NCBI Bookshelf. A service of the National Library of Medicine, National Institutes of Health.
Committee on the Learning Health Care System in America; Institute of Medicine; Smith M, Saunders R, Stuckhardt L, et al., editors. Best Care at Lower Cost: The Path to Continuously Learning Health Care in America. Washington (DC): National Academies Press (US); 2013 May 10.
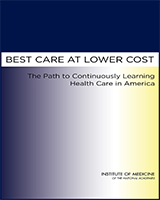
Best Care at Lower Cost: The Path to Continuously Learning Health Care in America.
Show detailsIn 2008, Ann Morrison received two all-metal hip replacements at the age of 50. Soon after the procedure, she experienced intense rashes, pain, and inflammation at the sites of her surgery. The injurious devices were replaced in 2010, just 2 years after she received her initial hip replacements; hip replacements typically last 15 years or more. Today, as a result of extensive tissue damage caused by metal debris shed from the original replacements, Ann requires a brace to walk, and she still has not been able to return to her work as a physical therapist. With the proper digital infrastructure—electronic health records, the use of clinical data to compare the effectiveness and efficiency of different interventions, and registries to track side effects and safety—Ann's experience could have been avoided. Instead, the U.S. health care system currently lacks the data, monitoring, and analysis capabilities necessary to effectively evaluate, disseminate, and implement the ever-increasing amount of health information and technologies (Meier and Roberts, 2011).
Although an unprecedented amount of information is available in journals, guidelines, and other sources, patients and clinicians often lack access to information they can feel confident is relevant, timely, and useful for the circumstances at hand. Moreover, the current system for disseminating knowledge is strained by the quantity of information now available, which means that new evidence often is not applied to care. After explaining the need for a new approach to generating clinical and biomedical knowledge, this chapter describes emerging capacities, methods, and approaches that hold promise for helping to meet this need. It then examines what is necessary to create the data utility that will be essential to a continuously learning and improving health care system. Next, the critical issue of building a learning bridge from knowledge to practice is explored. This is followed by a discussion of the crucial role of people, patients, and consumers as active stakeholders in the learning enterprise. The chapter concludes with recommendations for achieving the vision of a health care system that generates and applies knowledge in real time.
NEED FOR A NEW APPROACH TO KNOWLEDGE GENERATION
The current approach to generating new medical knowledge falls short in delivering the evidence needed to support the delivery of quality care. The evidence base is inadequate, and methods for generating medical knowledge have notable limitations.
Inadequacy of the Evidence Base
Clinical and biomedical research emerges at a remarkable rate, with almost 2,100 scientific publications, 75 clinical trials, and 11 systematic reviews being produced every day (Bastian et al., 2010).1 Although clinicians need not review every study to provide high-quality care, the ever-increasing volume of evidence makes it difficult to maintain a working knowledge of new clinical information.
Even so, however, the availability of such high-quality evidence is not keeping pace with the ever-increasing demand for clinical information that can help guide decisions on different diagnostics, interventions and therapies, and care delivery approaches (see Box 6-1 for an example of this information paradox). Rather, the gap between the evidence possible and the evidence produced continues to grow, and studies indicate that the number of guideline statements backed by evidence is not at the level that should be expected. In some cases, 40 to 50 percent of the recommendations made in guidelines are based on expert opinion, case studies, or standards of care rather than on multiple clinical trials or meta-analyses (Chauhan et al., 2006; IOM, 2008, 2011b; Tricoci et al., 2009). A study of the strength of the current recommendations of the Infectious Diseases Society of America, for example, found that only 14 percent were based on more than one randomized controlled trial, and more than half were based on expert opinion alone (Lee and Vielemeyer, 2011). Another study, examining the joint cardiovascular clinical practice guidelines of the American College of Cardiology and the American Heart Association, found that the current guidelines were based largely on lower levels of evidence or expert opinion (Tricoci et al., 2009).
The inadequacy of the evidence base for clinical guidelines has consequences for the evidence base for care delivered. Estimates vary on the proportion of clinical decisions in the United States that are adequately informed by formal evidence gained from clinical research, with some studies suggesting a figure of just 10–20 percent (Darst et al., 2010; IOM, 1985). These results suggest that there are substantial opportunities for improvement in ensuring that the knowledge generated by the clinical research enterprise meets the demands of evidence-based care.
Even after identifying relevant information for a given condition, clinicians still must ensure that the information is of high quality—that the risk of contradiction by later studies is minimal, that the information is uncolored by bias or conflicts of interest, and that it applies to a particular patient's clinical circumstances. Several recent publications have observed that the rate of medical reversals is significant, with one recent paper finding that 13 percent of articles about medical practice in a high-profile journal contradicted the evidence for existing practices (Ioannidis, 2005b; Prasad et al., 2011). Another concern is managing conflicts of interest—which can occur in the research, education, and practice domains. As noted in the 2009 Institute of Medicine (IOM) report Conflict of Interest in Medical Research, Education, and Practice, patients can benefit when clinicians and researchers collaborate with the life science industry to develop new products, yet there are concerns that financial ties could unduly influence professional judgments. These tensions must be balanced to ensure that conflicts of interest do not negatively impact the integrity of the scientific research process, the objectivity of health professionals' training and education, or the public's trust in health care. There are approaches to managing conflicts of interest, especially financial relationships, without stifling important collaborations and innovations (IOM, 2009b).
Concerns exist as well about whether the current evidence base applies to the circumstances of particular patients. A study of clinical practice guidelines for nine of the most common chronic conditions, for example, found that fewer than half included guidance for the treatment of older patients with multiple comorbid conditions (Boyd et al., 2005). For patients and their health care providers, this lack of knowledge limits the ability to choose the most effective treatment for a condition. Furthermore, health care payers may not have the evidence they need to make coverage decisions for the patients enrolled in their plans. One analysis of Medicare payment policies for cardiovascular devices, for example, found that participants in the trials that provided evidence for coverage decisions differed from the Medicare population. Participants in the trials often were younger and healthier and had a different prevalence of comorbid conditions (Dhruva and Redberg, 2008).
Further, without greater capacity, the challenges to evidence production will only continue to grow. This is particularly true given the projected proliferation of new medical technologies; the increased complexity of managing chronic diseases; and the growing use of genomics, proteomics, and other biological factors to personalize treatments and diagnostics (Califf, 2004). As noted in Chapter 2, in one 3-year period, genome-wide scans were able to identify more than 100 genetic variants associated with nearly 40 diseases and traits; this growth in genetic understanding led to the availability in 2008 of more than 1,200 genetic tests for clinical conditions (Genetics and Public Policy Center, 2008; Manolio, 2010; Pearson and Manolio, 2008).
Even as clinical research strains to keep pace with the rapid evolution of medical interventions and care delivery methods, improving and increasing the supply of knowledge with which to answer health care questions is a core aim of a learning health care system. The current research knowledge base provides limited support for answering important types of clinical questions, including those related to comparative effectiveness and long-term patient outcomes (British Medical Journal, 2011; Gill et al., 1996; IOM, 1985; Lee et al., 2005a; Tunis et al., 2003). This lack of knowledge is demonstrated by the fact that many technologies are not adequately evaluated before they see widespread clinical use. For example, cardiac computed tomography angiography (CTA) has been adopted widely throughout the medical community despite limited data on its effectiveness compared with alternative interventions, the risks of its use, and its substantial cost (Redberg, 2007). New opportunities in technology and research design can mitigate these limitations and offer a dynamic view of evidence and outcomes; leveraging these opportunities can bridge the gap between research and practice to accelerate the use of research in routine care.
Limitations of Current Methods
At present, support for clinical research often focuses on the randomized controlled trial as the gold standard for testing the effectiveness of diagnostics and therapeutics. The randomized controlled trial has gained this status because of its ability to control for many confounding factors and to provide direct evidence on the efficacy of different treatments, interventions, and care delivery methods (Hennekens et al., 1987). Yet, while the randomized controlled trial has a highly successful track record in generating new clinical knowledge, it has, like most research methods available today, several limitations: such trials are not practical or feasible in all situations, are expensive and time-consuming, address only the questions they were designed to answer, and cannot answer every type of research question.
A study of head-to-head randomized controlled trials for comparative effectiveness research purposes found that their costs ranged from $400,000 to $125 million, with the average costs for larger studies averaging $15-$20 million (Holve and Pittman, 2009, 2011). Randomized controlled trials also are slow to address the research questions they set out to answer. Half of all trials are delayed, 80 to 90 percent of these because of a shortage of willing trial participants (Grove, 2011). As currently designed and operated, moreover, randomized controlled trials do not address all clinically relevant populations, which may limit a trial's generalizability to regular clinical practice and many patient populations (Frangakis, 2009; Greenhouse et al., 2008; Stewart et al., 2007; Weisberg et al., 2009). At a time when many patients have multiple chronic conditions (Alecxih et al., 2010; Tinetti and Studenski, 2011), for example, patients with comorbidities are routinely excluded from most randomized controlled trials (Dhruva and Redberg, 2008; Van Spall et al., 2007). In addition, many current trials collect data only for a limited period of time, which means they may not capture long-term effects or low-probability side effects and may not reflect the practice conditions of many health care providers.
Other research methods have limitations as well. For instance, the strength of observational studies is that they capture health practices in real-world situations, which aids in generalizing their results to more medical practices. This research design can provide data throughout a product's life cycle and allow for natural experiments provided by variations in care. However, observational studies are challenged to minimize bias and ensure that their results were due to the intervention under consideration. For this reason, as demonstrated by the use of hormone replacement therapy (see Box 6-2) and Vitamin E for the treatment of coronary disease, results of observational trials do not always accord with those of randomized controlled trials (Lee et al., 2005b; Rossouw et al., 2002), although some studies have shown concordance between the results derived from the two methods (Concato et al., 2000).
The challenge, therefore, is not determining which research method is the best for a particular condition but rather which provides the information most appropriate to a particular clinical need. Table 6-1 summarizes different research designs and the questions most appropriately addressed by each. In the case of examining biomedical treatments and diagnostic technologies, different types of studies will be more appropriate for different stages of a product's life cycle. Early studies will need to focus on safety and efficacy, which will require randomized controlled trials, while later studies will need to focus on comparative effectiveness and surveillance of unexpected effects, requiring a mix of observational studies and randomized controlled trials. (See Figure 6-1 for a depiction of the change in appropriate research methods over time.) As this report was being written, the methodology committee of the Patient-Centered Outcomes Research Institute (PCORI) had developed a translation table to aid in determining the research methods most appropriate for addressing certain comparative clinical effectiveness research questions (PCORI, 2012). Each study must be tailored to provide useful, practical, and reliable results for the condition at hand.
TABLE 6-1
Examples of Research Methods and Questions Addressed by Each.

FIGURE 6-1
Different types of research are needed at different stages of a medical product's life cycle. Early trials will need to focus on therapeutic efficacy, while later research will need to focus on comparative effectiveness and surveillance. SOURCE: Adapted (more...)
Conclusion 6-1: Despite the accelerating pace of scientific discovery, the current clinical research enterprise does not sufficiently address pressing clinical questions. The result is decisions by both patients and clinicians that are inadequately informed by evidence.
Related findings:
Clinical and biomedical research studies are being produced at an increasing rate. As noted in the findings supporting Conclusion 2-1, on average approximately 75 clinical trials and a dozen systematic reviews are published daily (see Chapter 2). The evidence basis for clinical guidelines and recommendations needs to be strengthened. In some cases, 40 to 50 percent of the recommendations made in guidelines are based on expert opinion, case studies, or standards of care rather than on multiple clinical trials or meta-analyses. Even at the current pace of production, the knowledge base provides limited support for answering many of the most important types of clinical questions. A study of clinical practice guidelines for nine of the most common chronic conditions found that fewer than half included guidance for the treatment of patients with multiple comorbid conditions. New methods are needed to address current limitations in clinical research. The cost of current methods for clinical research averages $15-$20 million for larger studies—and much more for some—yet the studies do not reflect the practice conditions of many health care providers.
