NCBI Bookshelf. A service of the National Library of Medicine, National Institutes of Health.
National Research Council (US) Roundtable on the Demography of Forced Migration. Demographic Assessment Techniques in Complex Humanitarian Emergencies: Summary of a Workshop. Washington (DC): National Academies Press (US); 2002.
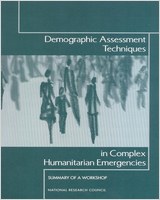
Demographic Assessment Techniques in Complex Humanitarian Emergencies: Summary of a Workshop.
Show detailsWhen data are poor and conditions are difficult, there are two principal approaches for obtaining demographic estimates. First, consistency checks are a useful tool; one can compare two data sets for the same population, compare the data for the area of interest with data from a model or a neighboring area, or examine data for internal consistency. For example, are the numbers or percentages of men and women or of different ages similar to other estimates for the population or what one would expect?
Second, indirect estimation techniques have been developed by demographers to produce estimates of a demographic parameter on the basis of information that is only indirectly related to its value. Indirect estimation is commonly used to obtain estimates of rare or difficult-to-measure demographic events, e.g., maternal mortality. Some examples include:
- using the proportion of children ever born to women aged 20-24 who have died by the date of the survey to estimate the probability of dying by age 2;
- using the incidence of orphanhood to estimate adult mortality;
- interviewing a migrant regarding the disposition of the household left behind; and
- interviewing survivors about the survivorship of their siblings.6
These indirect methods can be used to estimate demographic events among displaced populations or those they left behind when it is difficult to access actual data for the populations. For example, Robinson led a study in northern China that asked migrants from North Korea about mortality among the households of their relatives still in North Korea to estimate mortality due to famine.7
Cluster Sampling
Social scientists, epidemiologists, and statisticians alike are familiar with the principles of sampling. Sampling is selecting a subset of the population of interest in order to gain information about the entire population. A good sample will therefore be representative of the population. The design for a sample, however, varies greatly depending on the time, money, and staff available, the logistical challenges encountered, and the intended use of the data. The greater the precision required of the estimate, generally, the larger the sample size will need to be. The most basic type of sampling is called simple random sampling. A simple random sample, a type of probability sample, is one in which each member of the population has an equal probability of selection.
Cluster sampling is an approach in which each member of the population is assigned to a group (cluster) and then clusters are randomly selected and all members of selected clusters are included in the sample. Often combined with stratification techniques (in which case it is called multistage sampling8 ), cluster sampling is the approach most often used by epidemiologists. A statistical software package called EPI-Info (Centers for Disease Control and Prevention, Atlanta, Georgia, USA) is commonly used by field staff in emergency settings. However, in conducting statistical tests, most of the EPI-Info program assumes that it is working with simple random samples unless one specifically adds a variable to take into account the design effect of cluster sampling.9 Although adding a variable to adjust for the design effect is a way to correct for the effects of deviation from simple random sampling schemes (the CSAMPLE module in the EPI-Info program can correct for this), many field staff are not well versed in sampling techniques and therefore unaware of the need to correct for sampling design. This often results in artificially low estimates of the true underlying variance within a population.10 There was a lot of concern expressed at the workshop about potential misuse or misinterpretation of EPI-Info results. In addition, the standardized approach used by EPI-Info calculations for required sample size may not be appropriate for other situations (i.e., measuring human rights abuses that may be heterogeneous or rare, such as torture).
Paul Spiegel of the Centers for Disease Control and Prevention gave a presentation on the problems encountered when using cluster sampling for the estimation of populations in refugee camps or other displaced populations. Cluster sampling is appropriate for situations in which there is no readily available sampling frame11 of individuals (such as a camp census list), but for which it is easy to obtain lists of subgroups or clusters of individuals, e.g., compounds, buildings, or tents. It is generally cheaper and quicker than nonstratified sampling.
