians.
Constantine Gatsonis, founding chair of the Department of Biostatistics and the Center for Statistical Sciences at the Brown University School of Public Health, provided several examples of machine learning programs designed to aid diagnosis and treatment in oncology. One uses magnetic resonance imaging (MRI) features of rectal cancer patients to predict treatment response after chemotherapy and radiation therapy. In a retrospective study of 114 patients, the predictor performed better than qualitative assessments by radiologists (Horvat et al., 2018). Although this study and others have shown promising results, Gatsonis stressed that the clinical utility and stability of the algorithms have yet to be determined. Furthermore, it remains to be seen how reliably the algorithms will perform when tested on patients less similar to those on whose data and imaging they were trained. He also noted that these learning systems will continue to evolve as they are trained on new data.
Wui-Jin Koh, senior vice president and chief medical officer of the National Comprehensive Cancer Network (NCCN), asked how machine learning and AI might help primary care clinicians in the diagnosis of cancers at earlier stages. Bray Patrick-Lake, program director of the Duke Clinical Research Institute Research Together™ program, responded that computational oncology could aid primary care clinicians when deciding whether a suspicious lesion should be biopsied. Newcomer added that computational technologies are being applied to the analysis of circulating free DNA in blood samples to develop tests to detect early-stage cancers. However, he cautioned that some computational screening technologies may not add significant value. He said one study found that a computational method to improve mammography screening did not reduce the rate of false positives, and only increased the breast cancer detection rate by two-tenths of 1 percent (0.002%). Giovanni Parmigiani, associate director for population sciences at the Dana-Farber/Harvard Cancer Center, noted that machine learning and genetic tests could identify primary care patients at high risk for cancer and might motivate behavior change that could prevent cancer. “As we continue our policy discussions about precision oncology, I hope we don’t leave behind the precision prevention side,” he said.
Machine Learning Applications in Radiology
Hricak stressed that the future of medicine lies in the integration of technology with human skills. She said that the increasing complexity of technologies used in radiology will alter the role of the radiologist, so that instead of searching through images and identifying features, the radiologist will synthesize clinical features identified via technology to interpret the findings. Machine learning will help radiologists to evolve from spending a large portion of their time as “film readers” to functioning more fully as physician–consultants who facilitate diagnosis. Radiologists will need to “adapt or perish,” she added, quoting the motto in the logo of the SSG Heathe N. Craig Joint Theater Hospital at Bagram Airfield.
Hricak also noted that a computer program can reliably identify changes in a longitudinal series of patient images within a few minutes. This will save radiologists time, while allowing them to provide a more precise assessment of the treatment response. Lawrence Shulman, professor of medicine and deputy director of clinical services at the University of Pennsylvania Abramson Cancer Center, agreed. He added that machines are not likely to replace clinicians but will improve efficiency and accuracy in oncology.
Health Apps
Gatsonis noted that numerous smartphone apps allow the user to send a photograph of a mole or other skin feature to be evaluated by a clinician or an algorithm. Gatsonis reported the example of a single convolutional neural network (which has potential for smartphone applications) that performed as well as 21 dermatologists in classifying general skin lesions (Esteva et al., 2017).
Butte noted that a new smartphone app that can provide access to patients’ health records at multiple facilities will facilitate the creation and dissemination of other health apps. “That’s the future—I can imagine hundreds of apps in the next year helping patients navigate through their cancer care because I see people writing these apps to deal with health records directly without having to know about various health systems or medical health record systems,” Butte said.
But Frank Weichold, director for the Office of Critical Path and Regulatory Science Initiatives in the Office of the Chief Scientist and the Office of the Commissioner for the Food and Drug Administration (FDA), expressed concern that health data from apps may not be sufficiently authenticated and vetted for quality. Gatsonis added, “Before we put these to use, we need to know that they perform at a certain level. These apps have a lot of potential, but they can also cause a lot of angst and pressure on health care systems.” He said that few mobile apps have been clinically validated and regulated as they should be, especially if they have diagnostic uses (Chao et al., 2017; Wise, 2018). Although FDA has proposed some guidelines for evaluating such apps, “there are no real quality standards and regulatory framework for them,” he said (Chao et al., 2017). He added that there are also ethical concerns related to where the data go, who has access to them, how data will be mined, and whether the devices adequately protect patient privacy.
TRANSLATION CHALLENGES
Several participants described challenges in translating computational technologies for clinical use, including ensuring data quality and completeness, identifying methods to validate novel computational methods, ensuring appropriate regulatory oversight, communicating results and potential risks to patients, and achieving appropriate reimbursement under patients’ insurance plans.
Data Quality and Completeness
Reliable algorithms depend on reliable data, stressed Amy Abernethy, chief medical officer, scientific officer, and senior vice president of oncology at Flatiron Health.4 She noted that many factors affect the reliability of data used to train an algorithm (i.e., completeness, quality, diversity, relevancy, timeliness, and accuracy. Data completeness requires key data elements such as information about cancer diagnosis, treatment, and outcomes. Data quality refers to whether appropriate variables have been measured in valid and reliable ways. Another key feature of data quality is provenance, that is, the original source of the data and how the integrity of the data was maintained as it passed from one record or database to another. Abernethy stressed that potential data for precision oncology should be assessed against each of these requirements before they are used in any clinical or regulatory context, and added that inadequate data quality will result in poorly performing algorithms. McShane agreed, and highlighted the importance of involving individuals with appropriate expertise to make assessments regarding whether data quality is sufficient to be used in the development of algorithms.
Abernethy noted that different types of datasets have different features that affect their reliability. For example, although instrumentation data, such as those generated in genetic tests or by imaging, are initially reliable, they may be compressed, stored, transferred, analyzed, or reported in ways that can introduce error. Often, results from images or tests include textual information that needs to be curated (translated) into an analytical file that can be processed by an algorithm. This curation process can introduce errors if the person performing it misunderstands the material or has incomplete, changing, or conflicting information, Abernethy noted.
Abernethy also stressed the importance of data completeness. She noted that electronic health records (EHRs) are often missing key data that need to be gathered from other sources. For example, a patient whose cancer progresses may have imaging data throughout their treatment, but no pathology data after the initial diagnosis. Increasingly, patient data are not considered complete without information about biological features important for diagnosis and treatment decisions. Levy added that EHRs do not consistently record response to clinical therapy or reasons for treatment discontinuation. In addition, she said, “There’s a lot of missing information, especially when you are limited by the EHR of a single institution, as we miss what happens to patients once they have left the institution, or we’re missing the data that came before they are at the institution.” Furthermore, dates entered into EHRs are often inaccurate when patients are asked to recall past care episodes that occurred at the beginning of their illness.
Abernethy noted that data reliability can be described for each data point in a standardized format. “Standardized reporting of data completeness now can be appended directly to datasets, so you have this information in your hands as you start to work with them,” she stressed. The creation of research-ready datasets that are fit for purpose requires documenting data quality, not only in source datasets, but also in the derived datasets used for final analyses and dataset generation, she added (see Figure 1).

FIGURE 1
The process of making a fit-for-purpose real-world dataset. NOTE: RWD = real-world dataset. SOURCES: Abernethy presentation, October 29, 2018; Daniel et al., 2018.
Risk of Bias
Several participants stressed that inadequately representative datasets that do not include diverse populations lead to the creation of invalid and biased algorithms. Kadija Ferryman, postdoctoral scholar at the Data & Society Research Institute, described a study demonstrating how underrepresentation of black individuals in health research data led to the creation of a genetic test for heart disease that incorrectly classified risk for black patients (Manrai et al., 2016). “A lack of inclusive populations impacts how these genetic variants are classified,” Ferryman noted. She gave another example of databases used to develop algorithms for melanoma detection that included few images of skin lesions in people of African descent (Adamson and Smith, 2018; Lashbrook, 2018). Ferryman noted that since the passage of the 1993 Revitalization Act, which aimed to increase diversity in clinical trials, less than 2 percent of the more than 10,000 cancer clinical trials funded by the NCI included enough minority participants to meet the goals of the National Institutes of Health (NIH) (Chen et al., 2014; Oh et al., 2015). “If this problem of inclusion persists, there are still going to be parts of the population that are going to be left out” when developing precision oncology algorithms with clinical trial data, Ferryman said.
Ferryman stressed that precision medicine focuses on improving care for individuals, but it is also critical to consider its effects on population subgroups before these technologies become widespread. “If we don’t address these issues at the forefront, it’s likely there will be negative impacts as the technologies develop. We need to ensure precision oncology that is equitable and leads to improvement for all of us,” she said.
The need for large and diverse datasets presents a challenge as cancer populations are increasingly divided into small subsets based on genomic classifiers. “More and more big data is moving toward small data,” Abernethy said, so that there are more datasets that represent small numbers of patients. Small datasets run the risk of not being representative of the population. Abernethy also noted that the likelihood that patients have genomic analyses of their tumors differs by race, ethnicity, and age, and that in itself may affect the representation of data in available genomic databases (Presley et al., 2018). “This is particularly important as we think about getting balance in our algorithms going forward,” she said.
Otis Brawley, Bloomberg Distinguished Professor at Johns Hopkins University, also cautioned against drawing population-wide conclusions from analyses performed on small samples of population subgroups, noting that findings from such analyses may not be generalizable. He gave the example of a study of azidothymidine in patients with HIV that concluded the drug was not effective in African Americans based on a subgroup analysis embedded within the larger study. A deeper analysis identified that the apparent effect was confounded by socioeconomic status, and African American patients had failed to respond because they could not consistently access the medication. Brawley concluded, “You can harm the public health by doing these subset analyses just as much as you might be able to help them.”
Validation of Computational Precision Oncology
Prior to entering the market, medical tests need to be validated to ensure that they consistently and accurately measure the intended target and provide clinically meaningful and actionable information. Some workshop participants noted that validation can be especially challenging for omics-based diagnostics and imaging, as well as for the predictive algorithms that drive precision oncology (IOM, 2012; NASEM, 2016). This creates a bottleneck that can slow implementation of new technologies.
One challenge for validation, identified by Gatsonis, is the “moving target” nature of machine learning algorithms. “The software evolves constantly so there’s a big moving target problem,” he said, raising the question “At what point do you evaluate such an algorithm? We need criteria for deciding when a modality is ready to move forward.” Gatsonis noted that one Institute of Medicine report, Evolution of Translational Omics: Lessons Learned and the Path Forward, suggests criteria for omics diagnostics, but not for radiomics (IOM, 2012). Advanced machine learning (i.e., AI) raises additional questions, such as whether there has been appropriate training for the algorithm, and how to calibrate and monitor its performance. Gatsonis stated, “We need to establish processes for monitoring performance and ensuring safety.” He also questioned the reproducibility of some omics tests and algorithms, and recommended that such tests be evaluated both technically and clinically prior to widespread implementation.
Test Development and Analytical Validation Steps
McShane outlined steps for developing and validating an omics predictor prior to clinical testing (McShane et al., 2013a,b). The first step is ensuring the specimen, such as a tumor sample, is adequate for the test. Improper handling and storage can affect the quality of the specimen and outcome of the test. The specimen also needs to be of sufficient size, and should be screened to ensure its DNA or RNA has not degraded. McShane provided the example of a diagnostic study in which 80 of 100 specimens collected were unusable because of improper freezing, and suggested that test developers need to create standard operating procedures to ensure proper handling.
Once a specimen is deemed adequate, the next step is to ensure the test itself is run properly and consistently. Small changes in methodology can have dramatic effects on results. McShane suggested the use of standard operating procedures and quality monitoring, as well as pre-established criteria for assessing specimen quality and batch effects. Researchers also need to assess the analytic performance of a test, including its sensitivity, specificity, bias, accuracy, precision, and reproducibility (Becker, 2015; Jennings et al., 2009).
