NCBI Bookshelf. A service of the National Library of Medicine, National Institutes of Health.
National Research Council (US) Committee on Computer-Assisted Modeling. Computer-Assisted Modeling: Contributions of Computational Approaches to Elucidating Macromolecular Structure and Function. Washington (DC): National Academies Press (US); 1987.
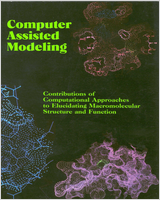
Computer-Assisted Modeling: Contributions of Computational Approaches to Elucidating Macromolecular Structure and Function.
Show detailsWe turn our attention from structural considerations to the more complex questions surrounding biological function. This section contains discussions of enzyme catalysis, protein design, and ligand/substrate design.
CATALYSIS
The Theory of Enzyme Catalysis
Enzyme catalysis is one of the most crucial and certainly the most intriguing aspects of the kinetic behaviors of proteins. The central question about catalysis is, which aspects of protein structure and dynamics cause the often enormous enhancements of rates of reaction over the rates in water? This question is, at best, incompletely answered and at worst very much open. One can not expect that molecular dynamics, with its use of a classical mechanical forcefield, will, by itself, provide definitive answers. Empirical potentials in molecular dynamics or molecular mechanics calcu lations are approximations chosen to model thermodynamically stable minima in energy surfaces. Catalytic reactions are by their very nature dependent on barriers or maxima in these surfaces. Not even the form of the potential used in most classical calculations would be correct. However, it is reasonable to think that this problem will eventually be solved by an approach that combines molecular dynamics and quantum mechanical methods. Molecular dynamics techniques can handle the motion of many atoms, and quantum mechanics can be used to represent events along the reaction pathway at the catalytic site and in the reactants (substrates), that is, wherever chemical bonds are broken and/or formed. Recent studies of simple organic reactions in solution by Jorgensen (in press) and studies of enzyme mechanisms by Warshel (1986) exemplify this combined approach.
In work on simple organic reactions in solution, the reaction path has been investigated by a series of ab initio quantum me chanical calculations of the reactants in vacuo in different states of reaction, and molecular mechanical (Monte Carlo) simulation of the solvation of each state. When combined, the results of these two calculations yielded estimates of the free energy profile along the reaction coordinate, from which reaction kinetics can be estimated. Although the quantum mechanical calculations did not take into account the response of the reactants to the solvent environment, Jorgensen (in press) nevertheless obtained very promising results for three different reaction types: SN1, SN2, and addition reactions.
Parallel studies of enzyme mechanisms pose additional prob lems, simply because the systems, including the reacting species, contain many more atoms. Except in a few simple reactions such as catalysis by carbonic anhydrase, the substrates are much larger than the reactants in the models chosen by Jorgensen. Also, in many enzyme-catalyzed reactions, a chemical bond forms between enzyme and substrate in an intermediate product of the reaction. In these cases the ab initio quantum mechanics calculation, which by its nature is currently restricted to systems of a few atoms, will have to be performed on a fragment or fragments of the chemically reacting species. It is not yet clear how this can be done without introducing large errors. Warshel has introduced the use of a more approximate quantum mechanics method, the ab initio empirical valence bond (EVB) method, into this problem to replace the quantum mechanical component. Although it can handle more atoms, the EVB method initially had the drawback of having to be calibrated by simulations designed to predict known molecular properties. In this instance, acidities of ionizing groups were used. However, this was done successfully, and Warshel and Sussman (1986) and Hwang and Warshel (1987) found that the method now can rationalize observations of changes in catalytic efficiency of mutant enzymes. These results emphasize the critical role of stabilization of the reaction's transition state by electrostatic interactions. Because of the empirical character of the EVB method and a lack of general experience with it, these conclusions await confirmation through further study with the EVB method and ab initio methods.
Given the great interest in the theory of enzyme catalysis, investigators have already begun to apply a combination of ab initio quantum mechanics and molecular dynamics (Rao et al., 1987). This will generate new problems to be solved. As noted, a major problem will be encountered for those enzyme reactions in which a chemical bond is formed between enzyme and substrate in an intermediate step. It will also be necessary to establish the magnitude of the systematic error caused by transfer of a quantum mechanical result obtained without solvation, not only to a solvated situation, but also to a protein active site environment, where the rates are much enhanced. Some very careful work will be required before this approach can be applied reliably to enzyme mechanisms. However, caveats notwithstanding, these studies are worth doing.
DESIGNING NEW PROTEIN STRUCTURES
The following section discusses the future of protein design, which is one of the key areas of growth in macromolecular modeling.
In the same way as Levinthal's (1966) pioneering studies initiated computer-assisted modeling, Richardson's (1981) work on the anatomy and taxonomy of proteins signaled the transition from molecular archeology to molecular design, for protein chemists. We call our drug design project computer-assisted molecular design—there are more types of molecules than proteins. Richardson presented a framework for understanding the organization of protein architecture. This framework condenses the observations of protein structure and architecture from individual structure solutions into a form that allows us to think of protein architecture as manipulable. Site-directed mutagenesis of protein structure is a simple way of altering proteins without really altering protein architecture. The technique of producing chimeric proteins by gross manipulation of gene structure is another early approach to the manipulation of protein architecture. At this point, the problems involved in designing new proteins are formidable. Consider the process of designing an ordinary protease. Proteases typically have 250 amino acids. Since there are 20 amino acids, there are 20250 possible proteins of length 250. The only sensible way to reduce the number of possible proteins to one design is to use several levels of decomposition that specify how portions of the protein are to be organized architecturally, spatially, and functionally. Our understanding of the rules of thumb triggered by the Richardson paper is now evolving rapidly.
