NCBI Bookshelf. A service of the National Library of Medicine, National Institutes of Health.
National Academies (US) Committee on Measuring Economic and Other Returns on Federal Research Investments. Measuring the Impacts of Federal Investments in Research: A Workshop Summary. Washington (DC): National Academies Press (US); 2011.
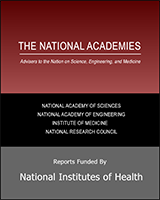
Measuring the Impacts of Federal Investments in Research: A Workshop Summary.
Show detailsIrwin Feller
Senior Visiting Scientist, American Association for the Advancement of Science and Professor Emeritus, Economics, Pennsylvania State University
I often say that when you can measure what you are speaking about, and express it in numbers, you know something about it; but when you cannot measure it, when you cannot express it in numbers, your knowledge is of a meager and unsatisfactory kind; it may be the beginning of knowledge, but you have scarcely in your thoughts advanced to the state of Science, whatever the matter may be.
-Baron William Thomson Kelvin.
When you can measure it, when you can express it in numbers, your knowledge is still of a meager and unsatisfactory kind.
-Jacob Viner
INTRODUCTION
Performance measurement is a politically powerful but analytical diffuse concept. Its meanings and implementation can vary from forcing fundamental changes in the ways in which public sector organizations and assessed and thus public funds allocated, as evinced by recent state government initiatives across all levels of U.S. education, to constituting old wine in new bottles, especially to empirically oriented economists, program evaluators and those weaned in the days of program-planning-budgeting.
Addressing this analytical diffuseness, this paper assesses the promises and limitations of performance measures as means of measuring economic and other returns of the Federal government’s investments in basic and applied research. Referring to promises and limitations in the same sentence implies differences in perspectives and assessments about the relevance, reliability, validity, transparency, and suitability of performance measures to guide decision making. These differences exist. A stylized dichotomization is as follows:
- endorsement of requirements for, belief in, scholarly search supportive of, and opportunistic provision of performance measures that respond or cater to executive and legislative branch expectations or hopes that such measures will facilitate evidence-based decision-making;
- research and experientially based assessment that even when well done and used by adepts, performance measures at best provide limited guidance for future expenditure decisions and at worst are rife with potential for incorrect, faddish, chimerical, and counterproductive decisions.
The tensions created by these differences are best captured by the observation of Grover Cleveland, 22d and 24th President of the United States: “It’s a condition we confront — not a theory.” The condition is the set of Congressional and Executive requirements upon Federal agencies to specify performance goals and to provide evidence, preferably in quantitative form, that advances towards these goals have been made. The set includes by now familiar legislation such as the Government Performance and Results (GPRA) Act of 1993, the Government Performance and Results Modernization Act of 2010, and requirements of the 2009 American Recovery and Reinvestment Act’s (ARRA) that Federal agencies provide evidence that their expenditures under the Act have stimulated job creation. It also includes comparable Executive branch directives. These include the Bush II Administration’s articulation in 2002 of R and D Investment Criteria, subsequent implementation of these criteria by the Office of Management and Budget (OMB) via its Performance Assessment Rating Tool (PART) procedures, and the Obama Administration’s 2009 OMB memorandum on Science and Technology Priorities for the FY 2011 Budget, that states that “Agencies should develop outcome-oriented goals for their science and technology activities…”, and “… develop science of science policy” tools that can improve management of their research and development portfolios and better assess the impact of their science and technology investments.” To these formal requirements may be added recent and likely increasing demands by congressional authorization and appropriations committees that agencies produce quantitative evidence that their activities have produced results, or impacts.
Theory here stands for a more complex, bifurcated situation, creating what Manski has termed dueling certitudes: internally consistent lines of policy analysis that lead to sharply contradictory predictions. (Manski, 2010). One theoretical branch is the currently dominant new public sector management paradigm branch. This paradigm emphasizes strategic planning, accountability, measurement, and transparency across all public sector functions, leading to, and requiring the use of evidence as the basis for informed decision making (OECD, 2005; Kettl, 1997).
The second branch is the accumulated and emerging theoretical and empirical body of knowledge on the dynamics of scientific inquiry and the processes and channels by which public sector support of research produces societal impacts. This body of knowledge performs a dual role. Its findings undergird many of the conceptualizations and expectations that policymakers have of the magnitude and characteristics of the returns to public investments in research and of the ways in which these returns can (or should) be measured. However, it is also a major source of the cautions, caveats, and concerns expressed by agency personnel, scientists, and large segments of the academic and science policy research communities that efforts to formally employ performance measures to measure public returns (of whatever form) to research and to then tie support for research to such measures are overly optimistic, if not chimerical, and rife with the potential for counterproductive and perverse consequences.
It is in the context of these differing perspectives that this paper is written. Its central thesis is that the promises and limitations of performance impact measures as forms of evidence relate to the decision-making context in which they are used. Context here means who is asking what type of question(s) with respect to what type of decision(s) and for what purpose(s). It also means the organizational characteristics of the Federal agency—can the activities of its operators be observed, and can the results of these activities be observed? (Wilson, 1989, pp. 158–171).
This emphasis on context produces a kaleidoscopic assessment, such that promises and limitations change shape and hues as the decision and organizational contexts shift. An emphasis on context also highlights the analytical and policy risks of assessing the promises and limitations of performance impact measures in terms of stylized characteristics. Performance measures for example can be used for several different purposes, such as monitoring, benchmarking, evaluation, foresight, and advocacy (making a case) (Gault, 2010). Consistent with the STEP-COSEPUP workshop’s stated objective to provide expert guidance to Federal policymakers in the Executive and Legislative branches about what is known and what needs to be better known about how to assess economic and other returns to Federal investments in science and technology—the paper’s focus is mainly on evaluation, although it segues at times into the other functions.
Approached in this way, performance is a noun, not an adjective. It also is a synonym for impact. This strict construction is made to separate the following analysis from the larger, often looser language associated with the topic in which performance is an adjective, as in the setting of strategic or annual (performance) goals called for by GPRA; as an indicator of current, changed or comparative (benchmarking) position, as employed for example in the National Science Foundation’s biennial Science and Engineering Indicators reports; or as symptomatic measures of the health/vitality/position of facets of the U.S. science, technology and innovation enterprise, as represented for example in Rising Above the Gathering Storm (2007), where they are employed as evidence that things are amiss or deficient —a performance gap—in the state of the world.
The paper proceeds in a sequential, if accelerated manner. Section II contains a brief literature review and an outline of the paper’s bounded scope. Section III presents a general discussion of the promises and limitations of performance measures to assess the impacts of Federal investments in research. Section IV illustrates the specific forms of the promises and limitations of performance measures in the context of what it terms the “big” and “small” questions in contemporary U.S. science policy. Section V offers a personal, “bottom line” perspective on what all this means.
Analytical Framework and Scope
The paper’s analytical framework and empirical findings derive mainly from economics, although its coverage of performance measures is broader than economic statistics and its treatment of impact assessment is based mainly on precepts of evaluation design. The choice of framework accords with the workshop’s objective, which is suffused with connotations of efficiency in resource allocation, or more colloquially, seeking the highest possible returns on the public’s (taxpayer’s) money. Adding to the appropriateness and relevance of the chosen approach is that many of the arguments on behalf of Federal investments in research, both basic and applied, draw upon economic theories and findings. As Godin has noted, “We owe most of the quantitative analysis of S and T to economists” (Godin, 2005, p. 3).
An immediate consequence of treating the workshop’s objective in this manner is that a goodly number of relevant and important subjects, policy issues, and analytical frameworks are touched upon only briefly, while others are ignored completely. Thus, only passing attention is taken of the historical, institutional and political influences that in fact have shaped and continue to shape the setting of U.S. national science priorities and Federal R and D budgets, whether viewed in terms of allocations by broad objectives, agencies, fields of science, or modes of support. Moreover, interpreting the workshop’s objective as a search for measures related to allocative efficiency obviously sidesteps topics and rich streams of research related to political influences on national research priorities (e.g., Hedge and Mowery, 2010) or which generate earmarks, set asides, and sheltered capacity building competitions that palpably diverge from efficiency objectives (e.g., Savage, 1999; Payne, 2006). Likewise omitted are consideration of the normative goals underlying Federal support of research and the distributive effects or societal impacts that flow from it (Bozeman and Sarewitz, 2011).