EMERGING CAPACITIES, METHODS, AND APPROACHES
As discussed above, there is a clear need for new approaches to knowledge generation, management, and application to guide clinical care, quality improvement, and delivery system organization. The current clinical research enterprise requires substantial resources and takes significant time to address individual research questions. Moreover, the results provided by these studies do not always generate the information needed by patients and their clinicians and may not always be generalizable to a larger population. New research methods are needed that address these serious limitations. Developments in information technology and research infrastructure have the potential to expand the ability of the research system to meet this need. For example, the anticipated growth in the adoption of digital records presents an unprecedented opportunity to expand the supply of data available for learning, generating insights from the regular delivery of care (see the discussion of the data utility in the next section for further detail on these opportunities). These new developments can increase the output derived from the substantial clinical research investments of agencies and foundations, including the Agency for Healthcare Research and Quality (AHRQ), the National Institutes of Health (NIH), and PCORI.
New tools are extending research methods and overcoming many of the limitations highlighted in the previous section (IOM, 2010a). The scientific community has recognized the need for change. High-profile efforts—including NIH's Clinical and Translational Science Awards and the U.S. Food and Drug Administration's Clinical Trials Transformation Initiative—have been undertaken to improve the quality, efficiency, and applicability of clinical trials, and new translational research paradigms have been developed (Lauer and Skarlatos, 2010; Luce et al., 2009; Woolf, 2008; Zerhouni, 2005). Based on these efforts and the work of academic research leaders, new forms of experimental designs have been developed, including pragmatic clinical trials, delayed design trials, and cluster randomized controlled trials2 (Campbell et al., 2007; Eldridge et al., 2008; Tunis et al., 2003, 2010). Other new methods have been devised to develop knowledge from data produced during the regular course of care. Initial results derived with these new methods have shown promise (see Box 6-3 for a description of one new method). Advanced statistical methods, including Bayesian analysis, allow for adaptive research designs that can learn as a study advances, making studies more flexible (Chow and Chang, 2008). Taken together, these new methods are designed to reduce the expense and effort of conducting research, improve the applicability of the results to clinical decisions, improve the ability to identify smaller effects, and be applied when traditional methods cannot be used.
In addition to new research methods, advances in statistical analysis, simulation, and modeling have supplemented traditional methods for conducting trials. Given that even the most tightly controlled trials show a distribution in patient responses to a given treatment or intervention, new statistical techniques can help segment results for different populations. Further, new Bayesian techniques for data analysis can separate out the effects of different clinical interventions on overall population health (Berry et al., 2006). With the growth in computational power, new models have been developed that can replicate physiological pathways and disease states (Eddy and Schlessinger, 2003; Stern et al., 2008). These models can then be used to simulate clinical trials and individualize clinical guidelines to a patient's particular situation and biology; this approach thus holds promise for improving health status while reducing costs (Eddy et al., 2011). As computational power grows, the potential applications of these simulation and modeling tools will continue to increase. Despite the opportunities afforded by new research methods, several challenges must be addressed as these methods are improved. One such challenge for the clinical research enterprise is keeping pace with the introduction of new procedures, treatments, diagnostic technologies, and care delivery models. As currently structured, clinical trials often are not comparable, so that a new trial must be conducted to compare the effectiveness of new treatments, diagnostics, or care delivery models with that of existing ones. One solution to this problem is to create standard comparators for a given disease or clinical condition, which would allow new innovations to be compared easily using existing data for current treatments or diagnostic technologies. Additionally, as the research enterprise is expanded, additional emphasis may be required in fields that are underserved by the current clinical research paradigm, such as pediatrics (Cohen et al., 2007; IOM, 2009c; Simpson et al., 2010). One exception to this observation is pediatric cancer care. Virtually all of the treatment provided in pediatric oncology is recorded and applied to registries or active clinical trials, which then inform future care for children undergoing treatment (IOM, 2010b; Pawlson, 2010).
CREATION OF THE DATA UTILITY
In considering how to take advantage of opportunities to create a more nimble, timely, and targeted clinical research enterprise, three basic questions should be considered: (1) What does the system need to know? (2) How will the information be captured and used? and (3) How will the resulting knowledge be organized and shared? These questions have important ramifications for the design and operation of the overall data system.
With respect to the first question, stakeholders in the health care system are interested in comparing the effectiveness of different treatments and interventions, monitoring the current safety of medical products through surveillance, undertaking quality improvement activities, and understanding the quality and performance of different providers and health care organizations. Achieving these goals will require capturing data on the care that is delivered to patients, such as processes and structures of care delivery, and the outcomes of that care, such as longitudinal health outcomes and other outcomes important to patients. With respect to how these data will be used to generate new health care knowledge, uses will include comparing the effects of different treatments, interventions, or care protocols; establishing guidelines and best practices; and searching for unexpected effects of treatments or interventions. Finally, the new knowledge generated will have little impact if not shared broadly with all involved in delivering care for a given patient or, for research cases, all those involved in research. Each of these three questions is explored in further detail below.
What Does the System Need to Know?
Data on how patients respond to diagnostic technologies, treatments, interventions, or care delivery methods are the raw material for generating new clinical knowledge. However, gathering this raw material currently requires significant effort through specialized research protocols. Substantial quantities of clinical data are generated every day in the regular process of care. Unfortunately, most of this information remains locked inside paper records, which are difficult to access, transfer, and query. As of 2011, only about 34–35 percent of office-based physicians were using a basic electronic health record (EHR) system (Decker et al., 2012; Hsiao et al., 2011), while only 18 percent of hospitals had a basic system (DesRoches et al., 2012).
The anticipated growth in the adoption of digital records presents an unprecedented opportunity to improve the supply of data available for learning, particularly as data sources are designed to capture information generated during the delivery of care. Examples of such sources include larger clinical and administrative databases, clinical registries, personal electronic devices (such as smartphones and mobile sensors), clinical trials for regulatory purposes (such as new drug applications), and advanced EHR systems. New sources for data capture are fueled in part by the infusion of capital provided by the Health Information Technology for Economic and Clinical Health (HITECH) Act,3 which included financial incentives for the meaningful use of EHR systems. Just as the information revolution has transformed many other fields, growing stores of data hold the same promise for improving clinical research, clinical practice, and clinical decision making.
Health care providers play a critical role in supplying clinical data for research and ensuring the quality of the data. To achieve strong provider participation in the learning enterprise, data capture must be seamlessly integrated into providers' daily workflow and must not disrupt the clinical routine. In addition, professional and specialty societies might be engaged to increase the number of providers willing to participate in the clinical research enterprise. Finally, aligning financial incentives and reimbursement can encourage providers and health care organizations to gather, store, and manage clinical data. Currently, many individuals and organizations donate their time when collecting data for research, which limits the amount of effort they can expend on these initiatives. Specific incentives for generating clinical data could increase the supply of data available for research and the quality of the overall enterprise.
How Will the Information Be Captured and Used?
New sources of health care data, combined with existing resources, offer unprecedented opportunities to learn from health care delivery and patient care. These sources include, for example, EHR systems; registries on diseases, treatments, or specific populations; claims databases from insurers and payers; and mobile devices and sensors that capture local data. In addition to the capacity these sources bring to the collection of clinical data, they also support clinical effectiveness research; surveillance for safety, public health, and other purposes; quality improvement initiatives; population health management; cost and quality reporting; and tools for patient education.
As noted above, EHR systems provide a substantial opportunity for learning by unlocking information currently stored in paper medical records. For example, one study found that real-time analysis of clinical data from EHRs could have identified the increased risk of heart attack associated with rosiglitazone, a diabetes drug, within 18 months of its introduction, as opposed to the 7–8 years between the medication's introduction and when concerns were raised publicly (Brownstein et al., 2010). In considering how to maximize the clinical knowledge gained from EHR systems, a tension exists between the data needs of research studies and the resources required to collect and store clinical data on care processes and patient outcomes. Given the range of health care research studies, it is likely to be infeasible for every system to capture the full amount of data needed to fulfill all potential research needs. A compromise solution to this tension is to identify those core pieces of information that are needed for many research questions and ensure that this limited set of information is captured faithfully by most digital health record systems. This method of identifying a core dataset that satisfies both research and clinical care needs has been used by several organizations. For example, the National Quality Forum's (NQF's) Quality Data Model defines a set of standardized clinical and administrative data that are needed to calculate quality measures using information from EHRs (National Quality Forum, 2010), while the HMO Research Network's Virtual Data Warehouse (discussed further on page 165) maps data from the EHRs and medical claims of multiple health maintenance organization (HMO) plans into a standardized dataset. Other efforts focus on population health; for example, popHealth software integrates with providers' EHRs to automate and simplify the reporting and exchange of quality data on the providers' patient populations, and the Query Health project is setting data standards to enable research on population health (Fridsma, 2011; popHealth, 2012). In addition to the research benefits, routine adoption of core datasets in EHRs can enhance the capacity for exchange of consistent health information across systems and organizations, thereby supporting improved coordination of health services.
As EHR systems become more widespread, it will be necessary to provide flexibility to address new and unforeseen research questions. The sheer scale and complexity of the digital utility, its use by a variety of individuals with conflicting needs, and its constant evolution will require new ways to set standards, develop applications, and interact with the users of clinical data. One technological solution is to ensure that these digital systems are designed in the modular fashion popular in other industries, as with smartphone applications and computer software. This modular approach could also provide additional capacity for meeting new research needs without necessitating an overhaul of the central structure of the digital system.
Registries, which are distinguished by their focus on a specific disease, procedure, treatment, intervention, or resource use, are another important tool for developing new knowledge (Robert Wood Johnson Foundation, 2010) (see Box 6-4). A registry collects uniform clinical data using observational methods to evaluate specified outcomes for a specific population and for a specific purpose (AHRQ, 2010). By collecting detailed data not contained in other sources, registries have been able to determine the clinical effectiveness of a variety of health care interventions and treatments (Akhter et al., 2009; Grover et al., 2001; Meadows et al., 2009; Savage et al., 2003). Further, the clinical and financial payoffs of this method of aggregating and generating knowledge can be substantial.
In addition to EHRs and registries, mobile technologies for providers and patients will play an increasingly important role in capturing and storing health care data. These technologies include a wide range of patient-focused devices that monitor patient health, with the potential to support improved diagnosis or treatment. Provider-focused tools include applications that are built into existing personal digital assistants, smartphones, and tablet computers to store patient health information or access clinical databases. According to industry reports, global sales of these portable devices for health care uses reached $8.2 billion in 2009, and growth of up to 7 percent per year is projected for the next 5 years (Kalorama Information, 2010).
Conclusion 6-2: Growing computational capabilities to generate, communicate, and apply new knowledge create the potential to build a clinical data infrastructure to support continuous learning and improvement in health care.
Related findings:
The application of computing capacity and new analytic approaches enables the development of real-time research insights from existing patient populations. One study found that real-time analysis of clinical data from electronic health records could have identified the increased risk of heart attack associated with rosiglitazone, a diabetes drug, within 18 months of its introduction. Computational capabilities offer the prospect of speeding the delivery of important new insights from the care experience. For example, a comprehensive disease registry in Sweden has helped facilitate a 65 percent reduction in 30-day mortality and a 49 percent decrease in 1-year mortality for heart attack patients. Computational capabilities present promising, as yet unrealized, opportunities for care improvement. For example, mining data on patient outcomes and care processes at Intermountain's LDS Hospital allows for continuous improvement of clinical practice guidelines. Implementation of an improved guideline for acute respiratory distress syndrome increased patient survival from 9.5 percent to 44 percent (see Chapter 9).
How Will Knowledge Be Organized and Shared?