The next step in test development and validation is to perform preliminary evaluations of the model and algorithm used to make predictions. At this step, researchers need to ensure the quality of the data, and use appropriate statistical methods for model development, performance assessment, and validation. The latter includes defining the clinical context and patient population for the test, and whether its clinical use would be prognostic, predictive, or serve another clinical purpose.
Statistical Validation
McShane noted that a common pitfall in the early validation steps is statistical overfitting, in which an algorithm is trained too closely to a particular set of data, and may therefore fail to fit additional data or reliably predict future observations. Overfitting can occur when the data that were used to develop a predictor are also used to test it. “Plugging in the same data into the model that you used to develop it is a useless thing to do because it will always look like you have a great predictor when in fact you might have a completely useless predictor,” McShane said. She suggested developers test their assays using several different external datasets, as was done for the FDA-approved Oncotype DX test,5 which predicts recurrence in women with early-stage breast cancer (Carlson and Roth, 2013).
Parmigiani also stressed the need to perform cross-study validation using external datasets. He noted, “If you are training a classifier using a machine learning technique, the ability of that classifier to do well on a set-aside piece of the original dataset is going to be way too optimistic in predicting what is going to happen when you take it elsewhere. That is well established.” He added that “if you teach your classifiers on multiple datasets, they will not only learn about what predicts patient outcome, but we will also learn about how that will vary from one context to another and we will only retain the features that are more stable across multiple studies.”
Parmigiani continued, “The ability to take classifiers trained in one context and take them to different contexts is an essential part of the translational process that goes from machine learning to bedside,” adding, “This is one of the important lessons the clinical community has been learning often the hard way over the past few years.” He also noted that the datasets used to train algorithms for health care are often much smaller than those used to develop other applications, and thus the predictions made by these machine learning systems may be less reliable.
Parmigiani and Steven Goodman, associate dean for clinical and translational research at the Stanford University School of Medicine, also stressed the importance of ensuring that validation datasets are unbiased. Goodman suggested using real-world data for validation purposes, noting that race, ethnicity, age, and other factors that may not have been in the eligibility requirements for the original training dataset may influence validation. He added that the validation dataset should include real-world variation in the test procedure, including variation in sample preparation, handling, and transport. “You have to make sure that your training and validation sets reflect some sort of reality,” Goodman stressed.
McShane outlined in more detail the steps for the rigorous validation of a predictor (see Box 3). “There are a lot of really important things that can be done with omics. We just have to make sure that we continue to educate people on how to do things correctly and have the data resources, time, and right kind of expertise for validation for successful development and clinical translation,” she noted.
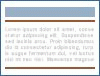
BOX 3
Requirements for a Rigorous Validation of a Predictor.
Clinical Validation
Gatsonis stressed that for clinical validation, algorithms should be evaluated in well-designed prospective studies. McShane added that in a clinical trial, study methodology and endpoints should be defined a priori, and there should be “an honest broker in the form of a statistician or computational specialist, who is the one who can make the link to the outcome data and knows the code, even if he or she can’t look inside it.” But McShane also acknowledged that it may not be possible to design and recruit patients for a clinical trial for every clinical validation study. “That’s why we’re going to have to have some very high-quality databases and specimen banks, so we can do some of these in very carefully designed prospective–retrospective studies,” McShane said. Lukas Amler, senior director of the Late-Stage Oncology Biomarker Development Department at Genentech, agreed with McShane, noting, “We need very significant scale as far as data go.” He suggested that clinical validation for some cancers will likely require a combination of real-world data and data from clinical trials.
With regard to machine learning algorithms that are continually evolving, Gatsonis noted that
we don’t know the way to guarantee that these algorithms are always going to hit a minimum level of performance. There’s no math that can specify conditions and say this is always going to be the case, hence we don’t know that this thing is not going to go off some deep end, depending on what it sees and what it learns. That’s why it’s so important that we need to be able to understand how they work and have new ways to have minimum performance standards as the system evolves.
Gatsonis also suggested establishing processes for monitoring performance and ensuring safety.
Richard Schilsky, senior vice president and chief medical officer of the American Society of Clinical Oncology, stressed that tests need to be validated for a specific use. “People often lose sight of this. They say ‘I’ve got a great technology and it can be used for a million things.’ Well, what should it be used for? Because unless you can declare that up front, then it makes it very difficult to design the appropriate validation studies,” he said.
David Chu, president of the Institute for Defense Analyses, suggested that FDA could rely more on academic partners for validation of new precision oncology technologies because academic institutions should not have a vested interest in the product’s outcome. “Think about the academic establishment as your partner in this to certify results, including the quality of data used for the development of the tools,” he said.
Face Validity
George Oliver, vice president for clinical informatics at the Parkland Center for Clinical Innovation, stressed the importance of transparency and face validity—the perception that an algorithm is taking appropriate computational steps in its decision making. Amler agreed, saying that “A black box isn’t going to work. We have to establish causality so there’s enough reason for us to follow this up to make current drug treatments better.” Gatsonis added, “We care about the face validity in order to be able to educate the physician as to what they have seen and why they’re making a particular diagnosis. If we can’t do that, we’re not going to be able to convince anybody. You cannot make a transparent treatment decision on the basis of a piece of information that is not transparent to you.” Gatsonis stressed that algorithms are decision support tools, and ultimately the clinician retains responsibility for making the appropriate diagnosis or treatment selection. Cogle reinforced this notion, stating, “If a medical oncologist is legally responsible for using the data in an app, we want to make sure we understand how the app or the computational system came to the conclusion it did.” Clinicians need to be able to demonstrate the face validity of decision support algorithms to justify their prescribing choices to insurers, he said.
However, Gatsonis noted that it can be difficult to achieve face validity for complex algorithms. “It is reverse engineering the neural net to be able to understand why it did what it did.” Schilsky pointed out that many oncologists use Oncotype DX, a genomic test to inform breast cancer treatment, without understanding how its algorithm works. “They accept at face value that this is a test that’s gone through an extensive validation process and that the recurrence score actually provides information that can be useful to them,” he said. Shah added, “We think explaining the algorithm is important but should not be rate limiting in creating new knowledge to help patients. We shouldn’t be using the black box phenomena as an excuse to put technology out of the hands of patients.”
Gaps in Reproducibility
Several speakers noted that algorithms for precision oncology often do not hold up to repeated attempts at validation. Goodman provided a number of explanations for this lack of reproducibility, including factors such as:
- Poor training of researchers in experimental design;
- Increased emphasis on making provocative statements rather than presenting technical details;
- Publications that do not report basic elements of experimental design;
- Coincidental findings that happen to reach statistical significance, coupled with publication bias; and
- “Over-interpretation of creative ‘hypothesis-generating’ experiments, which are designed to uncover new avenues of inquiry rather than to provide definitive proof for any single question” (Collins and Tabak, 2014, p. 612).
Goodman added another factor, citing an investigator who had 19 retractions of published research from his laboratory and claimed as his defense: “In these days of complex, interdisciplinary research, one depends on the trustworthiness of colleagues who use the methodologies with which one has no personal experience” (McCook, 2017). As Goodman noted, “This is part of the problem. With computational technologies, even the best of clinical and biologic researchers may have no idea what is going on in the computer and do not know how to look over the shoulders of their colleagues who do have this expertise.” He said in one online survey of 1,576 researchers, nearly 90 percent of respondents agreed that reproducibility of research findings could be improved by having a better understanding of statistics (Baker, 2016). Goodman outlined three types of reproducibility that are relevant in assessing precision oncology tests: methods reproducibility, results reproducibility, and inferential reproducibility.
Methods reproducibility Methods reproducibility is the extent to which research methodology is sufficiently described so that a repetition of the same experiment would produce the same result. Failure to achieve methods reproducibility may be due to a lack of transparency for methods, data and codes, and materials used to conduct the research. Achieving this form of reproducibility requires providing, in a shareable form, the analytic dataset, the methods by which the analytic results were produced, the computer code in human-readable form, the software environment, and documentation so researchers can try to replicate study results (Peng et al., 2006).
Results reproducibility Results reproducibility is the degree of support that subsequent studies provide for the original claim.
Inferential reproducibility Inferential reproducibility is whether the results are interpreted the same way by different people, that is, whether there is consensus in the scientific community about what the results mean. Goodman noted that inferential reproducibility is determined by the strengths of claims made, and the degree of proof, validation, or generalizability.
Regulatory Oversight
Several workshop participants spoke about the importance of regulatory oversight for computational precision oncology and discussed how computational precision oncology’s entry into the clinical market should be regulated. “What level of proof or evidence ought there be for computational algorithms used as treatment decision supports? Is a randomized trial testing their use versus standard of care enough, or do we need a new FDA regulatory mechanism for these algorithms?” Newcomer asked. Cogle added, “At what level do we have reasonable expectation that this technology is ready for prime time? Do we need survival data as a green light or can we use surrogate measures for survival?”
FDA has multiple regulations and standards relevant to omics tests, algorithms, and decision support tools, including regulation of digital data quality, performance standards for diagnostic tests, and regulation of devices, as described below.
Digital Data Standards
FDA regularly receives outcomes data from sponsors from registration of clinical trials as well as from postmarketing surveillance. To facilitate interpretation and use of these data, the agency established data standards through the Clinical Data Interchange Standards Consortium6 (CDISC) for all data it receives. “Traditionally, we used to get data in a variety of different formats that followed a variety of different standards. Every sponsor had their own data center,” said Sean Khozin, associate director of FDA’s Oncology Center of Excellence. The CDISC standards require a unified framework for organizing study data, including templates for datasets, standard names for variables, and standard ways of creating common derived variables. This standardization allows FDA to receive, process, review, and archive submissions more efficiently and effectively (FDA, 2017b). “We can automate a lot of the functions during the review process because the data can be linked, and we can look at data in a more holistic way by looking across several different studies in meta-analyses,” Khozin said, adding, “We are thinking about using the data we have to train algorithms that can be open source and help the entire drug development community.”
CDISC is primarily a clinical data standard and does not address genomic data. However, Khozin noted that there are also standards for reporting genomic data. For FDA purposes, sponsors are asked to provide a spreadsheet with all the relevant genomic data outputs. Khozin explained that FDA does not acquire the raw genomic data because of the complexities of data transmission and lack of technology for analyzing such data as part of regulatory decision making.
Khozin pointed out that as oncology drugs become more targeted and effective, fewer participants need to be treated in a clinical trial to demonstrate efficacy, although large numbers of patients may still need to be screened to identify a subset of patients meeting eligibility for enrollment in the trial. “So now there is a greater need to understand the postmarket experience, that longitudinal journey of the patient. And that is one of the areas where real-world evidence has been very useful and promising—in following the patient’s longitudinal journey,” Khozin said. FDA requires long-term patient follow-up that varies depending on the nature of the drug and observations from clinical testing. FDA also receives spontaneous postmarket reports on drugs from clinicians and patients via their website. “We have a continuous cycle for postmarket safety surveillance and pharmacovigilance,” Khozin said.
Devices
Nicholas Petrick, deputy director for the Division of Imaging, Diagnostics and Software Reliability of FDA’s Center for Devices and Radiological Health, reported that for regulation purposes, FDA divides devices into three classes based on the risk they pose to patients (FDA, 2018a) (see Table 1).
TABLE 1
Device Class and Premarket Requirements.
For all devices, FDA requires sponsors to follow good manufacturing practices, register the device with FDA, and report any adverse events. Class I devices (e.g., stethoscopes and tongue depressors) pose the least risk, and FDA typically does not require safety data for these devices to enter the market. Class II devices (e.g., computed tomography [CT], MRI, and ultrasound scanners), which are considered to pose moderate risk, may have additional requirements, such as postmarket surveillance or premarket data that may require a clinical study. Typically, Class II devices are able to enter the market following the 510(k) pathway, which allows sponsors to demonstrate that the device is substantially equivalent to a product already on the market. Alternatively, Class II devices may come to market via the de novo pathway if there are no similar devices already in use, and general or special controls are considered adequate for ensuring safety and effectiveness.