Another consequence is that the paper is primarily about performance measurement as a generic approach rather than about the reliability and validity of specific measures. Where reference is made to specific measures, it is to illustrate larger themes. In fact, there is no shortage of “metrics”, in GPRA-speak- to measure the outputs and outcomes of Federal investments in research. Geisler (2000; pps. 254–255) offers a well presented catalogue of 37 “core” metrics. These are organized in terms of immediate outputs (e.g., number of publications in refereed journals; number of patents); intermediate outputs (e.g., number of improved or new products produced; cost reductions from new and improved products/processes); pre-ultimate outputs (e.g., savings, cost reductions, and income generated by improved health, productivity, safety, and mobility of the workshop at sectoral and national levels); and ultimate outputs (e.g., improved GDP/capital; improved level of overall satisfaction and happiness of population.) The list is readily expanded to include combinations of single indicators, new data sets that permit disaggregation of existing measures, and new and improved versions of mainstream measures–the rapid and seemingly accelerating move from publication counts to citation measures to impact factors to h-indices and beyond being one such example.
Also in abundance are various scorecards or rankings based on assemblages and weightings of any number of performance measures related to scientific advance, technological advance, competitiveness, innovativeness, university performance, STEM-based educational proficiency and the like that have been used to position US performance within international hierarchies or norms. Indicator construction for science and technology has become a profession in its own stead, with regular international conferences—The European Network of Indicators Designers will hold its 2011 Science and Technology Indicators Conference in Rome, Italy, in September, 2011— and a well recognized set of journals in which new work is published.
Plentiful too and continuously being updated are compendia and manuals covering international best practice on how to evaluate public sector R and D programs. These works cover a wide range of performance impact measures and methodologies, including benefit-cost analysis, patent analysis, network analysis, bibliometrics, historical tracings, innovation and on the outputs produced by several different Federal agencies—health, energy, agriculture, environmental protection, international competitiveness, employment. (For recent overviews, see Wagner and Flanagan, 1995; Ruegg and Feller, 2003; Godin, 2005, chpt. 15; Kanninen and Lemola, 2006; Grant, et.al, 2009; Foray, 2009; Gault, 2010; Link and Scott, 2011).
Finally, in setting expectations for the workshop, it is perhaps helpful to note that the topics and issues to be discussed are not new ones. Rather, they form the substance of at least 60 years of theoretical, empirical and interpretative work, producing what by now must be a five foot high stack of reports and workshop proceedings, including a sizeable number originating under National Academies’ auspices. The recurrent themes addressed in this previous work, evident since the program-planning-budgeting initiatives of the 1960s and continuing on through its several variants, are a search for decision algorithms that will lead to the improvement in government budgeting and operations and a search for criteria for setting priorities for science (Shils, 1969). Noting these antecedents is not intended to diminish the importance of current activities (nor, for that matter, of this paper). Instead, it is to suggest the complexities of the issues under consideration and as a reminder of the richness and contemporary relevance of much that has been written before.
Performance Impact Measures
Differences in assessments about the potential positive and negative features of requiring strategic plans and performance measures into how Federal agencies set research priorities and assessed performance were visible at the time of GPRA’s enactment. They continue to this day.1
In 1994, almost immediately after GPRA’s passage, I organized a session at the American Association for the Advancement of Science’s (AAAS) Colloquium on Science and Technology Policy on the applicability of GPRA to budgeting for science and technology. Taking a “neutral” stand on the subject, I invited, among other panelists, Robert Behn, a leading scholar of and advocate for the new public management paradigm subsumed within GPRA and like requirements, and Paul David, a leading researcher in the economics of science and technology.
The title of Behn’s talk captured its essence: “Here Comes Performance Assessment-And it Might Even be Good for You.” (Behn, 1994). Among the several benefits (or promises) cited by Behn were the following:
Having objectives (“knowing where you want to go”) is helpful; Objectives provide useful baseline for assessing each of 4 modalities of accountability–finance, equity, use of power and performance.
Well defined objectives and documentation of results facilitate communication with funders, performers, users, and others.
For his part, David outlined what he termed “very serious problems…with outcome goal setting for federal programs in general and for research in particular” (David, 1994, p. 294). David’s central argument was that an “outcome reporting may have a perverse effect of distorting the perception of the system of science and technology and its relationship to economic growth” (ibid, p. 297). He further observed that “ Agencies should define appropriate output and outcome measures for all R and D programs, but agencies should not expect fundamental basic research to be able to identify outcomes and measure performance in the same way that applied research or development are able to.” What follows is essentially an expanded exposition of these two perspectives, presented first as promises and then as limitations.
Promises
- Performance measurement is a (necessary) means towards implementing (and enforcing) the audit precepts – especially those linked to accountability and transparency–contained within GPRA and like requirements.
- Performance measures can assist agencies make improved, evidence-based decisions both for purposes of program design and operations (formative evaluations) and longer term assessments of allocative and distributive impacts ( summative evaluations). In these ways, performance measures assist agencies in formulating more clearly defined, realistic, and relevant strategic objectives and in better adjusting ongoing program operations to program objectives.
- Well defined, readily measured, and easily communicated performance measures aids both funders and performers to communicate the accomplishments and contributions of the public investments to larger constituencies, thereby maintaining and strengthening the basis of long term public support of these investments.
- The search for measures that accurately depict what an agency/program has accomplished may serve as a focusing device, guiding attention to the shortcomings of existing data sets and thus to investments in obtaining improved data.
- Performance measurement focuses attention on the end objectives of public policy, on what has happened or happening outside the black box, rather than on the churning of processes and relationships inside the black box. This interior churning produces intermediate outputs and outcomes (e.g., papers, patents) that may be valued by performers (or their institutions, stakeholders, or local representatives), but these outputs and outcomes do not necessarily connect in a timely, effective, or efficient manner to the goals that legitimize and galvanize public support.
- Requiring agencies to set forth explicit performance research goals that can be vetted for their societal importance and to then document that their activities produced results commensurate with these goals rather than some diminished or alternative set of outputs and outcomes is a safeguard against complacency on the part of funders and performers that what might have been true, or worked in the past, is not necessarily the case today, or tomorrow. Jones, for example, has recently noted, “Given that science is change, one may generally imagine that the institutions that are efficient in supporting science at one point in time may be less appropriate at a later point in time and that science policy, like science itself, must evolve and continually be retuned” (Jones, 2010, p. 3). Measurement of impacts is one means of systematically attending to the consequences of this evolution.
- Performance measurement is a potential prophylactic against the episodic cold fusion-type viruses that have beset the formulation of U.S. science policy. As illustrated by the continuing debates set off by Birch’s claims on the disproportionate role of small firms as sources of job creation (cf. Haltiwanger, J., R. Jarmin, and J. Miranda (2010)) or the challenge posed to the reflexive proposition that the single investigator mode of support is the single best way to foster creative science by Borner, et.al. findings that “Teams increasingly dominate solo scientists in the product of high-impact, highly cited science; (Borner, et. al. 2010, p. 1), U.S. science and innovation policy contains several examples of Will Roger’s observation that, “It isn’t what we don’t know that gives us trouble, it’s what we know that ain’t so.”
- Presented as a method of assessing returns to Federal investments in research, performance measurement provides policymakers and performers with an expanded, more flexible and adaptable set of measures than implied by rate of return or equivalent benefit-cost calculations. Criticism of what is seen as undue reliance on these latter approaches is longstanding; they are based in part on technical matters, especially in the monetization of non-market outputs, but also on the distance between the form that an agency’s research output may take and the form needed for this output to have market or other societal impacts.