Although each individual data source presents an opportunity for learning, the capacity for learning increases exponentially when the system can draw knowledge from multiple sources. Expanding the ability to share data requires developing technological solutions, building a data sharing culture, and addressing privacy and security concerns. Nevertheless, several organizations have successfully overcome these hurdles and implemented large-scale data sharing. Examples include large health care delivery organizations with extensive EHR capabilities, such as Kaiser Permanente and the Veterans Health Administration, and major initiatives in data sharing between different organizations, such as the Nationwide Health Information Network, the Care Connectivity Consortium, the Shared Health Research Information Network, and Informatics for Integrating Biology and the Bedside (i2b2) (Kuperman, 2011; Lohr, 2011; Murphy et al., 2010; Weber et al., 2009).
The technological aspects of sharing depend on the sources of the data. For EHR systems, sharing is complicated by the fact that there is a variety of EHR systems, each of which stores data using different methods and in different formats (Detmer, 2003). An additional complication is the inevitability of systems of different ages being in use, some that incorporate newer technologies and others that are legacy systems. Overcoming these barriers will require several technological solutions, such as interoperability strategies; methods for highlighting the quality of the data; and ways to identify the source, context, and provenance of the data (IOM, 2011c). The challenge to sharing between registries and EHRs is that many registries were developed before EHRs existed, so that in most cases, the two are not interoperable (Physician Consortium for Performance Improvement, 2011). However, improved sharing of data from EHRs may provide a new means of populating registries. One additional technological and policy hurdle is the difficulty of linking records for the same patient across multiple data sources, as different methods (from statistical linkages to unique patient identifiers) strike different balances between the desire for research accuracy and concerns about the privacy of health information (Detmer, 2003).
One method for sharing data securely and efficiently is through distributed data networks. In this design, each organization in the network stores its information locally, often in a common format. When a researcher seeks to answer a specific research question, the organizations execute identical computer programs that analyze the organizations' own data, create a summary of the results for each site, and share those summaries with the entire network. The advantage of this approach is that the institutions share only deidentified summary data instead of patient records. (See Box 6-5 for a description of one distributed data network, the Virtual Data Warehouse of the HMO Research Network, alluded to earlier.) Other models that could be used to share data include centralized databases, whereby data are submitted to and accessed at one central source, and alternative distributed designs, whereby clinical data are shared directly between different institutions (Brown et al., 2010).
While the above technical considerations are important, problems associated with data ownership may pose a greater challenge to the sharing and exchange of information (Blumenthal, 2006; Let data speak to data, 2005; Piwowar et al., 2008). Researchers have invested significant energy and resources in collecting data and thus may be hesitant to share the data freely with others. Clinical data may be viewed as a proprietary good that belongs to its owner, rather than a societal good that can benefit the population at large. Overcoming this barrier will require a shift toward research and organizational cultures that value open sharing of data. This culture change will in turn require efforts on the part of organizational and national leadership, recognition and rewards for data sharing, and education of researchers in the potential benefits of data sharing (Piwowar et al., 2008).
Significant testimony as to the importance of patient and public engagement, support, and demand for the use of clinical data to produce new knowledge is offered by the misinterpretation of the privacy rule of the Health Insurance Portability and Accountability Act (HIPAA), which led to restricted use of data for new insights. Privacy is a highly important societal and personal value, but the current formulation and interpretation of this rule not only offer limited protection to patients, but also may impede the broader health research enterprise (IOM, 2009a). In a 2007 survey, 68 percent of researchers reported that the HIPAA privacy rule had made research more difficult (Ness, 2007). The impediments arise from both actual and perceived barriers to data sharing attributed to the law and its associated regulations. In surveys, approximately half of health researchers have reported that HIPAA regulations have decreased recruitment of research participants; 80–90 percent have indicated that the regulations have increased research costs; and 50–80 percent have said they have increased the time needed to conduct research and noted that different institutional interpretations of the law and its associated regulations have impeded collaboration (Association of Academic Health Centers, 2008; Goss et al., 2009; Greene et al., 2006; IOM, 2009a; Ness, 2007). As suggested in the IOM report Beyond the HIPAA Privacy Rule, solving these problems will likely require a reformulation of the rule, as well as improved guidance to limit disparities in its interpretation (IOM, 2009a).
Conclusion 6-3: Regulations governing the collection and use of clinical data often create unnecessary and unintended barriers to the effectiveness and improvement of care and the derivation of research insights.
Related findings:
Implementation of current regulations promulgated to improve privacy offers limited protection to patients and may impede the broader health research enterprise. In a 2007 survey, 68 percent of researchers reported that the HIPAA privacy rule had made research more difficult. Current regulations have made it difficult to recruit research participants, increased the cost and time needed to conduct research, and impeded collaboration. In surveys of researchers, approximately half have indicated that HIPAA regulations have decreased recruitment of research participants; 80–90 percent have indicated that the regulations have increased research costs; and 50–80 percent have said they have increased the time needed to conduct research.
THE LEARNING BRIDGE: FROM KNOWLEDGE TO PRACTICE
Unless the products of the nation's clinical data utility and research enterprise are disseminated and applied in practice, their results are meaningless. Current systems that generate and implement new clinical knowledge are largely disconnected and poorly coordinated. While clinical data contribute to the development of many effective, evidence-based practices, therapeutics, and interventions every year, only some of these become widely used. Many others are used only in limited ways, failing to realize their transformative potential to improve care (IOM, 2011a).
Historically, research discoveries in health care have been disseminated through the publication of study results, typically in medical journals. Clinicians are expected to set aside time to read these published results, consider how to integrate them into their practice, and change their behavior accordingly. As noted earlier in this chapter, the extraordinary number of journal articles outstrips any clinician's ability to read and process the information. Even if a clinician could read all of this information, its growth is rapidly outstripping human cognitive capacity to integrate the full body of literature when considering a specific clinical situation and a specific patient. As noted in Chapter 2, this growth in complexity can hamper a clinician's ability to make decisions. Moreover, clinicians' patterns for seeking out information have changed. Fully 86 percent of physicians now use the Internet to gather health, medical, or prescription drug information (Dolan, 2010). Of these physicians, 71 percent use a search engine to start their search for information. This change in information-seeking behavior has consequences for how medical information can be organized and publicized in a way that maximizes its chances of being implemented in clinical practice.
Unfortunately, evidence suggests that simply providing information, albeit more quickly, rarely changes clinical practice (Avorn and Fischer, 2010; Schectman et al., 2003). Multiple reasons may explain this situation. Sometimes, clinicians fail to change their behavior because they are unaware that new knowledge exists. Sometimes they may disagree that a research discovery would improve care for their patients. At other times, they do not perceive a great enough benefit to outweigh the burden of changing established practices (Cabana et al., 1999).
The challenge, therefore, is how to diffuse knowledge in ways that facilitate uptake by clinicians (McCannon and Perla, 2009). Many approaches currently are used to disseminate knowledge throughout the health care system, and these could be leveraged to increase the rate at which knowledge is disseminated. A further challenge is to disseminate knowledge that is useful for the clinical decisions faced by individual patients. To this end, traditional dissemination methods must be modified so that general research knowledge is adapted to the particular circumstances faced by each patient. While logistically demanding, this adaptation holds promise for improving the effectiveness and value of care while meeting the aim of improved patient-centeredness.
One technological tool for bringing research results into the clinical arena is clinical decision support. A clinical decision support system integrates information on a patient with a computerized database of clinical research findings and clinical guidelines. The system generates patient-specific recommendations that guide clinicians and patients in making clinical decisions (IOM, 2001a). One study, for example, found that digital decision support tools helped clinicians apply clinical guidelines, improving health outcomes for diabetics by 15 percent (Cebul et al., 2011). Tools under development may tailor the information to the individual patient, allowing the clinician to predict how an intervention would affect that patient. Further enhancing clinicians' predictive capacities are advanced informatics and simulation systems that can use data to model likely outcomes for similar patients receiving various treatments or supportive services. Clinical decision support systems also can help address cognitive errors (as discussed in Chapter 2), such as attribution, availability bias, and anchoring,4 all of which may contribute to errors and wrong diagnoses (Blue Cross Blue Shield of Massachusetts Foundation, 2007). Greater adoption of clinical decision support could be achieved through advances in interoperability with EHR and computerized physician order entry (CPOE) systems from multiple vendors, allowing this technology to be embedded seamlessly in the standard clinical workflow (Sittig et al., 2008; Wright and Sittig, 2008).
Regardless of the channels used to distribute new clinical knowledge, the clinical research system needs to account for the many factors that promote (or inhibit) the use of this knowledge. These factors will vary in their importance according to different types of clinicians, health care organizations, geographic locations, patient populations, and other factors. In general, the dissemination of a research discovery is dependent on three broad categories of factors: attributes of the discovery, characteristics of the potentially adopting clinician or health care organization, and environmental factors. Figure 6-2 illustrates these factors and their relationships. As depicted, the process of diffusion and scale-up is messy, organic, and dynamic. An individual or organization does not move linearly from research to development to implementation, but rather moves between these stages based on perceived needs and individual concerns (Greenhalgh et al., 2004).

FIGURE 6-2
Multiple factors affect whether new clinical knowledge is disseminated and implemented across the health care system.
The most obvious factor affecting the dissemination of a research discovery is its relative advantage over other competing interventions, therapeutics, or practices (Berwick, 2003; Cain and Mittman, 2002; Della Penna et al., 2009). Simply put, people are more likely to implement a new idea if they believe it can help them with a problem. In a health care context, this relative advantage could take multiple forms, from improved clinical effectiveness over existing treatments, to convenience in delivering the intervention, to reduced cost. While relative advantage is an important factor, other characteristics of a research discovery also have been found to be important, including whether the discovery's results can be observed easily and quickly, whether a potential adopter can try it without committing to it, its perceived complexity, and its ability to be modified to fit local circumstances (Rogers, 2003; Shih and Berliner, 2008; Vos et al., 2010). Many of these factors are not objective measures, but are based on the perceptions of potential adopters. This means the factors change based on the setting, the potential adopter, and time (Berwick, 2003; Greenhalgh et al., 2004).
A related cluster of factors that affect the dissemination of a research discovery encompasses the characteristics of the potentially adopting clinician. Evidence from adult learning reveals that clinicians' previous experiences and knowledge will affect their learning about new ideas and practices (Committee on Developments in the Science of Learning et al., 2000). In a related way, the dissemination of a research discovery will depend on individual clinicians' values and culture, as well as their inclination to experiment with new ideas (Bate et al., 2004; Berwick, 2003; Green and Plsek, 2002). For instance, some individuals are more willing to try new ideas, while others favor traditional methods. Dissemination will also depend on the clinician's social networks and those networks' views of the knowledge, practice, or technology (Cain and Mittman, 2002; Dopson et al., 2002; McCullough, 2008; Shih and Berliner, 2008).
This cluster of factors changes when the potential adopter is an organization instead of a clinician. For potentially adopting hospitals and health care organizations, dissemination will vary based on the type of hospital and its resources, especially whether it has resources available for implementing new ideas (McCullough, 2008). Specific capabilities that promote the adoption of new ideas are the support of the organization's leadership and management, the existence of robust channels for sharing knowledge, and the presence of structures that can discover potentially beneficial ideas from outside of the organization (Della Penna et al., 2009; Ferlie and Shortell, 2001; Green and Plsek, 2002; Nolan et al., 2005; Norton and Mittman, 2010; Pisano et al., 2001).
Finally, environmental factors that are distinct from the previous two clusters affect the dissemination of research discoveries. Financial incentives, reimbursement, the insurance environment, and regulations all impact whether an idea is adopted (Cutler et al., 1996; Mandel, 2010; McCullough, 2008; Robinson et al., 2009; Shih and Berliner, 2008). As with the previous clusters, these factors are not absolutes, but will vary depending on the specific discovery.