Class III devices (e.g., novel imaging systems, leadless pacemakers, some in vitro diagnostic tests, and computer software and algorithms) pose the greatest potential risk. Most Class III devices require premarket approval, for which sponsors must submit clinical evidence of safety and effectiveness. To facilitate identification of the appropriate approval pathway, device makers are able to consult with FDA prior to submitting their device for review. These consults (called Q-subs) provide an opportunity for FDA to advise manufacturers on the appropriate regulatory pathway and suggest whether additional evidence may be required. “With Q-subs, companies have the opportunity to ask questions and get feedback before they delve into a large clinical study and potentially get in the wrong pathway,” Petrick said.
Petrick noted that in FDA decisions regarding validation requirements, the indication for a device is equally as important as the type of technology. “It is not just ‘here is my device and this is what it does,’ but what are the actual intended uses? On what populations will the device be applied? These are really important questions that have a large impact on the type of data we might see,” Petrick stressed.
Software as a Medical Device
Petrick identified a new category of devices that the International Medical Device Regulators Forum (IMDRF) calls Software as a Medical Device (SaMD). This software (e.g., machine learning algorithms used to diagnose or monitor disease) is intended for medical purposes independent of a hardware medical device (IMDRF SaMD Working Group, 2013). Petrick noted that many precision oncology tools would be classified as SaMDs.
In 2017, FDA adopted the IMDRF’s basic principles for SaMDs as guidance (FDA, 2017a). The principles for clinical evaluation of SaMDs stipulate that there first must be a valid clinical association between the SaMD and a targeted clinical condition. Medical literature and professional guidelines may be used to establish this association, but it also may require a secondary data analysis or a clinical trial. The next step is to demonstrate analytical validity by providing evidence that the software meets various technical requirements and specifications. The final component of a clinical evaluation is demonstrating clinical validation, that is, evidence that the SaMD has been tested in a target population for its intended use and has generated clinically meaningful outcomes.
Petrick noted that FDA has been regulating SaMDs, especially in imaging, for about two decades. Similar to how devices are classified, FDA has been regulating SaMDs according to how much potential risk they may pose. Bakul Patel, associate director of the FDA Center for Devices and Radiological Health, added that when considering how to regulate algorithms, FDA considers unknown risks as well as known risks of implementing the technology in a clinical setting.
Petrick pointed out that there is a wide range of SaMDs, including both software that will be used by patients in their homes (e.g., software that collects and analyzes data about heartbeats and heart rates) and software in devices used in the clinic. He noted that software systems for displaying images may not require clinical data, but clinical data may be required for devices used for diagnosis or treatment (see Figure 2).
Petrick noted that FDA has received substantial input regarding machine learning and AI tools, especially related to imaging, but their approach to regulating these technologies is still evolving. The agency hopes to develop new guidance for the regulation of SaMDs to meet the influx of new technologies expected in the near future. Weichold noted that as FDA builds its knowledge base regarding computational algorithms and how best to regulate them, it maintains open dialogue with stakeholders. “It’s a learning process and not everything is set in stone. We have to learn together,” he said.
Butte noted that in the past 18 months, FDA approved seven machine learning health applications, including one that is cloud based. However, McShane suggested that many computationally based tests are not being evaluated by FDA because they do not fit in its regulatory framework. Consequently, many oncologists rely on NCCN guidelines when deciding whether to use these tests in patient care. These guidelines are informed by findings published in the medical literature, and McShane expressed skepticism about their validity. “We need to be thinking a little bigger about how we might find alternative mechanisms to give good evaluations of some of these algorithms,” McShane said.
There was substantial discussion about the regulatory challenges posed by modeling algorithms used for clinical decision support. Levy said these algorithms should be able to adapt to the latest data and knowledge, noting that “Because of the nature of the changing therapeutic landscape within oncology, we can’t just go to the FDA with a black box algorithm and say ‘I’m going to lock it down forever.’ We need new and adaptive models that allow us to bring decision support tools based on knowledge that is ever-changing.” McShane, however, expressed concern about this flexibility. She said,
These algorithms rely on certain input variables, and if those input variables are coming from assays that are versioned over time, how does that affect these models? As people mine more data and decide to tweak their computational algorithm a little bit so it works better, how do we evaluate those kinds of things? I’m concerned there can be drift and we’re never going to know it.”
Petrick said FDA is wrestling with how to regulate algorithms that continually learn from data extracted in clinical settings. “It may be great to have these algorithms change and possibly work better over time, but if I have an algorithm specific for the California population, what happens if a clinician using it moves to New York?” he asked. Schilsky noted that clinicians want assurance that an algorithm can help them make better decisions for their specific clinical context.
Schilsky asked if software would be subject to regulatory oversight if it was used as a clinical decision support tool to select drug treatments for a cancer patient based on the results of a multiplex genomic test. Petrick responded that FDA would likely want to regulate such an algorithm, and would require clinical data to support its use. However, he noted that this regulation is still evolving. He said,
Even if the device is not working completely independently but in conjunction with the clinician, its type of analysis would be something that would be regulated and the question is what type of data is going to be needed for that. That’s the type of question we are trying to work through now—what types of data are needed under what scenarios.
Cogle noted that FDA requires explicit labels for drugs that include indications and mechanisms of action, and asked whether those same labeling requirements could be applied to software. He noted that the goal of these labeling requirements is to achieve transparency. “Would software developers be comfortable putting portions of their code in a prescriber’s label?” Cogle asked. Shah responded affirmatively that computer code and data should be made publicly available, but he reiterated that traditional regulatory approaches may be less relevant for algorithms.
Quantitative Imaging Devices
Petrick also discussed assessment of quantitative imaging devices. Quoting from the Quantitative Imaging Biomarker Alliance, Petrick stated that “quantitative imaging is the extraction of quantifiable features from medical images for the assessment of normal or the severity, degree of change, or status of a disease, injury, or chronic condition relative to normal” (Radiological Society of North America, 2018). Quantitative imaging devices can extract a single feature, such as volume measurements, or multiple features from an image or from non-imaging data. FDA is currently developing guidance for single-feature quantitative imaging measurements, which are not typically regulated. “If a company comes in and says ‘I have this tool that can measure the size of a lesion,’ as long as that is under the clinician’s control and there are no claims associated with how well it performs, we do not see data on it and it just goes into the market,” Petrick noted. He suggested quantitative imaging tools could undergo technical assessment and clinical validation, which usually requires a randomized clinical study with patient outcome data. He also stressed that the performance of a single marker, such as CT lesion volume assessment, should be consistent when the same tool is used at different clinical sites and incorporated into devices made by different companies.
Petrick noted that technical assessment of quantitative imaging devices includes evaluation of accuracy and precision. The evaluation of accuracy is often complicated by the lack of reference standards against which results from the device can be compared. For example, radiologists’ assessments of lesion size in a CT image often vary, which makes it difficult to assess the accuracy of lesion size determined by a quantitative imaging device. Evaluation of accuracy of quantitative imaging may also involve an assessment of statistical linearity, which Petrick defined as a measure of how change in the reference reflects proportional change in the measurement. Linearity indicates how well measurements reflect clinical realities. Petrick noted that assessment of accuracy should also include consideration of statistical bias (i.e., the difference between the estimated expected value and the reference standard). He noted that bias may be acceptable in longitudinal assessments as long as it is consistent, so that change observed between two measurements is still accurate. Precision is evaluated in the clinical context and involves assessing the repeatability and reproducibility of findings when measurements are repeated under the same conditions or under types of conditions seen in the clinic.
Laboratory Developed Tests
A laboratory developed test (LDT) is “a type of in vitro diagnostic test that is designed, manufactured, and used within a single laboratory” (FDA, 2018b). LDTs are used often in precision oncology to match patients to appropriate treatments. These tests are not currently regulated by FDA,7 but laboratories that conduct LDTs are subject to requirements under the Clinical Laboratory Improvement Amendments (CLIA), which are overseen by the Centers for Medicare & Medicaid Services (CMS) (CMS, 2013). Laboratories conducting LDTs are required to adhere to certain standards and practices to receive CLIA certification. Companion diagnostics,8 which are included in drug labels, undergo FDA review as part of the regulatory pathway for the drugs with which they are used. However, Flaherty noted that for “any other form of diagnostic, particularly ones that come post approval of initial therapy, [there is] a woefully inadequate system for how to develop and establish them.” Flaherty called for strengthened regulation of LDTs, stating, “We need to establish a bar for how diagnostic tests need to perform and then we need to raise that bar. We need to aspire, at least in cancer, to have LDTs become FDA-approved tests.”
Patient Privacy
Deven McGraw, general counsel and chief regulatory officer for Ciitizen, reported on the evolving legal landscape for patient privacy. She noted that health care privacy is important to patients, and that patients with concerns about confidentiality may withhold health information or fail to seek treatment. Cancer patients in particular often express concerns about whether their health status will affect future employment or their ability to acquire health insurance. “There’s a population of people for whom assuring them that their data will be kept confidential is really essential to them getting care in the first place. These sensitive populations have fears about how that data might be used against them,” McGraw said. However, she also noted that privacy regulations should still allow legitimate use of data, stating “Privacy is about enabling appropriate use of data and good and responsible data stewardship.”
McGraw noted that privacy laws in the United States and abroad are founded on the Fair Information Practice Principles, which were published by the Department of Health, Education, and Welfare in 19739 and later incorporated into the Organisation for Economic Co-operation and Development guidelines (OECD, 2013). Those principles include informed consent and assurance of autonomous choice for patients with respect to sharing health information, as well as safeguards and accountability to ensure that patients’ privacy wishes are honored. However, McGraw explained that consent is not the overriding principle because it is often difficult or impractical to obtain (e.g., in the conduct of retrospective research). Other principles include transparency about how data are being used and data minimization (using the minimal data needed in order to accomplish a legitimate purpose). De-identifying data, that is, stripping it of identifiers, is one way to accomplish data minimization.
McGraw noted that under U.S. law, it is possible to have informed consent waived for some purposes, including retrospective research on large datasets. However, most organizations prefer to de-identify data to satisfy privacy requirements. McGraw explained that there is also a trend for regulators to enable individuals to provide generalized consent for the use of their health information for future research purposes without the need to specify for which projects those data will be used. However, it is unclear how much detail about the nature of future research must be included in consent forms, and it can be difficult to balance the need to provide details about future research with the need for simplicity and conciseness. Other trends in privacy include broadening the definition of “identifiable” or “personal” data and increasing individual rights with respect to data (i.e., right to access and portability, right of amendment, right to restrict uses, right to withdraw consent, and right to be forgotten).
McGraw reported on several recent changes in privacy laws, including the European Union’s (EU’s) General Data Protection Regulation (GDPR) and California’s recent passage of the California Consumer Privacy Act of 2018 (CCPA).
General Data Protection Regulation
GDPR went into effect in the European Union in May 2018. It applies only to data “controllers” and “processors” (European Commission, 2016, 2018). GDPR also covers entities not located in the European Union, but who offer goods and services to EU residents or collect data from subjects within the European Union.
GDPR applies to “personal data” unless the data are made public by an individual or an individual is no longer living. McGraw reported that GDPR defines personal data as
any information relating to an identified or identifiable natural person (“data subject”); an identifiable natural person is one who can be identified, directly or indirectly, by reference to an identifier such as a name, an identification number, location data, an online identifier or to one or more factors specific to the physical, physiological, genetic, mental, economic, cultural, or social identity of that natural person. (European Commission, 2016, Chapter 1, Article 4)
There is some relaxation of individual rights provisions when data are “pseudonymized” or “coded,” but the GDPR does not define “de-identified” data.
