NCBI Bookshelf. A service of the National Library of Medicine, National Institutes of Health.
Dehmer SP, Maciosek MV, Flottemesch TJ. Aspirin Use to Prevent Cardiovascular Disease and Colorectal Cancer: A Decision Analysis: Technical Report [Internet]. Rockville (MD): Agency for Healthcare Research and Quality (US); 2015 Sep. (Evidence Syntheses, No. 131s.)
This publication is provided for historical reference only and the information may be out of date.
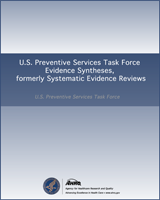
Aspirin Use to Prevent Cardiovascular Disease and Colorectal Cancer: A Decision Analysis: Technical Report [Internet].
Show detailsHealthPartners Institute for Education and Research ModelHealth™: Cardiovascular Disease
1. Model Overview
The decision analysis of aspirin use for the primary prevention of cardiovascular disease (CVD) and colorectal cancer (CRC) in this study was conducted using an adapted version of the HealthPartners Institute for Education and Research ModelHealth™: Cardiovascular disease microsimulation model. Adaptations for this analysis include a full integration of detailed tobacco use functions from the ModelHealth™: Tobacco microsimulation model, and the addition of four categories of cancer aligning with those considered by the systematic evidence reviews that were conducted in parallel with this study [1, 2]. The final analysis reflects only the inclusion of CRC, based on the systematic evidence review's findings, which lacked support for a significant effect of aspirin on the other cancer types. Herein, the adapted model used for this study is referred to as ModelHealth: CVD.
ModelHealth: CVD is a collection of scientific evidence-based parameters, mathematical functions, and procedural logic—implemented using Visual Basic 6 and Microsoft Excel—designed to evaluate cardiovascular disease prevention policies at the population level. The primary unit of observation in this model is an individual representative person who takes on a variety of detailed attributes (such as age, sex, race/ethnicity, BMI, systolic blood pressure, disease status, etc.). The lifetime progression of these characteristics is simulated over time. Epidemiological data sourced from the Framingham Heart Study—a major cardiovascular disease surveillance study ongoing since 1948—plays an important role in this model's construction.
Although the mechanics of ModelHealth: CVD center on individuals—i.e., through microsimulation—policy relevance is achieved through aggregating a sufficient number of individuals to be representative of a policy-relevant group, such as the U.S. population. ModelHealth: CVD can be scaled easily to simulate the lifetime progression of hundreds, thousands, or even millions of individuals. Policy interventions are evaluated by simulating the same population twice—once with the policy intervention of interest, such as a clinical preventive service, imposed, and once without it. In practice, this evaluation approach is comparable to a randomized clinical trial (RCT) design, with the treatment and the placebo being applied to the same hypothetical research population.
2. Model description
ModelHealth: CVD involves a considerable number of “moving parts.” To provide a high-level overview before proceeding to model specifics, this section briefly describes the general mechanisms underlying the model design.
Initialization
Figure B1 illustrates the process flow of ModelHealth: CVD. As a microsimulation, the unit of observation is a hypothetical person. Each new simulation first involves initializing a person at a specific age (e.g., 40), with individual characteristics (such as sex and race/ethnicity) and initial health parameters (such as cholesterol and blood pressure levels and BMI) all drawn from U.S. statistically representative distributions. Thereafter, ModelHealth: CVD simulates the hypothetical person's lifespan and the natural history of cardiovascular disease using annual Markov cycles.

Appendix B Figure 1
ModelHealth: CVD Flow Diagram.
Preventive Services
At the beginning of each annual Markov cycle, the model determines whether the simulated individual receives a preventive service, such as a screen for hypertension or high cholesterol or aspirin counseling. Eligibility for preventive services may be dictated by the parameters of a policy intervention—such as aspirin use among all persons older than age 40 without prior history of GI bleeding or hemorrhagic stroke in the treatment arm—or by contemporary rates of background preventive services (i.e., applied to both policy arms) observed in the population. Upon receiving a preventive service, the model determines whether the individual is eligible for treatment (e.g., taking statins for high cholesterol). Pharmacological treatment criteria for dyslipidemia and hypertension are implemented to be consistent with the Adult Treatment Panel III [3] and the JNC-7 [4] recommendations, respectively.
Treatment
The effect of treatment for high cholesterol or high blood pressure is realized through its impact on high- and low-density lipoprotein cholesterol (HDL-C/LDL-C) or systolic blood pressure (SBP), respectively. For example, an individual with high cholesterol could be treated with a statin and see a 30 percent reduction in LDL and a 10 percent increase in HDL, but taking a statin does not translate to a direct reduction in the individual's risk of a myocardial infarction. Instead, these changes will translate to lowered risk of disease, as determined by the customized risk engine described in the following section. In contrast, taking aspirin on a daily basis directly alters the relative risk of having an event (such as a myocardial infarction or a gastrointestinal bleed).
Disease Events
The next step in each annual Markov cycle (following prevention/treatment) is to determine whether the individual experiences any non-fatal disease events during that year. Specifically, a person may: (1) have a myocardial infarction, (2) have an ischemic stroke, (3) have a hemorrhagic stroke, (4) experience angina pectoris, (5) develop congestive heart failure, (6) develop intermittent claudication, (7) develop diabetes, (8) experience a gastrointestinal bleed, (9) develop CRC, and/or (10) develop another type of cancer. The annual risks of (1)-(7) are determined by equations derived specifically for this model using data from the Framingham Heart Study [5, 6]. If a person has a cardiovascular event—that is, one or more of (1)-(6)—and survives, that person becomes eligible for secondary prevention. Treatment for dsylipidemia and hypertension for secondary prevention similarly based on ATP III and JNC-7 guidelines, respectively, and men and women who have a non-fatal myocardial infarction or ischemic stroke are also eligible for aspirin chemoprophylaxis.
Each year a person also faces a risk of dying from cardiovascular disease, a fatal case of cancer, or from other causes. The annual risk of death from CVD-related causes also is based on a study-specific equation derived from the Framingham Heart Study. The probability that a cancer episode is fatal is derived from Centers for Disease Control and Prevention (CDC) data. The probability of dying from a cause other than CVD or cancer is derived from U.S. life tables. A person who dies of any cause—or reaches the age of 100—exits the model, lifecycle complete.
Aging and Progression of Natural History
Finally, when a person survives a cycle, that individual's health status and parameters must be transitioned for the next cycle. Each cycle is annual, and therefore, the individual's age will simply increment by one. Cardiovascular risk factors—namely, HDL, LDL, SBP, and BMI—naturally progress over time, and annual transitions are modeled by a two-step process. First, it is determined whether the individual's risk factor increases, decreases, or stays the same. These probabilities are based on a multinomial logistic equation (which accounts for age, previous values, and other individual characteristics). Second, if a specific risk factor is determined to increase or decrease, a secondary set of equations determines the size of this change. The process repeats itself until the simulated person dies (or reaches age 100).