The largest promise of performance measurement, though, likely arises not from recitation of the maxims of the new public management but from the intellectual ferment now underway in developing new and improved data on the internal processes of scientific and technological research, the interrelationships of variables within the black box, and improved methods for assembling, distilling and presenting data. Much of this ferment, of course, relates to Dr. Marburger’s call for a new science of science policy, the activities of the National Science and Technology Committee’s (NSTC) Committee on Science, and the research currently being supported by the National Science Foundation’s Science of Science and Innovation Policy program (SciSIP). No attempt is made here to present a full précis of the work underway (Lane, 2010). Having been a co-organizer, along with Al Teich, of two AAAS workshops at which SciSIP grantees presented their preliminary findings and interacted with Federal agency personnel, however, it is a professional pleasure to predict that a substantial replenishment and modernization of the intellectual capital underlying existing Federal research policies and investments can be expected.
To illustrate though the nature of recent advances, I cite two developments non-randomly selected to reflect the focus of my own research interests. They are the NSF’s Business R and D and Innovation Survey (BRDIS), itself in part redesigned in response to the 2005 NRC study, Measuring Research and Development Expenditures in the U.S. Economy, and advances in the visualization of the (bibliometric) interconnections of disciplines. The NRC report articulated longstanding concerns that NSF’s existing survey of industrial R and D needed methodological upgrading, lagged behind the structure of the U.S. economy in not adequately covering the growth of the service sector or the internationalization of sources and performers of R and D, and did not adequately connect R and D expenditures with downstream “impact” measures, such as innovations. The result has been a major revision of these surveys, undertaken by NSF’s Science Resources Statistics Division.
Early findings from the new BRDIS survey on the sources and characteristics of industrial innovation fill a long recognized data gap in our understanding of relationships between and among several variables, including private and public R and D expenditures, firm size and industrial structure, human capital formation and mobility, and managerial strategies. (Boroush, 2010). Combined with pending findings from a number of ongoing SciSIP projects and juxtaposed to and compared with data available from ongoing international surveys, these newly available data hold promise of simultaneously providing policymakers with a finer grained assessment of the comparative and competitive position of the technological performance of the U.S. economy and researchers and evaluators finer grained data to assess the impacts of selected science and technology program and test existing and emerging theories.
Science is a set of interconnected, increasingly converging disciplines, so run the claims of many scientists (Sharp, et. al, 2011). But precisely in what ways and with what force do these interconnections flow? Does each field influence all other fields and with equal force, or are there discernible, predictable differences in patterns of connection and influence? Prospectively, being able to answer these questions would provide policymakers with evidence about relative priorities in funding fields of science, presumably giving highest priority to those that served as hubs from which other fields drew intellectual energy. Recent research in data visualization, illustrated by Boyack, Klavans, and Borner’s Mapping the Backbone of Science (2005), combines bibliometric techniques, network theories, and data visualization techniques to offer increasingly accessible “maps” of the structure of the natural and social sciences, thereby providing one type of answer to these questions.
Limitations
The above noted emphasis on context surfaces immediately in considering the limitations of performance measurement. Perhaps the most obvious and important difference in the use of such measures in between ex ante and ex post decision making settings. Fundamental differences exist in the theoretical, analytical and empirical knowledge bases for using performance measures to determine whether past investments have produced the research expected of them and using such measures to decide upon the magnitude and direction of new funds.
If retrospective assessment was all that was implied by the call for performance measures of impacts, the task before this audience, and for Federal science agencies in satisfying new planning and reporting requirements, while challenging, especially in reconciling and distilling divergent, at times conflicting findings, as say in the cases of the Bayh-Dole Act (Larsen, 2010; NRC, 2010) or the SBIR program’s generation of sustainable increases in employment, would at least be relatively straightforward. There is no shortage of well crafted assessments of past Federal investments in basic and applied research. Such work has been and continues to be a staple component of research on the economics of science and technology and of previous NRC reports over the past 50 years. A short list would include the rich empirical literatures on the returns to Federal investments in agricultural research (Evenson, Ruttan, and Waggoner, 1979; Heisey, et. al, 2010), biomedical research (Murphy and Topel, 2006; Stevens, et. al, 2011), energy efficiency research (Link, 2010), and applied technology programs, such as NIST’s Advanced Technology Program (Ruegg and Feller, 2003).2
Manifestly though, more than an assessment of past investments as a possible guide to future decisions is intended in recent requirements and continuing calls for measures of performance impact for research. The central premise underlying the mantra of evidence-based decision making is that some combination of findings about the impacts from previous findings or findings from some form of in situ or heuristic experiment provides the best possible predictor of the expected impacts that will follow upon Federal research expenditures. This is a far different matter than assessing the impacts of past research expenditures.3 The premise though must confront considerable research‐based agnosticism of many scholars of the extent to which findings based on past studies can be used to forecast the specific magnitude and characteristics of future Federal investments in research. As noted by Crespi and Guena, for example, “After more than 50 years scholarly work on the importance of academic research, there is still little systematic evidence on how such investments can lead to increase levels of scientific output, improved patenting and innovative output, better economic performance and, ultimately, to increase national wealth” (Crespi and Geuana, 2008, p. 555).
The primary limitation of using performance measures to shape future Federal investments in research flows from the well documented tale, widely recounted in both the scholarly and policy literatures, that the outcomes of scientific research are unpredictable as to when they will occur, who will be responsible for them, and even more so with respect to their end uses. This last influence appears to be of increasing importance in confounding projections of returns to future Federal research investments as ‘users” become increasingly influential in transforming platform scientific findings or technological advances into their own new products and processes (von Hippel, 2005).
Additionally, again to restate familiar propositions, the impacts of basic and applied research occur only over extended periods of time, often extending beyond budgetary and planning horizons. They also frequently require further “investments”—downstream in terms of prototype development, manufacturability, and marketing–and upstream, in terms of related scientific discoveries or technological breakthroughs– before their impacts are felt. Many, if not most of these necessary complementary activities are outside the purview of the agencies funding the research.
To cite two examples from the literature on the economics and history of science and technology that express these propositions. First, Rosenberg: “From an economic point of view, perhaps the most striking peculiarity of knowledge production is that is not possible to establish the nature of its production function. We can never predict the output which will be generated by a given volume of inputs. By its very nature knowledge production deals with forays into the unknown. It involves the combination of resources to an exploratory process the outcome of which may be a large number of dead ends rather than the hoped-for-discovery of knowledge or techniques possessing profitable economic applications.” (Rosenberg, 1972, p. 172). Second, Mowery and Rosenberg: “It is essential to emphasize the unexpected and unplanned, even it-or especially if-it renders serious quantification impossible. In fact, the difficulties in precisely identifying and measuring the benefits of basic research are hard to exaggerate” (Mowery and Rosenberg, 1989, p. 11).
These assessments are widely shared. To cite an earlier National Academies endeavor not unlike today’s, “History…shows us how often basic research in science and engineering leads to outcomes that were unexpected or took many years or even decades to emerge…The measures of the practical outcomes of basic research usually must be retrospective and historical and…the unpredictable nature of practical outcomes is an inherent and unalterable feature of basic research” (National Academies, 1999). They are also found in Executive budget documents. For example, although suffused with an emphasis on quantitative performance measures, OMB’s earlier articulation of R and D Investment Criteria expressed nuanced understanding of the uncertainties surrounding returns from Federal investments in basic research: “Agencies should define appropriate output and outcome measures for all R and D programs, but agencies should not expect fundamental basic research in the same way that applied research or development are able to do. Highlighting the results of basic research is important, but it should not come at the expense of risk-taking and innovation” (OMB, PART 2008, Appendix C, p. 76).
To briefly illustrate these propositions with some specifics, the above tale is well told by the historical linkages between and among the work of physicists, Pauli, Purcell, and Bloch in identifying and measuring nuclear magnetic resonance (NMR); the use of NMR by chemists to determine the structure of molecules; the sequential development by Varian of increasingly more user-friendly NMR machinery, and the subsequent, still contested priority race between supporters of Damadian and Lauterbur to apply NMR to medical imagining, along with a host of other advances in mathematics, computer science, and technologies, a number of which originated with firms such as EMI in the United Kingdom and GE in the U.S., leading to the now ubiquitous presence of MRI (Kelves, 1997; Roessner, et. al, 1997).