The strategy used to communicate a discovery is a particularly important environmental factor. Some successful strategies have involved using in-person educational methods, providing feedback on the process, employing opinion leaders or developing champions, or outlining an overall vision (Davis and TaylorVaisey, 1997; Flodgren et al., 2011; McCannon et al., 2006; O'Connor et al., 1996; Schectman et al., 2003; Soumerai et al., 1998). Another successful communication strategy is the creation of learning or improvement networks (Podolny and Page, 1998). Such networks provide a structure for the exchange of information and include those individuals necessary for the implementation of change on a larger scale (Carnegie Foundation for the Advancement of Teaching, 2010). This type of tool may be useful for managing the high degree of variation across the health care field, because information can be shared about how to customize guidelines, practice patterns, and other knowledge to fit local conditions (McCannon and Perla, 2009). Finally, reporting of data on performance and practice variation can spur the adoption of evidence-based practices (see Chapter 8 for a discussion of the use of reporting).
The complex interplay of the above factors is illustrated by a case study on disseminating a change across a large organization. In 2005, a large, integrated health care delivery system concluded a randomized controlled trial of several palliative care models, identifying the model that improved patient satisfaction and outcomes most successfully. The next year, after the organization's national executive leadership had set the expectation that all its member hospitals would implement this care model within 1 year, the organization established a large-scale initiative to disseminate the model. Within a 2-year period, the model was in place at all 32 network hospitals, the number of palliative care consults had risen from 1,572 to 16,293, and the number of interdisciplinary palliative care teams had more than doubled. One of the more important factors responsible for this successful dissemination was the clear relative advantage of the palliative care model in terms of patient satisfaction, outcomes, and cost, as demonstrated by the randomized controlled trial. This initiative also was compatible with clinician values, which spurred an emotional pull to improve care during advanced illness. Additional reasons for the dissemination included the involvement of senior leadership and opinion leaders, existing communication channels throughout the organization, and broad social networks that shared information. While many positive factors encouraged dissemination, several impediments were faced as well, including resource constraints, the competition of preexisting palliative care models, and ambiguous accountability for implementation (Della Penna et al., 2009).
As demonstrated by this example, a considerable amount is known about the factors that contribute to successful dissemination and scale-up. For any individual case, however, it is unknown which factors will best yield widespread implementation; the success of any particular knowledge, practice, or technology is context specific and depends on local conditions and human factors (Davidoff, 2009) (see Chapter 9 for a discussion of the spread of ideas within an organization). Also unknown is how the factors that influence dissemination interact with one another to increase (or decrease) its likelihood.
A final element in understanding dissemination is customization to local conditions. As new technologies and procedures diffuse into clinical practice, health care professionals further modify and extend their application by discovering new populations, indications, and long-term effects. This observation highlights the importance of measuring the health and economic outcomes of clinical interventions in everyday practice (IOM, 2010a). The case of coronary artery bypass graft surgery offers an example of how the use of treatments changes over time: it is estimated that only 4 to 13 percent of patients who underwent this surgery a decade after its introduction would have met the eligibility criteria of the trials that determined its initial effectiveness (Hlatky et al., 1984). Similar results have been noted for other interventions; for example, slightly more than half of patients receiving the antiplatelet agent clopidogrel for vascular disease would have been eligible for the clinical trials that demonstrated its effectiveness (Choudhry et al., 2008). The ultimate use of a treatment or intervention may be very different from what its developers initially envisioned.
Conclusion 6-4: As the pace of knowledge generation accelerates, new approaches are needed to deliver the right information, in a clear and understandable format, to patients and clinicians as they partner to make clinical decisions.
Related findings:
The slow pace of dissemination and implementation of new knowledge in health care is harmful to patients. For example, it took 13 years for most experts to recommend thrombolytic drugs for heart attack treatment after their first positive clinical trial (see Chapter 2). Available evidence often is unused in clinical decision making. One analysis of the use of implantable cardioverter-defibrillator (ICD) implants found that 22 percent were implanted in circumstances outside of professional society guidelines (see Chapter 3). Decision support tools, which can be broadly provided in electronic health records, hold promise for improving the application of evidence. One study found that digital decision support tools helped clinicians apply clinical guidelines, improving health outcomes for diabetics by 15 percent.
PEOPLE, PATIENTS, AND CONSUMERS AS ACTIVE STAKEHOLDERS
Given the critical role of patients and consumers in the health care system, patients need to be more fully engaged in clinical research and the data utility. The success of both enterprises depends on patient support and investment in their aims. For clinical research, this means incorporating patient perspectives and greater public participation (see also Chapter 7) to ensure that the research enterprise addresses patient needs (IOM, 2011d). For the data utility, the public has an important role in motivating its expansion to improve care and build knowledge.
Currently, public awareness of and participation in the clinical research enterprise remains limited, as exemplified by a reduced willingness to participate in clinical trials during the past decade (Woolley and Propst, 2005). In addition, national surveys from 2005 and 2010 found that approximately two-thirds of respondents had concerns about the privacy and security of their health information (Holmes and Karp, 2005; Undem, 2010). Improving this situation will require new efforts to build trust in the clinical research enterprise among patients, consumers, and the public. Building this trust will require effort on multiple fronts, including increasing trust in the results of clinical research, being open and honest about the risks and benefits of this type of research, and ensuring that appropriate privacy and security safeguards are in place for health data.
Opportunities exist for improving patient engagement in clinical research. There is some evidence that patients with complex conditions, such as cancer, may be open to sharing data for research purposes, with one study finding that 60–70 percent of cancer patients agreed their deidentified clinical data should be shared to improve clinical knowledge (Beckjord et al., 2011). Similarly, a 2004 survey found that almost 70 percent of respondents would willingly share deidentified health information to improve health care services, and a similar percentage would share their deidentified data with researchers (Research!America, 2004). A recent national survey of consumers found that almost 90 percent of respondents strongly or somewhat agreed that their health data should be used “to help improve the care of future patients who might have the same or similar condition” (Alston and Paget, 2012).
Ideally, clinical decisions should balance the health benefits of a given intervention against potential harms, taking into consideration the patient's preferences, needs, and values. Research that incorporates patient perspectives will potentially be more useful for clinicians and patients making such decisions. One means of accomplishing this is to collect information on outcomes from patients with respect to their quality of life, such as their level of function or emotional state. While important, however, it can be difficult to design instruments that can collect high-quality data reflecting a health concept of interest (Rothman et al., 2009). One promising initiative is the NIH Patient-Reported Outcomes Measurement Information System (PROMIS), which incorporates a series of items measuring different aspects of physical, mental, and social health (Cella et al., 2007, 2010). Continued improvements in the collection of this type of clinical data hold promise for improving the ability of research to help patients understand how therapies and interventions may affect their quality of life.
In addition, novel technologies allow for new means of collecting health care data directly from patients. Enabled by advances in digital technologies and informatics, patients and consumers now have the ability to be involved in collecting and sharing data on their personal condition. This vision is being actualized in biobanks operated by disease-specific organizations, in addition to social networking sites. Examples of social networking sites that aim to promote patient participation in research include PatientsLikeMe®, Love/Avon Army of Women, and Facebook health groups (see Box 6-6). While there are specific challenges for these patient-initiated approaches, due especially to bias in self-reporting, as well as issues of data quality and protection against discrimination, the prevalence of such approaches can only be expected to increase.
One major recent initiative that focuses attention on patients in clinical research is PCORI, which was established by the Patient Protection and Affordable Care Act of 2010. As noted in its mission statement, PCORI seeks to help consumers and patients make informed health care decisions by encouraging research guided by patients, caregivers, and the entire health care community (Washington and Lipstein, 2011). Because PCORI is relatively new, it is in the process of considering methods and standards for research focused on patient-centered outcomes, drafting national priorities, and developing a research agenda. This type of research holds promise for increasing the patient-centeredness of the entire clinical research enterprise.
FRAMEWORK FOR ACHIEVING THE VISION5
Knowledge generation in the U.S. health care system presents a fundamental paradox. While the clinical research enterprise generates new insights at an ever-increasing rate, the demand for knowledge at the point of care remains unmet. The result is decisions by clinicians and patients that are inadequately informed by evidence. In addition, the data generated from every patient encounter hold tremendous promise to serve as a clinical data infrastructure that, through the use of new research techniques, can begin to meet the system's need for real-time clinical knowledge.
Given advances in computing and other technologies, the potential exists to create a clinical data utility that provides a substantial opportunity for learning (President's Information Technology Advisory Committee, 2001, 2004). The creation of this data utility will require action on the technological, clinical, research, and administrative fronts—from identifying the data that need to be captured, to encouraging broader sharing and communication of the data, to effecting the data's widespread clinical use. Recommendation 1 details the steps necessary to develop a clinical data infrastructure that supports clinical care, improvement initiatives, and research.
Recommendation 1: The Digital Infrastructure
Improve the capacity to capture clinical, care delivery process, and financial data for better care, system improvement, and the generation of new knowledge. Data generated in the course of care delivery should be digitally collected, compiled, and protected as a reliable and accessible resource for care management, process improvement, public health, and the generation of new knowledge.
Strategies for progress toward this goal:
Health care delivery organizations and clinicians should fully and effectively employ digital systems that capture patient care experiences reliably and consistently, and implement standards and practices that advance the interoperability of data systems. The National Coordinator for Health Information Technology, digital technology developers, and standards organizations should ensure that the digital infrastructure captures and delivers the core data elements and interoperability needed to support better care, system improvement, and the generation of new knowledge. Payers, health care delivery organizations, and medical product companies should contribute data to research and analytic consortia to support expanded use of care data to generate new insights. Patients should participate in the development of a robust data utility; use new clinical communication tools, such as personal portals, for self-management and care activities; and be involved in building new knowledge, such as through patient-reported outcomes and other knowledge processes. The Secretary of Health and Human Services should encourage the development of distributed data research networks and expand the availability of departmental health data resources for translation into accessible knowledge that can be used for improving care, lowering costs, and enhancing public health. Research funding agencies and organizations, such as the National Institutes of Health, the Agency for Healthcare Research and Quality, the Veterans Health Administration, the Department of Defense, and the Patient-Centered Outcomes Research Institute, should promote research designs and methods that draw naturally on existing care processes and that also support ongoing quality-improvement efforts.
Legal and regulatory restrictions can serve as a barrier to real-time learning and improvement. Results of previous surveys of health researchers suggest that the current formulation of the HIPAA privacy rule has increased the cost and time needed to conduct research, that different institutional interpretations of HIPAA and associated regulations have impeded collaboration, and that the rule has made it difficult to recruit subjects (Association of Academic Health Centers, 2008; Goss et al., 2009; Greene et al., 2006; IOM, 2009a; Ness, 2007). While privacy is an important societal and personal value, the current formulation of the privacy rule not only offers limited protection to patients but also may impede the broader health research enterprise (IOM, 2009a). Recommendation 2 outlines actions needed to address this challenge, drawing on the IOM report Beyond the HIPAA Privacy Rule (IOM, 2009a).
Recommendation 2: The Data Utility
Streamline and revise research regulations to improve care, promote the capture of clinical data, and generate knowledge. Regulatory agencies should clarify and improve regulations governing the collection and use of clinical data to ensure patient privacy but also the seamless use of clinical data for better care coordination and management, improved care, and knowledge enhancement.
Strategies for progress toward this goal:
The Secretary of Health and Human Services should accelerate and expand the review of the Health Insurance Portability and Accountability Act (HIPAA) and institutional review board (IRB) policies with respect to actual or perceived regulatory impediments to the protected use of clinical data, and clarify regulations and their interpretation to support the use of clinical data as a resource for advancing science and care improvement. Patient and consumer groups, clinicians, professional specialty societies, health care delivery organizations, voluntary organizations, researchers, and grantmakers should develop strategies and outreach to improve understanding of the benefits and importance of accelerating the use of clinical data to improve care and health outcomes.