The EPI-Info module called CSAMPLE is commonly used for immunization and nutrition surveys, based on a sample of 30 clusters, which is the minimum number of clusters generally used for these types of surveys by field staff. In the first stage, probability proportional to size sampling (PPS)—a method that ensures that smaller clusters are not overrepresented in the sample—is used to choose clusters, but this can be problematic. First, estimated population sizes for each cluster in complex emergencies are generally not accurate. Second, displacement among subpopulations is unlikely to be proportional across a region or district because of the chaotic nature of forced migration and the nearly constant inflows and outflows of migrants in many situations. Thus the adjustment for the displacement is often imprecise. One approach to adjust for population displacement is to use the latest information available (e.g., food distribution censuses or other data) to update baseline population figures before sampling.
In the second stage, once sample clusters are chosen, households are chosen. At this stage, the sample of households may not be truly probabilistic; the sample may be biased toward households on roads or in the center of the settlement if interviewers are not careful in their household selection methods. The interviewers' judgment on sampling households may affect the validity of results. Some potential solutions to these problems are to use systematic sampling—the selection of every nth household from a prepared list of households in the sample clusters—or to use maps to create a sampling frame of households. The accuracy of the parameter estimate being measured can be increased by increasing the number of clusters sampled. However, this method also increases the costs and time for survey, both of which are scarce in most emergency settings.
During the final stage of the sampling process, all those in the sampled households are selected or one member of each household is selected. There was some question among the participants whether individuals or households should be counted as the unit of analysis (see below).12 Practical considerations can create limits as well; nonresponse can make it very difficult to reach one's predetermined sample size. Nonresponse may also make statistical inference questionable.13
Donna Brogan of Emory University commented on some of the statistical issues surrounding the use of cluster sampling in refugee camps. First, one must decide whether the unit of analysis is the individual, the household, or the family; this in turn will guide the sampling process. Cluster sampling is often used because the World Health Organization (WHO) Programme on Immunization has popularized it (using CSAMPLE in EPI-Info for analysis), but it is not the only option, and the assumptions underlying it may not be appropriate for every circumstance. The WHO cluster survey method assumes an equal probability selection of individuals, which may be an invalid assumption if cluster size estimates are inaccurate. In this case, weighted analyses can be done to obtain unbiased (or approximately unbiased) estimates on the basis of updated information on cluster size.14 When cluster sampling is used, 30 is the minimum number of clusters in the sample (not 30 individuals).
Sampling methods ultimately depend on the event to be measured and the criteria that are valued most by the researcher, such as costs, time, and sampling error. Unfortunately, the WHO cluster survey method, which was originally developed to measure vaccination levels in a stable population, has been used indiscriminately in situations involving forced migration. Different techniques and types of samples need to be considered in crisis situations, so it is important that field staff understand the limitations and potential weaknesses of the various methodologies.15
Spatial Sampling
Spatial sampling—which is a variant of cluster sampling and is also known as area probability sampling—is the use of geographic area and population density to estimate population size or proportions, often using handheld global positioning systems (GPS) units or geographical information systems (GIS).16 This method can be used when maps and censuses of the geographic area under study do not exist. Vincent Brown of Epicentre/ MSF in Paris, introduced a method of sampling that is frequently used in very acute emergency situations. The quadrate method—as Brown called it—counts the population in small square blocks of equal areas. Blocks are randomly chosen within the refugee camp (or other defined area). The average sample data is then extrapolated to the level of the total camp population.
The first stage is to draw a map of the camp with a compass, measuring all sides of the camp by foot or with a vehicle odometer. The angles between each side are measured and then a map with a square grid superimposed on top is constructed. The second stage is to select a random sample of blocks (again, usually 30) and collect population data for all of the households in each block. The average population per block is calculated and extrapolated to obtain the total. There are many potential challenges with this method, including choosing the size of the blocks, the number of blocks to sample, and taking differing population densities into account. According to Brown, at the field level, these decisions have been based mainly on common sense. Although this approach is an important tool that can be used systematically at the beginning of a crisis, further research is needed to improve the statistical validity of the method.17
Denis Coulombier of the Institut de Veille Sanitaire (the French National Institute for Public Health Surveillance) compared a method known as T-square estimation with the quadrate method. Although the quadrate method is the typical method used for estimating populations in disasters or forced migrations, the T-square method—a method that is often used in agronomy—is a potential alternative. It involves sampling a number of random points, measuring the distance between each point and the nearest household or family unit and then measuring the distance between that household and the next closest one. In this way, one can estimate population density. Coulombier and colleagues tested this method at a festival in France and the results were very comparable with results obtained using the quadrate method and using exhaustive entry registration. The table below compares the advantages and disadvantages of the two methods.
