NCBI Bookshelf. A service of the National Library of Medicine, National Institutes of Health.
Making Healthcare Safer IV: A Continuous Updating of Patient Safety Harms and Practices [Internet]. Rockville (MD): Agency for Healthcare Research and Quality (US); 2023 Jul-.
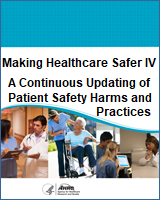
Making Healthcare Safer IV: A Continuous Updating of Patient Safety Harms and Practices [Internet].
Show detailsStructured Abstract
Objectives:
Patient safety practices (PSPs) focused on sepsis prediction and recognition, encompass interventions designed to identify patients with sepsis early and improve timely adherence to guidelines. Our objectives were to review the evidence published after the previous Making Healthcare Safer (MHS) report to determine the effectiveness of sepsis prediction and recognition PSPs on patient safety related outcomes.
Methods:
We searched PubMed and the Cochrane library for systematic reviews and primary studies published from January 2018 through August 2023, supplemented by gray literature searches. We included reviews and primary studies of sepsis prediction and recognition PSPs reporting measures of clinical process (time to diagnosis or treatment, adherence to guidelines, Severe Sepsis and Septic Shock Early Management Bundle), patient outcomes (hospital or intensive care unit (ICU) length of stay, mortality), implementation (use, barriers, and facilitators), or costs.
Findings:
We focused on 7 systematic reviews and 8 primary studies that were eligible for full review, and briefly summarized 36 pre-post studies that lacked a separate comparison group. All the sepsis prediction and recognition PSPs were multi-component interventions. Across the systematic reviews and primary studies of neonates, the PSPs improved clinical process measures (low strength of evidence), but evidence was insufficient about length of stay or mortality outcomes. Across the systematic reviews and primary studies of adults, the PSPs did not demonstrate an effect on clinical process, length of stay, or mortality outcomes. In primary studies of adults, evidence was insufficient in the prehospital setting for mortality, length of stay, and clinical process measures. In the emergency department setting, strength of evidence was low for mortality and clinical process measures and insufficient for length of stay. In ward or hospitalwide settings, strength of evidence was low across all three outcome types. The secondary outcome of alerting system performance (e.g., positive predictive value) could not be meaningfully compared across studies due to diversity in populations and interventions.
Conclusions:
This review finds that recent primary studies and systematic reviews do not support that specific PSPs for sepsis prediction and recognition are effective at reducing mortality or length of stay or improve clinical processes in adults in pre-hospital, emergency department, or hospitalwide settings as compared to usual care. Sepsis prediction and recognition PSPs may improve clinical process outcomes in neonates in ICUs.
1. Background and Purpose
The Agency for Healthcare Research and Quality (AHRQ) Making Healthcare Safer (MHS) reports consolidate information for healthcare providers, health system administrators, researchers, and government agencies about practices that can improve patient safety across the healthcare system — from hospitals to primary care practices, long-term care facilities, and other healthcare settings. In Spring of 2023, AHRQ launched its fourth iteration of the MHS Report (MHS IV). Sepsis prediction and recognition PSPs were prioritized for inclusion in the MHS IV series based on a modified Delphi technique used by a Technical Expert Panel (TEP) that met in December 2022. The TEP included 15 experts in patient safety with representatives of governmental agencies, healthcare stakeholders, clinical specialists, experts in patient safety issues, and a patient/consumer perspective. See the MHS IV Prioritization Report for additional details.1
Sepsis is a life-threatening medical emergency involving a dysregulated host response to an infection, most commonly bacterial, wherein the host response damages tissues and organs. Anyone can be affected by sepsis, although neonates, young children, pregnant or recently-pregnant women, older persons and individuals with underlying chronic conditions are at an elevated risk.2 Sepsis is common, life-threatening, and financially burdensome.2,3 The Centers for Disease Control and Prevention (CDC) estimate that at least 1.7 million adults in America develop sepsis every year, and one in three people who die in a hospital had sepsis during their hospital stay.4 The estimated annual cost of sepsis for Medicare beneficiaries is $41.5 billion, with mortality rates for sepsis ranging from 27% for unspecified sepsis diagnoses to 60% for septic shock diagnoses.3 Early detection and treatment of sepsis greatly impacts outcomes,5,6 spurring efforts toward rapid detection and intervention. However, diagnosis is challenging because common sepsis symptoms are nonspecific (e.g., fever, nausea, vomiting, muscle pain), particularly early in the course, and sepsis has variable presentations. The human and financial burdens of sepsis spurred the development of the ‘Surviving Sepsis’ campaign over twenty years ago and have driven continued evolution of sepsis diagnostic criteria, treatment guidelines, and care bundles.7 In 2018, the Centers for Medicare & Medicaid Services (CMS) launched the Severe Sepsis and Septic Shock Early Management Bundle (SEP-1) performance measure. Adherence to this bundle of interventions and tasks varies greatly across institutions, with conflicting results about the association of the SEP-1 performance measure and clinical outcomes.8,9 Such studies have renewed calls for new sepsis interventions8,9 and better performance measures.10
1.1. Overview of the Patient Safety Practice
PSPs that focus on sepsis prediction and recognition seek to identify patients with sepsis early and improve timely adherence to best practice guidelines. The MHS III report included three categories of PSPs with goals of improving patient outcomes: manual screening tools, automated alerting systems, and multicomponent sepsis interventions.11 For manual screening tools, the MHS III report found variable sensitivity and specificity across tools and settings with particularly poor performance in the pre-hospital setting. The MHS III report also found moderate strength of evidence linking manual screening tool use to process measure improvement (e.g., time to treatment), but only sparse evidence supporting an impact on patient outcomes (e.g., mortality, hospital length of stay, intensive care unit transfer). For automated systems, the MHS III report concluded that results across studies were inconsistent, but the strength of evidence was moderate linking use of automated systems to improved process and outcome measures. The multicomponent sepsis interventions included in the MHS III review were multifaceted programs aimed at improving the full spectrum of sepsis recognition and care. All five of the included PSPs had a manual screening tool or patient monitoring system component, but the other components of each program varied. All studies of the multicomponent sepsis interventions reported improvement in at least one process measure, but only two showed improvements in outcome measures. During the prioritization process, the MHS IV TEP reached 100% consensus on inclusion of sepsis prediction and recognition PSPs in the MHS IV report and did not suggest changes to the definitions or scope.