Further, new knowledge can be poorly integrated into regular clinical care, highlighting the need for new approaches to deliver the right information to the point of care. To ensure the availability of clinical knowledge when and where needed, Recommendation 3 outlines actions that can be taken to disseminate clinical knowledge broadly and ensure its widespread application.
Recommendation 3: Clinical Decision Support
Accelerate integration of the best clinical knowledge into care decisions. Decision support tools and knowledge management systems should be routine features of health care delivery to ensure that decisions made by clinicians and patients are informed by current best evidence.
Strategies for progress toward this goal:
Clinicians and health care organizations should adopt tools that deliver reliable, current clinical knowledge to the point of care, and organizations should adopt incentives that encourage the use of these tools. Research organizations, advocacy organizations, professional specialty societies, and care delivery organizations should facilitate the development, accessibility, and use of evidence-based and harmonized clinical practice guidelines. Public and private payers should promote the adoption of decision support tools, knowledge management systems, and evidence-based clinical practice guidelines by structuring payment and contracting policies to reward effective, evidence-based care that improves patient health. Health professional education programs should teach new methods for accessing, managing, and applying evidence; engaging in lifelong learning; understanding human behavior and social science; and delivering safe care in an interdisciplinary environment. Research funding agencies and organizations should promote research into the barriers and systematic challenges to the dissemination and use of evidence at the point of care, and support research to develop strategies and methods that can improve the usefulness and accessibility of patient outcome data and scientific evidence for clinicians and patients.
Collectively, implementation of the above recommendations would increase the supply of clinical data, reduce legal and regulatory barriers to the creation of new knowledge, and improve the integration of new knowledge into regular clinical practice. Addressing the issues targeted by these recommendations can increase the knowledge available to answer relevant clinical questions while promoting the use of new clinical information in regular patient care.
REFERENCES
- AHRQ (Agency for Healthcare Research and Quality). Registries for evaluating patient outcomes: A user's guide. 2nd ed. Rockville, MD: AHRQ; 2010.
- Akhter N, Milford-Beland S, Roe MT, Piana RN, Kao J, Shroff A. American Heart Journal. 1. Vol. 157. 2009. Gender differences among patients with acute coronary syndromes undergoing percutaneous coronary intervention in the American College of Cardiology-National Cardiovascular Data Registry (ACC-NCDR) pp. 141–148. [PubMed: 19081410]
- Alecxih L, Shen S, Chan I, Taylor D, Drabek J. Individuals living in the community with chronic conditions and functional limitations: A closer look. 2010. [June 23, 2011]. http://aspe
.hhs.gov/daltcp /reports/2010/closerlook.pdf. - Alston C, Paget L. Communicating evidence in health care: Engaging patients for improved health care decisions. 2012. [August 31, 2012]. http://iom
.edu/∼ /media/Files/Activity%20Files /Quality /VSRT/IC%20Meeting%20Docs /ECIC%2006-07-12 /Lyn%20Paget%20and%20Chuck%20Alston .pdf. - Association of Academic Health Centers. HIPAA creating barriers to research and discovery: HIPAA problems widespread and unresolved since 2003. 2008. [June 9, 2011]. http://www
.aahcdc.org /policy/reddot/AAHC _HIPAA_Creating_Barriers.pdf. - Avorn J, Fischer M. Health Affairs. 10. Vol. 29. 2010. “Bench to behavior”: Translating comparative effectiveness research into improved clinical practice; pp. 1891–1900. [PubMed: 20921491]
- Bastian H, Glasziou P, Chalmers I. PLoS Medicine. 9. Vol. 7. 2010. Seventy-five trials and eleven systematic reviews a day: How will we ever keep up; p. e1000326. [PMC free article: PMC2943439] [PubMed: 20877712]
- Bate P, Robert G, Bevan H. Quality & Safety in Health Care. 1. Vol. 13. 2004. The next phase of healthcare improvement: What can we learn from social movements; pp. 62–66. [PMC free article: PMC1758052] [PubMed: 14757802]
- Beckjord EB, Rechis R, Nutt S, Shulman L, Hesse BW. Journal of Oncology Practice. 4. Vol. 7. 2011. What do people affected by cancer think about electronic health information exchange? Results from the 2010 Livestrong Electronic Health Information Exchange Survey and the 2008 Health Information National Trends Survey; pp. 237–241. [PMC free article: PMC3140446] [PubMed: 22043188]
- Behrman RE, Benner JS, Brown JS, McClellan M, Woodcock J, Platt R. New England Journal of Medicine. 6. Vol. 364. 2011. Developing the sentinel system—a national resource for evidence development; pp. 498–499. [PubMed: 21226658]
- Berry DA, Inoue L, Shen Y, Venier J, Cohen D, Bondy M, Theriault R, Munsell MF. Journal of the National Cancer Institute Monographs. 36. 2006. Modeling the impact of treatment and screening on U.S. breast cancer mortality: A Bayesian approach; pp. 30–36. [PubMed: 17032892]
- Berwick DM. Journal of the American Medical Association. 15. Vol. 289. 2003. Disseminating innovations in health care; pp. 1969–1975. [PubMed: 12697800]
- Bevers TB, Anderson BO, Bonaccio E, Buys S, Daly MB, Dempsey PJ, Farrar WB, Fleming I, Garber JE, Harris RE, Heerdt AS, Helvie M, Huff JG, Khakpour N, Khan SA, Krontiras H, Lyman G, Rafferty E, Shaw S, Smith ML, Tsangaris TN, Williams C, Yankeelov T, Yaneeklov T., National Comprehensive Cancer Network. Journal of the National Comprehensive Cancer Network. 10. Vol. 7. 2009. NCCN clinical practice guidelines in oncology: Breast cancer screening and diagnosis; pp. 1060–1096. [PubMed: 19930975]
- Blue Cross Blue Shield of Massachusetts Foundation. How doctors think—interview with Dr. Jerome Groopman. 2007. [August 31, 2012]. http:
//bluecrossmafoundation .org/∼ /media/Files/Newsroom /Press%20Releases/090626PodcastPR.pdf. - Blumenthal D. Journal of Academic Medicine. 2. Vol. 81. 2006. Data withholding in genetics and the other life sciences: Prevalences and predictors; pp. 137–145. [PubMed: 16436574]
- Bocchino CA. Learning what works: Infrastructure required for comparative effectiveness research. Institute of Medicine. Washington, DC: The National Academies Press; 2011. Public-private partnerships: Health plans. In; pp. 293–300.
- Boyd CM, Darer J, Boult C, Fried LP, Boult L, Wu AW. Journal of the American Medical Association. 6. Vol. 294. 2005. Clinical practice guidelines and quality of care for older patients with multiple comorbid diseases: Implications for pay for performance; pp. 716–724. [PubMed: 16091574]
- British Medical Journal. Clinical evidence. 2011. [October 7, 2011]. http:
//clinicalevidence .bmj.com/ceweb/about/knowledge.jsp. - Brown JS, Holmes JH, Shah K, Hall K, Lazarus R, Platt R. Medical Care. Suppl. 6. Vol. 48. 2010. Distributed health data networks: A practical and preferred approach to multi-institutional evaluations of comparative effectiveness, safety, and quality of care; pp. S45–S51. [PubMed: 20473204]
- Brownstein JS, Murphy SN, Goldfine AB, Grant RW, Sordo M, Gainer V, Colecchi JA, Dubey A, Nathan DM, Glaser JP, Kohane IS. Diabetes Care. 3. Vol. 33. 2010. Rapid identification of myocardial infarction risk associated with diabetes medications using electronic medical records; pp. 526–531. [PMC free article: PMC2827502] [PubMed: 20009093]
- Cabana MD, Rand CS, Powe NR, Wu AW, Wilson MH, Abboud PA, Rubin HR. Journal of the American Medical Association. 15. Vol. 282. 1999. Why don't physicians follow clinical practice guidelines? A framework for improvement; pp. 1458–1465. [PubMed: 10535437]
- Cain M, Mittman R. Diffusion of innovation in health care. Oakland: California HealthCare Foundation; 2002.
- Califf RM. Health Affairs (Millwood). 1. Vol. 23. 2004. Defining the balance of risk and benefit in the era of genomics and proteomics; pp. 77–87. [PubMed: 15002630]
- Campbell MJ, Donner A, Klar N. Statistics in Medicine. 1. Vol. 26. 2007. Developments in cluster randomized trials and statistics in medicine; pp. 2–19. [PubMed: 17136746]
- Carnegie Foundation for the Advancement of Teaching. Getting ideas into action: Building networked improvement communities in education. 2010. [June 17, 2011]. http://www
.carnegiefoundation .org/spotlight /webinar-bryk-gomez-building-networked-improvement-communities-in-education. - Cebul RD, Love TE, Jain AK, Hebert CJ. New England Journal of Medicine. 9. Vol. 365. 2011. Electronic health records and quality of diabetes care; pp. 825–833. [PubMed: 21879900]
- Cella D, Yount S, Rothrock N, Gershon R, Cook K, Reeve B, Ader D, Fries JF, Bruce B, Rose M., PROMIS Cooperative Group. Medical Care. 5, Suppl. 1. Vol. 45. 2007. The Patient-Reported Outcomes Measurement Information System (PROMIS): Progress of an NIH roadmap cooperative group during its first two years; pp. S3–S11. [PMC free article: PMC2829758] [PubMed: 17443116]
- Cella D, Riley W, Stone A, Rothrock N, Reeve B, Yount S, Amtmann D, Bode R, Buysse D, Choi S, Cook K, Devellis R, DeWalt D, Fries JF, Gershon R, Hahn EA, Lai JS, Pilkonis P, Revicki D, Rose M, Weinfurt K, Hays R., PROMIS Cooperative Group. Journal of Clinical Epidemiology. 11. Vol. 63. 2010. The Patient-Reported Outcomes Measurement Information System (PROMIS) developed and tested its first wave of adult self-reported health outcome item banks: 2005–2008; pp. 1179–1194. [PMC free article: PMC2965562] [PubMed: 20685078]
- Chauhan SP, Berghella V, Sanderson M, Magann EF, Morrison JC. American Journal of Obstetrics and Gynecology. 6. Vol. 194. 2006. American College of Obstetricians and Gynecologists practice bulletins: An overview; pp. 1564–1572. [PubMed: 16731072]
- Choudhry NK, Levin R, Avorn J. American Heart Journal. 5. Vol. 155. 2008. The economic consequences of non-evidence-based clopidogrel use; pp. 904–909. [PubMed: 18440340]
- Chow SC, Chang M. Orphanet Journal of Rare Diseases. Vol. 3. 2008. Adaptive design methods in clinical trials—a review; p. 11. [PMC free article: PMC2422839] [PubMed: 18454853]
- Cohen E, Uleryk E, Jasuja M, Parkin PC. Journal of Clinical Epidemiology. 2. Vol. 60. 2007. An absence of pediatric randomized controlled trials in general medical journals, 1985–2004; pp. 118–123. [PubMed: 17208117]
- Committee on Developments in the Science of Learning, Committee on Learning Research and Educational Practice, and National Research Council. How people learn: Brain, mind, experience, and school. (expanded edition). Washington, DC: National Academy Press; 2000.