My personal favorite example of the meanderings of new scientific and technological knowledge into uses not anticipated by those funding or performing the underlying research is the answer I received from Penn State undergraduates enrolled in my course in science and technology in the pre-IPod, circa 2000 period, when asked to identify the most pressing national S and T policy issue. The overwhelming response was the then legal imbroglio relating to downloading Napster files. So much for DARPA and the search to link high-end, computing-intensive research.
The limitations of performance measures as forms of evidence to guide investments in research extend beyond this general case. There are other specific limitations that arise in or bear upon specific decisions in specific contexts. A partial listing of them is as follows:
- Performance measures for research may undervalue lack of performance, or failure. Science has been described as the only field where failure is to be expected. After all, it was Edison who when challenged with the number of failed experiments rejoined, “I have not failed. I’ve just found 10,000 ways that won’t work.” Indeed, given the well known skewness of the distribution of research findings, at least as measured by bibliometric data, recent agency initiatives to promote high-risk, high-reward research if interpreted formally implies increased frequency of projects that fail to achieve their stated objectives.
- There is an implied but at times illusive exactitude in first speaking about the promise/benefits of performance measurement and then moving to the selection and operationalization of specific performance measures. As illustrated by current national debates over the specification of performance measures in K-12 and higher education, the transition is seldom that simple. For example, is the performance of public colleges, and thus their state appropriations under a system of performance based budgeting, to be based on graduation rates, time to degree, mastery of general knowledge, mastery of specialized knowledge, or life time earnings, preferably within state borders? These measures reflect different concepts of performance, several of which point provide different sets of incentives for university administrators and faculty. Alternative articulations of performance likewise are subsumed within the global objectives set for Federal research programs–productivity increase, for example, is not synonymous with job creation.
- Leaving aside issues associated with data availability and quality, the casual linkages between program/agency objectives and the choice of measures to be used can be fuzzy. Empirically, reservations can be expressed about the metrics that agencies have to date employed and been accepted by OMB to document agency performance. My earlier brief of the performance metrics contained in OMB’s PART review pointed to a diverse set of measures across agencies and programs. Some related to technical specifications (i.e., achieve a certain level of performance advance), some to economic gains (threshold and above benefit-cost ratios), some to societal impacts (e.g., reduction in traffic fatalities), and more. No clear analytical or empirical distinction though seemed to be made between what seemed in some cases to be final impacts and in others to be intermediate impacts. There is an admitted logic to this variability. Agencies differ not only in objectives, but in the technical ease with which it is possible to measure performance relative to these objectives. It is easier to measure rates of return to commodity-oriented agricultural research, where market data on inputs and outputs are readily available, than to investments in research on particle physics. But the hodgepodge of performance measures in use undercuts any attempt to systematically compare performance across agencies in formulating research budgets. In practice, the specification of the measures to be used, as well as the target to be reached, likely are the outcome of some form of negotiation and compromise between OMB and the agency, perhaps with some formal or informal understanding of what is acceptable to the relevant congressional committee. This is conjecture, though, awaiting confirmation. At present, the specification of performance measures across agencies and programs should be viewed as a new policy and research black box.
- In a circular process, unless a program’s objective is defined in terms of a single performance measure, any single measure is at best a partial indicator of the objective being pursued. In most cases, single performance measures are only loosely connected to higher order performance objectives. Moreover, employment of single measures can produce findings that incorrectly suggest that the objectives for which research support is being provided are not being met, and thus be misleading guides to public policy. Hill’s 1986 study, undertaken for the House Committee on Science and Technology, of the relationship between US Nobel Prize awards and aggregate U.S. performance objectives in economic growth and health highlights these risks (U.S. Congress, 1986; p.65). As illustrated in Figure C-1, Hill’s study, undertaken at the height of angst about U.S. international economic competitiveness, shows a negative relationship in growth in gross domestic product and national Nobel Prize awards in physics and chemistry!

FIGURE C-1
Growth in Gross Domestic Product and Nobel Prize-winning in Physics and Chemistry.
Connecting U.S. performance in Nobel prizes to health outcomes produces only a slightly better “picture.” The mapping of Nobel prizes in physiology and medicine for the period 1945–1984 with health statistics shows a slight positive relationship in reductions in infant mortality but no apparent association to gains in life expectancy. Compare this use of this single performance measure with Cutler and Kadiyala’s estimate that an average 45-year old in 1994 had a life expectancy 4 ½ years longer than in 1950 because cardiovascular disease mortality had decreased. This finding leads them to the “unambiguous conclusion …that medical research on cardiovascular disease is clearly worth the cost” (2002, p.113). Cast in benefit-cost terms, this increase is estimated to yield a 4 to 1 return for medical treatment and a 30-to-1 return for research and dissemination costs related to behavioral change.
More generally, to the extent that single measures are used, they become the de facto criterion by which performance is judged, and in a system of performance-based budgeting the basis on which decisions about which future funding is made. Measures, though, are means to ends. As the recent if by now jaded maxim put it, if you can’t measure it, you can’t manage it. But measures also can shape the ends. What is measured is what is managed, or promised. The value of impacts not measured is thereby diminished, or ignored. More tellingly, what is measured is what is produced if measured performance is tied to resources or rewards of whatever form.
An obvious implication of the vignettes from health research is the risk of using any single performance measure to gauge the impacts of Federal investments in research. This proposition is stated so frequently and explicitly in contemporary exegesis on assessment of science programs (Schmoch, et. al, 2010) that it would not be worth mentioning, except that it is frequently ignored. Thus, the contributions of a university’s research activities to national or state level economic growth are often reduced to counts of numbers of patents or licenses, or even worse to the ratio of patents to external R and D funding, while the job creation expectations associated with the research funding in ARRA have taken on a life of its own, creating an assessment cynosure about that performance measure to the exclusion of other, possibly broader and deeper impacts (Goldston, 2009).
- Most federal research programs, though, have multiple rather than single objectives. Multiplicity creates its own set of problems for use of performance measures on impacts. It increases the prospects for non-commensurable findings because of intractable technical issues of measuring things that are heterogeneous within a single comprehensive measure and because of the implicit normative weighting accorded different objectives (Gladwell, 2011). The presence of multiple objectives also increases the likelihood of variable performance (satisfactory, unsatisfactory; results not proven) among them, leaving open or requiring normative assessments about relative weights. The setting also is rife with the prospects of strategic retreats on objectives, so that performance becomes measured in terms of what an agency/program can produce, not what it was set up to produce. Moving to multiple measures, as in scorecards, also raises questions of possible co-linearity among seemingly independent measures, so that what seems to be the richness of the approach in effect reduces to variants of a single measure. Perhaps most importantly, the presence of multiple objectives for a program increases the likelihood of trade-offs among facets of performance, so that an increase in one agency/program objective can be achieved only at the expense of a decrease in another objective. A substrata tension, or inconsistency, thus seems to exist between the simultaneous pursuit of multi-item performance measurement scorecards and acceptance of the organizational mantra that one can’t be all things to all people.
- Effectiveness and efficiency are different concepts. This difference is frequently overlooked in interpreting measures of the performance of Federal programs. It is one thing to say that a program has produced positive outcomes with respect to one or more of its several stated objectives; it is another to say that it is achieving these outcomes in the most efficient manner relative to the next best uses to which its program funds could have been or could be put. It is axiomatic that any large scale Federal program, research or otherwise, especially if longstanding, will have many accomplishments to report. A corollary is that the larger and longer standing the program, the larger the absolute number of outputs.The potential consequences for misinterpreting evidence and subsequent questionable decisions when effectiveness and efficiency are confounded takes on special importance in light of the recent OMB memorandum, “Increased Emphasis on Program Evaluations.” The memorandum states that “Rigorous, independent program evaluations can be a key resource in determining whether government programs are achieving their intended outcomes as well as possible and at the lowest possible cost.” It also notes that, “And Federal programs have rarely evaluated multiple approaches to the same problem with the goal of identifying which ones are most effective.” Absent some form or control or comparison group or other explicit standard, performance measures provide little basis for determining a program’s cost-effectiveness or efficiency.