Protein design cannot be divorced from the issues of protein expression and folding. The complete cycle for the design, expression, and folding of proteins can be represented as in Figure 7-1.

FIGURE 7-1
The cycle of protein design and expression.
To be able to design a protein effectively, one must be able to traverse this design cycle rapidly and often. At present, several important conceptual problems prevent us from completing this cycle at all. Suppose that we could design a hypothetical protein using the architectural concepts. The output would be a three-dimensional model of the protein embodying a particular amino acid sequence. Given this hypothetical design, the next task would be to construct a gene. The problem here is that the amino acid sequence of the hypothetical protein, in general, specifies only two of the three bases in each codon of the gene. One way to resolve this issue is to choose a random third base (See Figure 7-2).

FIGURE 7-2
The current pattern of the flow of information in the cycle of protein design and expression.
Once the DNA of the constructed gene has been transcribed to messenger RNA and edited, there is a linear sequence of three-base codes that, as Nirenberg (1965) has described, exist in 64 combinations of the bases. However, the 64 codons code for only 20 amino acids, so there is, at this point in the cycle, a surplus of information. The ribosome translates the messenger RNA codons into nascent polypeptide.
The Anfinsen (1975) experiment involving the denaturation and renaturation of ribonuclease has been used to convince us that proteins fold into their active three-dimensional structure solely on the basis of the information contained in their sequence. Many scientists have been trying for the last two decades to predict the secondary and tertiary structures of proteins from the amino acid sequences alone. Their efforts have met with partial success at best. We lack information about the protein folding portion of the design cycle. The surpluses of information (denoted by pluses in the upper portion of Figure 7-2) and the deficiencies of information (denoted by the minuses) can be abstracted to form the cycle pattern in the lower portion of the same figure. Clearly, our current perception of the design cycle is flawed. Recent experiments show, for example, that a gene that is moved from its native host to another expression vector does not necessarily produce well-folded proteins. Even when the codon utilization statistics of the new expression vector are mimicked, complete protein folding does not necessarily occur. This suggests that third base redundancy may be partially used to control protein folding, especially for complex proteins.
Experiments should be designed to explore how third base redundancy influences protein folding. With such data, the information from a hypothetical protein design could be used to properly construct the DNA of a gene. A proper gene would then transcribe and translate properly to yield a polypeptide that folds properly. If these conditions were met, the design cycle abstraction would be as shown in Figure 7-3.

FIGURE 7-3
The ideal pattern of flow of information in the cycle of protein design and expression.
If the information flow around the protein design and implementation cycle is preserved, then it should be possible for protein engineers to rapidly traverse this cycle in the design and perfection of novel proteins.
Computer Representation
Computer-assisted modeling of molecules has been evolving since the original work of Levinthal (1966). His work was the first time that a computer, a PDP-1 from the then-infant Digital Equipment Corporation (DEC), was used to draw the three-dimensional structure of a small organic molecule. It used one line segment to represent each chemical bond. With simple software controls, the molecule could be rotated in space and redisplayed. Similarly, the conformation of the molecule could be changed by rotating one portion of the molecule around a bond that formed an isthmus between it and the remainder of the molecule. The display of the molecule was done in pairs of images where the image of one molecule was rotated 5 degrees around the vertical axis. This produced a stereoscopic effect that permitted the three-dimensional structure of the molecule to be perceived without having to rotate it continually. All of molecular graphics has simply been an extension and refinement of these powerful ideas. The number of line segments drawn per second has risen dramatically. Color has been added. Hardware stereo devices have been developed, and very recently powerful array processors have been added to permit the rapid calculation of molecular energetics during modeling.
These techniques for display and modeling were developed and refined in a few academic research laboratories. They began to diffuse to biochemical and genetics laboratories in academic and industrial institutions worldwide. Over the past 20 years, the manufacturers of computer and graphics hardware have begun to recognize that molecular graphics and modeling is a substantial market. We are now at the critical point in this respect. Hardware manufacturers are now willing to design workstations (i.e. integrated computational and graphics machines for individual use) for the molecular modeling and design market. A new class of workstations is expected in the next year. Two members of the class of personal supercomputers (PSCs) have been identified, and collaborations are in place to insure that these machines, when they enter the commercial market, will be fully conditioned “chemistry engines”. The four functions, molecular energy computation, molecular configuration control, molecular graphics, and reasoning about molecular structure, will be integrated in one computer system.
The PSCs will provide a nearly ideal package for mass distribution of CAMD capabilities. Market forces can be expected to expand the number of different machines and the features that each machine offers. Standards at various levels, as defined by the International Standards Organization (ISO), will permit existing and new program systems to be transported rapidly onto the PSC class members. The standardization efforts will permit a decoupling of the computational support systems (i.e. hardware, graphics, operating systems, and the molecular modeling and design programs) from the intellectual uses of such systems.