- Concato J, Shah N, Horwitz RI. New England Journal of Medicine. 25. Vol. 342. 2000. Randomized, controlled trials, observational studies, and the hierarchy of research designs; pp. 1887–1892. [PMC free article: PMC1557642] [PubMed: 10861325]
- Cutler DM, McClellan MB., National Bureau of Economic Research. NBER working paper series working paper 5751. 1996. [August 31, 2012]. The determinants of technological change in heart attack treatment. In. http://www
.nber.org/papers/w5751 .pdf?new_window=1. - Darst JR, Newburger JW, Resch S, Rathod RH, Lock JE. Congenital Heart Disease. 4. Vol. 5. 2010. Deciding without data; pp. 339–342. [PMC free article: PMC4283550] [PubMed: 20653700]
- Davidoff F. Journal of the American Medical Association. 23. Vol. 302. 2009. Heterogeneity is not always noise: Lessons from improvement; pp. 2580–2586. [PubMed: 20009058]
- Davis DA, TaylorVaisey A. Canadian Medical Association Journal. 4. Vol. 157. 1997. Translating guidelines into practice—a systematic review of theoretic concepts, practical experience and research evidence in the adoption of clinical practice guidelines; pp. 408–416. [PMC free article: PMC1227916] [PubMed: 9275952]
- Davis RL, Eastman D, McPhillips H, Raebel MA, Andrade SE, Smith D, Yood MU, Dublin S, Platt R. Pharmacoepidemiology and Drug Safety. 2. Vol. 20. 2011. Risks of congenital malformations and perinatal events among infants exposed to calcium channel and beta-blockers during pregnancy; pp. 138–145. [PMC free article: PMC3232183] [PubMed: 21254284]
- Decker SL, Jamoom EW, Sisk JE. Health Affairs (Millwood). 5. Vol. 31. 2012. Physicians in nonprimary care and small practices and those age 55 and older lag in adopting electronic health record systems; pp. 1108–1114. [PubMed: 22535502]
- DellaPenna R, Martel H, Neuwirth EB, Rice J, Filipski MI, Green J, Bellows J. BMC Health Services Research. Vol. 9. 2009. Rapid spread of complex change: A case study in inpatient palliative care; p. 245. [PMC free article: PMC2811707] [PubMed: 20040099]
- DesRoches CM, Worzala C, Joshi MS, Kralovec PD, Jha AK. Health Affairs (Millwood). 5. Vol. 31. 2012. Small, nonteaching, and rural hospitals continue to be slow in adopting electronic health record systems; pp. 1092–1099. [PubMed: 22535503]
- Detmer DE. BMC Medical Information Decision Making. Vol. 3. 2003. Building the national health information infrastructure for personal health, health care services, public health, and research; p. 1. [PMC free article: PMC149369] [PubMed: 12525262]
- Dhruva SS, Redberg RF. Archives of Internal Medicine. 2. Vol. 168. 2008. Variations between clinical trial participants and Medicare beneficiaries in evidence used for Medicare national coverage decisions; pp. 136–140. [PubMed: 18227358]
- Dolan PL. American Medical News. Jan 11, 2010. 86% of physicians use Internet to access health information.
- Dopson S, FitzGerald L, Ferlie E, Gabbay J, Locock L. Health Care Management Review. 3. Vol. 27. 2002. No magic targets! Changing clinical practice to become more evidence based; pp. 35–47. [PubMed: 12146782]
- Eddy DM, Schlessinger L. Diabetes Care. 11. Vol. 26. 2003. Archimedes: A trial-validated model of diabetes; pp. 3093–3101. [PubMed: 14578245]
- Eddy DM, Adler J, Patterson B, Lucas D, Smith KA, Morris M. Annals of Internal Medicine. 9. Vol. 154. 2011. Individualized guidelines: The potential for increasing quality and reducing costs; pp. 627–634. [PubMed: 21536939]
- Eldridge S, Ashby D, Bennett C, Wakelin M, Feder G. British Medical Journal. 7649. Vol. 336. 2008. Internal and external validity of cluster randomised trials: Systematic review of recent trials; pp. 876–880. [PMC free article: PMC2323095] [PubMed: 18364360]
- Ferlie EB, Shortell SM. Milbank Quarterly. 2. Vol. 79. 2001. Improving the quality of health care in the United Kingdom and the United States: A framework for change; pp. 281–315. [PMC free article: PMC2751188] [PubMed: 11439467]
- Fiore LD, Brophy M, Ferguson RE, D'Avolio L, Hermos JA, Lew RA, Doros G, Conrad CH, O'Neil JA, Sabin TP, Kaufman J, Swartz SL, Lawler E, Liang MH, Gaziano JM, Lavori PW. Clinical Trials. 2. Vol. 8. 2011. A point-of-care clinical trial comparing insulin administered using a sliding scale versus a weight-based regimen; pp. 183–195. [PMC free article: PMC3195898] [PubMed: 21478329]
- Flodgren G, Parmelli E, Doumit G, Gattellari M, O'Brien MA, Grimshaw J, Eccles MP. Cochrane Database of Systematic Reviews. 8. 2011. Local opinion leaders: Effects on professional practice and health care outcomes; p. CD000125. [PMC free article: PMC4172331] [PubMed: 21833939]
- Frangakis C. Clinical Trials. 2. Vol. 6. 2009. The calibration of treatment effects from clinical trials to target populations; pp. 136–140. [PMC free article: PMC4137779] [PubMed: 19342466]
- Fridsma D. HealthITBuzz. Sep 23, 2011. Join query health in developing national standards for population queries. In.
- Genetics and Public Policy Center. FDA regulation of genetic tests. 2008. [January 4, 2010]. http://www
.dnapolicy .org/policy.issue.php?action =detail&issuebrief_id =11&print=1. - Gill P, Dowell AC, Neal RD, Smith N, Heywood P, Wilson AE. British Medical Journal. 7034. Vol. 312. 1996. Evidence based general practice: A retrospective study of interventions in one training practice; pp. 819–821. [PMC free article: PMC2350715] [PubMed: 8608291]
- Go AS, Magid DJ, Wells B, Sung SH, Cassidy-Bushrow AE, Greenlee RT, Langer RD, Lieu TA, Margolis KL, Masoudi FA, McNeal CJ, Murata GH, Newton KM, Novotny R, Reynolds K, Roblin DW, Smith DH, Vupputuri S, White RE, Olson J, Rumsfeld JS, Gurwitz JH. Cardiovascular Quality and Outcomes. 2. Vol. 1. 2008. The Cardiovascular Research Network: A new paradigm for cardiovascular quality and outcomes research; pp. 138–147. [PubMed: 20031802]
- Goss E, Link MP, Bruinooge SS, Lawrence TS, Tepper JE, Runowicz CD, Schilsky RL. Journal of Clinical Oncology. 24. Vol. 27. 2009. The impact of the privacy rule on cancer research: Variations in attitudes and application of regulatory standards; pp. 4014–4020. [PubMed: 19620480]
- Grady D, Rubin SM, Petitti DB, Fox CS, Black D, Ettinger B, Ernster VL, Cummings SR. Annals of Internal Medicine. 12. Vol. 117. 1992. Hormone therapy to prevent disease and prolong life in postmenopausal women; pp. 1016–1037. [PubMed: 1443971]
- Green PL, Plsek PE. Joint Commission Journal on Quality Improvement. 2. Vol. 28. 2002. Coaching and leadership for the diffusion of innovation in health care: A different type of multi-organization improvement collaborative; pp. 55–71. [PubMed: 11838297]
- Greene SM, Geiger AM, Harris EL, Altschuler A, Nekhlyudov L, Barton MB, Rolnick SJ, Elmore JG, Fletcher S. Annals of Epidemiology. 4. Vol. 16. 2006. Impact of IRB requirements on a multicenter survey of prophylactic mastectomy outcomes; pp. 275–278. [PubMed: 16005245]
- Greenhalgh T, Robert G, Macfarlane F, Bate P, Kyriakidou O. Milbank Quarterly. 4. Vol. 82. 2004. Diffusion of innovations in service organizations: Systematic review and recommendations; pp. 581–629. [PMC free article: PMC2690184] [PubMed: 15595944]
- Greenhouse JB, Kaizar EE, Kelleher K, Seltman H, Gardner W. Statistics in Medicine. 11. Vol. 27. 2008. Generalizing from clinical trial data: A case study. The risk of suicidality among pediatric antidepressant users; pp. 1801–1813. [PMC free article: PMC2963861] [PubMed: 18381709]
- Grodstein F, Manson JE, Colditz GA, Willett WC, Speizer FE, Stampfer MJ. Annals of Internal Medicine. 12. Vol. 133. 2000. A prospective, observational study of postmenopausal hormone therapy and primary prevention of cardiovascular disease; pp. 933–941. [PubMed: 11119394]
- Grodstein F, Clarkson TB, Manson JE. New England Journal of Medicine. 7. Vol. 348. 2003. Understanding the divergent data on postmenopausal hormone therapy; pp. 645–650. [PubMed: 12584376]
- Grodstein F, Manson JE, Stampfer MJ. Journal of Women's Health. 1. Vol. 15. 2006. Hormone therapy and coronary heart disease: The role of time since menopause and age at hormone initiation; pp. 35–44. [PubMed: 16417416]
- Grove A. Science. 6050. Vol. 333. 2011. Rethinking clinical trials; p. 1679. [PubMed: 21940863]
- Grover FL, Shroyer AL, Hammermeister K, Edwards FH, Ferguson TB, Dziuban SW, Cleveland JC, Clark RE, McDonald G. Annals of Surgery. 4. Vol. 234. 2001. A decade's experience with quality improvement in cardiac surgery using the Veterans Affairs and Society of Thoracic Surgeons national databases; pp. 464–472. [PMC free article: PMC1422070] [PubMed: 11573040]
- Harrison TR. Principles of internal medicine. 4th. New York: Blakiston Division, McGraw-Hill; 1962.
- Hennekens CH, Buring JE, Mayrent SL. Epidemiology in medicine. 1st. Boston, MA: Little, Brown; 1987.
- Hlatky MA, Lee KL, Harrell FE, Califf RM, Pryor DB, Mark DB, Rosati RA. Statistics in Medicine. 4. Vol. 3. 1984. Tying clinical research to patient-care by use of an observational database; pp. 375–384. [PubMed: 6396793]
- Holmes B, Karp S. National consumer health privacy survey 2005. 2005. [August 21, 2012]. http://www
.chcf.org/∼ /media/MEDIA%20LIBRARY%20Files /PDF /C/PDF%20ConsumersHealthInfoTechnologyNationalSurvey.pdf. - Holve E, Pittman P. A first look at the volume and cost of comparative effectiveness research in the United States. Washington, DC: AcademyHealth; 2009.
- Holve E, Pittman P. Learning what works: Infrastructure required for comparative effectiveness research: Workshop summary. Institute of Medicine. Washington, DC: The National Academies Press; 2011. The cost and volume of comparative effectiveness research. In; pp. 89–96. [PubMed: 22013609]
- Hornbrook MC, Hart G, Ellis JL, Bachman DJ, Ansell G, Greene SM, Wagner EH, Pardee R, Schmidt MM, Geiger A, Butani AL, Field T, Fouayzi H, Miroshnik I, Liu L, Diseker R, Wells K, Krajenta R, Lamerato L, Dudas CN. JNCI Monographs. 35. Vol. 2005. 2005. Building a virtual cancer research organization; pp. 12–25. [PubMed: 16287881]
- Hsiao C-J, Hing E, Socey TC, Cai B. Electronic health record systems and intent to apply for meaningful use incentives among office-based physician practices: United States, 2001–2011. Hyattsville, MD: National Center for Health Statistics; 2011. [PubMed: 22617322]
- Ioannidis JPA. Journal of the American Medical Association. 2. Vol. 294. 2005a. Contradicted and initially stronger effects in highly cited clinical research; pp. 218–228. [PubMed: 16014596]
- Ioannidis JPA. PLoS Medicine. 8. Vol. 2. 2005b. Why most published research findings are false; pp. 696–701.
- IOM (Institute of Medicine). Assessing medical technologies. Washington, DC: National Academy Press; 1985. [PubMed: 25032428]
- IOM. Crossing the quality chasm: A new health system for the 21st century. Washington, DC: National Academy Press; 2001a. [PubMed: 25057539]
- IOM. Mammography and beyond: Developing technologies for the early detection of breast cancer. Washington, DC: National Academy Press; 2001b.