3. Model Data Sources and Parameters
A computational model with the degree of detail contained within ModelHealth: CVD requires a considerable amount of data and scientific evidence to specify all necessary parameters and inform the key transitional mechanisms. This lengthy section describes all the data sources (and in cases, assumptions) required for the model to operate.
3.1. Parameter Initialization
Each iteration of ModelHealth: CVD begins with the initialization of a new representative individual to simulate. Initial demographic characteristics, including age, sex, race/ethnicity, and U.S. Census region, are derived from the American Community Survey three-year sample [7]. Analyses for this study were stratified by 10-year age group, sex, and baseline 10-year CVD risk. Persons meeting the characteristics of each strata are oversampled from the characteristics of the general U.S. population. Initial education is derived from the combined 2009-2012 Current Population Surveys [8]. Initial CVD risk factors, including BMI, SBP, LDL, and HDL are derived from the combined 2001-2010 National Health and Nutrition Examination Survey (NHANES) surveys [9-13]. Diabetes and prior CVD status at model initialization also are derived from the combined NHANES surveys. Initial smoking status is derived from the 2007 National Health Interview Survey[14] and calibrated to estimates by the Congressional Budget Office [15]. Initial smoking status is described in Section 3.6. Baseline characteristics of the simulated U.S. population cross-section are presented in Table B1.
Appendix B Table 1
Baseline Characteristics of Simulated U.S. Population Cross-Section (Ages 35+).
3.2. Progression of Risk Factors
After each annual Markov cycle in ModelHealth: CVD, an individual's time-dependent attributes must be transitioned to reflect the age progression and natural history of cardiovascular disease risk factors over one's lifetime. A person's age simply increments by one, but the remaining risk factors (BMI, HDL, LDL, and SBP) transition according to a two-step process. Change in smoking status is described in Section 3.6.
Step 1. Determine probability that a risk factor changes
In the first step of the process, a person faces a probability of increasing, decreasing, or staying the same in a particular risk factor. For LDL, HDL, and BMI, staying the same is defined as a change of +/-1 percent per year. Due to the greater variability in measuring blood pressure, staying the same in SBP is classified as being within +/-3.5 percent per year. In all cases, these probabilities were estimated using multinomial logistic regression. HDL, LDL, and SBP were estimated using annualized Framingham Heart Study data adjusting for age, sex, and BMI [5, 6]. BMI was estimated from Behavioral Risk Factor Surveillance System (BRFSS) survey data (from current weight and previous year recall) adjusting for age, sex, and race/ethnicity [16].
For year-to-year BMI transitions, the increasing or decreasing cases were split in two additional sub-cases. Specifically, one allows for small changes or “drifting” (i.e., an increase or decrease of 1 to 5 percent), and the other accommodates larger changes (i.e., an increase or decrease of 5 percent or more). Our analysis of Framingham Heart Study and BRFSS data indicate that these weight-change modalities reflect what people typically experience in real life, and the probabilities of each modality shift as we age. For example, a typical male may be most at risk for significant weight gain in his 20s, be more likely to have his BMI drift up in his 30s and 40s, and then face a stronger tendency towards weight stabilization in his 50s and 60s.
Step 2. Determine size of risk factor change
Once a person's transition modality has been determined, the second step is to determine the size of the change. Age, sex, and (in the case of BMI) race/ethnicity-specific equations were estimated for each of these cases. Whereas the first step in the process is stochastically determined in each cycle (i.e., facing a probability of each scenario), the second step is deterministic, with the transition applied as a percentage change (or zero change, in the case that a risk factor remains stable from the previous year). Table B2 summarizes the details of this two-step process of year-on-year transitions of risk factors.
Appendix B Table 2
ModelHealth: CVD Annual Progression of Risk Factors.
3.3. Risk of Cardiovascular Disease Events
Published risk calculators for cardiovascular disease—such as PROCAM [17], SCORE [18], QRisk [19], or those derived from the Framingham Heart Study[20]—generally estimate an individual's 10-year risk of disease. These are difficult to translate to a Markov model with annual cycles. In addition, existing risk profiles commonly combine outcomes (such as chronic heart disease or cardiovascular disease, generally, compared to myocardial infarction or ischemic stroke, specifically—for example, see [21]). The distinction is particularly important to accurately estimating costs associated with disease. They may also exclude potentially policy-relevant risk factors (such as differentiating current smokers from recent quitters or former smokers), and/or include clinical risk factors that may not be salient to population-level policy evaluation (such as evidence of left ventricular hypertrophy in the risk of stroke—for example, see [22]). For these reasons, we used data from the Framingham Heart Study to derive and develop customized 1-year risk equations for use in ModelHealth: CVD.
We developed risk equations for eight outcomes: myocardial infarction (MI), ischemic stroke, hemorrhagic stroke, angina pectoris, congestive heart failure, intermittent claudication, non-specific cardiovascular disease-related death, and diabetes. The risk analysis uses the Original Cohort (beginning in 1948 with 5,209 attendees) and the Offspring (beginning in 1971 with 5,124 attendees) arms of the Framingham Heart Study. Data were sourced from the National Heart, Lung, and Blood Institute's (NHLBI's) Biologic Specimen and Data Repository Information Coordinating Center (BioLINCC), with approval and human subjects oversight from the HealthPartners Institute for Education and Research's Institutional Review Board [5, 6]. Statistical survival analysis was performed using Stata, Version 11 (Statacorp, College Station, TX).
To use as much of this rich data source as possible, allow for time-varying covariates, and provide for a direct estimate of annual risk, we adopted a parametric over the more common semi-parametric Cox proportional hazard approach in our analysis. Similar parametric methods have been previously explored and validated by Framingham Heart Study researchers [23]. Age, BMI, HDL, LDL, SBP, and one's disease history are all included as potential time-varying covariates in the analyses.
Because age accounts for time within a single person's life and because we do not have strong evidence with respect to the impact of secular time trends, we estimated an individual's risk using the exponential proportional hazards model (which has a time independent or “memoryless” property). Specifically, estimation was conducted using the streg command in Stata. Time independence is particularly important when estimating annual risk (i.e., t = 1), because the additional information in the shape parameter (i.e., embodied in the so-called accelerated failure time metric) is never appropriately used and may otherwise systematically over-or under-estimate risk in a one year context. The resulting exponential model is estimated with a person j likelihood function of the risk of an event (dj ∈ {0,1}) between t0j and tj is
with an individual's probability of an event in the next year equal to F(1) = 1 − e(−eβ0+xjβ).
Appendix B Table 3
Summary of Risk Equations Derived From Framingham Heart Study Data.