The existence of standards, however, does not guarantee portable program code. Scientists who write new programs must know about these standards and write programs that conform to them. Commercial organizations that take existing scientific programs should shape them towards the standard style because, in the end, the size of the commercial market will depend on the ability of end users to piece together working systems from components made out of various standard programs. If these standardization efforts succeed, then in the future, the molecular modeling community will be able to routinely make smooth transitions to more powerful computer support systems.
Computer graphics representations offer alternative ways of understanding molecular structure and function. They started as the simplest white line drawings on black screens, then progressed to color images, to solid surfaces, to dot surfaces, and to electrostatic surfaces. Intergraph three-dimensional representations and white light hologram representations have been developed and used for molecular structure problems. Intergraph is composed of approximately 20 individual photographs where vertical strips are selected from each photograph and composed into one image. The composite image is viewed through a linear fresnel lens. The next generation of workstation, the PSC, will offer ray-traced images as part of the operating system. In a ray traced image the reflections on a surface are compared by calculating the trajectory of light beams from all possible light sources. This produces in the extreme the reflections of one object on another. A truly three-dimensional representation where the molecule would actually occupy three-dimensional space is needed. A breakthrough in a field such as plasma physics is necessary to make this a reality.
Impediments to Progress
The central bottleneck to progress in protein design is our inability to predict protein tertiary structure from amino acid sequence. The notion put forth by Anfinsen 25 years ago was that the amino acid sequence alone determines tertiary structure. This notion may be too simplistic, and there may indeed be a higher level code than the Nirenberg nucleic acid to amino acid conversion by the ribosome. Since the Anfinsen conjecture and the experimental detail surrounding it are largely prohibitory in nature, they had the effect of discouraging experimentation in expression and folding of proteins. Scientists who are concerned with protein expression are content with the Nirenberg code and explain away anomolous results because they see no need for any other effect. Scientists concerned with protein folding cannot explain how proteins fold, but then are discouraged by the Anfinsen conjecture from asking for more information from the geneticists. A theory and experiment linking codon utilization in gene structure with the folding of protein structure would be a major step toward reconciling these views.
PREDICTING FUNCTION FROM A PREDICTED THREE-DIMENSIONAL STRUCTURE
In principle, the information needed to predict the function of a biological macromolecule is encoded in its three-dimensional structure. We assume that we must know the three-dimensional structure of a macromolecule before we can fully understand its function. The problem is, how do we decode the rules that govern the relationship between structure and function? A subset of this problem will be discussed below: the prediction of the change in the functioning of a protein that results from the binding of a ligand.
Recently, the possibility of computer-assisted drug design based on the three-dimensional structure of target biomolecules has received much attention in the scientific literature (Beddell, 1984; Goodford, 1984; Hol, 1986). Those in the field believe that medicinal chemistry is poised to undergo a revolution as dramatic as the events in the 1950s and 1960s that transformed organic chemistry from a descriptive to a predictive science. Since we are at the beginning of a new age, the many challenges ahead do not diminish the excitement of knowing that the solutions are also on the horizon. We have a sense that, at last, we know what it is that we have to learn and have at least the rudiments of the necessary tools at hand.
In anticipating this revolution, we are presupposing that we can or soon will be able to predict the functions of proteins from their structures. In particular, we would need to be able to predict the ability of a protein to recognize and bind a ligand and to predict the structure of the “optimum” ligand. Beyond that, however, we would need to be able to predict how the protein carries out its function and how it recognizes and interacts with other macromolecules to alter its own functions and theirs. Although we have learned much about these topics, there are unanswered questions that we must be able to answer before we will be able to make accurate predictions.
Experience in Ligand Design from Experimental Protein Structures
One illustration of our current state of achievement is given by work on hemoglobin. Ligands affect the function and properties of hemoglobin in complex ways. Investigators began to attempt to design ligands based on the three-dimensional structure of a protein as soon as such structures were available. In the early 1970s, the group headed by Goodford at Wellcome Laboratories in England began to explore the possibilities of ligand design by receptor fit (Beddell, 1984; Goodford, 1984). They used the structure of hemoglobin as determined by protein crystallography and constructed a wire model that was hinged so that they could examine both the oxy- and deoxy- states.
The first studies involved the design of ligands (using mechanical models) to fit the diphosphoglycerate (Figure 7-4, compound 1) binding site and then to mimic its function. The investigators used simple concepts of complementary shapes, electrostatic interactions, and possible covalent bonds. The designed compounds (Figure 7-4, designated compounds 2-4) do indeed mimic the effect of diphosphoglycerate on the dissociation of oxygen from hemoglobin. Subsequent crystallographic work supported the proposed binding mode. In addition, the relative binding energy of various analogues to a number of different hemoglobins was measured for 29 protein-inhibitor combinations. Statistical analysis revealed a highly significant correlation between the strength of binding and the number of covalent and ionic interactions. The use of computer graphics for the design would have accelerated this process since it took three months to construct the physical wire model of the protein.