McGraw noted that GDPR uses a broader definition of personal data than the Privacy Rule10 promulgated under the Health Insurance Portability and Accountability Act of 1996 (HIPAA). “It is generally believed this is a higher standard than [the HIPAA Privacy Rule], but we don’t know enough about how this law is being interpreted by regulatory authorities to know for sure that is the case,” McGraw said. Under GDPR, all data processing must be “lawful,” with the assumption that consent is required (explicit consent in the case of health information) unless there is a lawfully permitted exception. Security safeguards are required, but the expectations for those safeguards are not set out in detail. A Data Protection Impact Assessment (and in some cases regulatory review) is required for certain high-risk processing activities, such as health data processed in large volume. GDPR permits pseudonymization (replacing personal identifiers with pseudonyms) in order for researchers to process data without acquiring consent, but McGraw noted that it is yet to be seen how this will be enforced. She pointed out that with GDPR, patients not only have the right to acquire their own data, but also have the right to acquire data in a machine-readable format, a requirement that is not included in the HIPAA Privacy Rule (see Table 2).
TABLE 2
Individual Rights Under the General Data Protection Regulation and the HIPAA Privacy Rule.
California Consumer Privacy Act of 2018
CCPA will go into effect in January 2020. The regulation applies to businesses that have gross revenues greater than $25 million, as well as to those that buy, sell, or receive large volumes of California consumers’ personal information (California Legislature, 2018). McGraw noted that many businesses located outside of California will be subject to the law. CCPA broadly defines personal information as information that identifies, relates to, describes, is capable of being associated with, or could reasonably be linked, directly or indirectly, with a particular consumer or household. This information includes biometric and genetic data. CCPA defines de-identified data as information that cannot reasonably identify, relate to, describe, be capable of being associated with, or be linked, directly or indirectly, to a particular consumer, provided that a business that uses de-identified information provides technical safeguards, and business practices prohibit re-identification. McGraw said this de-identification standard is believed to be stricter than the HIPAA Privacy Rule; thus, the HIPAA de-identification standard may not be sufficient for future research.
McGraw noted that CCPA provides some exemptions for health care entities, including for limited types of data collected as part of a clinical trial, although this is a narrow exemption that does not apply broadly to medical research. McGraw also noted that protected health information (PHI) subject to HIPAA are exempt from CCPA. However, once data are de-identified according to the HIPAA standard, these data are no longer considered PHI and lose their exemption to CCPA. To make patient data exempt from CCPA, health care entities may opt to de-identify data using CCPA’s more stringent de-identification standard.
Communicating Results and Risks to Patients
Results from genetic analyses and their association with health risks are often quite complex. Patients’ interpretation of these results is complicated by the prevalence of low health literacy11 and numeracy. Galen Joseph, associate professor in the Department of Anthropology, History & Social Medicine at the University of California, San Francisco, noted that more than 36 million U.S. adults, approximately 20 percent of the population, struggle to read, write, or do math above a third grade level (Kutner et al., 2007; Rampey et al., 2016). Even among a college-educated population, 20 percent of adults cannot identify whether 1 percent, 5 percent, or 10 percent indicates a higher level of risk (Lipkus et al., 2001). Despite the likelihood that patients and families struggle with literacy and numeracy, Joseph noted that clinicians commonly overestimate patients’ skills. “Communication is the most common procedure in medicine,” Joseph stressed, so it is important that this is done well.
One component of poor communication between clinicians and patients is clinicians’ frequent failures to address patients’ health concerns. Joseph described a study on communication of genetic breast cancer risk in which she and colleagues identified “a profound mismatch between what the genetic counselors talked about and what the women actually wanted to know about” (Joseph et al., 2017). Participants were most concerned about their cancer’s likelihood of recurrence and whether family members were at risk. By contrast, counselors typically spent 45 minutes explaining basic information about genetics and describing the patient’s risk for a hereditary condition. Genetics was unfamiliar for most of the participants interviewed in the study, and many found the information so overwhelming they did not fully engage in decision making about testing. “All that information kind of shut them down,” Joseph noted. Health care interpreters also had difficulties with the genetic information they were asked to translate for patients. “They were unfamiliar with genetics and genomics because that’s not something they’re trained in,” Joseph said.
Joseph also described the design of an ongoing study to evaluate strategies for genetic counseling communication (Amendola et al., 2018). Participants who elect to undergo whole exome sequencing to assess hereditary cancer risk are randomized to receive either traditional counseling or modified counseling focused on health literacy. In the modified counseling arm, counselors use simple and direct language that emphasizes connection, and work to build rapport between the participant and counselor (see Table 3).
TABLE 3
Results Disclosure Communication Approaches.
Many of the decisions made in the course of precision oncology therapy require an understanding of risk prediction or probability (e.g., the probability that a treatment will work or the risk that a cancer is aggressive). Many factors affect patients’ risk perceptions in addition to numeracy skills, including the consequences of the risk information, tolerance for uncertainty, prior beliefs about risk level, and cognitive and emotional traits such as pessimism or optimism (Lautenbach et al., 2013). Each patient will interpret information about risk or probability differently depending on these factors, leading them to different treatment decisions, Joseph said. She also noted that some methods of describing risk are easier for patients to understand. For example, most studies of risk communication recommend framing information in terms of absolute risk rather than relative risk.
Joseph concluded by noting that equal access to advanced therapies and technologies is not sufficient to ensure health care equity—effective communication is necessary for the ethical implementation of precision oncology. When considering how to broadly integrate precision oncology into clinical practice, the health care community must develop communication strategies to ensure that information is interpretable by all patients and clinicians. Joseph suggested that strategies for communicating information about precision oncology could be informed by the well-established principles of effective clinician–patient communication. These principles include
- Use plain language that is direct, concrete, jargon free, and communicated in the active voice;
- Verify patient comprehension;
- Adapt communication and documentation for the patient’s literacy and numeracy; and
- Take responsibility for communicating understandably to patients because clinicians, not patients, are responsible for effective communication.
Challenges for Payers
Newcomer described challenges that precision oncology poses for health care payers. He noted that although the cost of genomic sequencing has declined, the cost of many targeted drugs has not. Some payers that cover the cost of tumor genomic sequencing may not cover therapies indicated by the genetic markers identified. Payers are particularly concerned that reimbursing treatments based on tumor mutation rather than cancer type will set a precedent that is considered a new coverage policy, and that this will lead to expectations of drug reimbursement even in cases where there is no clinical evidence of the drug’s efficacy. Newcomer said insurance payments are highly regulated and expected to be consistent. If a medication to treat a particular cancer is covered by an insurer for one patient, it must be covered for other patients as well.
To address the problem of missing evidence of clinical efficacy, Newcomer suggested a proof-of-concept clinical trial in which all patients would have genomic analyses of their tumors. The control group would be randomized to receive the standard of care, including any FDA-approved therapies for treatment of cancers with the identified mutations. The experimental group would receive any therapy targeting the mutations identified, regardless of whether there was clinical evidence to support that choice of therapy. Newcomer noted that if outcomes in the experimental group surpassed those in the control group, it would provide evidence to support the selection of therapies based on tumor mutation in the absence of other clinical trial data, and would establish a path toward payer coverage for such therapies. Michael Kelley, national program director for oncology for the Department of Veterans Affairs (VA), suggested that clinical data from the VA could be used to conduct a retrospective proof-of-concept study that would approximate the clinical trial suggested by Newcomer.
Newcomer noted that the complexity of genomic sequencing tests also presents a reimbursement challenge for precision oncology. He explained that compensation for clinical laboratories traditionally has been based on a model of low cost and high volume. Genomic tests challenge this model because of the time and expertise required for the interpretation of results. Newcomer said that interpretation of genomic sequencing results can require as much as a full day’s time by a Ph.D. scientist, yet there is no existing mechanism to bill for this effort. Newcomer stated, “We have to think about how we define complex decision support systems and what we pay for them if we’re going to see this field continue to progress.”
McLeod agreed that reimbursement for complexity will be a key driver of the integration of precision oncology in clinical care. He added that reimbursement policy could provide incentives to reduce complexity of diagnostic readouts provided by new technologies. As an example, he noted that the readouts for electrocardiogram machines have become simpler as the technology has progressed, with much of the complexity of interpretation accomplished by the machine rather than the clinician. Levy added that unless the complex tests are being performed and interpreted at the same institution, as they are at Intermountain Healthcare, there is no way to recoup the expense of providing molecular interpretation services. “The only way we can get reimbursed for these types of things is to actually lay hands on the patient and see them in a clinical environment. There needs to be some way to facilitate reimbursement so that new decision support tools can be developed,” Levy said.
Newcomer responded that a value-based reimbursement model would incentivize precision oncology as a method of identifying effective therapies, and would circumvent the need to find payment within a fee-for-service paradigm. He said that bundled care could provide financial support for these computational tools “because if the tools you use lead to more efficient care, the decision support algorithms would be paid for by the shared savings component.”
Newcomer also suggested that claims data could be used for post-approval surveillance. He noted that claims data can be used to follow a patient over time, and can serve as powerful tools to study effectiveness and cost-of-treatment regimens if integrated with clinical data. “Think about claims as a tool to integrate into our decision-making process because it gives us a longitudinal record you can’t get anywhere else,” Newcomer stressed.
Oliver suggested implementing payer-supported, data-driven quality improvement methods in health care systems, akin to what is done in the CMS Innovation Center’s Oncology Care Model. These initiatives could include metrics that provide positive feedback and financial rewards for clinicians and health care systems that adhere to quality standards. Joseph Chin, deputy director of the Coverage and Analysis Group at CMS, suggested that CMS could drive adoption of computational decision-making tools by reimbursing their costs, similar to how CMS reimburses costs for shared decision making prior to lung cancer screening. “Once these tools are available and validated, there are mechanisms for a payer to encourage their use by providers,” he said. Chin also noted that CMS has used coverage with evidence development to encourage the collection of data through registries. For example, some reimbursement decisions for cardiology and radiology are tied to health care providers submitting data into registries for subsequent follow-up. These registries are linked to CMS administrative data.
RESEARCH NEEDED
Workshop participants discussed numerous evidence gaps that limit the clinical application of computational precision oncology. Many clinicians expressed frustration that genomic findings often identify genetic defects for which there are currently no effective treatments. “Most genomic results are still not actionable,” Schilsky stressed, noting that FDA lists only 11 genomic alterations as actionable, a small percentage of the more than 500 variants found in some genomic profiles (Lee et al., 2017). Flaherty noted that only 35 percent of genetic defects detected in the NCI MATCH trial could be treated by either an approved or experimental drug. He added that some genes, such as tumor suppressor genes, are known to play a major role in multiple types of cancers, but there are no available treatments to target them. McLeod asked, “If we don’t have good options for patients based on whatever it is we’re measuring in them, why are we doing it at all?”
McLeod noted that a retrospective study of patients with advanced non-small cell lung cancer identified no statistically significant difference in 12-month mortality between patients who underwent broad-based genomic sequencing and those who underwent routine genetic testing (Presley et al., 2018), although a difference in survival was observed at cancer centers that offered access to diverse clinical trials. Cogle described additional limitations of molecularly targeted therapy identified in the SHIVA trial, which enrolled patients with advanced solid tumor cancers for whom standard-of-care therapy had failed (Le Tourneau et al., 2015). All participants in the trial received large-scale genomic testing, and were then randomized to receive either a molecularly targeted agent or a treatment of their physician’s choice. However, there was no molecularly targeted treatment available for more than half of the trial participants. Furthermore, there was no statistically significant difference in survival between the treatment arms.