Baseline Risk of GI Bleeding Events
We estimate the baseline risk of gastrointestinal (GI) bleeding events using an analysis of Italian observational data [24]. Generally speaking, evidence suggests that men face higher risk of GI bleeds than women, and risk for both sexes increases with age. Probabilities for GI bleeding events are summarized in Table B4 below.
Appendix B Table 4
Summary of Risk for GI Bleeding Events in ModelHealth: CVD.
3.4. Treatment Effects
Aspirin for Primary Prevention
Model parameters for primary prevention are summarized in Table B5. CVD and bleeding relative risks were derived from seven low-dose primary prevention trials, defined as 100mg of aspirin per day or less, identified by the systematic evidence review [25-32].
Appendix B Table 5
Summary of Aspirin Treatment Effects (RR) for Primary Prevention of Cardiovascular Disease.
Aspirin for Secondary Prevention
Aspirin also may be initiated following a non-fatal CVD event for the purposes of reducing the risk of subsequent events (secondary prevention). A recent meta-analysis of 16 secondary prevention aspirin trials indicates a 31 percent reduction in MI risk (95% Rate Ratio [RR] CI: 0.60-0.80) and a 22 percent reduction in ischemic stroke risk (95% RR CI: 0.61-0.99) [33]. Similar to the primary prevention trials, secondary preventive use of aspirin does not show a statistically significant reduction in CVD-related or all-cause mortality.
Due to the relative rarity of hemorrhagic stroke and major GI bleeding and the smaller sample sizes of participants in secondary trials, the estimates of increased risk of adverse events from aspirin in secondary prevention are less precise. Instead of using these less precise estimates, we assume the increased risk of hemorrhagic stroke and GI bleeding from aspirin use in secondary prevention is the same as observed in the primary prevention trials. In all cases, we draw an individual-specific effect size from a triangle distribution based on the 95 percent confidence intervals. A summary of the aspirin treatment effects when used for primary prevention of CVD is given in Table B6.
Appendix B Table 6
Summary of Aspirin Treatment Effects for Secondary Prevention of Cardiovascular Disease.
Statins
Due to the overwhelming use of statins (i.e., HMG-CoA reductase inhibitors) in the treatment of high cholesterol—recent estimates suggest rates in excess of 90 percent of Americans seeking pharmacological treatment [34]—we simplified treatment of dyslipidemia in ModelHealth: CVD to this drug class. We used several recent (and/or otherwise relevant) meta-analyses/reviews of statins to identify major (of 1,000 or more persons) randomized controlled trials comparing lipid reduction associated with statins to a placebo [35-40]. Included trials—accounting for a total of 67,815 subjects—had a follow-up period of at least 52 weeks, involved subjects for primary or secondary prevention, were subject-blinded (at a minimum), and reported changes in LDL or HDL cholesterol as an outcome. Trials were excluded if additional (open label) lipid-lowering drugs were allowed for use in the placebo group (unless observed at rates lower than 10 percent). The trials included in our analysis are summarized in Table B7.
Appendix B Table 7
Summary of Statin Trials Included in Estimation of Treatment Effects.
To accommodate differential drug response according to baseline (only one included trial included stepped treatment in its experimental protocol [41]), we estimated treatment effects on cholesterol levels using a simple weighted ordinary least squares regression, with baseline LDL or HDL levels (respectively) as the only predictor:
The average effect size of statins on LDL was estimated to be a 42.9 mg/dL reduction, with an additional marginal impact of 0.014 mg/dL reduction per mg/dL of baseline LDL. The average effect size of statins on HDL was estimated to be a 2.2 mg/dL increase, with a marginal decremental impact of 0.017 mg/dL reduction per mg/dL of baseline HDL. These results indicate that the typical lipid modifying response to statin therapy is not highly sensitive to baseline lipid levels.
To accommodate interpersonal differences in the impact of drug therapy on LDL cholesterol in ModelHealth: CVD, we constructed a triangle distribution centered on the mean effect size described above, with upper and lower limits defined by the standard deviation in effect size observed in statin trials, to draw person-specific effect sizes. We estimated the standard deviation in LDL cholesterol reduction using a meta-analysis of (generally smaller/shorter) placebo controlled trials rather than the major trials summarized in Table B7, because the primary endpoints in these trials were cardiovascular disease outcomes (and as a result, standard deviations in cholesterol changes were not typically reported). We did find not good evidence on the interpersonal variability of treatment effects from statins on HDL, and we incorporate only mean treatment effects in this case.
Finally, all trials—with exception of WOSCOPS [49]—reported results solely based upon intention-to-treat analyses. The average weighted adherence to the treatment across study arms among included trials reporting this measure was 89.4 percent. To account for diminished average treatment effects attributable to non-adherence to prescribed therapy, we estimate an appropriate adjustment by dividing lipid impact by 0.9 in the base case. Statin treatment effects in ModelHealth: CVD are summarized in Table B8.
Appendix B Table 8
Summary of Statin Treatment Effects.
Antihypertensives
We used recent meta-analyses/reviews of antihypertensive therapy to identify major (of 1,000 or more persons) randomized controlled trials comparing blood pressure reduction associated with drug therapy to a placebo [50-58]. Included trials—accounting for a total of 54,863 subjects—had a follow-up period of at least 52 weeks, involved subjects for primary or secondary prevention, were subject-blinded (at a minimum), and reported changes in SBP as an outcome. In addition, due to the considerable heterogeneity in observed blood pressure lowering drug therapy strategies—including differences in first-line drugs, doses, and combinations [59]—we required treatment arm protocol to include stepped therapy (and preferably matched stepped therapy of a placebo in the control arm). Trials were excluded if additional (open label) blood pressure lowering drugs were allowed for use in the placebo group (unless observed at rates lower than 10 percent). The trials included in our analysis are summarized in Table B9.
Appendix B Table 9
Summary of Antihypertensive Drug Trials Included in Estimation of Treatment Effects.
To accommodate diverse treatment strategies (i.e., stepped and combination) with respect to baseline blood pressure relative to goal, we estimated treatment effects on blood pressure levels using a simple weighted ordinary least squares regression, with baseline SBP levels (respectively) as the only predictor:
The average effect size of antihypertensive drugs on SBP was estimated to be a 40.1 mmHg increase, counterintuitively, but this is offset by an additional marginal impact of 0.31 mmHg reduction per mmHg of baseline SBP (Table B10). Hence, the intercept on the treatment effect is negative, implying that antihypertensives begin to raise blood pressure around SBP baseline levels of 108 mmHg or lower. In practice, this threshold is well below standard SBP goals (140 mmHg for most patients, 135 mmHg for diabetics), and such blood pressure raising effects will not be invoked by the model.
Appendix B Table 10
Summary of Antihypertensive Drug Treatment Effects.