- The informational content of performance measures may change over time. This may result from employing measures in different ways than they had formerly been used, especially if a different set of incentives is attached to them—faculty member’s patenting of their research shifting over time in promotion and tenure reviews from a negative indicator of distraction from disinterested Bohr- cell research to a positive indicator of fulfillment of a university’s “third mission” objectives in promotion and tenure packets. It may also change as a result of legislative or court decisions and/or firm strategies in the uses made of the measured activity- reported shifts, for example, in increased patent activities by firms and the trend towards using them as a source of revenue as well as a means of protecting intellectual property (Cohen, 2005). Freeman and Soete formalize and label this changing informational content as the science, technology, and innovation (STI) version of Goodhart’s law: “once STI indicators are made targets for STI policy, such indicators lose most of the informational content that qualify them to play such a role” (2009, p. 583). Along similar lines, Moed, in his review of bibliometric indicators has noted the argument that indicators applied in research performance assessment should be modified every ten years or so, replacing indicators normally applied by new types (Moed, 2005, p. 320).
The Use of Performance Measures in Federal Research Policy Decisions
Context matters critically as one attempts to relate the above characteristics of performance measures to the type of decisions that U.S. policy makers are called upon to make with respect to Federal investments in research. The applicability and thus promise and limitations of performance measures, singly or collectively, can vary greatly from holding high potential for providing useful information on program and project level activities to low, problematic and potentially counterproductive for overarching decisions concerning levels and broad allocation patterns of Federal support.
Schematically and historically, U.S. science and technology policy has consisted of a continuing discourse between a stock of big questions and a comparable stock of big answers. This discourse underpins the continuity of the main features of U.S. policy, albeit with short-term economic and political perturbations. It also provides the intellectual and policy capital base for consideration of a continuing flow of smaller questions and smaller answers about specific science and innovation policy issues. These latter issues flare up to dominate near-term science policy forums, and then through some amalgam of a modicum of resolution, overtaking by the eruption of new policy agenda items, or by morphing into the big questions lose their immediate saliency, only to pop again anew, not infrequently with new terms being used to describe recurring questions.
The big three science policy questions, in the U.S. as well as elsewhere, are (1) the optimal size of the federal government’s investments in science and technology programs; (2) the allocation of these investments among missions/agencies/and programs and thus fields of science; and (3) the selection of performers, funding mechanisms, and the criteria used to select projects and performers.4 Permeating each of these questions is the question of “why,” namely, the appropriate, effective and efficient role of the Federal government in supporting public investments in science and technology including nurturance of a STEM-qualified labor force. Allowing for variations in language and precipitant events, the questions are strikingly unchanged between the 1960s ferment on criteria for scientific choice and those posed by Dr. John Marburger’s call for a new science of science policy. Only the first and second questions are treated here.
The big answers to these questions appear in the sizeable and ever more sophisticated theoretical, descriptive and empirical literature that has dealt with these topics since at least the 1960s. These answers form the basis for statement in the NSTC’s 2008 report, The Science of Science Policy: a Federal Research Roadmap termed, a “…well developed body of social science knowledge that could be readily applied to the study of science and innovation.”
The big answers, in summary form, relate to: (1) The contributions of productivity increase to increases in GDP/capita. This answer is the intellectual and policy legacy of a continuing stream of work from Solow-Abramovitz, through the growth accounting work and debates of economists such as Denison, Jorgensen, Baumol, more recently recast in terms of endogenous growth theory and spillover effects. Thus, the opening paragraph of the National Academies highly influential report, Rising Above the Gathering Storm, states that “Economic studies conducted even before the information-technology revolution have shown that as much as 85% of measured growth in U.S income per capital was due to technological change.” (2) Market failure propositions associated with the work of Arrow and Nelson that competitive markets fail to supply the (Pareto-) optimal quantity of certain types of R and D5 and (3) Mansfield-Griliches-type analytical frameworks, augmented increasingly with attention to knowledge spillovers, based on divergences between social and private rates of return to R and D, that have been used to justify Federal investments across a swathe of functional domains - health, agriculture, environmental protection.
Historically, these big answers have become the ideas of academic scribblers that influence those in power in power today, or least most of them. They have contributed to shaping a broad political consensus, ranging from President Obama to George Will, about the appropriateness, indeed necessity, of Federal support of basic research, at the same time leaving in dispute the legitimacy of Federal support for technologically oriented civilian-oriented research programs.
Because these answers are so much a part of contemporary discourse, it is easy today to lose sight of their transformative impacts. These answers invert most of core tenets of pre-1950 Federal science and technology policy in which support was provided for mission oriented, applied research but not for basic research (DuPree, 1957). Likewise, the current major role of U.S. universities as performers of Federally funded basic research–a role today much valued, extolled and defended by these institutions–had to overcome fears expressed by leaders of the National Academy of Sciences about “government interference with the autonomy of science…” (Geiger, 1986, p. 257).
But even as the broad policy propositions derived from the big answers remain essentially correct in shaping the overall contours of US science policy, they are seen as of limited value by decision makers because in their view the answers do not correspond to the form in which they confront decisions about how much to investment in research and how these investments should be allocated among national government objectives, fields of science, and agencies (Feller, 2009).
For example, in addressing the first overarching question of how much the Federal government should spend in the aggregate to R and D, abstracting here from thorny analytical, measurement, and institutional issues involved in linking R and D with technical change and/or productivity change, that the business sector funds two-thirds of US R and D, and that total Federal R and D expenditures are the sum of multiple House and Senate appropriations’ bills, how does one move from the 85% share of growth in per capita income attributed to technological progress contained in Rising Above the Gathering Storm to findings such as Boskin and Lau’s estimate that 58% of the economic growth of the United States between 1950–1998 was attributable to technical progress to determining the optimal R and D/GDP ratio? How would the optimal level of Federal expenditures on R and D change if new findings suggested that existing estimates overstated the contribution of technical progress by 20/30 percentage points, or conversely, understated this contribution by a like amount?
Using a different performance measuring stick, given candid assessments from European officials that the European Union’s 2000 Lisbon Strategy’s 3% goal was a political rather than an economic construct, what’s the empirical basis of the Obama Administration’s 3% goal? Achieving, or surpassing the goal would reverse declines in real terms in Federal support of R and D since 2004 (Clemins, 2010), and raise the ratio from its current estimated level of about 2.8%. Achieving this goal would also move the US closer to the top of all other OECD nations, even possibly overtaking Finland or Sweden (Boroush, 2010). But other than asserting that more is better than less, what other basis exists for determining whether 3% is too high, too low, or just right? Given all the above cited reservations about the complexity of linking public sector research expenditures to desired outcomes, how can performance measures be used to exist to judge the merit of recent proposals that the U.S. should be spending 6 %, not 3% of GDP on R and D (Zakaria, 2010)?
Similar questions arise when or if one attempts to start from treating science not as the handmaiden of economic growth but having its own internal dynamics. The cover page of the 125th anniversary issue of Science, 1 July 2005, is titled: 125 Questions: “What Don’t We Know?” Assume, as seems appropriate, that these questions accurately represent the current frontiers of knowledge, that advances in many if not all directions at the frontier hold the promise of societal benefits, and that excellent proposals addressing each question are awaiting submission to the relevant Federal agency. What would be the total cost? What share of GDP or of the Federal budget would be required to fund these proposals once added to other national or mission agency R and D priorities? If not all these proposals could be funded, what means should be used to select from among them? What measures of performance/output/outcomes should be used to assess past performance in determining-out year investments or near-term R and D priorities. Exciting as it may be to envision the prospects of societal impacts flowing from frontier, high-risk, transformative risk, it serves only to bring one full circle back to the policymaker’s priority setting and resource allocation questions noted above.
The same issues arise when trying to compute the proper level of support or estimate the returns to public investments for functional objectives, agencies, and fields of science. An impressive body of research, for example, exists on the contributions to the health status of the American population produced by Federal investments in biomedical research. It’s an analytical and empirical stretch to say that this research provides evidence that can be used to determine whether current or proposed levels of appropriation for NIH are right, too little, or too high. No evident empirical basis existed for the doubling of NIH’s budget over a 5-year time period, and the consequences now observed while unintended were not unpredictable (Freeman and van Reenan, 2008). At issue here is what Sarawitz had termed the myth of infinite benefits: “If more science and technology are important to the well-being of society, then the more science and technology society has, the better off it will be” (1996; p. 18). Indeed, arguably, if the budget decision had any lasting impacts, it was to elevate “balance” of funding across agencies as a resource allocation criteria and to set doubling as a formulaic target for other science oriented agencies.