FIGURE 7-4
Some molecules synthesized to aid in elucidating the relation of structure to biological activity of some macromolecules. See text for details.
This work was then expanded in an attempt to design a compound for the treatment of sickle cell anemia. The goal was to develop a compound that would affect the oxygen-dissociation curve in a way opposite to that of diphosphoglycerate. An intensive biochemical, physiological, and structural examination of the problem suggested that a ligand that binds between the alpha subunits of oxyhemoglobin might have the desired effect. Since no natural ligand for this site was known, the ligands were designed from the protein structure alone and designated compounds 5 and 6 (See Figure 7-4). Although the proposed binding mode has not been experimentally verified, the designed compounds did produce the expected change in function of hemoglobin. One of the compounds is now in clinical trials for the treatment of sickle cell disease. Thus, using rather primitive tools, the Wellcome group was able to predict the effect of a small molecule on the function of a protein.
The recent experience of Perutz et al. (1986) emphasizes both the important accomplishment made by these workers and the limits of our molecular understanding. Perutz and coworkers experimentally demonstrated several of the potential binding sites that a molecule might recognize in hemoglobin. Specifically, they solved the crystal structure of eight ligand-hemoglobin complexes and showed that there are at least six different positions on the protein at which a ligand might form a tight complex. Since the ligands were selected on the basis of their perceived structural similarity, this result must be carefully considered by those who attempt to design ligands to fit a particular site on a protein. Three of the molecules bind in sites that overlap; two of these raise the minimum gelling concentration of hemoglobin S, whereas the third lowers it. Each of the bound ligands changes the structure of the proteins so little that the change is barely detectable, yet some of the ligands increase the gelling concentration, some decrease it, and others do not change it at all. This work makes it clear that even after studying structure and function of hemoglobin for 25 years, an investigator may still be puzzled by the functional consequences of the minute structural changes that accompany ligand binding.
The work of Perutz et al. (1986) also illustrates that the design of a drug is more complicated than the mere design of a tightly bound ligand. Clofibrate raises the gelling concentration of hemoglobin S. Also, it has been used clinically for other disorders and so is known to be absorbed, metabolized, and nontoxic. Yet it cannot be used to treat sickle cell disease because it is so tightly bound to serum albumin that it is not available to bind to the hemoglobin. The lesson to be drawn from this is that when we design a new drug from theoretical principles, we must somehow incorporate the possible interaction of the proposed ligand with all other macromolecules of the body.
The work cited and other studies on hemoglobin underscore both the promise and the challenges in predicting the changes in the function of a protein that are brought about by formation of a protein-ligand complex. This work also highliglits the further challenges of predicting all of the interactions of the ligand with the organism.
The design of inhibitors of dihydrofolate reductase was aided by the three-dimensional structures of the proteins. Research efforts of two pharmaceutical companies and their collaborators culminated in the crystallographic determination of the structure of the dihydrofolate reductase from several species, some with bound ligands (Beddell, 1984; Blaney et al., 1984; Goodford, 1984). Each group designed a trimethoprim (Figure 7-4, compound 7) analogue (Figure 7-4, compounds 8 and 9), that was proposed to form a new interaction with a nearby arginine. Goodford (1985) was able to verify this interaction (Figure 7-4, compound 8) by crystallography. Both new analogues show greatly enhanced binding affinity. However, neither shows enhanced antibacterial activity, presumably because they do not easily penetrate into the bacterial cell. Additionally, neither has any antibacterial activity in whole animals, so they are not candidates for development as new therapeutic agents. Thus, although this work did successfully predict the local functional consequences of structural modification of a previously marketed drug, it did not predict all of those properties needed to convert a biologically interesting compound into a therapeutically useful one.
In summary, efforts to design inhibitors of dihydrofolate reductase have shown that, although knowing the structure of the target biomolecule is very useful when designing a ligand, this alone is not enough information to design a drug. Furthermore, these protein structures have been available for approximately five years, yet neither company has capitalized on them, nor have other research groups used the published enzyme structures to design new compounds.
Many other groups have used crystal structures of proteins to design analogues of known ligands. In an early example, a physical model of lysozyme and a proposed mechanism of its hydrolytic action were used to design a transition-state inhibitor (Goodford, 1984). The compound inhibits the enzyme; it binds 32 times more strongly than the corresponding substrate. The proposed binding mode has also been confirmed by x-ray diffraction studies.
In the first use of color computer graphics in the design of ligands to bind to a protein, workers at the University of California at San Francisco used interactive potential energy calculations and molecular graphics to dock thyroxine analogues into the binding site in the crystallographic structure of pre-albumin (Blaney et al., 1982). They designed and synthesized several more strongly bound ligands. The predictions were confirmed experimentally. Since the function of pre-albumin appears to be transport of thyroxine, no further predictions of the consequence of ligand-protein interaction were made.
Experience in Ligand Design from Predicted Protein Structures
Protein structures modeled by homology to proteins whose three-dimensional structure is known have also proved useful in the design of novel ligands. For example, workers at two different pharmaceutical companies have used a structure of the enzyme renin that was modeled from other members of the aspartic proteinase class (Anonymous, 1986; Boger, 1986). Such models suggested the structures of new inhibitors. The compounds were shown to be potent inhibitors in vivo as well as in vitro.