To accommodate interpersonal differences in the impact of drug therapy on SBP in ModelHealth: CVD, we constructed a triangle distribution centered on the mean effect size described above, with upper and lower limits defined by the standard deviation in effect size observed in the antihypertensive trials, to draw person-specific effect sizes. The standard deviation of drug treatment on SBP was estimated from the subset of trials from Table B9 that reported this measure [61, 67, 68].
Finally, all trials reported results solely based upon intention-to-treat analyses. The average weighted adherence to the treatment across study arms among included trials reporting this measure was 81.9 percent. To account for diminished average treatment effects attributable to non-adherence to prescribed therapy, we estimate an appropriate adjustment by dividing lipid impact by 0.8 in the base case. Average blood pressure lowering effects of antihypertensive drugs used in ModelHealth: CVD are summarized in Table B10.
3.5. Acceptance of Screening and Adherence to Treatment
Good evidence is lacking for the percentage of individuals who would accept prevention screening/counseling—in accordance with USPSTF recommendations—when offered. We assume 90 percent of individuals will accept any of the USPSTF-recommended clinical preventive services. This is implemented as a person-level parameter, such that a person who accepts screening will always do so and one who does not accept, will never do so.
Good and consistent evidence is also lacking for long-term adherence rates among those taking aspirin or drug therapy for the prevention of cardiovascular disease. Treatment adherence rates from clinical trials are generally not representative of the population. Individuals who enroll in a clinical trial are believed to be more motivated to regularly take study drugs, and clinical trial subjects also tend to receive more consistent and intensive attention from healthcare providers than does the general population. Retrospective or claims-based studies capture a more representative population (although, generally biased toward over-representing those with health insurance coverage), but these studies are likely to miss patients who are prescribed treatment but never fill a prescription (i.e., primary non-adherence) and overstate nonadherence for patients lost to other insurers, providers, lost coverage, etc. Due to such limitations, we restrict our assumptions to point estimates of average adherence in the cases of primary and secondary prevention.
Evidence regarding differences in adherence to lipid modifying and blood pressure lowering drug therapies is mixed [69-72]. Although factors such as cost (statin therapy is generally more expensive than antihypertensive therapy) and regimen complexity (antihypertensive treatment strategies can often incorporate use of two, three, or even four drugs in combination) could drive differences in adherence in drug therapies, we simplify by assuming similar average adherence between treating lipids and hypertension. Systematic reviews of antihypertensives show long-term adherence (i.e., 2 years or more) ranging typically (varying considerably by drug class) from 30 to 50 percent, with shorter-term adherence (i.e., 1 year or less) a bit higher [73, 74]. A recent review of adherence to statins shows slightly wider estimates in long-term adherence, typically ranging from roughly 20 to 70 percent [75]. Analyses in both cases suggest prior cardiovascular disease increases likelihood as much as 50-70 percent [75-77]. Taking this all into account, we assume 40 percent adherence to statins and antihypertensives for primary prevention in the base case, and we assume 60 percent adherence for secondary prevention.
Appendix B Table 11
Summary of Treatment Adherence Assumptions in ModelHealth: CVD.
3.6. Modeling smoking behavior
Overview
Individuals may be in one of four smoking states: never smoker, current smoker, recent quitter, or former smoker. The probability that an individual is in a given smoking state is determined by two sets of multivariate risk equations that account for age, sex, race/ethnicity, and—for those older than age 25—the lifetime educational attainment at introduction into the model. Similarly, the likelihood that an agent who is currently in the never-smoker state begins smoking within a given cycle is conditioned on his/her age, sex, race/ethnicity, and—if older than age 25—lifetime educational attainment. Two different data sources informed these risk equations. Estimates of risk equations corresponding to ages 18 and younger used Youth Risk Behavior Survey (YRBS) data [79]. Estimates of risk equations corresponding to ages 19 and older used data from the National Health Interview Survey (NHIS) [14].
Although the specific final multivariable risk equations vary in terms of covariates and dependent variables, several criteria were consistent. The statistical relationships between each covariate and other predictors were screened. If inclusion of a covariate violated assumptions (e.g., co-linearity, normality, disproportionate cell size) appropriate adjustments (e.g., center around mean, transformation, re-categorization) were made or its inclusion reconsidered. Interaction terms (e.g., differential rates of initiation between young women and young men, differential rates of cessation between African-Americans with higher education and those without a high-school diploma, etc.) were considered based on the following criteria: representing at least 10% of the larger groups (e.g., at least 10% of women and at least 10% of those younger than age 18, at least 10% of African-Americans within each educational category, etc.), and a coefficient significant at the 10% level.
Initial smoking status
A multinomial logistic regression with outcomes corresponding to the four smoking states was used to estimate the likelihood of an individual having an initial smoking status given his/her age, sex, race/ethnicity, and lifetime educational attainment. The estimated distribution across potential smoking states was used to determine each agent's initial smoking status at introduction into the model.
Neither the YRBS nor the NHIS directly asks respondents about their current smoking status. Instead, the following definitions were used:
Never smoker: | Having smoked fewer than 100 cigarettes in their lifetime |
Current smoker: | Having smoked at least 100 cigarettes in their lifetime and having smoked in the last week |
Recent quitter: | Having smoked at least 100 cigarettes in their lifetime and having quit for less than 4 years |
Former smoker: | Having smoked at least 100 cigarettes in their lifetime and having quit for 4 or more years |
The usual definitional prerequisite of having smoked at least 100 cigarettes in their lifetime was applied to exclude experimental smoking. The results of the estimation are contained in Table B12. Time in state (i.e., the number of years as a smoker and/or the number of years since quitting) partially determines the likelihood of quitting or relapsing. An age of initiation is assigned to those initialized as current smokers, recent quitters, or former smokers. For those initialized as recent quitters or former smokers, an age of cessation also is assigned.
Appendix B Table 12
Results of Multinomial Estimation Predicting Initial Smoking Status.
Smoking status initialization is implemented in a two-step process. In Step 1, for all agents initialized as a current smoker, recent quitter, or former smoker, a random draw (from a distribution drawn configured to initiation rates estimated from the NHIS) determines the age at which the person first started smoking (e.g., age 19). Then, for those initialized as recent quitters and former smokers (Step 2), a random draw from a second distribution configured to cessation rates estimated from NHIS and truncated at the age of initiation determines the age of cessation (e.g., age 26). These two ages are used to determine the time spent smoking and time since cessation, which are used in the model when determining future smoking behavior.
Lifetime smoking behavior
An individual's “risk” of changing smoking status (i.e., transitioning to another smoking state), is determined by current state, time in that state, and demographics. Individuals who have never smoked can either remain in the never smoker state or begin smoking and transition to the current smoker state. A current smoker who is in the current smoker state can remain or quit and transition to the recent quitter state. A recent quitter either remains in the recent quitter state, relapses into the current smoker state, or moves to the former smoker state once four years have passed. A former smoker either relapses into the current smoker state or remains in the former smoker state.