Similar problems arise too in attempting to formulate analytically consistent criteria based on performance measures for allocating funds among fields of science and technology, — how much for chemistry?; physics?; economics?- especially as among national objectives and agencies, as well as within agencies. These are the perennial practical questions across the spectrum of Federal science policymakers, yet perhaps with the exception of basing program level allocations on estimated returns from impacts, as in the cases of agriculture (Ruttan, 19820 and health (Gross, Anderson, and Power, 1999) for which few good answers, or funding algorithms, exist. For example, a recent NRC panel tasked with just such an assignment concluded in its report, A Strategy for Assessing Science, “No theory exists that can reliability predict which research activities are most likely to lead to scientific advances or to societal benefits” (2007, p. 89).
One would like to do better than this. Here, if anywhere, is where performance measurement may have a role. The challenge at this point is not the absence of performance measures relating Federal investments in research to specific outputs or studies pointing to high social rates of return within functional areas but the sheer number of them and the variations in methodologies that produce them. The result is a portfolio of options about performance measures, each more precisely calibrated over time but still requiring the decision maker to set priorities among end objectives.
Thus, the Boyack, et. al, bibliometric study cited above highlights the “centrality” of biochemistry among published papers. Using this study and its implied emphasis on scientific impact as a basis for resource allocation decisions among scientific fields would presumably lead to increased relative support for biochemistry. If one instead turns to the Cohen-Nelson-Walsh survey-based study (2002) of the contributions of university and government laboratory research i.e., (“public”) research to industrial innovation, which contains an implied policy emphasis on economic competitiveness, one finds both considerable variation across industries in the importance of public research and variations in which fields of public research are cited as making a contribution. An overall finding though is that, “As may be expected, more respondents consider research in the engineering fields to contribute importantly to their R and D than research in the basic sciences, except for chemistry” (2002, p. 10). The authors however mute this distinction of the relative contribution of fields of science with the caution that “the greater importance of more applied fields does not mean that basic science has little impact, but that its impact may be mediated through the more applied sciences or through the application of industrial technologists’ and scientists’ basic scientific training to the routine challenges of conducting R and D” (p. 21). But the upshot of the study still would seem to be the need for increased (relative) support of engineering related disciplines. Advocates for increased Federal research for computer science and engineering, for their part may turn to Jorgenson, Ho, and Samuels’ recent estimates of the contribution of the computer equipment manufacturing industry to the growth in US productivity between 1960–2007 (Jorgenson, Ho, and Samuels, 2010).
An obvious conclusion, indeed the standard one in discussion of this issue, is that the interconnectedness of fields of science requires that each be supported. And this of course is how the present U.S. system functions. There are considerable differences, however, between funding each field according to its deeds and each according to its needs. Moreover, the interconnectedness argument applies to historical determinants and levels of support; it is of limited guidance in informing budget decision—show much more or less, given existing levels of support?
Little of this should be a surprise. The gap between estimates of returns to public investments in research and using these estimates to formulate budget allocations among missions, agencies, and disciplines was identified by Mansfield in the opening text of the social returns to R and D. Referring to the number of independent studies working from different models and different data bases that have pointed to very higher social rates of return, he noted, “But it is evident that these studies can provide very limited guidance to the Office of Management and Budget or to the Congress regarding many pressing issues. Because they are retrospective, they shed little light on current resource allocation decisions, since these decisions depend on the benefits and costs of proposed projects, not those completed in the past” (Mansfield, 1991, p. 26). The gap has yet to be closed.
Similar issues arise in using bibliometric data to allocate resources across fields. Over the last three decades, even as the U.S. position in the life sciences has remained strong, its world share of engineering papers has been cut almost in half, from 38 percent in 1981 to 21 percent in 2009, placing it below the share (33 percent) for the EU27. Similar declines in world share are noted for mathematics, physics, and chemistry (National Science Foundation, 2007; Adams and Pendlebury, 2010). One immediate, and simple interpretation of these data is that aggregate bibliometric performance is a function of resource allocation: a nation gets what it funds. But this formulation begs first the question if what it is producing is what it most needs, and then if what it is producing is being produced in the most efficient manner.
Conclusion
Having studied, written about, participated in, organized workshops on, and as an academic research administrator been affected by the use of performance measures, something more than an “on the one hand/on the other hand” balance sheet, a concluding section seems in order.
It’s simpler to start with the limitations of performance measures for they are real. These include the attempt to reduce assessment of complex, diverse, and circuitously generated outcomes, themselves often dependent on the actions of agents outside the control of Federal agencies, to single or artificially aggregated measures; the substitution of bureaucratically and/or ideologically driven specification and utilization of selective measures for the independent judgment of experts; and the distortion of incentives for science managers and scientists that reduces the overall performance of public investments. To all these limitations must be added that to date there is little publically verifiable evidence outside the workings of OMB-agency negotiations that implementation of a system of performance measurement has appreciably improved decision making with respect to the magnitude or allocation of Federal research funds. When joined with reservations expressed by both scholars and practitioners about the impacts of the new public management paradigm, it produces assessments of the type, “Much of what has been devised in the name of accountability actually interferes with the responsibilities that individuals in organizations have to carry out work and to accomplish what they have been asked to do” (Radin, 2006, p.7; also Perrin, 1998; Feller, 2002; Weingert, 2005; Auranen and Niemien, 2010).
The promises, too, are likely to be real if and when they are realized. One takes here as a base the benefits contained in Behn’s presentation and the section on promises above. Atop this base are to be added the revised and new, expanded, disaggregated, and manipulable data sets emerging both from recent Federal science of science policy initiatives and other ongoing research (Lane and Bertuzzi, 2011). Thus, Sumell, Stephan, and Adams’ recent research on the locational decisions on new Ph.D.s working in industry accords with and provides an empirical base for the recent calls by the National Science Foundation’s Advisory Committee for GPRA Performance Assessment 2008 to collect and provide data on the “development of people” as an impact of agency support.
A different category of benefits owing less to improved public sector management practices and more to the realities of science policy decision making needs to be added to this list. The very same arguments cited above that the links between initial Federal investments in research are too long term and circuitous to precisely specify in GPRA or OMB planning or budget formats serves to increase the value for intermediate measures. For policymakers operating in real time horizons, even extending beyond the next election cycle, performance measures of the type referred to above are likely as good as they are to get. We live in a second best world. Although it may be analytically and empirically correct to state say that none of the proximate intermediate output measures, patents or publications for example, are good predictors of the ultimate impacts that one is seeking–increased per capita income, improved health–some such measures are essential to informed decision making.
Adding impetus to this line of reasoning is that the environment in which U.S. science policy is made is a globally competitive one, which increases the risks of falling behind rivals. Akin to an arms race or advertising in imperfectly competitive markets, support of research is necessary to maintain market share, even if the information on which decisions are made is imperfect.
Finally, as an empirically oriented economist whose work at various times has involved generating original data series of patents and publications and use of a goodly portion of the performance measures and methodologies now in vogue in evaluations of Federal and State science and technology programs, there is a sense of déjà vu to much of the debate about the promises and limitations of performance measures of impacts. The temptation is to observe somewhat like Monsieur Jordain in Moliere’s play, Le Bourgeois Gentilhomme, “Good heavens! For more than forty years I have been doing performance measurement without knowing it.”
Performance measures viewed either or both as a method for explicating needs assessments or conducting impact assessments are basic, indispensible elements in policy making, program evaluation, and scholarly research. What are open to issue are:
- the specification of the appropriate measures for the decision(s) under review– a complex task involving technical, political, and normative considerations;
- the proper interpretation and incorporation of existing and newly developed data and measures used in retrospective assessments of performance into decisions relating to estimating the prospective returns from alternative future Federal investments in research– decisions made within a penumbra of scientific, technical, economic, and societal uncertainties that performance measures reduce but do not eliminate; and
- providing evidence that use of performance measures as forms of evidence in fact improves the efficiency or rate(s) of return to Federal investments in research.