Flaherty noted that insufficient understanding of the molecular pathways driving cancer partly explains the small number of actionable genetic mutations and the lack of documented long-term effectiveness of targeted therapies. He said that research is at the early stage of understanding heterogeneity of response across cancer types that share common molecular features. Flaherty pointed out a number of biological factors that continue to make treatment response unpredictable and require more research to elucidate. These factors include epigenetic changes that affect gene expression but are not identified in genomic tests, as well as compensatory molecular signaling mechanisms that allow cancer cells to resist treatment (e.g., switching to a different form of metabolism that does not rely on the target inhibited by a treatment). Additionally, the immune response and other tumor microenvironment features influence treatment outcomes in ways that are not fully understood. Flaherty noted, “We have all these other mediators of drug resistance that were really unanticipated and not modeled in preclinical systems.” He added, “The downstream consequences of these drugs are complex to say the least, which heightens the challenge of trying to predict outcomes at the individual patient level.” When trying to understand variability in treatment response, it would also be helpful to have patient tumor biopsies when their disease progresses while being treated, Amler suggested.
More research is also needed on how therapeutic agents can be combined to maximize their efficacy. Flaherty said there is a need both for additional drugs and for diagnostic tests that can identify effective drug combinations for individual patients. Amler agreed, noting the importance of developing diagnostics that can predict appropriate treatment combinations. “We’re committed not just to developing drugs, but also the means for clinicians and their patients to actually do something meaningful with them,” he stressed.
Levy said there will be a growing need for implementation research as computational precision oncology becomes more common in clinical practice. She called for further assessment of factors associated with implementation, noting that clinical research on therapeutic efficacy and safety is held to a high standard, while factors associated with implementation (e.g., decision support) are often ignored. Levy suggested conducting pragmatic studies to assess the impact of decision support tools, and noted that she is currently conducting a randomized, prospective study of a tool used to match cancer patients to clinical trials. Weichold also suggested designing more pragmatic and adaptive clinical trials in order the bridge the gap between clinical research and real-world clinical care. “We cannot continue to separate the two and conduct clinical trials in a bubble,” he said.
Parmigiani noted that an essential step in validating and translating computational algorithms is to take classifiers trained in one context and apply them to a different setting (e.g., a different genetic population). This process will reveal variability in performance driven by patient characteristics, data collection characteristics, and characteristics of the biological assay. “There is always going to be some variation across studies and it’s important to understand this variability because it often contributes genuine scientific insights,” Parmigiani said. He suggested that validation not be viewed as a binary process that an algorithm either passes or fails, and noted that even failures of validation can provide valuable information.
Khozin agreed, noting even if there is only one response to a treatment out of 1,000 patients, that one response is likely a real effect of the therapeutic agent. He suggested that most advanced tumors “do not shrink just by chance.” Khozin noted that such findings from exceptional responders should be further studied. McShane agreed, stressing the importance of studying why some patients also have exceptionally toxic or negative responses to drugs. “We have an obligation to not consider our job done just when we’ve put out our first computational algorithm. We need to go back to these data and mine them for things that are not easy to study prospectively,” she said. Shah agreed, adding that prospective adaptive clinical trials can be used to enrich patient populations with rare genetic variants. Parmigiani added, “The general concept is that a substantially increased degree of adaptivity is what is needed to come to much smaller patient strata that are much more homogeneous and where the level of prediction is much more accurate.”
EXAMPLES OF CARE DELIVERY MODELS FOR COMPUTATIONAL PRECISION MEDICINE
Several participants discussed care delivery models for computational precision medicine, including models that have been implemented at the Moffitt Cancer Center, Intermountain Healthcare, the VA, the University of California, and the Vanderbilt–Ingram Cancer Center.
Moffitt Cancer Center Precision Oncology Program
McLeod reported that the Moffitt Cancer Center built its own precision oncology system to provide clinicians with genetic information about patients’ tumors. This information is represented graphically, numerically, and qualitatively to aid treatment decision making. McLeod said a critical component of Moffitt’s system is its molecular tumor boards, which are composed of multiple physician specialties, including radiologists, pathologists, and oncologists, as well as genetic counselors and bioinformaticists. These boards meet regularly to discuss patient cases and can provide recommendations for patient care management on request.
Moffitt also has a quality improvement pilot to guide drug selection and dosing by identifying patients with a genetic predisposition to adverse drug effects. McLeod noted that the goal of the pilot is to reduce adverse drug effects and improve the quality of patient care, as well as reduce the costs associated with treatment toxicities. The system is assessed on a monthly and biannual basis, and results are used to guide improvements and inform long-term analyses. Metrics of the assessment include the number of patients who saw genetic counselors; to what extent genetic testing altered therapy choice; the incidence of neuropathy and cardiovascular toxicities; net revenue or financial loss from the pilot; and patient and clinician feedback on the testing process and quality of care. “Quality improvement is needed to find the right fit for your health system,” McLeod noted. He added that “rational therapeutics, risk mitigation, and budget impact analysis endpoints will really help us go forward in terms of quality of care, but also influence our payer strategies. Payers are interested in data and want to make good decisions, so they can be good partners on this.”
Intermountain Healthcare Precision Oncology Program
Nadauld reported on the Intermountain Healthcare system for precision oncology decision support. Intermountain is composed of 23 hospitals, 180 medical group clinics, and nearly 1 million patients insured by its health plan. Patients do not need to be covered by its health plan to be treated at Intermountain facilities, nor do clinicians need to be employed by the system to practice in its clinics and hospitals.
Nadauld noted that Intermountain followed a workflow map when implementing its precision oncology system (see Figure 3). Genomic sequencing is performed internally to decrease turnaround times and improve data quality and cost control. For every patient’s tumor, Intermountain provides a listing of all relevant mutation types in 165 genes.
After a survey revealed that clinicians were uncomfortable interpreting the data provided by this tumor analysis, Intermountain established an internal molecular tumor board that also includes external experts. This board meets regularly to review each tumor sequencing test ordered and performed within the Intermountain system. The board’s interpretation of the genetic analysis is presented in a report that identifies findings salient for treatment decisions. For example, the report recommends appropriate drugs and lists them in order of priority. More detailed information is also provided later in the report for clinicians who find it useful. “What they really want is right there on the front page, and we even include an order button so that if the doc liked the gene–drug match that they saw, they could go ahead and order the drug and we would engage a drug procurement team to help obtain that drug,” Nadauld said.
To assess the efficacy of its precision oncology approach, Intermountain identified patients with advanced cancer and compared outcomes between patients who received standard next-line therapy and those who received genomic testing and targeted therapy. This study found that patients in the precision oncology group had nearly double the overall survival rate compared with patients given standard therapy (median overall survival of 52 weeks versus 26 weeks) (Haslem et al., 2018). Furthermore, the cost of treatment per week of survival was lower in the precision oncology group than in the standard therapy group. Compared with those receiving standard therapy, patients in the precision oncology group also had lower overall costs in the last 3 months of life. Outpatient drug costs were 300 percent higher in the precision oncology group, although some of this additional cost was offset by savings from reduced inpatient hospital events. Based on these findings, Intermountain’s health plan changed its policy to cover payment for molecular testing in patients with advanced cancer.
Nadauld noted that Intermountain’s precision oncology efforts have been informed by its biorepository of 150,000 patient tumor samples that are linked with clinical outcomes data. Intermountain recently launched a high-throughput sequencing center with the capacity to analyze 20,000 genomes per year. He said they are
now in the process of pulling samples out of our biorepository, doing the whole-genome analysis, combining that with the clinical outcomes, and then making predictions about how to treat future patients based on what has happened to past patients, using a variety of AI tools to better understand and characterize these different patient cohorts.
Department of Veterans Affairs’ National Precision Oncology Program
Kelley reported on the VA’s National Precision Oncology Program, which began in 2016. In this program, patients’ solid tumors are tested for variants of 20 genes. The agency has recently started screening blood cancers as well. More than half of all VA centers now offer this testing. Together, they are genetically analyzing nearly 1,000 tumor samples every quarter.
Kelley said the test results suggest FDA-approved “on-label” treatments for only 8 percent of patients. Nearly half of the treatment recommendations are for “off-label” therapies, for which there is no clinical evidence of efficacy. He said the VA does not have many clinical trials in which to enroll these patients, and is currently trying to increase the opportunities to offer off-label treatments in clinical trials by collaborating with the NCI and other partners.
Kelley noted that in addition to genomic testing, the VA employs an on-demand and patient-specific electronic consult service that is facilitated by its unified EHR system. This service allows clinicians to seek advice on patient care from multidisciplinary teams that consider a patient’s entire clinical context and provide recommendations with 72 hours. The VA also has a molecular tumor board, as well as a genomic medicine service that provides telehealth genetic counseling for patients found to have germline genetic alterations.
Kelley noted that the data from the VA’s genomic sequencing vendors is provided to the patient’s clinicians and placed into a central database (see Figure 4). This database allows the VA to augment genomic results with data on drug exposures, clinical response, and other patient elements.

FIGURE 4
Department of Veterans Affairs’ National Precision Oncology Program data flow. NOTES: FASTQ is a sequence file format. PDF = portable document format; VCF = variant call file. SOURCE: Kelley presentation, October 30, 2018.
University of California
Butte reported on the University of California’s health data research effort, funded by the Chan Zuckerberg Initiative, that is working to integrate precision medicine in clinical care. He said that all 10 University of California campuses are partnered with UnitedHealth Group, with the plan to combine the campuses into a single accountable care organization over the next decade. This merger will require standardizing health care data from six University of California medical schools and health care systems. Four of the six health care systems use Epic for EHR data. These health records are harmonized using a translational research platform called Informatics for Integrating Biology and the Bedside, and placed into a central database. At a Center for Data-Driven Insights and Innovation, the EHR data are combined with limited financial data, state regulatory data, claims data, and death registry data (see Figure 5).

FIGURE 5
Infrastructure of the Center for Data-Driven Insights and Innovation. SOURCE: Butte presentation, October 29, 2018.
Butte reported that the University of California, San Francisco, conducts its own genomic testing, but patients may also have testing performed by a private facility. An optical character recognition code enables computerized reading and processing of both internal and external genomic reports. University of California researchers use these genetic data in research, including genome-wide association studies, deep-learning models for image-based diagnostics, and predictive models for drug efficacy. To facilitate research, the Observational Medical Outcomes Partnership Common Data Model12 is used to create standardized de-identified datasets.
Vanderbilt–Ingram Cancer Center’s My Cancer Genome
Levy reported on the Vanderbilt–Ingram Cancer Center’s My Cancer Genome, which curates the clinical significance of genomic alterations in cancer. This knowledge-driven, Web-based application for clinicians, patients, caregivers, and researchers gives up-to-date information on mutations that drive cancers and the related therapeutic implications. Users can search for mutation-specific treatments and clinical trial options locally, nationally, and internationally.
Since 2010, the system has incorporated the genetic profiles of tumors from more than 8,000 patients. Of those, more than 900 patients went on genomically informed interventional clinical trials. “We consider that to be a huge success based on the fact that we’ve largely been sequencing patients who have metastatic disease,” Levy said.
LESSONS LEARNED FROM IMPLEMENTING COMPUTATIONAL PRECISION ONCOLOGY CARE
Several participants provided lessons learned from implementing computational precision oncology care. These lessons include the need for strategic and financial support, a patient-centered and clinician-friendly design, standards, and multidisciplinary teamwork. Other lessons concerned the need to improve clinician understanding of omics and analytics, share data, and implement regulatory and payer measures that could help foster translation of computational precision oncology into the clinic.
Financial Support
Several participants discussed the need for institutional financial support in the implementation of computational precision oncology, including funds for establishing and maintaining databases, conducting validation studies, and developing health care systems that can seamlessly integrate precision oncology data.
McShane noted that although the NCI has worked to establish databases for precision oncology, the effort has been complicated by the lack of resources available for the creation and maintenance of these databases. She stated, “If we want good quality data and we want to really capture a lot of data, somebody has to pay for that. Even the preparation of a dataset to put into one of these data archives requires time and resources and we’re not paying well for that.”