Three separate logistic regressions determine the risk of smoking initiation by comparing initiators to never smokers. The first, which uses YBRS data, applies to ages younger than 18. The second and third, which use NHIS data, applied to ages 18-24 and 25 and older, respectively. Similar to the initial smoking status risk equations, the 19-24 specification was distinguished by inclusion of lifetime educational achievement. Tables B13 and B14 contain the results of these estimations.
Appendix B Table 13
Youth Tobacco Smoking Initiation Rates.
Appendix B Table 14
Results of Logistic Regressions Predicting Adult Smoking Status.
We assumed no cessation among youth younger than age 18 and estimated two cessation risk equations for adults. From the NHIS data, we identified quitters as those indicating they had ceased cigarette use within the last 12 months with no indication of relapse. Two logistic regressions (18-24 and 25 and older) compared quitters to current smokers to determine the likelihood of smoking cessation. Once again, the 19-24 specification was distinguished by inclusion of lifetime educational achievement.
Relapse after quitting tobacco use is time-sensitive. The longer a person has successfully quit smoking, the less likely he or she is to relapse. The cross-sectional design of both the YBRS and NHIS surveys made estimation of relapse rates that account for time since cessation difficult. Instead, we used published estimates based on longitudinal studies. These values were adjusted during calibration to provide reasonable values of age-, sex-, and race/ethnicity-specific tobacco use rates. Table B15 contains these rates.
Appendix B Table 15
Baseline Smoking Tobacco Relapse Rates.
Calibration of smoking behaviors to CBO model
Tobacco prevalence was calibrated to reflect baseline tobacco use projections of the Congressional Budget Office (CBO) prior to final analysis [15]. These calibrated initiation and cessation rates are used for all estimates. We were unable to obtain details regarding how the CBO parameterizes specific population groups. Instead, we worked with estimates derived from Figure 1-1 in the 2012 CBO report (page 3) [15]. CBO only reports its projection of smoking prevalence among all adults in Figure 1-1. Our model determines annual smoking prevalence based on initiation, cessation and relapse, as mediated by sex, age, race-ethnicity and educational attainment. The average adult smoking prevalence reported in Figure 1-1 of the CBO report could have been be reproduced with infinite number of changes to patterns of smoking initiation, cessation, and relapse rates among males and females of different ages, race/ethnicities, and educational attainment. In addition, predictions of smoking prevalence among adults depend heavily on recent, current and near-term teen smoking initiation rates. Therefore, with only Figure 1-1 and a general description of the CBO's approach as a guide, we tested a reasonable set of parameter modifications to adjust the smoking prevalence rates produced by our model over the next 10 years to better reflect CBO's baseline.
Three key sources of deviation from the CBO model were identified and adjusted for within the model. The first source was the estimated initiation patterns from NHIS age-based categories that created a stepped function and subsequent “jagged” initiation patterns. The resolution was to smooth initiation rates using a moving average process across ages that held constant prevalence within each age group. This adjustment removed “jumps” in prevalence among birth cohorts, but initiation remained relatively high.
The second source of deviation was that NHIS-based estimates suggest stable or increasing smoking prevalence among young adults and adolescents. Thus, prevalence in the original model differed from the CBO model, which shows a secular trend toward decreasing prevalence over time. The resolution to this issue was to decrease initiation rates across lower age ranges by lowering implied prevalence to 24-year-old prevalence and smoothing using a 10-year moving average process. The effect of this was a lowered prevalence among new birth cohorts that was a closer approximation to initial cohort and a prevalence pattern that approximated those of current 10- to 24-year-olds. This results in a new “steady-state” population prevalence of approximately 13-14%, which is lower than the current population-wide prevalence. Finally, the third source of deviation was that initial former smokers exhibited high relapse rates among older age groups (ages 50 or older), causing higher prevalence relative to the CBO model. The approach to resolve this issue was to utilize an exponential distribution, which decreased likelihood of relapse among initial former smokers, and relapse was eliminated for former smokers older than age 50.
3.7. Modeling cancer
Modeling cancer incidence and fatality
Cancers were modeled using an incidence and case-fatality rate approach, which tracked cancer incidence and mortality for each agent. Within the model, four categories of cancer were modeled: 1) trachea, lung, and bronchus, 2) colorectal cancer, 3) other cancers with smoking-attributable risk, and 4) other cancers with no smoking-attributable risk. Lung, bronchial and trachea site and morphology are: lung and bronchus, trachea, mediastinum and other respiratory organs. Colon and rectal site and morphology are: colon and rectum. All smoking-related site and morphology are: oral cavity and pharynx, esophagus, stomach, liver, pancreas, larynx, lung and bronchus, cervix uteri, urinary bladder, kidney and renal pelvis, acute myeloid leukemia. Site and morphology for cancers unrelated to smoking are: oral cavity and pharynx, esophagus, stomach, colon and rectum, liver, pancreas, larynx, lung and bronchus, cervix uteri, urinary bladder, kidney and renal pelvis, acute myeloid leukemia. These categories were used to sharpen adjustments by smoking behavior, aspirin effectiveness, and racial and ethnic differences.
Baseline incidence and case fatality rates by age and sex for each cancer category were estimated from Surveillance, Epidemiology, and End Results (SEER) data using SEER*Stat software [82]. Rates for colorectal cancer also were stratified by race/ethnicity. These baseline incidence and case-fatality rates were further adjusted by the age, sex and smoking status specific relative risks provided by the Smoking-Attributable Mortality, Morbidity, and Economic Costs (SAMMEC) tool maintained by the Center for Disease Control (CDC) [83]. Final incidence and case fatality rates are listed in Tables B17-B20.
Appendix B Table 16
Cancer Incidence and Case-Fatality Rates of Trachea, Lung, and Bronchus.
Appendix B Table 17
Colorectal Cancer Incidence Rates.
Appendix B Table 18
Colorectal Case-Fatality Rates.
Appendix B Table 19
Incidence and Case-Fatality of Other Cancers With Smoking-Attributable Risk.
Appendix B Table 20
Incidence and Case-Fatality of Other Cancers With No Smoking-Attributable Risk.
Although each of the four cancer categories has unique risks, durations, and quality-adjusted life year (QALY) decrements, the basic algorithm employed to model disease incidence and burden is the same across all four categories. This algorithm is presented in Figure B2.

Appendix B Figure 2
Algorithm for Modeling Cancer Incidence and Case Fatality.