Participants were optimistic about the potential of using the T-square method in future situations. At the very least, it could be used as a rapid, low-cost estimation technique in the early stages of a crisis, and it is a good alternative to relying on politically biased estimates from governments or other groups. It also may be the least intrusive method of estimating a population, which could be helpful in particularly sensitive situations. Further testing is planned at voluntary mass gatherings.
Quadrate Method | T-Square Method | ||
---|---|---|---|
• | Small square sizes can achieve a design effect of one or less | • | Does not produce confidence intervals |
• | Relatively accurate | • | Relatively accurate |
• | Resource intensive and relatively slow | • | Rapid (can be done in one-third of the time of the quadrate method) and uses relatively few resources |
• | Visualization of squares is easy | • | Difficult to select true random points |
• | Potential for omission or duplication | • | Potential for measurement errors |
• | Spatial distribution is homogeneous between squares (but spatial heterogeneity can be accounted for by using 25-meter squares) | • | Spatial distribution is aggregated between shelters |
Qualitative Techniques
Although qualitative methods are not the first to come to mind when thinking about how to estimate populations in emergency settings, they can offer some important insights for researchers throughout the various phases of a crisis. William Weiss of Johns Hopkins University gave a brief presentation on how qualitative techniques can assist those who are attempting to estimate forced migrant populations and their characteristics.
A qualitative method is a dynamic process that involves purposive or random sampling and generally small samples.18 Key characteristics are triangulation, flexibility, iterativeness, and open-ended inquiry.19 Qualitative research can help researchers reduce non sampling bias by using team members with different perspectives and by crosschecking sources.
Weiss argued that qualitative methods can give insights into such processes as food sharing, shelter building and sharing, credit transactions, and the use of health care services. They also allow refugees to be a part of the planning and problem-solving process. There are a variety of techniques that can be quite useful in emergency settings and refugee camps. For example, participatory mapping is a technique that has been used for assessment among refugees in Kenya. Houses are drawn on a map and then refugees are interviewed to learn more information about each household, such as the age and gender of the household members. A walkabout is a simple observation method in which researchers walk around a camp to observe the layout and amenities; it can be used to confirm maps and create checklists of program needs. Creating a timeline of refugees' migration history can give insight into their personal history and health status, and can also later be used to draw insights about events and their consequences.
Commenting on the potential uses of qualitative methods in emergencies, Giovanna Merli of the University of Wisconsin, Madison, argued that they should not be thought of as separate from quantitative methods, because the two are complementary. In fact, the various methods can be seen as part of a continuum. Different methods can be used in tandem to validate findings, elucidate processes, and refine other methods. Merli noted that many issues could be addressed using qualitative methods, including vulnerability, poverty, population processes, resource distribution, reasons for migration, and—especially—security. Some demographers—typically thought of as quantitative researchers—are now developing mixed-method approaches that use both quantitative and qualitative methods. Researchers must be careful when using qualitative participatory methods, however, to make sure that they truly understand the underlying demographic and social processes they are trying to measure. They should also be fluent in the language of the displaced and understand the political and social contexts of the population.20
In sum, the best sampling or estimation method for a given situation is context-specific. Several participants suggested that it would be helpful to have a manual that gives some guidance on the different methods and approaches and their use in different phases of and in different types of complex humanitarian emergencies. It could serve as a common reference point for researchers and field staff involved in these situations.
Footnotes
6 For more detailed descriptions of indirect estimation techniques, see United Nations 1983.
7 See Robinson et al. 1999.
8 Multistage sampling is a technique whereby clusters are selected as in cluster sampling and then sample members are selected from the cluster members using simple random sampling. More than one stage of clustering may be used.
9 Design effect is a measure of the contribution of the sampling design of the survey to the variance of the estimates. Thus, in this case it refers to the increased uncertainty of estimates obtained from samples selected using cluster sampling in comparison with simple random sampling. See Henry 1990:107-109 for a more detailed explanation.