Given the above recitation of promises and limitations, the optimal course of action seems to be what Feuer and Maranto have termed science advice as procedural rationality (2010). It is to (1) have policymakers employ performance impact measures that correspond to what is known or being learned about how public investments in basic and applied science relate to the attainment of given societal objectives; (2) have the body of existing and emerging knowledge of how Federal in basic and applied research impact on societal objectives connect to the form of the decisions that policymakers are called upon to make; and (3) use gaps that may exist between (1) and (2) to make explicit the nature of the limits to which theory-based/evidence-based knowledge can contribute to informed decision making (Aghion, David, and Foray, 2009). Viewed in terms of preventing worse case outcomes, the objective should be to avoid the pell-mell drive now in vogue in State governments towards formula shaped coupling of performance measures and budgets, a trend as applied to Federal funding of research that is fraught with the risks of spawning the limitations described above.
To the extent that the STEP-COSEPUP workshop contributes to producing this course of action, it will have made an important contribution to the formulation of US research policy.
REFERENCES
- Adams J, Pendlebury D. Global Research Report: United States (Thomas Reuters). 2010.
- Aghion P, David P, Foray D. Can We Link Policy Practice with Research on ‘STIG’ Systems? Toward Connecting the Analysis of Science, Technology and Innovation Policy with Realistic Programs for Economic Development and Growth. In: Foray D, editor. The New Economics of Technology Policy. Cheltenham, UK: Edward Elgar; 2009. pp. 46–71.
- Auranen O, Nieminen M. University Research Funding and Publication Performance-An International Comparison. Research Policy. 2010;39:822–834.
- Behn R. Here Comes Performance Assessment-and It Might Even be Good for You. In: Teich A, Nelson S, McEnaney C, editors. AAAS Science and Technology Policy Yearbook-1994. Washington, DC: American Association for the Advancement of Science; 1994. pp. 257–264.
- Borner K, Contractor N, Falk-Krzesinski HJ, Fiore SM, Hall KL, Keyton J, Spring B, Stokols D, Trochin W, Uzzi B. A Multi-Systems Perspective for the Science of Team Science. Science Translational Medicine. 2010;2:1–5.
- Boroush M. National Science Foundation Infobrief. Arlington, VA: National Science Foundation; Jan, 2010. New NSF Estimates Indicate that U.S. R and D Spending Continued to Grow in 2008.
- Boroush M. National Science Foundation Info Brief. Arlington, VA: National Science Foundation; Oct, 2010. NSF Releases New Statistics on business Innovation.
- Boskin M, Lau L. Generalized Solow-Neutral Technical Progress and Postwar Economic Growth NBER Working Paper 8023. Cambridge, MA: National Bureau of Economic Research; 2000.
- Bozeman B, Sarewitz D. Public Value Mapping and Science Policy Evaluation. Minerva. 2011;49:1–23.
- Clemins P. Historic Trends in Federal R and D in Research and Development FY2011. Washington, DC: American Association for the Advancement of Science; 2010. pp. 21–26.
- Cohen WR. Patents and Appropriation: Concerns and Evidence. Journal of Technology Transfer. 2005;30:57–71.
- Cohen W, Nelson R, Walsh J. Links and Impacts: The Influence of Public Research on Industrial R and D. Management Science. 2002;48:1–23.
- Crespi G, Geuna A. An Empirical Study of Scientific Production: A Cross Country Analysis, 1981–2002. Research Policy. 2008;37:565–579.
- Cutler D, Kadiyala S. The Return to Biomedical Research: Treatment and Behavioral Effects. In: Murphy K, Topel R, editors. Measuring the Gains from Medical Research. Chicago, IL: University of Chicago Press; 2003. pp. 110–162.
- David P. Difficulties in Assessing the Performance of Research and Development Programs, AAAS Science and Technology Policy Yearbook-1994, op. cit. 1994. pp. 293–301.
- DuPree AH. New York: Harper; Torchbooks: Science in the Federal Government. 1957
- Executive Office of the President, Office of Management and Budget, Science and Technology Priorities for the FY 2012 Budget, M-10-30.
- Evenson R, Ruttan V, Waggoner PE. Economic Benefits from Research: An Example from Agriculture. Science. 1979;205:1101–1107. [PubMed: 17735033]
- Feller I. Performance Measurement Redux. American Journal of Evaluation. 2002;2007;23:435–452.
- Feller I. Mapping the Frontiers of Evaluation of Public Sector R and D Programs. Science and Public Policy. 2007;34:681–690.
- Feller I. A Policy-Shaped Research Agenda on the Economics of Science and Technology. In: Foray D, editor. The New Economics of Technology Policy. Cheltenham, UK: Edward Elgar; 2009. pp. 99–112.
- Feller I, Gamota G. Science Indicators as Reliable Evidence. Minerva. 2007;45:17–30.
- Feuer M, Maranto C. Science Advice as Procedural Rationality: Reflections on the National Research Council. Minerva. 2010;48:259–275.
- Freeman C, Soete L. Developing Science, Technology and Innovation Indicators: What We Can Learn from the Past. Research Policy. 2009;38:583–589.
- Freeman R, van Reenan J. Issues in Science and Technology. Washington, DC: National Academies Press; Fall. 2008. Be Careful What You Wish For: A Cautionary Tale about Budget Doubling.
- Gault F. Innovation Strategies for a Global Economy. Cheltenham, UK: Edward Elgar; 2010.
- Geiger R. Oxford, UK: Oxford University Press; To Advance Knowledge. 1986
- Geisler E. The Metrics of Science and Technology. Westport, CT: Quorum Books; 2000.
- Gladwell M. The Order of Things, New Yorker. Feb 14, 2011. p. 68ff. 2011.
- Godin B. Measurement and Statistics on Science and Technology. London, UK: Routledge; 2005.
- Goldston D. Mean What You Say, Nature. Vol. 458. 2009. p. 563. Published online 1 April 2009. [PubMed: 19340055]
- Grant J, Brutscher P, Kirk S, Butler L, Woodring S. Report to the Higher Education Funding Council for England, DB‐578-HEFCE. Cambridge, UK: RAND Europe; 2009. Capturing Research Impacts: A Review of International Practice.
- Gross C, Anderson G, Powe N. The Relation between Funding by the National Institutes of Health and the Burden of Disease. New England Journal of Medicine. 1999;340(24):1881–1887. [PubMed: 10369852]
- Haltiwanger J, Jarmin R, Miranda J. National Bureau of Economic Research Working Paper 16300. Cambridge, MA: National Bureau of Economic Research; 2010. Who Creates Jobs? Small vs Large vs Young.
- Hedge D, Mowery D. Politics and Funding in the U.S. Public Biomedical R and D System. Science. 2008;322(19):1797–1798. [PubMed: 19095928]
- Heisey P, King K, Rubenstein K, Bucks D, Welsh R. Economic Research Report Number 95. United States Department of Agriculture, Economic Research Service; 2010. Assessing the Benefits of Public Research Within an Economic Framework: The Case of USDA’s Agricultural Research Service.
- Jones B. As Science Evolves, How Can Science Policy? National Bureau of Economic Research Working Paper 16002. Cambridge, MA: National Bureau of Economic Research; 2010.
- Jorgenson D, Ho M, Samuels J. New Data on U.S. Productivity Growth by Industry. Paper presented at the World KLEMS Conference, Harvard University; August 19 –20, 2010. 2010.
- Kanninen S, Lemola T. Methods for Evaluating the Impact of Basic Research Funding. Helsinki, Finland: Academy of Finland; 2006.
- Kelves B. New Brunswick, NJ: Rutgers University Press; Naked to the Bone. 1997
- Kettl D. The Global Revolution in Public Management: Driving Themes, Missing Links. Journal of Policy Analysis and Management. 1997;16:446–462.
- Koopmans TJ. Measurement without Theory. Review of Economic Statistics. 1947;39:161–172.