For each cancer category, the model first checks to see if the agent is experiencing a current cancer episode. If they are, their time in that state (i.e., dwell time) is checked to determine if the it has expired. If the dwell time has expired, the episode's terminal condition (death or resolution) is checked. If an episode's dwell time has not expired, disease and terminal condition-specific QALY decrements are applied and the episode continues.
If the agent is not in a current cancer episode, the model determines if a new cancer episode has begun. If it has, the eventual terminal condition of that state (death or resolution) is determined. The duration (dwell time) and QALY decrements of the cancer episodes are contained in Table B21.
Appendix B Table 21
Duration and QALY Decrement for Cancer Episodes.
5 References
- 1.
- Whitlock EP, et al. Aspirin Use in Adults: Cancer, All-Cause Mortality and Harm. Agency for Healthcare Research and Quality; Rockville, MD: 2015. (Prepared by Kaiser Permanente Research Affiliates Evidence-based Practice Center under Contract No. HHSA-290-2012-00151-I, Task Order No. 4.) [PubMed: 26491756]
- 2.
- Chubak J, et al. Aspirin Use for the Prevention of Colorectal Cancer: An Updated Systematic Evidence Review for the U.S. Preventive Services Task Force. Agency for Healthcare Research and Quality; Rockville, MD: 2015. (Prepared by the Kaiser Permanente Research Affiliated Evidence-based Practice Center under Contract No. HHSA-2900-2012-00015-I, Task Order No. 2) [PubMed: 26491758]
- 3.
- Third Report of the National Cholesterol Education Program (NCEP) Expert Panel on Detection, Evaluation, and Treatment of High Blood Cholesterol in Adults (Adult Treatment Panel III) final report. Circulation. 2002;106(25):3143–421. [PubMed: 12485966]
- 4.
- Chobanian AV, et al. Seventh report of the Joint National Committee on Prevention, Detection, Evaluation, and Treatment of High Blood Pressure. Hypertension. 2003;42(6):1206–52. [PubMed: 14656957]
- 5.
- Framingham Heart Study-Cohort. Biologic Specimen and Data Repository Information Coordinating Center, National Institutes of Health; 2010.
- 6.
- Framingham Heart Study-Offspring. Biologic Specimen and Data Repository Information Coordinating Center, National Institutes of Health; 2010.
- 7.
- Ruggles S, et al. Integrated Public Use Microdata Series: IPUMS-USA, American Community Survey 2011 3-yr Sample. Minneapolis, MN: Minnesota Population Center; 2013.
- 8.
- King M, et al. Integrated Public Use Microdata Series, Current Population Survey: 2009-2012. Minneapolis, MN: Minnesota Population Center; 2014.
- 9.
- Centers for Disease Control and Prevention. National Health and Nutrition Examination Survey Data (2001-2002). Hyattsville, MD: U.S. Department of Health and Human Services, Centers for Disease Control and Prevention; 2004.
- 10.
- Centers for Disease Control and Prevention. National Health and Nutrition Examination Survey Data (2003-2004). Hyattsville, MD: U.S. Department of Health and Human Services, Centers for Disease Control and Prevention; 2005.
- 11.
- Centers for Disease Control and Prevention. National Health and Nutrition Examination Survey Data (2005-2006). Hyattsville, MD: U.S. Department of Health and Human Services, Centers for Disease Control and Prevention; 2007.
- 12.
- Centers for Disease Control and Prevention. National Health and Nutrition Examination Survey Data (2007-2008). Hyattsville, MD: U.S. Department of Health and Human Services, Centers for Disease Control and Prevention; 2009.
- 13.
- Centers for Disease Control and Prevention. National Health and Nutrition Examination Survey Data (2009-2010). Hyattsville, MD: U.S. Department of Health and Human Services, Centers for Disease Control and Prevention; 2011.
- 14.
- National Center for Health Statistics. National Health Interview Survey, 2007. Hyattsville, Maryland: National Center for Health Statistics, Centers for Disease Control and Prevention; 2008.
- 15.
- Congressional Budget Office. Raising the Excise Tax on Cigarettes: Effects on Health and the Federal Budget. Congressional Budget Office; Washington: 2012.
- 16.
- Centers for Disease Control and Prevention. Behavioral Risk Factor Surveillance System Survey Data (2009). Atlanta, Georgia: U.S. Department of Health and Human Services, Centers for Disease Control and Prevention; 2010.
- 17.
- Assmann G, et al. Assessing risk of myocardial infarction and stroke: new data from the Prospective Cardiovascular Munster (PROCAM) study. Eur J Clin Invest. 2007;37(12):925–32. [PubMed: 18036028]
- 18.
- Conroy RM, et al. Estimation of ten-year risk of fatal cardiovascular disease in Europe: the SCORE project. Eur Heart J. 2003;24(11):987–1003. [PubMed: 12788299]
- 19.
- Hippisley-Cox J, et al. Derivation and validation of QRISK, a new cardiovascular disease risk score for the United Kingdom: prospective open cohort study. BMJ. 2007;335(7611):136. [PMC free article: PMC1925200] [PubMed: 17615182]
- 20.
- D'Agostino RB Sr., et al. General cardiovascular risk profile for use in primary care: the Framingham Heart Study. Circulation. 2008;117(6):743–53. [PubMed: 18212285]
- 21.
- Wilson PW, et al. Prediction of coronary heart disease using risk factor categories. Circulation. 1998;97(18):1837–47. [PubMed: 9603539]
- 22.
- D'Agostino RB, et al. Stroke risk profile: adjustment for antihypertensive medication. The Framingham Study. Stroke. 1994;25(1):40–3. [PubMed: 8266381]
- 23.
- Odell PM, Anderson KM, Kannel WB. New models for predicting cardiovascular events. J Clin Epidemiol. 1994;47(6):583–92. [PubMed: 7722571]
- 24.
- De Berardis G, et al. Association of aspirin use with major bleeding in patients with and without diabetes. JAMA. 2012;307(21):2286–94. [PubMed: 22706834]
- 25.
- Guirguis-Blake JM, et al. Aspirin for the Primary Prevention of Cardiovascular Events: A Systematic Evidence Review for the U.S. Preventive Services Task Force. Agency for Healthcare Research and Quality; Rockville, MD: 2015. (Prepared by Kaiser Permanente Research Affiliates Evidence-based Practice Center under Contract No HHSA-290-2012-00015-EPC4, Task Order 2) [PubMed: 26491760]
- 26.
- Fowkes FG, et al. Aspirin for prevention of cardiovascular events in a general population screened for a low ankle brachial index: a randomized controlled trial. JAMA. 2010;303(9):841–8. [PubMed: 20197530]
- 27.
- Hansson L, et al. Effects of intensive blood-pressure lowering and low-dose aspirin in patients with hypertension: principal results of the Hypertension Optimal Treatment (HOT) randomised trial. HOT Study Group. Lancet. 1998;351(9118):1755–62. [PubMed: 9635947]
- 28.