10 A confidence interval is a range of estimated values within which the true value of the population parameter (e.g., population size, mortality rate) can be expected to be located with a certain probability (i.e., degree of confidence). If one does not include a variable to account for the design effect when cluster sampling is used, then confidence intervals may be artificially small. See Henry 1990:118-123 for further information.
11 A sampling frame is the list from which the sample will be selected and ideally should consist of every member of the population of interest. If there are differences between the population and the sampling frame, they will constitute a form of nonsampling bias.
12 The unit of analysis is the entity being analyzed by a study and for which data are collected (e.g., individual person, household, school district).
13 Nonresponse is the lack of valid responses from some members of the sample and it can occur when a respondent refuses to participate in the survey or refuses to answer a specific question. This is to be distinguished from noncontact, which occurs when the respondent cannot be reached, and vacancy, which occurs when a dwelling is empty. All of these constitute part of nonsampling bias or error.
14 Weighting biased estimates is a technique that can be used during analysis to correct for sampling error. A weight is a numerical factor reflecting the sample design that is applied to the estimates.
15 For examples of cluster surveys as they have been used in emergency settings, see Binkin et al. 1995, Boss et al. 1994, Malilay et al. 1995, and Spiegel and Salama 2000.
16 The global positioning system (GPS) is a system of satellites that provide precise location information that can be accessed using handheld electronic units. Geographical information systems (GIS) are computerized systems to store, record, analyze, and produce maps based on spatial data.
17 See Brown et al. 2001 for a complete description of the quadrate method.
18 Purposive sampling is another term for nonprobability sampling. Nonprobability sampling methods are frequently used to sample for rare populations or events. These methods involve subjective judgment on the part of the researcher to select the sample in order to achieve the objectives of the research. Unlike probability samples, each member of a nonprobability sample does not have an equal chance of being selected.
19 Triangulation refers to a process of comparing data from several different sources to obtain a more precise result.
20 Two useful publications offer further guidance on using qualitative techniques. Catholic Relief Services has published a manual entitled Rapid Rural Appraisal and Participatory Rural Appraisal Manual for estimating livelihoods and food security in rural areas, particularly when there is upheaval (http://www
.catholicrelief .org/what/overseas/rra_manual.cfm). Also, the Johns Hopkins School of Public Health's Center for Refugee and Disaster Studies has a publication entitled Rapid Assessment Procedures (RAP): A Guide to Understanding the Perceived Needs of Refugees and Internally Displaced Populations (http://www .jhsph.edu/refugee/rap_desc .html).
- ESTIMATING POPULATION SIZE AND STRUCTURE - Demographic Assessment Techniques in ...ESTIMATING POPULATION SIZE AND STRUCTURE - Demographic Assessment Techniques in Complex Humanitarian Emergencies
- Nardia scalaris isolate FATOL540 tRNA-Leu (trnL) gene, partial sequence; trnL-tr...Nardia scalaris isolate FATOL540 tRNA-Leu (trnL) gene, partial sequence; trnL-trnF intergenic spacer, complete sequence; and tRNA-Phe (trnF) gene, partial sequence; chloroplastgi|671728826|gb|KJ802077.1|Nucleotide
- Nardia scalaris isolate L1428 tRNA-Leu (trnL) gene, partial sequence; trnL-trnF ...Nardia scalaris isolate L1428 tRNA-Leu (trnL) gene, partial sequence; trnL-trnF intergenic spacer, complete sequence; and tRNA-Phe (trnF) gene, partial sequence; chloroplastgi|671727699|gb|KF943074.1|Nucleotide
- Nardia scalaris isolate L1428 NADH dehydrogenase subunit 1 (nad1) gene, partial ...Nardia scalaris isolate L1428 NADH dehydrogenase subunit 1 (nad1) gene, partial cds; mitochondrialgi|671727977|gb|KF943232.1|Nucleotide
- Nardia scalaris clone 4 pentatricopeptide repeat protein (PPR) gene, partial cdsNardia scalaris clone 4 pentatricopeptide repeat protein (PPR) gene, partial cdsgi|190612758|gb|EU495508.1|Nucleotide
Your browsing activity is empty.
Activity recording is turned off.
See more...