- Lane J, Bertuzzi S. Measuring the Results of Science Investments. Science. 2011;331(6018):678–680. [PubMed: 21310987]
- Larsen M. The Implications of Academic Enterprise for Public Science: An Overview of the Empirical Literature. Research Policy. 2011;40:6–10.
- Link A. Retrospective Benefit-Cost Evaluation of U.S. DOE Vehicle Combustion Engine R and D Investments, Department of Economics Working Paper Series. 2010.
- Link A, Scott J. Oxford, UK: Oxford University Press; Public Goods, Public Gains. 2011
- Lundvall B, Borras S. Science, Technology, and Innovation Policy. In: Fagerberg J, Mowery D, Nelson R, editors. The Oxford Handbook of Innovation. Oxford, UK: Oxford University Press; 2005. pp. 599–631.
- Mansfield E. Social Returns from R and D: Findings, Methods and Limitations. Research Technology Management. 1991;34:6.
- Manski C. NBER Working Paper Series #16207>. Cambridge, MA: National Bureau of Economic Research; 2010. Policy Analysis with Incredible Certitude.
- Massachusetts Institute of Technology. The Third Revolution: The Convergence of the Life Sciences, Physical Sciences, and Engineering, Letter to our Colleagues. Jan, 2011. 2011.
- Moed H. Citation Analysis in Research Evaluation. Dordrecht, The Netherlands: Springer; 2005.
- Mohr L. Impact Analysis for Program Evaluation. 2. Thousand Oaks, CA: SAGE; 1995.
- Mowery D, Rosenberg N. Technology and the Pursuit of Economic Growth. Cambridge, UK: Cambridge University Press; 1989.
- Murphy K, Topel R. The Value of Health and Longevity. Journal of Political Economy. 2006;114:871–904.
- National Academies of Sciences. Evaluating Federal Research Programs. Washington, DC: National Academy Press; 1999.
- National Academies of Sciences. Rising Above the Gathering Storm. Washington, DC: National Academies Press; 2007.
- National Academies of Sciences. A Strategy for Assessing Science. Washington, DC: National Academies Press; 2007.
- National Academies of Sciences. Managing University Intellectual Property in the Public Interest. Washington, DC: 2010.
- National Academies Press, National Science Foundation. Changing U.S. Output of Scientific Articles: 1988–2003–Special Report. Arlington, VA: National Science Foundation; 2007.
- Office of Management and Budget. Program Assessment Rating Tool Guidance, No. 2007-02. 2008.
- Organisation for Economic Cooperation and Development. Modernising Government. Paris, FR: Organisation for Economic Cooperation and Development; 2005.
- OECD Science, Technology and Industry Outlook. Paris, FR: Organiszation for Economic Cooperation and Development; 2010.
- Payne A. Earmarks and EPSCoR. In: Guston D, Sarewitz D, editors. Shaping Science and Technology Policy. University of Wisconsin Press; 2006. pp. 149–172.
- Perrin B. Effective Use and Misuse of Performance Measurement. American Journal of Evaluation. 1998;19:367–379.
- Radin B. Washington, DC: Georgetown University Press; Challenging the Performance Movement. 2006
- Roessner D, Bozeman B, Feller I, Hill C, Newman N. The Role of NSF’s Support of Engineering in Enabling Technological Innovation, Report to the National Science Foundation. Arlington, VA: SRI International; 1997.
- Rosenberg N. New York, NY: Harper; Torchbooks: Technology and American Economic Growth. 1972
- Rosenberg N. Learning by Using, Inside the Black Box. Cambridge, UK: Cambridge University Press; 1982. pp. 120–140.
- Ruegg R, Feller I. A Toolkit for Evaluating Public R and D Investment. NIST GCR 03-857. Gaithersburg, MD: National Institute of Standards and Technology; 2003.
- Ruttan V. Minneapolis, MN: University of Minnesota Press; Agricultural Research Policy. 1982
- Sarawetz D. Philadelphia, PA: Temple University Press; Frontiers of Illusion. 1996
- Savage J. Cambridge, UK: Cambridge University Press; Funding Science in American: Congress, Universities, and the Politics of the Academic Pork Barrel. 1999
- Schmoch U, Schubert T, Jansen D, Heidler R, von Gortz R. How to Use Indicators to Measure Scientific Performance: A Balanced Approach. Research Evaluation. 2010;(19):2–18.
- Schubert T. Empirical Observations on New Public Management to Increase Efficiency in Public Research-Boon or Bane? Research Policy. 2009;38:1225–1234.
- Shils E, editor. Cambridge, MA: MIT Press; Criteria for Scientific Development: Public Policy and National Goals. 1969
- Stokols D, Hall K, Taylor B, Moser R. The Science of Team Science. American Journal of Preventive Medicine. 2008;35:S77–S89. [PubMed: 18619407]
- U.S. House of Representatives, Committee on Science and Technology. The Nobel Prize Awards in Science as a Measure of National Strength in Science. Science Policy Study Background Report No. 3; 99th Congress, Second Session. 1986.
- Von Hippel E. Cambridge, MA: MIT Press; Democratizing Innovation. 2005
- Wagner C, Flanagan A. Workshop on the Metrics of Fundamental Science: A Summary. Washington, DC: Critical Technologies Institute; 1995. Prepared for Office of Science and Technology Policy, PM-379-OSTP.
- Weingert P. Impact of Bibliometrics upon the Science System: Inadvertent Consequences? Scientometrics. 2005;(62):117–131.
- Wilson J. Bureaucracy. New York, NY: Basic Books; 1989.
- Zakaria F. How to Restore the American Dream, Time. Oct 21, 2010. 2010.
Footnotes
- 1
A natural experiment occurring on February 15–16, 2011 highlights the continuing character of these differing perspectives. OSTP’s release on February 10, 2011 of its R and D Dashboard, that contains data about NIH and NSF R and D awards to research institutions and “links those inputs to outputs—specifically publications, patent applications, and patents produced by researchers funded by those investments”– produced an immediate flurry of comments and exchanges on SciSIP’s list server. Most of this exchange contained the point-counterpoint themes in the Behn-David exchange cited above, as well as those recounted in this paper. Among these were: how were outcomes defined? could they be measured? is there reasonable consensus on what they are? One rejoinder to these comments raised in response to specific reservations about the meaningfulness of patent data was that when Congress asks what are we getting from these billions spent on R and D, it is helpful to have patent number to point to as one outcome of the nation’s investment.
- 2
An added value of calling attention to these retrospective studies is that they all entail studying the relationship between a cause and an effect: “between the activities involved in a public program and any outcome of that program…” (Mohr, 1995, p. 1). Without a theoretical foundation that specifies the set of dependent and independent variables to be, performance measures represent what Koopman’s has termed, “measurement without theory.” (1947, p. 161).
- 3
The implicit assumption throughout this paper is that decisions follow or at least are influenced by, the evidence contained in performance measures. That is, evidence of good/high performance leads to the continuation/expansion of a program; evidence of poor/low performance leads to termination/contraction. This is obviously a stylized proposition. Technically sophisticated assessments of the contributions of the ATP program did little to save it from the congressional budget axe, and in light of the current political environment one can anticipate that a similar fate awaits Federal research programs related to environmental protection and climate change, however well done and rich with societal impacts they may be.
- 4
“The major issues in science policy are about allocating sufficient resources to science, to distribute them wisely between activities, to make sure that resources are used efficiently and contribute to social welfare” (Lundvall, B. and S. Borras, 2005, p. 605)
- 5
These propositions have turned out to be a double-edged theoretical sword for purposes of Federal research policy. They were initially advanced to justify public sector support for basic research but as interpreted in the 2002 OSTP‐OMB R and D Investment Criteria and then implemented in the PART process, they have become the theoretical basis for excising several domestic technology development programs.
- PubMedLinks to PubMed
- THE PROMISES AND LIMITATIONS OF PERFORMANCE MEASURES - Measuring the Impacts of ...THE PROMISES AND LIMITATIONS OF PERFORMANCE MEASURES - Measuring the Impacts of Federal Investments in Research
Your browsing activity is empty.
Activity recording is turned off.
See more...