Approximate target macromolecule structures have also been used to design new agents. The classic example is the design of captopril (Figure 7-4, compound 9), an inhibitor of angiotensin-converting enzyme and a clinically successful antihypertensive agent (Petrillo, 1982). Captopril was designed from a proposed structure of the substrate when bound to the enzyme. The structure of the enzyme was assumed to be similar to that of carboxypeptidase A because of mechanistic similarities between the two enzymes.
Inferring Binding Sites
Much of this section has addressed issues related to determining and analyzing the structures of proteins of known sequence but unknown three-dimensional structure. Once these structures are known, detailed studies can be carried out of the relationships between those structures and the corresponding functions of a protein. Proteins express their functions through binding of other molecules, often termed effectors, with or without concomitant transformation of the effector, e.g., degradation or chemical reactions at functional groups. We have discussed structure/activity studies of the binding of effector molecules to putative sites in a protein of known structure. A separate body of research has focused on a complementary problem: relating the structures of several effector molecules to one another in order to determine information about binding sites, often termed active sites, in proteins of unknown structure. When such studies are successful, they can obviously provide structural information about a protein that can be used in conjunction with some of the techniques for structure determination discussed earlier in this report.
The general problem of inferring binding sites can be stated simply. Given a set of molecules that are presumed to bind to the same site in a given protein of unknown structure, we must infer the size, shape, and binding characteristics of the active site. Several problems are subsidiary to this general problem. We mention them here, but a detailed analysis is beyond the scope of this report. For example, one must consider the process ofrecognition of the effector molecules prior to the actual binding in the active site. One must consider the possibility of conformational changes of both an effector and an active site during recognition and binding. One must perform very careful studies to ensure that the measured biological or chemical responses for several effectors are in fact due to binding in the same active site. A useful introduction to this research area, with leading references, has been presented (Olson and Kristoffersen, 1979).
At least three approaches have been used to infer the structure of receptor sites:
- thereceptor mapping aproach of Humber et al. (1979);
- theactive analog approach of Marshall et al. (1979);
- the DYLOMMS program of Wise et al. (1983).
These approaches are closely related. All follow the same basic principles, but in different ways. All begin with the assumption that similar effectors possess relatedpharmacophoric patterns, i.e., similar dispositions in three-dimensional space of similar structural features important for binding. Independent studies are used to postulate pharmacophoric patterns of active molecules, generally using the most conformationally rigid molecules to form hypotheses. Once such a pattern is assigned, all possible conformations of each effector are examined to determine if there are low energy conformations that present the pattern. This both tests the hypothetical pattern and begins building a set of molecules that can be superimposed based on the pattern. Once superpositions are established, the volume occupied by the molecules can be used to define the cavity of the active site. Molecules of related structure that can yield the pharmacophoric pattern, but that display no activity, can be used to define the walls of the cavity, further elaborating its shape.
Recently, practicing medicinal chemists have become enthusiastic about these uses of computational and computer graphics techniques to compare the three-dimensional structures of ligands that bind to a receptor. They use the common features of the aligned structures to propose tentative maps of the receptor topography. These maps are then used to design new compounds (Ghose and Crippen 1985; Hopfinger, 1985; Humblet and Marshall, 1981). These techniques have benefited from the knowledge gained through protein crystallography. In particular, current applications of receptor-mapping methods usually compare the location of the projection of ligand atoms to possible binding sites, rather than identifying the location of the ligand atoms themselves as had been done previously.
The final category of computer-assisted prediction of the biological properties of a small molecule is also the oldest. This type of methodology, Quantitative Structure/Activity Relation ships (QSAR) uses statistical or pattern recognition methods to explore the possible relationship between the biological and physical or substructural (presence or absence of certain functional groups) properties of molecules. Given the known utility of QSAR methodology to predict the potency of untested analogues (Hopfinger, 1985; Martin, 1981), it is important that the developers of this methodology are actively pursuing the challenge of evaluating the reliability of linear free-energy equations for cases in which the protein structure is known. In the case of dihydrofolate reductase, several investigators have compared the conclusions from QSAR and molecular graphics modeling of the inhibitors (Blaney et al., 1984). The conclusions derived from the two methods agree closely, confirming the proposal that the QSAR equations contain information about the types of noncovalent interactions between the inhibitors and the enzyme. However, a major advantage of QSAR over other computer-based methodologies is that one can attempt to develop equations for any biological response. For example, equations have been developed for the enzyme inhibition, antibacterial, and whole-animal antitumor activity of dihydrofolate reductase inhibitors (Blaney et al., 1984). Thus, QSAR is a logical complement to the more structure-based computer methodologies. It could be used to model the potential whole-animal activity of new ligands and perhaps to search for unanticipated interactions with other macromolecules.
Computer Tools for Ligand Design from Three-Dimensional Protein Structure
The recent excitement in computer-assisted drug design has arisen because scientists now have available the elements of each of the important tools for such an activity. Two types of computer hardware are necessary: high-speed color graphics and affordable but powerful computers dedicated to modeling. In addition, a growing body of data on the three-dimensional structure of proteins is becoming available, as our understanding increases of some of the relationships between structure and function of proteins. Finally, software is also available for the graphics display of the molecules and for modeling the energetics and thermodynamics of the binding.