- IOM. Saving women's lives: Strategies for improving breast cancer detection and diagnosis: A Breast Cancer Research Foundation and Institute of Medicine symposium. Washington, DC: The National Academies Press; 2005. [PubMed: 22379646]
- IOM. Knowing what works in health care: A roadmap for the nation. Washington, DC: The National Academies Press; 2008.
- IOM. Beyond the HIPAA privacy rule: Enhancing privacy, improving health through research. Washington, DC: The National Academies Press; 2009a. [PubMed: 20662116]
- IOM. Conflict of interest in medical research, education, and practice. Washington, DC: The National Academies Press; 2009b. [PubMed: 20662118]
- IOM. Initial national priorities for comparative effectiveness research. Washington, DC: The National Academies Press; 2009c.
- IOM. Redesigning the clinical effectiveness research paradigm: Innovation and practice-based approaches: Workshop summary. Washington, DC: The National Academies Press; 2010a. [PubMed: 21210561]
- IOM. Transforming clinical research in the United States: Challenges and opportunities: Workshop summary. Washington, DC: The National Academies Press; 2010b. [PubMed: 21210556]
- IOM. Clinical data as the basic staple of health learning: Workshop summary. Washington, DC: The National Academies Press; 2011a.
- IOM. Clinical practice guidelines we can trust. Washington, DC: The National Academies Press; 2011b. [PubMed: 24983061]
- IOM. Digital infrastructure for the learning health system: The foundation for continuous improvement in health and health care: A workshop summary. Washington, DC: The National Academies Press; 2011c. [PubMed: 22379651]
- IOM. Public engagement and clinical trials: New models and disruptive technologies: Workshop summary. Washington, DC: The National Academies Press; 2011d. [PubMed: 22514814]
- Kalorama Information. Handhelds in healthcare: The world market for PDAs, tablet PCs, handheld monitors & scanners. 2010. [August 31, 2011]. http://www
.kaloramainformation .com/Handhelds-Healthcare-PDAs-2703662/ - Kasper DL, Harrison TR. Harrison's principles of internal medicine. 16th. Vol. 2. New York: McGraw-Hill, Medical Publications Division; 2005.
- Kuperman GJ. Journal of the American Medical Informatics Association. 5. Vol. 18. 2011. Health-information exchange: Why are we doing it, and what are we doing; pp. 678–682. [PMC free article: PMC3168299] [PubMed: 21676940]
- Larson EB. The learning healthcare system: Workshop summary. Institute of Medicine. Washington, DC: The National Academies Press; 2007. The HMO research network as a test bed. In; pp. 223–232. [PubMed: 21452449]
- Larsson S, Lawyer P, Garellick G, Lindahl B, Lundström M. Health Affairs (Millwood). 1. Vol. 31. 2011. Use of 13 disease registries in 5 countries demonstrates the potential to use outcome data to improve health care's value; pp. 220–227. [PubMed: 22155485]
- Lauer MS, Skarlatos S. Circulation. 7. Vol. 121. 2010. Translational research for cardiovascular diseases at the National Heart, Lung, and Blood Institute: Moving from bench to bedside and from bedside to community; pp. 929–933. [PMC free article: PMC4001816] [PubMed: 20177007]
- Lazarus R, Yih K, Platt R. BMC Public Health. Vol. 6. 2006. Distributed data processing for public health surveillance; p. 235. [PMC free article: PMC1618842] [PubMed: 16984658]
- Lee DH, Vielemeyer O. Archives of Internal Medicine. 1. Vol. 171. 2011. Analysis of overall level of evidence behind Infectious Diseases Society of America practice guidelines; pp. 18–22. [PubMed: 21220656]
- Lee HY, Ahn HS, Jang JA, Lee YM, Hann HJ, Park MS, Ahn DS. International Journal of Clinical Practice. 8. Vol. 59. 2005a. Comparison of evidence-based therapeutic intervention between community-and hospital-based primary care clinics; pp. 975–980. [PubMed: 16033623]
- Lee IM, Cook NR, Gaziano JM, Gordon D, Ridker PM, Manson JE, Hennekens CH, Buring JE. Journal of the American Medical Association. 1. Vol. 294. 2005b. Vitamin E in the primary prevention of cardiovascular disease and cancer—The Women's Health Study: A randomized controlled trial; pp. 56–65. [PubMed: 15998891]
- Let data speak to data. Nature. 7068. Vol. 438. 2005. p. 531. [PubMed: 16319843]
- Lohr S. The New York Times. Apr 6, 2011. Big medical groups begin patient data-sharing project.
- Luce BR, Kramer JM, Goodman SN, Connor JT, Tunis S, Whicher D, Schwartz JS. Annals of Internal Medicine. 3. Vol. 151. 2009. Rethinking randomized clinical trials for comparative effectiveness research: The need for transformational change; pp. 206–209. [PubMed: 19567619]
- Mandel KE. Journal of the American Medical Association. 7. Vol. 303. 2010. Aligning rewards with large-scale improvement; pp. 663–664. [PubMed: 20159876]
- Manolio TA. Redesigning the clinical effectiveness research paradigm: Innovation and practice-based approaches: Workshop summary. Institute of Medicine. Washington, DC: The National Academies Press; 2010. Emerging genomic information. In; pp. 189–206. [PubMed: 21210561]
- Manson JE. Redesigning the clinical effectiveness research paradigm: Innovation and practice-based approaches: A workshop summary. Institute of Medicine. Washington, DC: National Academies Press; 2010. Hormone replacement therapy. In; pp. 89–104. [PubMed: 21210561]
- Manson JE, Hsia J, Johnson KC, Rossouw JE, Assaf AR, Lasser NL, Trevisan M, Black HR, Heckbert SR, Detrano R, Strickland OL, Wong ND, Crouse JR, Stein E, Cushman M., Women's Health Initiative Investigators. New England Journal of Medicine. 6. Vol. 349. 2003. Estrogen plus progestin and the risk of coronary heart disease; pp. 523–534. [PubMed: 12904517]
- McCannon CJ, Perla RJ. Joint Commission Journal on Quality and Patient Safety. 5. Vol. 35. 2009. Learning networks for sustainable, large-scale improvement; pp. 286–291. [PubMed: 19480384]
- McCannon CJ, Schall MW, Calkins DR, Nazem AG. British Medical Journal. 7553. Vol. 332. 2006. Saving 100,000 lives in US hospitals; pp. 1328–1330. [PMC free article: PMC1473113] [PubMed: 16740565]
- McCullough JS. Health Economics. 5. Vol. 17. 2008. The adoption of hospital information systems; pp. 649–664. [PubMed: 18050147]
- Meadows TA, Bhatt DL, Hirsch AT, Creager MA, Califf RM, Ohman EM, Cannon CP, Eagle KA, Alberts MJ, Goto S, Smith SC, Wilson PW, Watson KE, Steg PG., REACH Registry Investigators. American Heart Journal. 6. Vol. 158. 2009. Ethnic differences in the prevalence and treatment of cardiovascular risk factors in US outpatients with peripheral arterial disease: Insights from the reduction of atherothrombosis for continued health (REACH) registry; pp. 1038–1045. [PubMed: 19958873]
- Meier B, Roberts J. New York Times. 2011. [January 16, 2012]. Hip implant complaints suge, even as the dangers are studied. http://www
.nytimes.com /2011/08/23/business /complaints-soar-on-hip-implants-as-dangers-are-studied .html?pagewanted=all. - Murphy SN, Weber G, Mendis M, Gainer V, Chueh HC, Churchill S, Kohane I. Journal of the American Medical Informatics Association. 2. Vol. 17. 2010. Serving the enterprise and beyond with informatics for integrating biology and the bedside (i2b2) pp. 124–130. [PMC free article: PMC3000779] [PubMed: 20190053]
- National Comprehensive Cancer Network. NCCN clinical practice guidelines in oncology: Breast cancer. Fort Washington, PA: National Comprehensive Cancer Network; 2012.
- National Quality Forum. Quality data model 2.1. 2010. [April 27, 2011]. http://www
.qualityforum .org/Projects/h/QDS_Model /Quality_Data_Model .aspx#t=2&s=&p= - Ness RB. Journal of the American Medical Association. 18. Vol. 298. 2007. Influence of the HIPAA privacy rule on health research; pp. 2164–2170. [PubMed: 18000200]
- Nolan K, Schall MW, Erb F, Nolan T. Joint Commission Journal on Quality and Patient Safety. 6. Vol. 31. 2005. Using a framework for spread: The case of patient access in the Veterans Health Administration; pp. 339–347. [PubMed: 15999964]
- Norton WE, Mittman BS. Scaling-up health promotion/disease prevention programs in community settings: Barriers, facilitators, and initial recommendations. West Hartford, CT: The Patrick and Catherine Weldon Donaghue Medical Research Foundation; 2010.
- O'Connor GT, Plume SK, Olmstead EM, Morton JR, Maloney CT, Nugent WC, Hernandez F, Clough R, Leavitt BJ, Coffin LH, Marrin CA, Wennberg D, Birkmeyer JD, Charlesworth DC, Malenka DJ, Quinton HB, Kasper JF. Journal of the American Medical Association. 11. Vol. 275. 1996. A regional intervention to improve the hospital mortality associated with coronary artery bypass graft surgery. The Northern New England Cardiovascular Disease Study Group; pp. 841–846. [PubMed: 8596221]
- Pawlson G. Redesigning the clinical effectiveness research paradigm: Innovation and practice-based approaches: Workshop summary. Institute of Medicine. Washington, DC: The National Academies Press; 2010. Course-of-care data. In; pp. 325–331. [PubMed: 21210561]
- PCORI (Patient-Centered Outcomes Research Institute). Preliminary draft methodology report: Our questions, our decisions: Standards for patient-centered outcomes research. 2012. [July 6, 2012]. http://www
.pcori.org /assets/PCORI-MC-Research _Methods_Framework-Review_vFinalv3 .pdf. - Pearson TA, Manolio TA. Journal of the American Medical Association. 11. Vol. 299. 2008. How to interpret a genome-wide association study; pp. 1335–1344. [PubMed: 18349094]
- Physician Consortium for Performance Improvement. Advancing health care improvement through patient registries: Moving forward. 2011. [August 30, 2011]. http://www
.ama-assn.org /resources/doc/cqi /registry-meeting-paper.pdf. - Pisano GP, Bohmer RMJ, Edmondson AC. Management Science. 6. Vol. 47. 2001. Organizational differences in rates of learning: Evidence from the adoption of minimally invasive cardiac surgery; pp. 752–768.
- Piwowar HA, Becich MJ, Bilofsky H, Crowley RS., on behalf of the caBIG Data Sharing and Intellectual Capital Workspace. PLoS Medicine. 9. Vol. 5. 2008. Towards a data sharing culture: Recommendations for leadership from academic health centers; pp. 1315–1319. [PMC free article: PMC2528049] [PubMed: 18767901]
- Platt R. Redesigning the clinical effectiveness research paradigm: Innovation and practice-based approaches: Workshop summary. Institute of Medicine. Washington, DC: The National Academies Press; 2010. Distributed data networks. In; pp. 253–261. [PubMed: 21210561]
- Podolny J, Page K. Annual Review of Sociology. Vol. 24. 1998. Network forms of organization; pp. 57–76.