Parmigiani said it can be difficult to identify expertise and financial resources for validation of computation methods. “Replicability and reproducibility are extremely expensive and difficult to do. It is a resource-intensive enterprise,” he said. Gatsonis recommended additional financial support for validation and development, noting that these efforts are supported primarily by NIH funding and venture capital.
Oliver stated that securing ongoing investment from philanthropic, governmental, or pharmaceutical organizations will be a key factor in the successful implementation of computational precision oncology in health. He described his institution’s investment in a nonprofit focused on the implementation of prescriptive analytics and machine learning applications in clinical care. “We have investments in IT [information technology] data architects, data scientists, statisticians, and clinical investigators, who are all brought under one roof,” Oliver added. He said financial incentives will drive the field of computational precision oncology, and that leadership at the executive level of organizations can also “help bring focus to the problem.” McLeod added, “Financial and strategic support from leadership is very important and they have to be able to see that spending a dollar will save them money or will give them market advantage, or in some way make it worthwhile going forward.” Butte agreed, saying, “It’s amazing what you can do when the health system CEO gets what you’re trying to do.”
To avoid future expenses and efforts, McLeod suggested that precision oncology systems should be flexible to accommodate new information and technologies. He noted that precision medicine has been structured around the implementation of genomics in oncology care. However, if new tools such as metabolomics and proteomics become more widespread, systems will need to incorporate new types of data.
Patient-Centered and Clinician-Friendly Design
Workshop participants discussed the importance of designing computational precision oncology applications that are user friendly for both clinicians and patients. Noting that the burden posed by EHRs contributes to clinician burnout, Levy stated that it is important to design precision oncology systems to fit into the workflow of clinical care. She suggested that precision oncology systems should be evaluated not only for their ability to improve care outcomes, but also for their harmony with clinical workflows. Oliver added that it is helpful to implement new clinical systems in stages so they can be validated and calibrated prior to full deployment across a health system.
Patrick-Lake stressed the importance of ensuring that patients remain at the center of computational precision oncology, and suggested that patients should be engaged in the development of new analytic strategies. She noted that engagement is “a bidirectional relationship that creates mutual benefit,” and that developers should use patient expertise and return value to patient populations whose data are used to create precision oncology techniques. Patrick-Lake suggested these goals can be achieved through the development of patient-centered strategic plans that are supported with adequate resources and include measures of desired outcomes.
Patrick-Lake also expressed concern about racial and ethnic disparities, and stated that it is important to investigate the fundamental causes of minority underrepresentation in datasets used for precision oncology. She suggested that one cause of minority underrepresentation is the perceived lack of empowerment during interactions with health care systems, and she suggested that patient advisory groups and patient advocates could help to engage diverse patients and communities and to reengage groups that feel disenfranchised.
Cogle also advocated for patient-centered development and application of computational precision oncology, and noted that patients’ concerns are often different from those of their clinicians. He suggested that patients could be engaged in the design and implementation of computational precision oncology tools to ensure they address outcomes important to the patient. McLeod said one outcome important to patients is treatment toxicity, noting that it is a frequent reason for stopping therapy. He suggested that clinicians should devote greater attention to toxicity and its effect on quality of life. McLeod noted, “We need to have more of a focus on the patient and what their goals are if we’re going to achieve precision medicine goals, beyond just having a fancy technology.”
Khozin noted that although patients’ clinical outcomes are the ultimate measure of therapeutic value, current regulation of diagnostics and treatments is focused on the product, not the patient. For example, each of the three phases of premarket drug development, as well as the postmarket phase, use a different sample of patients. Khozin suggested reconfiguring this evaluation platform to align it with the longitudinal experiences of patients. “If we are interested in the patient’s journey, why not follow the same patient from the time that they enter the clinical trial until the postmarket phase of clinical development? If we do this right, hopefully we will have a development paradigm that is patient oriented instead of product oriented,” Khozin said.
Informed Consent
Several participants suggested simplifying the consent process within oncology, noting that long and complex informed consent documents fail to communicate important information to patients. David Magnus, Thomas A. Raffin Professor of Medicine and Biomedical Ethics, professor of pediatrics and medicine, and director, Center for Biomedical Ethics, Stanford University, suggested that the research community needs to reevaluate the way it communicates with patients, noting that written communication is insufficient for communicating complex information to patients. He said, “We need to go back to square one in terms of how we communicate with patients, knowing it is going to be imperfect, but the question is what are the different ways we can use informed consent and other tools to demonstrate respect for patients.” He suggested that innovative strategies such as illustrated comics and videos tend to be more effective. McGraw agreed that the framework for informed consent should be revised, and suggested that presenting patients with a nearly unintelligible consent form fails to honor their contributions to research.
Schilsky said that the range of potential outcomes from precision oncology is often not communicated clearly and that patients often do not understand the potential limitations of genomic analysis, including the possibility that analysis will identify tumor mutations for which there are no therapies with demonstrated clinical efficacy. “We have to take some responsibility as a community for setting the record straight on where we are in precision oncology and conveying that clearly to patients,” he said.
Improve Clinician Understanding of Omics and Analytics
Butte, Schilsky, and Cogle noted that precision oncology reports are complex and may be confusing to clinicians who have not had sufficient training. Cogle said there is often a 6-month learning curve before medical oncologists begin to accept an algorithm’s recommendations for effective therapies. Chu suggested that medical schools should offer additional training on statistics and data analytics to better prepare clinicians to interact with computational systems. Oliver agreed, and suggested updating medical, nursing, and paraprofessional curricula to include training for understanding, evaluating, and applying predictive models. Petrick added that clinicians need to be trained in how to interact with AI so they understand its limitations. Hricak agreed, saying, “That’s why we call it augmented intelligence and not artificial intelligence.”
Standards
Workshop participants suggested several ways in which greater standardization could improve the field of computational precision oncology. These suggestions included standardization across databases and system interoperability, the development of standards for validating new technologies, and the development of standards for cancer genomic testing.
Abernethy said that policies should help to ensure that datasets are accurate, complete, and incorporate relevant data types; that there is transparency about data reliability and quality; and that data outputs of algorithms are accurate. She also suggested creating standards for documenting reliability, quality, and accuracy at the level of the data source, dataset, and algorithm. Weichold stressed that “data quality, authenticity, and provenance are critical and we need to work together now to solve those problems.” He suggested developing consensus data standards with the support of stakeholder organizations, such as the Institute of Electrical and Electronics Engineers, and noted the BioCompute Object standard that FDA is in the process of developing. “We are struggling the most right now because we do not even understand the evidence that we are using to feed machines because we don’t have the opportunity to link it back to the source data,” Weichold said.
Standards for interoperability are also needed, Oliver stressed. He said some efforts already have been made in this regard, including the Fast Healthcare Interoperability Resources13 and Health Level Seven International.14 The former is a Web interface standard for which users can request all the laboratory data on a patient, for example, assuming the patients’ health care system has entered those results into the resource with a link to standard ontology such as Logical Observation Identifiers Names and Codes. “Most haven’t and so there are existing barriers to this,” he said.
A few participants emphasized the need for consistent evidence standards for data and interpretation in computational precision oncology. Oliver noted that although his institution has defined its own standards for evidence, other institutions have different standards, which makes it difficult to share resources when building analytics. Levy and Kelley agreed with the need for a standardized level of evidence framework, and Levy noted that “we clearly aren’t all talking about the same frameworks when we’re having these conversations.”
Newcomer stressed the importance of validation standards for computational algorithms. “They are not a drug, but don’t we want the same thing that we ask of drugs from these computational black boxes? We would like to know that when we use it, it will not cause harm, and more importantly, it will help us deliver the best possible care for that particular patient,” he said. Chin agreed, stating, “We want to see evidence on clinical utility—that using a test or computational method associated with those tests would actually improve health outcomes.” This is what CMS requires for coverage of interventions, he noted.
Oliver suggested that quality performance standards, such as the Healthcare Effectiveness Data and Information Set or similar standards, could include a recommendation that everyone at age 40 or older be offered genetic testing for cancer risk. This could help build the evidence base needed for validation studies that would put genetic risk assessment on firmer footing. Amler pointed out that making genetic testing a standard of care for patients diagnosed with cancer would also provide evidence that could be used to validate diagnostics, decision support tools, and new targeted therapies. Such standards might also facilitate the entry of patients into clinical trials because many of these trials require such testing. “We need to have comprehensive testing everywhere,” Amler said.
Data Sharing
Several participants noted that computational precision oncology requires large and inclusive datasets that can only be created via data sharing. Cogle said that health systems are increasingly participating in data-sharing consortia that will facilitate access to the massive datasets required for precision oncology, and he suggested that state-based consortia could be joined in an even larger data-sharing effort. Levy discussed a number of data gathering and data-sharing enterprises relevant to precision oncology, including Project GENIE15 (Genomics Evidence Neoplasia Information Exchange) as well as several for-profit companies such as Flatiron Health, Foundation Medicine, and Tempus. She also noted the growth of patient-driven data-sharing initiatives, such as Count Me In16 and the Memorial Sloan Kettering Make-an-IMPACT initiative,17 which allows patients to share their clinical and molecular data to advance medical research.
Abernethy suggested that there are also many types of real-world datasets that could be used for precision oncology, including imaging, instrumentation data generated by machines, EHR or administrative data, and patient-recorded data. However, unless these health data can be de-identified, they may not be sharable. Medical images, for example, are hard to de-identify. “You have to decide how you are going to deal with that in an appropriate way if you want to store and reuse images over time,” she said. Abernethy also stressed that researchers and clinicians need to be aware of the security of datasets, and ensure that data security is maintained as data are moved among databases. Butte noted that the HIPAA Privacy Rule regulations on data sharing are much less stringent for data from patients who are deceased. “We can collect a lot of more of this data and share what we know,” Butte said.
Large-Scale Public Databases
Several speakers noted the value of creating large, comprehensive, and shareable databases for precision oncology. Oliver suggested that data generated in the course of routine clinical care could be pooled to create a “data commons.” Kelley agreed, saying, “We need to be able to develop the knowledge base in a public way. There are lots of people who have siloed their annotated databases and it would be very helpful to have a public knowledge base about which genetic variants are pathogenic.” He pointed to Project GENIE (see Box 4) as well as the Clinical Genome Resource (ClinGen)18 and the ClinVar19 database as examples of public databases. Oliver added that the All of Us Research Program and the UK Biobank20 are also examples of projects with publicly accessible genomic data.
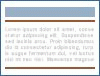
BOX 4
Project GENIE.
Incentives for Sharing Data
Several participants suggested providing incentives for researchers and institutions to share data. Levy discussed strategies to promote data sharing at research institutions. She noted that one barrier to sharing is the cost associated with contributing data to a shared resource, including the cost of converting the data into formats compatible with public repositories. These costs are not typically covered by the funding for precision oncology studies, but Levy suggested they could be incorporated in grant applications to provide financial support for data sharing. McShane said NIH could provide such incentives because of its role as the major funder of health research in the United States. She noted, however, that it can be difficult for NIH to enforce data-sharing requirements because of proprietary content and regulations that aim to protect human subjects of research. For example, Institutional Review Boards, which interpret those regulations at research institutions, may not allow genomic data to be shared without individual consent.
Another strategy Levy discussed is to acknowledge researchers who contribute their data to public databases. She suggested that acknowledging researchers who contribute data would provide credit for their contribution to the field and could be used as evidence to support academic promotion.
Enabling Patient Sharing of Data
Several participants noted that patients would like to share their data for research purposes. Magnus described a study that found that “patients are more excited about data sharing than researchers are” (Mello et al., 2018). McGraw agreed, noting that the patient groups she has interacted with advocate for sharing their data to help other patients. However, it is often difficult for patients to allow their data to be shared because health care institutions do not have systems in place for data sharing. Levy suggested that new technologies, such as Blue Button 2.0,21 could empower patients to share their data directly without relying on health care institutions to serve as a broker.