Specialized graphics tools for molecular design have also been developed. Some of these arose from the related activity of docking a known ligand into a protein. The display of the surface of the binding site is more useful for ligand design if it is color-coded to suggest the preferred type of noncovalent interaction at that point in space. For example, through such displays we can distinguish between surfaces near positively charged, negatively charged, hydrogen-bond accepting, hydrogen-bond donating, and hydrophobic regions of the protein.
Another helpful tool used with the graphics display is the immediate read-out of energy values as the ligand is docked into a putative binding site and as bonds in the ligand and/or the protein are rotated to facilitate the docking. Design of ligands at the computer screen is aided by stereoscopic viewing devices and implements that allow one to move an object being displayed (such as a ligand) in three dimensions while keeping the rest of the display as it was. Experience has shown that molecular mechanics energy minimizations are necessary to evaluate the geometry and energy of the proposed complexes (Pincus and Scheraga, 1979).
It was noted previously that one persistent but often hidden problem in ligand design is that a ligand may bind to a protein in a different orientation or at a totally different site than the investigator anticipated. Kuntz et al. (1982) have devised a computerized means of evaluating such possibilities based on shape alone.
The design of a new ligand molecule is aided by the graphics display of the energetically preferred sites on the protein for interaction with various types of possible ligand atoms (Goodford, 1985). Such sites are identified as the energy of interaction of the probe atom at each point on a three-dimensional grid surrounding a protein. The ligand would be designed to interact at as many of these sites as feasible.
Once a proposed ligand is designed, its thermodynamics of binding can be predicted with the free-energy perturbation method if it is a reasonably close analogue of a known compound.
If there are data on the relative energy of binding of other ligands to the protein, a QSAR or receptor mapping analysis discussed above may suggest regions on the target that are conformationally more flexible than the experimental structure may suggest. QSAR (or at least consideration of physical properties) is expected to also be useful in the design of ligands that will have the appropriate whole-animal properties.
Impediments to Ligand Design from Protein Structures
Proteins are conformationally mobile. They are not the static structures that the graphics display of the crystal structure suggests. For example, molecular dynamics calculations on myoglobin have shown that within 300 picoseconds, 2,000 different conformational minima are sampled (Elber and Karplus, 1987). The root-mean-square difference in the location of the atoms in the most different structures is 2 Å; this means that many atoms move substantially more than that.
Proteins also change conformation when ligands are bound to them; hence, ligand design methodologies must be able accurately to predict such movements. For example, when the antiviral compound VIN 52084 is bound to the human rhinovirus, 13 residues of the protein undergo measurable conformational change (Smith et al., 1986b). The main chain moves as much as 3 Å, the channel to the binding pocket opens to the solution, the isoelectric point of the system changes from 6.9 to 7.1, and the occupancy of Ca++ at a distant point on the virus increases.
Conformational responses to ligand binding may be part of the function of the protein. For example, in response to Ca++, the channel-forming proteins of the gap junction between cells show small cooperative rearrangements of the relative orientation of the subunits. This rearrangement results in the narrowing of the diameter of the Ca++ channel within the cell by 18Å and thus closes the channel to Ca++ passage (Unwin and Ennis, 1984). During this rearrangement, the conformation of each subunit does not change appreciably, only the orientation of each subunit changes with respect to the others.
Conformational responses to ligand binding may form the basis of the selectivity of ligands for very similar proteins. Evidence from crystallography, QSAR, and molecular graphics suggests that conformational changes in the enzyme in response to the binding of ligands is responsible for the selectivity of trimethoprim for bacterial dihydrofolate reductases in contrast to vertebrate enzymes (Blaney et al., 1984). In the chicken liver enzyme, a tyrosine residue moves 5.4 Å in response to the binding of trimethoprim.
Since there is no experimentally established three-dimensional structure of a membrane-bound receptor, for this type of protein we depend on indirect observation and inference for our notions about conformation and conformational changes in response to ligand binding. Current concepts of receptor function usually invoke a conformational change as part of the transduction of the signal of a binding event into the ultimate biochemical and physiological response. Thus, it is possible that the regulatory and second messenger binding sites on receptor proteins might become available only in the presence of the ligand. Furthermore, that certain compounds only partially activate a receptor suggests the possibility that a whole family of receptor conformations is available.
Thus, to use protein structure design a ligand that influences the action of a protein whose function requires more than one conformation or in which the putative binding site is very flexible, we would like to know the relevant three-dimensional structures of that protein and be able to predict the conditions under which each is stable. In other words, we would find it difficult to predict the function of a new ligand unless we had available structures of these protein conformations. We see this as a problem that will require at least as much study as the problem of finding the global minimum energy structure.
The ligand binds to a protein that is part of a system. In solution, a protein is part of a complex with water, ions, and cofactors. Alternatively, it may function while interacting with a membrane. These other species affect the strength of binding of the ligands of interest. For example, trimethoprim binds to dihydrofolate reductase with a 10,000-fold increase of affinity in the presence of its cofactor compared to its absence.