- Ogawa H, et al. Low-dose aspirin for primary prevention of atherosclerotic events in patients with type 2 diabetes: a randomized controlled trial. JAMA. 2008;300(18):2134–41. [PubMed: 18997198]
- 29.
- Belch J, et al. The prevention of progression of arterial disease and diabetes (POPADAD) trial: factorial randomised placebo controlled trial of aspirin and antioxidants in patients with diabetes and asymptomatic peripheral arterial disease. BMJ. 2008;337:a1840. [PMC free article: PMC2658865] [PubMed: 18927173]
- 30.
- de Gaetano G. Low-dose aspirin and vitamin E in people at cardiovascular risk: a randomised trial in general practice. Collaborative Group of the Primary Prevention Project. Lancet. 2001;357(9250):89–95. [PubMed: 11197445]
- 31.
- Thrombosis prevention trial: randomised trial of low-intensity oral anticoagulation with warfarin and low-dose aspirin in the primary prevention of ischaemic heart disease in men at increased risk. The Medical Research Council's General Practice Research Framework. Lancet. 1998;351(9098):233–41. [PubMed: 9457092]
- 32.
- Ridker PM, et al. A randomized trial of low-dose aspirin in the primary prevention of cardiovascular disease in women. N Engl J Med. 2005:1293–304. [PubMed: 15753114]
- 33.
- Baigent C, et al. Aspirin in the primary and secondary prevention of vascular disease: collaborative meta-analysis of individual participant data from randomised trials. Lancet. 2009;373(9678):1849–60. [PMC free article: PMC2715005] [PubMed: 19482214]
- 34.
- Mann D, et al. Trends in statin use and low-density lipoprotein cholesterol levels among US adults: impact of the 2001 National Cholesterol Education Program guidelines. Ann Pharmacother. 2008;42(9):1208–15. [PubMed: 18648016]
- 35.
- Taylor F, et al. Statins for the primary prevention of cardiovascular disease. Cochrane Database Syst Rev. 2011;(1) CD004816. [PMC free article: PMC4164175] [PubMed: 21249663]
- 36.
- Ward S, et al. A systematic review and economic evaluation of statins for the prevention of coronary events. Health Technol Assess. 2007;11(14):1–160. iii–iv. [PubMed: 17408535]
- 37.
- Rogers SL, et al. A dose-specific meta-analysis of lipid changes in randomized controlled trials of atorvastatin and simvastatin. Clin Ther. 2007;29(2):242–52. [PubMed: 17472817]
- 38.
- Baigent C, et al. Efficacy and safety of cholesterol-lowering treatment: prospective meta-analysis of data from 90,056 participants in 14 randomised trials of statins. Lancet. 2005;366(9493):1267–78. [PubMed: 16214597]
- 39.
- Law MR, Wald NJ, Rudnicka AR. Quantifying effect of statins on low density lipoprotein cholesterol, ischaemic heart disease, and stroke: systematic review and meta-analysis. BMJ. 2003;326(7404):1423. [PMC free article: PMC162260] [PubMed: 12829554]
- 40.
- Edwards JE, Moore RA. Statins in hypercholesterolaemia: a dose-specific meta-analysis of lipid changes in randomised, double blind trials. BMC Fam Pract. 2003;4:18. [PMC free article: PMC317299] [PubMed: 14969594]
- 41.
- Randomised trial of cholesterol lowering in 4444 patients with coronary heart disease: the Scandinavian Simvastatin Survival Study (4S). Lancet. 1994;344(8934):1383–9. [PubMed: 7968073]
- 42.
- Downs JR, et al. Primary prevention of acute coronary events with lovastatin in men and women with average cholesterol levels: results of AFCAPS/TexCAPS. Air Force/Texas Coronary Atherosclerosis Prevention Study. JAMA. 1998;279(20):1615–22. [PubMed: 9613910]
- 43.
- Holdaas H, et al. Effect of fluvastatin on cardiac outcomes in renal transplant recipients: a multicentre, randomised, placebo-controlled trial. Lancet. 2003;361(9374):2024–31. [PubMed: 12814712]
- 44.
- Sever PS, et al. Prevention of coronary and stroke events with atorvastatin in hypertensive patients who have average or lower-than-average cholesterol concentrations, in the Anglo-Scandinavian Cardiac Outcomes Trial--Lipid Lowering Arm (ASCOT-LLA): a multicentre randomised controlled trial. Lancet. 2003;361(9364):1149–58. [PubMed: 12686036]
- 45.
- Knopp RH, et al. Efficacy and safety of atorvastatin in the prevention of cardiovascular end points in subjects with type 2 diabetes: the Atorvastatin Study for Prevention of Coronary Heart Disease Endpoints in non-insulin-dependent diabetes mellitus (ASPEN). Diabetes Care. 2006;29(7):1478–85. [PubMed: 16801565]
- 46.
- MRC/BHF Heart Protection Study of antioxidant vitamin supplementation in 20,536 high-risk individuals: a randomised placebo-controlled trial. Lancet. 2002;360(9326):23–33. [PubMed: 12114037]
- 47.
- Prevention of cardiovascular events and death with pravastatin in patients with coronary heart disease and a broad range of initial cholesterol levels. The Long-Term Intervention with Pravastatin in Ischaemic Disease (LIPID) Study Group. N Engl J Med. 1998;339(19):1349–57. [PubMed: 9841303]
- 48.
- Shepherd J, et al. Pravastatin in elderly individuals at risk of vascular disease (PROSPER): a randomised controlled trial. Lancet. 2002;360(9346):1623–30. [PubMed: 12457784]
- 49.
- Shepherd J, et al. Prevention of coronary heart disease with pravastatin in men with hypercholesterolemia. West of Scotland Coronary Prevention Study Group. N Engl J Med. 1995;333(20):1301–7. [PubMed: 7566020]
- 50.
- Howe AJ, Shand JA, Menown IB. Advances in cardiology: clinical trial update. Future Cardiol. 2011;7(3):299–310. [PubMed: 21627472]
- 51.
- Czernichow S, et al. The effects of blood pressure reduction and of different blood pressure-lowering regimens on major cardiovascular events according to baseline blood pressure: meta-analysis of randomized trials. J Hypertens. 2011;29(1):4–16. [PubMed: 20881867]
- 52.
- Sever PS, Messerli FH. Hypertension management 2011: optimal combination therapy. Eur Heart J. 2011;32(20):2499–506. [PubMed: 21697169]
- 53.
- Staessen JA, et al. Implications of recently published trials of blood pressure-lowering drugs in hypertensive or high-risk patients. Hypertension. 2010;55(4):819–31. [PubMed: 20212274]
- 54.