Schilsky pointed out that although HIPAA regulations give patients the right to request their health data, health care providers do not necessarily have to provide those data in a usable format. He noted that patients who request their medical records are often provided with a PDF, which does not allow for easy data abstraction. McGraw responded that although there are companies trying to develop methods for abstracting data from PDFs, “all that work would not be necessary if the data were provided in a portable manner.” She noted that there are standards, certified by the Office of the National Coordinator for Health Information Technology,22 that describe how EHR data should be made available to patients. McGraw suggested that although these efforts are useful, additional work is needed to provide patients with their health care data in a portable and adaptable format.
Butte described a function of the iPhone’s native health app that can track and update medical records from multiple health sources based on a geocode for the user’s location. This can enable patients to access and share diverse health data from multiple facilities without needing to individually access patient portals. Butte suggested that 500 health systems have already agreed to share their data with Apple so patients can access their records via the app. Butte also noted that developers are working on health apps that are able to translate technical health information for lay consumers. “We’re going to write these apps to help patients understand their health data because patients can get these data today,” he said.
Although Butte agreed that “the more we can share, the more we can move forward together,” he stressed that “there’s only so much patients can do by themselves in disease-oriented groups. I think the responsibility is on the medical system to share, too.”
Khozin added that FDA has launched a block chain effort to enable data sharing. The neutral platform they provide allows for the exchange of data without the need for involvement of a third party. In the United States, data submitted to FDA becomes public information as soon as the product for which it was collected has obtained approval to go on the market. Depending on how certain FDA statutes are interpreted, patient data under review by a government process could also be public information, according to Weichold.
Data Protection
Several participants noted that legal protections for patient data determine if, when, and how easily data can be shared (see Patient Privacy subsection). McGraw suggested that sharing of clinical data for research purposes should be routine, but she also noted there should be standards to protect patient privacy. These standards should include data minimization techniques to reduce identifiability and responsible brokering of data access. McGraw suggested that sharing of patients’ EHR data should be subject to ethical and normative standards, and noted that GDPR does allow for processing of data to contribute to scientific research. However, there is confusion about the meaning of “research purposes” and what types of data processing are allowed without obtaining consent. In addition, McGraw noted that CCPA provides an exemption for health data shared in clinical trials, but does not discuss other types of research. McGraw suggested that future privacy regulations should strive for broad informed consent that enables patients to agree to reuse of data for research, similar to what is done for the All of Us Research Program. Newcomer said that in the United Kingdom, it is routine to share health data, with national consensus on the issue.
Patrick-Lake noted that patients who want to share their data also want to know how their data are being used and whether the data are accurate. McGraw responded that the Fair Information Practice Principles specify that patients should be informed about how their data are used. In practice, however, there is often little effort to engage directly with patients or explain data applications. McGraw noted that there are exceptions to this lack of patient engagement, including some projects of the National Patient-Centered Clinical Research Network, in which patients regularly provide input on the types of research projects of interest to them, and patients are informed about progress of the research in which their data are used. “There are lots of ways to do this and they all center around not treating people as subjects, but treating them as participants. Many research initiatives around the country have started to go down that path,” McGraw said.
Multidisciplinary Teamwork
McLeod stressed that creating and implementing a computational precision oncology system requires engaging multidisciplinary teams, including pathologists, oncologists, pharmacologists, statisticians, and health information technology specialists. Oliver and Chin added that mathematicians and genetic counselors are also important participants. Abernethy noted that even the construction of a dataset used to generate computational algorithms requires many different types of expertise, including clinical, analytic, software, hardware, and privacy. However, Ferryman noted that in her interviews with computer scientists who build algorithms, many said involving clinicians in data curation and interpretation was too expensive.
Several participants identified a need to bridge the gap between computer sciences and medicine. “We need to bring the machine learning community and the clinical community closer so they both talk the same language and so machine learning people can validate their tools on relevant data,” Parmigiani said. Hricak agreed, stating
There is a complexity of cancer diagnosis and treatment that requires a multidisciplinary approach on both the human side and the data side. Unless we work together, it will always be one gene-one-drug that didn’t work. We have integrated teams of oncologists, surgeons, radiologists, and pathologists, but we also have to have biomedical information specialists. They can no longer be in a different building far away, but instead have to be with us and understand the questions we are asking.
Shah described his new research lab, Health 0.0, which aims to facilitate these interdisciplinary collaborations (see Box 5).
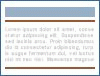
BOX 5
Health 0.0 at the Massachusetts Institute of Technology.
Ferryman also suggested that teams developing computational precision oncology should include public health researchers or other social scientists. Experts from these disciplines can contextualize the collected data and ensure that datasets do not embed biases that will prevent precision oncology advances from benefiting all members of society.
WRAP-UP
Cogle identified several key points from the workshop. He said computational technologies that interpret patient data should be evaluated for clinical utility and should be subject to FDA oversight. He called for greater clarity on regulatory oversight for computational interpretations of patient data, but also noted the value of FDA’s risk-based approach to regulating SaMD. He emphasized the need for well-designed prospective trials and appropriate training and calibration of machine learning algorithms, and added that strategies and criteria are needed for evaluating these tools and deciding when a software product is ready for clinical practice. He also noted the need for monitoring the ongoing performance of algorithms and machine learning applications used in clinical practice.
Cogle also stressed the importance of the face validity of how precision oncology algorithms aid diagnosis or treatment selection, and called for improved training on omics data and statistics to help clinicians understand the computational tools they use. He also pointed to the importance of molecular tumor boards in helping clinicians integrate precision oncology in patient care, noting that institutions lacking local expertise in precision oncology could use telemedicine consultations. Cogle also suggested that strong institutional leadership is important for successful clinical implementation, and that computational technologies can be added to existing EHR systems.
The difficulty in communicating omics findings to patients and their families should be addressed as well, Cogle said. He also noted that privacy laws protecting patient health data are becoming more complex and fragmented, and there is a need to adapt the informed consent process for use of patient data in research, and to protect data collected from health apps that patients use on their mobile devices or computers.
Cogle identified the need for better data standards and emphasized the importance of using high-quality data in the development and evaluation of computational methods, as well as the importance of transparency for precision oncology algorithms. He also stressed the importance of reproducibility of computational precision oncology findings across different datasets and contexts, and noted concerns that data used to develop decision support algorithms may be not representative of diverse populations. In addition, Cogle said that current computational precision oncology technologies often fail to consider heterogeneity within patients’ tumors, and he emphasized the power in sharing data among institutions.
REFERENCES
- Adamson AS, Smith A. Machine learning and health care disparities in dermatology. JAMA Dermatology. 2018;154(11):1247–1248. [PubMed: 30073260]
- Amendola LM, Berg JS, Horowitz CR, Angelo F, Bensen JT, Biesecker BB, Biesecker LG, Cooper GM, East K, Filipski K, Fullerton SM. The clinical sequencing evidence-generating research consortium: Integrating genomic sequencing in diverse and medically underserved populations. The American Journal of Human Genetics. 2018;103(3):319–327. [PMC free article: PMC6128306] [PubMed: 30193136]
- Baker M. Is there a reproducibility crisis? Nature. 2016;533(7604):452–454. [PubMed: 27225100]
- Becker R. Analytical validation of in vitro diagnostic tests. In: Matsui S, Buyse M, Simon R, editors. Design and analysis of clinical trials for predictive medicine. Boca Raton, FL: CRC Press; 2015. pp. 33–50.
- Burstein HJ, Mangu PB, Somerfield MR, Schrag D, Samson D, Holt L, Zelman D, Ajani J. American Society of Clinical Oncology clinical practice guideline update on the use of chemotherapy sensitivity and resistance assays. Journal of Clinical Oncology. 2011;29(24):3328–3330. [PubMed: 21788567]
- California Legislature. Assembly bill no. 375. 2018. [January 11, 2019]. https://leginfo
.legislature .ca.gov/faces/billTextClient .xhtml?bill_id =201720180AB375. - Carlson JJ, Roth JA. The impact of the Oncotype Dx breast cancer assay in clinical practice: A systematic review and meta-analysis. Breast Cancer Research and Treatment. 2013;141(1):13–22. [PMC free article: PMC3902996] [PubMed: 23974828]
- Chao E, Meenan CK, Ferris LK. Smartphone-based applications for skin monitoring and melanoma detection. Dermatologic Clinics. 2017;35(4):551–557. [PubMed: 28886812]
- Chen MS Jr, Lara PN, Dang JHT, Paterniti DA, Kelly K. Twenty years post-NIH Revitalization Act: (EMPaCT): Laying the groundwork for improving minority clinical trial accrual: Renewing the case for enhancing minority participation in cancer clinical trials. Cancer. 2014;120(Suppl 7):1091–1096. [PMC free article: PMC3980490] [PubMed: 24643646]
- CMS (Centers for Medicare & Medicaid Services). CLIA overview. 2013. [January 11, 2019]. https://www
.cms.gov/Regulations-and-Guidance /Legislation/CLIA /Downloads/LDT-and-CLIA_FAQs.pdf. - Collins FS, Tabak LA. Policy: NIH plans to enhance reproducibility. Nature. 2014;505(7485):612–613. [PMC free article: PMC4058759] [PubMed: 24482835]
- Daniel G, Silcox C, Bryan J, McClellan M, Romine M, Frank K. Characterizing RWD quality and relevancy for regulatory purposes. 2018. [January 9, 2019]. https:
//healthpolicy .duke.edu/sites/default /files/atoms/files /characterizing_rwd.pdf. - Esteva A, Kuprel B, Novoa RA, Ko J, Swetter SM, Blau HM, Thrun S. Dermatologist-level classification of skin cancer with deep neural networks. Nature. 2017;542:115. [PMC free article: PMC8382232] [PubMed: 28117445]
- European Commission. Regulation (EU) 2016/679 of the European Parliament and of the Council. 2016. [January 11, 2019]. https://eur-lex
.europa .eu/legal-content/EN /TXT/?qid=1528874672298&uri =CELEX%3A32016R0679. - European Commission. 2018 reform of EU data protection rules. 2018. [January 11, 2019]. https://ec
.europa.eu /commission/priorities /justice-and-fundamental-rights /data-protection /2018-reform-eu-data-protection-rules_en. - FDA (Food and Drug Administration). In vitro companion diagnostic devices: Guidance for industry and Food and Drug Administration staff. 2014. [January 11, 2019]. https://www
.fda.gov/media/81309/download. - FDA. Software as a medical device (SaMD): Clinical evaluation—guidance for industry and Food and Drug Administration staff. 2017a. [January 11, 2019]. https://www
.fda.gov/downloads /medicaldevices /deviceregulationandguidance /guidancedocuments /ucm524904.pdf. - FDA. Study data standards: What you need to know. 2017b. [January 11, 2019]. https://www
.fda.gov/downloads /Drugs/DevelopmentApprovalProcess /FormsSubmissionRequirements /ElectronicSubmissions /UCM511237.pdf. - FDA. Classify your medical device. 2018a. [January 11, 2019]. https://www
.fda.gov/MedicalDevices /DeviceRegulationandGuidance /Overview/ClassifyYourDevice/default .htm. - FDA. Laboratory developed tests. 2018b. [January 11, 2019]. https://www
.fda.gov/medicaldevices /productsandmedicalprocedures /invitrodiagnostics /laboratorydevelopedtests/default.htm. - Federal Register. Standards for privacy of individually identifiable health information. 2003. (45 CFR part 160, part 164 subpart e. 68(34)).