The covalent structures of some proteins are modified during the course of their function. The large family of receptor kinases are responsible for phosphorylatation of receptors as a means of regulating that receptor function. Thus, the addition of a single phosphate group to a protein can dramatically alter its function.
Other proteins are not functional until they are structurally modified after their synthesis. For example, sperm binds to its receptors on the egg only if these receptors are glycosylated. Additionally, posttranslational processing can impart subtle variations in properties to a protein. For example, it is thought that the benzodiazepine receptor is the same protein throughout the brain, but that it is glycosylated to a different extent in different regions of the brain. These differences in glycosylation are reflected in different relative affinities of the receptor for various ligands.
Thus, for accurate and realistic models on which to base theoretical ligand design, we need to be able to include such other species in the calculation. Unfortunately, there are often not molecular mechanics parameters for such cofactors and transition metals. Including these additional ions and molecules increases the complexity and time of the calculation enormously, partly because the number of atoms is increased but more dramatically because the search for the stable arrangement of atoms is much more complicated. This is the multiple-minimum problem, but with even fewer experimental constraints on the solution of the problem. Furthermore, we cannot use traditional molecular mechanics concepts for transition metals because they undergo changes in oxidation and spin states that dramatically affect the optimum geometric arrangement of ligands. To include such ions, we need a combination quantum and molecular mechanics calculation. Although progress has been made in such calculations (Warshel, 1981; Singh and Kollman, 1986), they still need refinement and testing and tend to be calculations that strain available computers. Thus, we see promise that the tools required will be available, but they are not yet in routine use.
There may be more than one binding made for the ligand. The experience with the binding of ligands to hemoglobin and the different binding orientations of methotrexate (Figure 7-4, structure 10) and dihydrofolate (Figure 7-4, structure 11) to dihydrofolate reductase highlight this problem (Blaney et al., 1984). The method of matching ligand shapes to protein cavities is helpful in predicting such alternate binding modes. However, it is currently limited because it considers only the correspondence of the shape of the ligand and the binding site and not their possible flexibility or electrostatic and hydrophobic contributions to binding energy. In principle, this problem could be solved by examining the relative energy of all potential conformations of the protein and the ligand and all potential relative orientations of the two. As noted above, for such calculations water and cofactor molecules and associated ions should also be included. Even if there are only two conformations of the protein each with two binding sites and two conformations or enantiomers of the ligand, the problem increases eight-fold! The challenge escalates when we consider that, in drug design, we would like to consider many possible analogues for synthesis. Thus, much more sophisticated techniques for pruning conformational and orientation hyperspace need to be developed before detailed calculations of this magnitude will be possible.
Even if we could predict the mode and strength of binding of a ligand to a protein, the effect of such binding on the function of the protein in the cell might not be obvious. The simplest case would seem to be the design of an enzyme inhibitor. If an enzyme is inhibited, we would expect that fewer substrate molecules would be transformed in a given unit of time. However, this is not necessarily true. For example, current evidence is that receptor kinases are present in the cell in high concentrations: the rate of phosphorylation of the receptor is apparently governed by the concentration of the cyclic nucleotide and the conformational state of the receptor and not the level of the enzyme. Inhibition of such an enzyme by even 90 percent might have no observable physiological effect. In other cases, the level of a particular enzymatic activity is regulated by feedback control. Inhibition of such an enzyme would be overcome by production of more enzyme. Alternatively, inhibition of an enzyme might simply lead to the presence of higher levels of substrate but the same rate of turn-over of substrate through the biochemical system. The physiological effects of such agents may be impossible to predict.
The situation is even more complex in proteins that have multiple domains that control multiple functions. A compound that prevented sickling of hemoglobin S would be useless as a drug if it also prevented oxygen binding or release or if, when bound, it promoted the crystallization of hemoglobin in a different crystal form.
A further complication in trying to understand function from structure is that a single protein may interact with several small molecules and other proteins in a complex regulatory scheme. Different subunits of domains of a protein may have different but interrelated functions. For example, all four subunits ofTorpedo californica acetylcholine receptor are necessary to elicit a nicotinic response to acetylcholine, whereas only the alpha subunit is refquired for binding the antagonist alpha-bungarotoxin (Mishina et al., 1984). Thus, the structure of the alpha subunit might help in the design of a ligand, but the structure and function of all four subunits might be needed to predict whether the compound would be an agonist or antagonist.
Other factors might make a ligand useless as a therapeutic agent. When a ligand is administered to an animal, it must survive the metabolic and structural defenses of the animal in order to reach its proposed site of action at the required concentration. The ligand may be a substrate for any one of many enzymes, some of which appear to have evolved broad specificity in order to metabolize foreign substances and thereby protect the organism from its unpredictable environment. Ultimately, we expect to be able to predict the biotransformations of small molecules from the structures of the enzymes involved, but we cannot do so today.