- Law MR, Morris JK, Wald NJ. Use of blood pressure lowering drugs in the prevention of cardiovascular disease: meta-analysis of 147 randomised trials in the context of expectations from prospective epidemiological studies. BMJ. 2009;338:b1665. [PMC free article: PMC2684577] [PubMed: 19454737]
- 55.
- Wright JM, Musini VM. First-line drugs for hypertension. Cochrane Database Syst Rev. 2009;(3) CD001841. [PubMed: 19588327]
- 56.
- Gaffney SM, et al. Key articles and guidelines in the management of hypertension: 2008 update. Pharmacotherapy. 2008;28(8):1041–58. [PubMed: 18657020]
- 57.
- Wang JG, et al. Systolic and diastolic blood pressure lowering as determinants of cardiovascular outcome. Hypertension. 2005;45(5):907–13. [PubMed: 15837826]
- 58.
- Law M, Wald N, Morris J. Lowering blood pressure to prevent myocardial infarction and stroke: a new preventive strategy. Health Technol Assess. 2003;7(31):1–94. [PubMed: 14604498]
- 59.
- Ma J, Stafford RS. Screening, treatment, and control of hypertension in US private physician offices, 2003-2004. Hypertension. 2008;51(5):1275–81. [PMC free article: PMC2671992] [PubMed: 18347229]
- 60.
- Liu L, et al. The Felodipine Event Reduction (FEVER) Study: a randomized long-term placebo-controlled trial in Chinese hypertensive patients. J Hypertens. 2005;23(12):2157–72. [PubMed: 16269957]
- 61.
- Beckett NS, et al. Treatment of hypertension in patients 80 years of age or older. N Engl J Med. 2008;358(18):1887–98. [PubMed: 18378519]
- 62.
- MRC trial of treatment of mild hypertension: principal results. Medical Research Council Working Party. Br Med J (Clin Res Ed). 1985;291(6488):97–104. [PMC free article: PMC1416260] [PubMed: 2861880]
- 63.
- Medical Research Council trial of treatment of hypertension in older adults: principal results. MRC Working Party. BMJ. 1992;304(6824):405–12. [PMC free article: PMC1995577] [PubMed: 1445513]
- 64.
- Randomised trial of a perindopril-based blood-pressure-lowering regimen among 6,105 individuals with previous stroke or transient ischaemic attack. Lancet. 2001;358(9287):1033–41. [PubMed: 11589932]
- 65.
- Prevention of stroke by antihypertensive drug treatment in older persons with isolated systolic hypertension. Final results of the Systolic Hypertension in the Elderly Program (SHEP). SHEP Cooperative Research Group. JAMA. 1991;265(24):3255–64. [PubMed: 2046107]
- 66.
- Dahlof B, et al. Morbidity and mortality in the Swedish Trial in Old Patients with Hypertension (STOP-Hypertension). Lancet. 1991;338(8778):1281–5. [PubMed: 1682683]
- 67.
- Liu L, et al. Comparison of active treatment and placebo in older Chinese patients with isolated systolic hypertension. Systolic Hypertension in China (Syst-China) Collaborative Group. J Hypertens. 1998;16(12 Pt 1):1823–9. [PubMed: 9869017]
- 68.
- Staessen JA, et al. Randomised double-blind comparison of placebo and active treatment for older patients with isolated systolic hypertension. The Systolic Hypertension in Europe (Syst-Eur) Trial Investigators. Lancet. 1997;350(9080):757–64. [PubMed: 9297994]
- 69.
- Newby LK, et al. Long-term adherence to evidence-based secondary prevention therapies in coronary artery disease. Circulation. 2006;113(2):203–12. [PubMed: 16401776]
- 70.
- Baroletti S, Dell'Orfano H. Medication adherence in cardiovascular disease. Circulation. 2010;121(12):1455–8. [PubMed: 20351303]
- 71.
- Kulkarni SP, et al. Long-term adherence with cardiovascular drug regimens. Am Heart J. 2006;151(1):185–91. [PubMed: 16368315]
- 72.
- Yeaw J, et al. Comparing adherence and persistence across 6 chronic medication classes. J Manag Care Pharm. 2009;15(9):728–40. [PMC free article: PMC10441195] [PubMed: 19954264]
- 73.
- Kronish IM, et al. Meta-analysis: impact of drug class on adherence to antihypertensives. Circulation. 2011;123(15):1611–21. [PMC free article: PMC3084582] [PubMed: 21464050]
- 74.
- Elliott WJ. Improving outcomes in hypertensive patients: focus on adherence and persistence with antihypertensive therapy. J Clin Hypertens (Greenwich). 2009;11(7):376–82. [PMC free article: PMC8673138] [PubMed: 19583634]
- 75.
- Mann DM, et al. Predictors of nonadherence to statins: a systematic review and meta-analysis. Ann Pharmacother. 2010;44(9):1410–21. [PMC free article: PMC3641194] [PubMed: 20702755]
- 76.
- Benner JS, et al. Long-term persistence in use of statin therapy in elderly patients. JAMA. 2002;288(4):455–61. [PubMed: 12132975]
- 77.
- Esposti LD, et al. Pharmacoeconomics of antihypertensive drug treatment: an analysis of how long patients remain on various antihypertensive therapies. J Clin Hypertens (Greenwich). 2004;6(2):76–84. [PMC free article: PMC8109607] [PubMed: 14872145]
- 78.
- Pignone M, et al. Aspirin use among adults aged 40 and older in the United States: results of a national survey. Am J Prev Med. 2007;32(5):403–407. [PubMed: 17478266]
- 79.
- Centers for Disease Control and Prevention. Youth Risk Behavior Survey (YRBS). 2011.
- 80.
- Hughes JR, et al. Measures of abstinence in clinical trials: issues and recommendations. Nicotine Tob Res. 2003;5(1):13–25. [PubMed: 12745503]
- 81.
- Wetter DW, et al. Late relapse/sustained abstinence among former smokers: a longitudinal study. Prev Med. 2004;39(6):1156–63. [PubMed: 15539050]
- 82.
- Surveillance Research Program, National Cancer Institute. SEER*Stat software version 8.1.5.
- 83.
- Center for Disease Control and Prevention. Smoking-Attributable Mortality, Morbidity, and Economic Costs (SAMMEC): Adult SAMMEC and Maternal and Child Health (MCH) SAMMEC software. 2007.
- Model Technical Documentation - Aspirin Use to Prevent Cardiovascular Disease an...Model Technical Documentation - Aspirin Use to Prevent Cardiovascular Disease and Colorectal Cancer
- Cronobacter sakazakii strain:FA-08Cronobacter sakazakii strain:FA-08Isolation and characterization of glyphosate and AMPA-degrading strains from agricultural soils in Mexico.BioProject
Your browsing activity is empty.
Activity recording is turned off.
See more...