- Haslem DS, Chakravarty I, Fulde G, Gilbert H, Tudor BP, Lin K, Ford JM, Nadauld LD. Precision oncology in advanced cancer patients improves overall survival with lower weekly healthcare costs. Oncotarget. 2018;9(15):12316–12322. [PMC free article: PMC5844748] [PubMed: 29552312]
- Horvat N, Veeraraghavan H, Khan M, Blazic I, Zheng J, Capanu M, Sala E, Garcia-Aguilar J, Gollub MJ, Petkovska I. MR imaging of rectal cancer: Radiomics analysis to assess treatment response after neoadjuvant therapy. Radiology. 2018;287(3):833–843. [PMC free article: PMC5978457] [PubMed: 29514017]
- IMDRF (International Medical Device Regulators Forum) SaMD (Software as a Medical Device) Working Group. Software as a medical device (SaMD): Key definitions. 2013. [January 11, 2019]. http://www
.imdrf.org /docs/imdrf/final/technical /imdrf-tech-131209-samd-key-definitions-140901.pdf. - IOM (Institute of Medicine). Evolution of translational omics: Lessons learned and the path forward. Washington, DC: The National Academies Press; 2012. [PubMed: 24872966]
- Jennings L, Deerlin VMV, Gulley ML. Recommended principles and practices for validating clinical molecular pathology tests. Archives of Pathology and Laboratory Medicine. 2009;133(5):743–755. [PubMed: 19415949]
- Joseph G, Pasick RJ, Schillinger D, Luce J, Guerra C, Cheng JKY. Information mismatch: Cancer risk counseling with diverse underserved patients. Journal of Genetic Counseling. 2017;26(5):1090–1104. [PMC free article: PMC5582075] [PubMed: 28289853]
- Kutner M, Greenberg E, Jin Y, Boyle B, Hsu Y, Dunleavy E. Literacy in Everyday Life: Results From the 2003 National Assessment of Adult Literacy (NCES 2007-480). U.S. Department of Education. Washington, DC: National Center for Education Statistics; 2007.
- Lashbrook A. AI-driven dermatology could leave dark-skinned patients behind. 2018. [January 9, 2019]. https://www
.theatlantic .com/health/archive /2018/08/machine-learning-dermatology-skin-color/567619. - Lautenbach DM, Christensen KD, Sparks JA, Green RC. Communicating genetic risk information for common disorders in the era of genomic medicine. Annual Review of Genomics and Human Genetics. 2013;14:491–513. [PMC free article: PMC3862080] [PubMed: 24003856]
- Le Tourneau C, Delord JP, Gonçalves A, Gavoille C, Dubot C, Isambert N, Campone M, Trédan O, Massiani MA, Mauborgne C, Armanet S. Molecularly targeted therapy based on tumour molecular profiling versus conventional therapy for advanced cancer (SHIVA): A multicentre, open-label, proof-of-concept, randomised, controlled phase 2 trial. The Lancet Oncology. 2015;16(13):1324–1334. [PubMed: 26342236]
- Lee J, Blumenthal GM, Hohl RJ, Huang SM. Cancer therapy: Shooting for the moon. Clinical Pharmacology and Therapeutics. 2017;101(5):552–558. [PMC free article: PMC5525193] [PubMed: 28418166]
- Lipkus IM, Samsa G, Rimer B. General performance on a numeracy scale among highly educated samples. Medical Decision Making. 2001;21(1):37–44. [PubMed: 11206945]
- Manrai AK, Funke BH, Rehm HL, Olesen MS, Maron BA, Szolovits P, Margulies DM, Loscalzo J, Kohane IS. Genetic misdiagnoses and the potential for health disparities. New England Journal of Medicine. 2016;375(7):655–665. [PMC free article: PMC5292722] [PubMed: 27532831]
- McCook A. Prominent NIH researcher up to a dozen retractions. 2017. [January 10, 2019]. https:
//retractionwatch .com/2017/03/16/prominent-nih-researcher-dozen-retractions. - McShane LM, Hayes DF. Publication of tumor marker research results: The necessity for complete and transparent reporting. Journal of Clinical Oncology. 2012;30(34):4223. [PMC free article: PMC3504327] [PubMed: 23071235]
- McShane LM, Cavenagh MM, Lively TG, Eberhard DA, Bigbee WL, Williams PM, Mesirov JP, Polley MYC, Kim KY, Tricoli JV, Taylor JM, Shuman DJ, Simon RM, Doroshow JH, Conley BA. Criteria for the use of omics-based predictors in clinical trials: Explanation and elaboration. BMC Medicine. 2013a;11(1):220. [PMC free article: PMC3852338] [PubMed: 24228635]
- McShane LM, Cavenagh MM, Lively TG, Eberhard DA, Bigbee WL, Williams PM, Mesirov JP, Polley MYC, Kim KY, Tricoli JV, Taylor JMG, Shuman DJ, Simon RM, Doroshow JH, Conley BA. Criteria for the use of omics-based predictors in clinical trials. Nature. 2013b;502:317. [PMC free article: PMC4180668] [PubMed: 24132288]
- Mello MM, Lieou V, Goodman SN. Clinical trial participants’ views of the risks and benefits of data sharing. BMC Medicine. 2018;378(23):2202–2211. [PMC free article: PMC6057615] [PubMed: 29874542]
- MIT (Massachusetts Institute of Technology). Health 0.0. 2019. [January 11, 2019]. https://www
.media.mit .edu/groups/health-0-0/overview. - NASEM (National Academies of Sciences, Engineering, and Medicine). Biomarker tests for molecularly targeted therapies: Key to unlocking precision medicine. Washington, DC: The National Academies Press; 2016. [PubMed: 26962610]
- NASEM. Improving cancer diagnosis and care: Patient access to oncologic imaging and pathology expertise and technologies: Proceedings of a workshop. Washington, DC: The National Academies Press; 2018. [PubMed: 30207656]
- OECD (Organisation for Economic Co-operation and Development). The OECD privacy framework. 2013. [January 11, 2019]. http://www
.oecd.org/sti /ieconomy/oecd_privacy_framework.pdf. - Oh SS, Galanter J, Thakur N, Pino-Yanes M, Barcelo NE, White MJ, de Bruin DM, Greenblatt RM, Bibbins-Domingo K, Wu AHB, Borrell LN, Gunter C, Powe NR, Burchard EG. Diversity in clinical and biomedical research: A promise yet to be fulfilled. PLoS Medicine. 2015;12(12):e1001918–e1001924. [PMC free article: PMC4679830] [PubMed: 26671224]
- Peng RD, Dominici F, Zeger SL. Reproducible epidemiologic research. American Journal of Epidemiology. 2006;163(9):783–789. [PubMed: 16510544]
- Presley CJ, Tang D, Soulos PR, Chiang AC, Longtine JA, Adelson KB, Herbst RS, Zhu W, Nussbaum NC, Sorg RA, Agarwala V, Abernethy AP, Gross CP. Association of broad-based genomic sequencing with survival among patients with advanced non-small–cell lung cancer in the community oncology setting. JAMA. 2018;320(5):469–477. [PMC free article: PMC6142984] [PubMed: 30088010]
- Radiological Society of North America. Quantitative Imaging Biomarkers Alliance. 2018. [January 11, 2019]. https://www
.rsna.org /en/research/quantitative-imaging-biomarkers-alliance. - Rampey BD, Finnegan R, Goodman M, Mohadjer L, Krenzke T, Hogan J, Provasnik S. Skills of U.S. unemployed, young, and older adults in sharper focus: Results from the Program for the International Assessment of Adult Competencies (PIAAC) 2012/2014: First look (NCES 2016-039rev). U.S. Department of Education. Washington, DC: National Center for Education Statistics; 2016. [April 18, 2019]. http://nces
.ed.gov/pubsearch. - Rana A, Yauney G, Lowe A, Shah P. Computational histological staining and destaining of prostate core biopsy RGB images with generative adversarial neural networks. 2018. [January 14, 2019]. https://arxiv
.org/abs/1811.02642. - The AACR Project GENIE Consortium. AACR Project GENIE: Powering precision medicine through an international consortium. Cancer Discovery. 2017;7(8):818–831. [PMC free article: PMC5611790] [PubMed: 28572459]
- U.S. Congress. H.R. 3103—Health Insurance Portability and Accountability Act of 1996. 1996. [January 11, 2019]. https://www
.congress .gov/bill/104th-congress/house-bill/3103. - WHO (World Health Organization). The mandate for health literacy. Paper presented at 9th Global Conference on Health Promotion. Shanghai, China: 2016.
- Wise J. Skin cancer: Smartphone diagnostic apps may offer false reassurance, warn dermatologists. BMJ. 2018;362:k2999. [PubMed: 29976751]
- Yauney G, Shah P. Reinforcement learning with action-derived rewards for chemotherapy and clinical trial dosing regimen selection. 2018. [January 14, 2019]. https://dam-prod
.media .mit.edu/x/2018/09 /06/MLHC%20paper-Pratik%20Shah.pdf.
Footnotes
- 1
The planning committee’s role was limited to planning the workshop, and the Proceedings of a Workshop was prepared by the workshop rapporteurs as a factual summary of what occurred at the workshop. Statements, recommendations, and opinions expressed are those of individual presenters and participants, and are not necessarily endorsed or verified by the National Academies of Sciences, Engineering, and Medicine, and they should not be construed as reflecting any group consensus.
- 2
See https://www
.nap.edu/catalog/25163 (accessed January 2, 2019). - 3
See http:
//nationalacademies .org/hmd/Activities /Disease/NCPF/2018-OCT-29.aspx (accessed January 2, 2019). - 4
In February 2019, Dr. Abernethy became Principal Deputy Commissioner of Food and Drugs at the Food and Drug Administration. The views expressed in this proceedings do not necessarily represent the official views or policies of FDA.
- 5
See https://www
.genomichealth .com/en-US/oncotype_iq_products /oncotype_dx /oncotype_dx_breast_cancer (accessed January 4, 2019). - 6
See https://www
.cdisc.org (accessed January 4, 2019). - 7
FDA has stated that it has statutory authority for the regulatory oversight of all tests used in patient care, but has used its enforcement discretion (meaning it has chosen to not exercise that authority) for the oversight of LDTs (NASEM, 2016).
- 8
FDA defines companion diagnostics as diagnostic devices that “provide information that is essential for the safe and effective use of a corresponding therapeutic product” (FDA, 2014).
- 9
See https://aspe
.hhs.gov /report/studies-welfare-populations-data-collection-and-research-issues /fair-information-practices (accessed January 7, 2019). - 10
The HIPAA Privacy Rule “establishes national standards to protect individuals’ medical records and other personal health information and applies to health places, health care clearinghouses, and those health care providers that conduct certain health care transactions electronically.” See https://www
.hhs.gov/hipaa /for-professionals/privacy/index .html (accessed March 29, 2019). - 11
Health literacy is defined as the degree to which individuals can access, comprehend, and use information and services needed to make appropriate health care decisions (WHO, 2016).
- 12
See https://www
.ohdsi.org /data-standardization /the-common-data-model (accessed January 8, 2019). - 13
See https://www
.hl7.org/fhir/overview.html (accessed January 8, 2019). - 14
See http://www
.hl7.org/about (accessed January 8, 2019). - 15
See https://www
.aacr.org /Research/Research/pages /aacr-project-genie.aspx (accessed January 8, 2019). - 16
See https:
//joincountmein.org (accessed January 8, 2019). - 17
See https://www
.mskcc.org /research-programs/molecular-oncology /make-impact (accessed January 8, 2019). - 18
See https://www
.clinicalgenome.org/about (accessed January 8, 2019). - 19
See https://www
.ncbi.nlm .nih.gov/clinvar/intro (accessed January 8, 2019). - 20
See http://www
.ukbiobank .ac.uk/about-biobank-uk (accessed January 8, 2019). - 21
See https://bluebutton
.cms.gov (accessed January 8, 2019). - 22
See https://www
.healthit.gov/topic/about-onc (accessed January 8, 2019).
- Proceedings of a Workshop - Improving Cancer Diagnosis and CareProceedings of a Workshop - Improving Cancer Diagnosis and Care
Your browsing activity is empty.
Activity recording is turned off.
See more...