The ligand may also fortuitously bind to other macromolecules in the body and, as a result, may not be available to the target protein. The ligand may have the correct physical and chemical properties to be rapidly excreted into the urine or bile before it has a chance to move to its target. Finally, the ligand may be so slightly soluble that it cannot achieve high enough concentrations in the blood or gastrointestinal tract for it to be distributed to its site of action. Again, we have some informal rules that allow us to attack these problems, but lack the basic knowledge we need to make true predictions. A ligand might also be useless in curing disease because it or one of its metabolites produces toxicity in the animal.
To use a ligand as a drug, it must be technically feasible to do so. This means that it must be possible to produce the compound in the required quantities and purity; it must be stable enough to ship to the patient; and an acceptable pharmaceutical form of the compound must be devised. A major advance has been made in the computer-assisted design of pathways for the synthesis of compounds. However, further enhancements would make this tool even more useful.
Economic factors also figure into feasibility; if the compound is to be sold, the patentability of the compound, the cost of its manufacture, the cost and effectiveness of competing therapy, and the expected incidence of the disease for which it is effective will also be issues in the decision to market the compound.
Other complications may also emerge when one is predicting function or designing ligands from predicted three-dimensional protein structures. First, the confidence in the exact coordinates of the protein structures will be lower. This greater uncertainty will complicate the investigation of proposed function or the design of ligands because the exact dimensions of the possible binding sites will be uncertain, as will the conformation of residues on the surface of the protein. In principle, these questions can be answered using extensive molecular dynamics and minimization calculations. The prediction of function might be straightforward if the unknown protein shows a strong sequence homology with a protein of known structure and function.
Another complication with the use of predicted structures is that we may be unaware of posttranslational modifications of the structure. Ultimately, we expect to be able to predict such modifications from the substrate specificities of the enzymes that perform them. However, we cannot do so today.
Consideration of protein structures based on DNA sequence may obscure the fact that the protein may function as part of a multisubunit assembly. Multiple subunit proteins are common. To predict the function of such a protein, we must realize that it binds to the other subunits. It is not enough to consider other proteins coded on the same chromosome; the genes that code for the two different protein chains that form the subunits of hemoglobin are located on different chromosomes. Hemoglobin illustrates a further complication in using DNA sequences: there are at least four different variants of the beta subunit. Only one of these is produced in quantity by the organism. Thus, to predict the function of the alpha chain of hemoglobin, we would need to recognize that it functions in a tetrameric structure with two subunits of a different type, and that, of those with which the alpha subunits could bind, only the beta subunit is produced in appreciable quantity.
The transcribed protein may have one activity and be transformed into a product that has a different activity. Peptide hormones usually arise by the limited hydrolysis of a larger protein that circulates in serum. Sometimes the same carrier protein can be cleaved at different sites to produce different peptide hormones. At present, we cannot predict such events. Only when we know the sequence of every peptide hormone would we be able to recognize the potential for a particular protein to be a carrier of a hormone.
In summary we do not adequately understand the relationship between the details of the three-dimensional structure of a protein and its function. Without such an understanding, we cannot predict the effect that a bound ligand will have on the function of the protein. We lack this understanding partly because three-dimensional structures of proteins have been determined only recently, and molecular graphics hardware and software are also newly available to experimental scientists. But in many cases, we do not know the three-dimensional structure of the protein of interest, nor do we have a good idea of all of its functions. We know even less about the relationship between structure and function of carbohydrates, because we have so little structural information on them. This is a problem that will not be solved in the short-term.
While there are methods to predict the potency of molecules once a structure is suggested, we need better tools for molecular design to help the chemist suggest molecules to examine experimentally or theoretically. The tools described above are primitive. Although some methods are available to match candidate molecules against proposed shape requirements for binding, it is not possible to also specify the chemical properties of the designed compound with existing software. The current methods process a file of three-dimensional coordinates of candidate molecules; this file is generated from experimental or theoretical studies and so is incomplete. Additionally, we cannot automatically compare a compound proposed by a computer program with those already in the world literature as tested for that activity, nor can we automatically detect if the proposed compound is identical or similar to compounds known to have some biological activity deleterious to that desired. It is expected that many of these tools will be developed rather soon.
- Functional Aspects of Proteins and Nucleic Acids - Computer-Assisted ModelingFunctional Aspects of Proteins and Nucleic Acids - Computer-Assisted Modeling
- Homo sapiens INSC spindle orientation adaptor protein (INSC), transcript variant...Homo sapiens INSC spindle orientation adaptor protein (INSC), transcript variant 3, mRNAgi|1890264384|ref|NM_001278313.2|Nucleotide
- PREDICTED: Rattus norvegicus microtubule-associated protein, RP/EB family, membe...PREDICTED: Rattus norvegicus microtubule-associated protein, RP/EB family, member 2 (Mapre2), transcript variant X1, mRNAgi|2678911204|ref|XM_039097095.2|Nucleotide
- bone morphogenetic protein 2, partial [Trachemys venusta]bone morphogenetic protein 2, partial [Trachemys venusta]gi|1959480726|gb|QQX07713.1|Protein
- cytochrome oxidase subunit I, partial (mitochondrion) [Heterophleps fusca]cytochrome oxidase subunit I, partial (mitochondrion) [Heterophleps fusca]gi|1424746655|gb|AXC02756.1|Protein
Your browsing activity is empty.
Activity recording is turned off.
See more...