- popHealth. An open source quality measure reference implementation. 2012. [June 13, 2012]. http:
//projectpophealth.org/about.html. - Prasad V, Gall V, Cifu A. Archives of Internal Medicine. 18. Vol. 171. 2011. The frequency of medical reversal; pp. 1675–1676. [PubMed: 21747003]
- President's Information Technology Advisory Committee. Transforming health care through information technology. 2001. [August 31, 2012]. http://www
.itrd.gov/pubs /pitac/pitac-hc-9feb01.pdf. - President's Information Technology Advisory Committee. Revolutionizing health care through information technology. 2004. [August 31, 2012]. http://www
.itrd.gov/pitac /meetings/2004/20040617 /20040615_hit.pdf. - Redberg RF. Health Affairs. 1. Vol. 26. 2007. Evidence, appropriateness, and technology assessment in cardiology: A case study of computed tomography; pp. 86–95. [PubMed: 17211017]
- Research!America. Taking our pulse: The parade/research!America health poll. 2004. [August 21, 2012]. http://www
.researchamerica .org/uploads/poll2004parade.pdf. - Robert Wood Johnson Foundation. White paper (High-Value Health Care Project). 2010. [August 31, 2012]. How registries can help performance measurement improve care. In. http://www
.healthqualityalliance .org/userfiles /Final%20Registries %20paper%20062110(1).pdf. - Robinson JC, Casilino LP, Gillies RR, Rittenhouse DR, Shortell SS, Fernandes-Taylor S. Medical Care. 4. Vol. 47. 2009. Financial incentives, quality improvement programs, and the adoption of clinical information technology; pp. 411–417. [PubMed: 19238102]
- Rogers EM. Diffusion of innovations. 5th. New York: Free Press; 2003.
- Rossouw JE, Anderson GL, Prentice RL, LaCroix AZ, Kooperberg C, Stefanick ML, Jackson RD, Beresford SAA, Howard BV, Johnson KC, Kotchen M, Ockene J. Journal of the American Medical Association. 3. Vol. 288. 2002. Risks and benefits of estrogen plus progestin in healthy postmenopausal women—principal results from the Women's Health Initiative Randomized Controlled Trial; pp. 321–333. [PubMed: 12117397]
- Rothman M, Burke L, Erickson P, Leidy NK, Patrick DL, Petrie CD. Value Health. 8. Vol. 12. 2009. Use of existing patient-reported outcome (PRO) instruments and their modification: The ISPOR good research practices for evaluating and documenting content validity for the use of existing instruments and their modification pro task force report; pp. 1075–1083. [PubMed: 19804437]
- Savage EB, Ferguson TB, DiSesa VJ. Annals Thoracic Surgery. 3. Vol. 75. 2003. Use of mitral valve repair: Analysis of contemporary United States experience reported to the Society of Thoracic Surgeons National Cardiac Database; pp. 820–825. [PubMed: 12645700]
- Schectman JM, Schroth WS, Verme D, Voss JD. Journal of General Internal Medicine. 10. Vol. 18. 2003. Randomized controlled trial of education and feedback for implementation of guidelines for acute low back pain; pp. 773–780. [PMC free article: PMC1494929] [PubMed: 14521638]
- Shih C, Berliner E. Health Affairs. 6. Vol. 27. 2008. Diffusion of new technology and payment policies: Coronary stents; pp. 1566–1576. [PubMed: 18997213]
- Simon SR, Chan KA, Soumerai SB, Wagner AK, Andrade SE, Feldstein AC, Lafata JE, Davis RL, Gurwitz JH. Journal of the American Geriatrics Society. 2. Vol. 53. 2005. Potentially inappropriate medication use by elderly persons in U.S. health maintenance organizations, 2000–2001; pp. 227–232. [PubMed: 15673345]
- Simpson LA, Peterson L, Lannon CM, Murphy SB, Goodman C, Ren Z, Zajicek A. Health Affairs (Millwood). 10. Vol. 29. 2010. Special challenges in comparative effectiveness research on children's and adolescents' health; pp. 1849–1856. [PubMed: 20921485]
- Sittig DF, Wright A, Osheroff JA, Middleton B, Teich JM, Ash JS, Campbell E, Bates DW. Journal of Biomedical Informatics. 2. Vol. 41. 2008. Grand challenges in clinical decision support; pp. 387–392. [PMC free article: PMC2660274] [PubMed: 18029232]
- Soumerai SB, McLaughlin TJ, Gurwitz JH, Guadagnoli E, Hauptman PJ, Borbas C, Morris N, McLaughlin B, Gao X, Willison DJ, Asinger R, Gobel F. Journal of the American Medical Association. 17. Vol. 279. 1998. Effect of local medical opinion leaders on quality of care for acute myocardial infarction: A randomized controlled trial; pp. 1358–1363. [PubMed: 9582043]
- Stern M, Williams K, Eddy D, Kahn R. Diabetes Care. 8. Vol. 31. 2008. Validation of prediction of diabetes by the Archimedes model and comparison with other predicting models; pp. 1670–1671. [PMC free article: PMC2494666] [PubMed: 18509203]
- Stewart WF, Shah NR, Selna MJ, Paulus RA, Walker JM. Health Affairs. 2. Vol. 26. 2007. Bridging the inferential gap: The electronic health record and clinical evidence; pp. w181–w191. [PMC free article: PMC2670472] [PubMed: 17259202]
- Tinetti ME, Studenski SA. New England Journal of Medicine. 26. Vol. 364. 2011. Comparative effectiveness research and patients with multiple chronic conditions; pp. 2478–2481. [PubMed: 21696327]
- Tricoci P, Allen JM, Kramer JM, Califf RM, Smith SC. Journal of the American Medical Association. 8. Vol. 301. 2009. Scientific evidence underlying the ACC/AHA clinical practice guidelines; pp. 831–841. [PubMed: 19244190]
- Tunis SR, Stryer DB, Clancy CM. Journal of the American Medical Association. 12. Vol. 290. 2003. Practical clinical trials: Increasing the value of clinical research for decision making in clinical and health policy; pp. 1624–1632. [PubMed: 14506122]
- Tunis SR, Benner J, McClellan M. Statistics in Medicine. 19. Vol. 29. 2010. Comparative effectiveness research: Policy context, methods development and research infrastructure; pp. 1963–1976. [PubMed: 20564311]
- Undem T. Consumers and health information technology: A national survey. 2010. [August 21, 2012]. http://www
.chcf.org/∼ /media/MEDIA%20LIBRARY%20Files /PDF /C/PDF%20ConsumersHealthInfoTechnologyNationalSurvey.pdf. - VanSpall HGC, Toren A, Kiss A, Fowler RA. Journal of the American Medical Association. 11. Vol. 297. 2007. Eligibility criteria of randomized controlled trials published in high-impact general medical journals; pp. 1233–1240. [PubMed: 17374817]
- Vickers AJ, Scardino PT. Trials. Vol. 10. 2009. The clinically-integrated randomized trial: Proposed novel method for conducting large trials at low cost; p. 14. [PMC free article: PMC2656491] [PubMed: 19265515]
- Vogt TM, Elston-Lafata J, Tolsma D, Greene SM. American Journal of Managed Care. 9. Vol. 10. 2004. The role of research in integrated healthcare systems: The HMO research network; pp. 643–648. [PubMed: 15515997]
- Vos L, Dückers ML, Wagner C, vanMerode GG. Implementation Science. Vol. 5. 2010. Applying the quality improvement collaborative method to process redesign: A multiple case study; p. 19. [PMC free article: PMC2837614] [PubMed: 20184762]
- Wagner EH, Greene SM, Hart G, Field TS, Fletcher S, Geiger AM, Herrinton LJ, Hornbrook MC, Johnson CC, Mouchawar J, Rolnick SJ, Stevens VJ, Taplin SH, Tolsma D, Vogt TM. JNCI Monographs. 35. Vol. 2005. 2005. Building a research consortium of large health systems: The Cancer Research Network; pp. 3–11. [PubMed: 16287880]
- Walach H, Falkenberg T, Fønnebø V, Lewith G, Jonas WB. BMC Medical Research Methodology. Vol. 6. 2006. Circular instead of hierarchical: Methodological principles for the evaluation of complex interventions; p. 29. [PMC free article: PMC1540434] [PubMed: 16796762]
- Washington AE, Lipstein SH. New England Journal of Medicine. 15. Vol. 365. 2011. The Patient-Centered Outcomes Research Institute—promoting better information, decisions, and health; p. e31. [PubMed: 21992473]
- Weber GM, Murphy SN, McMurry AJ, Macfadden D, Nigrin DJ, Churchill S, Kohane IS. Journal of the American Medical Informatics Association. 5. Vol. 16. 2009. The Shared Health Research Information Network (SHRINE): A prototype federated query tool for clinical data repositories; pp. 624–630. [PMC free article: PMC2744712] [PubMed: 19567788]
- Wei F, Miglioretti DL, Connelly MT, Andrade SE, Newton KM, Hartsfield CL, Chan KA, Buist DS. Journal of the National Cancer Institute Monograph. 35. 2005. Changes in women's use of hormones after the women's health initiative estrogen and progestin trial by race, education, and income; pp. 106–112. [PubMed: 16287895]
- Weisberg HI, Hayden VC, Pontes VP. Clinical Trials. 2. Vol. 6. 2009. Selection criteria and generalizability within the counterfactual framework: Explaining the paradox of antidepressant-induced suicidality; pp. 109–118. [PubMed: 19342462]
- Wicks P, Vaughan TE, Massagli MP, Heywood J. Nature Biotechnology. 5. Vol. 29. 2011. Accelerated clinical discovery using self-reported patient data collected online and a patient-matching algorithm; pp. 411–414. [PubMed: 21516084]
- Woolf SH. Journal of the American Medical Association. 2. Vol. 299. 2008. The meaning of translational research and why it matters; pp. 211–213. [PubMed: 18182604]
- Woolley M, Propst SM. Journal of the American Medical Association. 11. Vol. 294. 2005. Public attitudes and perceptions about health-related research; pp. 1380–1384. [PubMed: 16174697]
- Wright A, Sittig DF. International Journal of Medical Informatics. 10. Vol. 77. 2008. A four-phase model of the evolution of clinical decision support architectures; pp. 641–649. [PMC free article: PMC2627782] [PubMed: 18353713]
- Yih WK, Caldwell B, Harmon R, Kleinman K, Lazarus R, Nelson A, Nordin J, Rehm B, Richter B, Ritzwoller D, Sherwood E, Platt R. Morbidity and Mortality Weekly Report. Suppl. Vol. 53. 2004. National bioterrorism syndromic surveillance demonstration program; pp. 43–49. [PubMed: 15714626]
- Zerhouni EA. New England Journal of Medicine. 15. Vol. 353. 2005. Translational and clinical science—time for a new vision; pp. 1621–1623. [PubMed: 16221788]
Footnotes
- 1
The number of journal publications was determined from searches on PubMed for 2010 (National Library of Medicine: http://www
.ncbi.nlm.nih.gov/pubmed/) using the methodology described in Chapter 2. - 2
In pragmatic clinical trials, the questions faced by decision makers dictate the study design (Tunis et al., 2003b). In delayed design trials, participants are randomized to either receive the intervention or have it withheld for a period of time, with both groups receiving the intervention by the end of the study (Tunis et al., 2010). In cluster randomized controlled trials, groups of subjects, rather than individual subjects, are randomized (Campbell et al., 2007).
- 3
Included in the American Recovery and Reinvestment Act, Public Law 111-5, 111th Congress (February 17, 2009).
- 4
Attribution denotes a clinician's use of social stereotypes or attributes to link certain diagnoses to certain patients (Blue Cross Blue Shield of Massachusetts Foundation, 2007). Availability bias occurs when memorable cases or frequent clinical phenomena influence a clinician's diagnosis. Anchoring is a cognitive shortcut in which the first piece of clinical information heard by the clinician has an undue influence on the clinician's thought process going forward.
- 5
Note that in Chapters 6-9, the committee's recommendations are numbered according to their sequence in the taxonomy in Chapter 10.
- Generating and Applying Knowledge in Real Time - Best Care at Lower CostGenerating and Applying Knowledge in Real Time - Best Care at Lower Cost
Your browsing activity is empty.
Activity recording is turned off.
See more...