1.2. Purpose of the Rapid Review
The overall purpose of this review is to determine the effectiveness of sepsis prediction and recognition PSPs including the impact these PSPs have on clinical process measures (e.g., timeliness of diagnosis and treatment, and adherence to clinical best practices), patient outcomes (e.g., mortality and length of stay) and implementation measures (e.g., clinician use of predictive system recommendations, and barriers and facilitators to implementation). Additionally, this review describes the performance (e.g., sensitivity and specificity) of risk assessment tools and automated predictive systems. We provide information on other contextual issues to help interpret the results of this review.
1.3. Review Questions
- What is the frequency and severity of harm associated with sepsis?
- In PSPs designed to improve the prediction or recognition of sepsis, what patient safety measures or indicators have been used to examine the harms associated with sepsis?
- What PSPs have been used to improve sepsis prediction or recognition and in what settings have they been used?
- What is the rationale for PSPs used to improve sepsis prediction or recognition?
- What are the effectiveness and unintended effects of PSPs designed to improve the prediction or recognition of sepsis, and what new evidence has been published since the search was done for the MHS III report in 2019?
- What are common barriers and facilitators to implementing PSPs targeting the prediction or recognition of sepsis?
- What resources (e.g., cost, staff, time) are required for implementation?
- What toolkits are available to support implementation of the PSPs?
2. Methods
We followed processes proposed by the Agency for Healthcare Research and Quality (AHRQ) Evidence-based Practice Center (EPC) Program. The final protocol for this rapid review is posted on the AHRQ website at: https://www.ahrq.gov/research/findings/making-healthcare-safer/mhs4/index.html. We registered the protocol for this rapid review in PROSPERO.
For this rapid review, strategic adjustments were made to streamline traditional systematic review processes and deliver an evidence product in the allotted time. Adjustments included being highly specific about the questions, limiting the number of databases searched, modifying search strategies to focus on finding the most valuable studies (i.e., being flexible on sensitivity to increase the specificity of the search), and restricting the search to studies published in English and performed in the United States. For this report, we used the artificial intelligence (AI) feature of DistillerSR (AI Classifier Manager) as a second reviewer at the title and abstract screening stage.
We asked our content experts to help answer Review Questions 1 and 2 by drawing on domain knowledge and citing selected references that best answer the questions without conducting a systematic search for all evidence on the targeted harms and related patient safety measures or indicators. We focused on the harms and patient safety measures or indicators addressed in the studies included in answering Review Question 5.
For Review Question 2, we focused on identifying relevant measures that are included in the CMS patient safety measures, AHRQ’s Patient Safety Indicators, or the National Committee for Quality Assurance (NCQA) patient safety related measures. We asked content experts to help answer Review Questions 3 and 4 by citing selected references, including patient safety practices (PSPs) used and explanations of the rationale presented in the studies we found for Review Question 5. For Review Questions 6 and 7, we focused on the barriers, facilitators, and required resources reported in the studies we found for Review Question 5. For Review Question 8, we identified publicly available patient safety toolkits developed by AHRQ or other organizations that could help to support implementation of the PSPs. To accomplish that task, we reviewed AHRQ’s Patient Safety Network (PSNet) and AHRQ’s listing of patient safety related toolkits and we included any toolkits mentioned in the studies we found for Review Question 5.12,13 Any identified toolkits are not assessed or endorsed.
2.1. Eligibility Criteria for Studies of Effectiveness
We searched for original studies and systematic reviews on Review Question 5 (the question addressing effectiveness studies) according to the inclusion and exclusion criteria presented in Table 1.
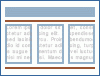
Table 1
Inclusion and exclusion criteria.
2.2. Literature Searches for Studies of Effectiveness
We searched PubMed and Cochrane, supplemented by a narrowly focused search for unpublished reports that are publicly available from governmental agencies or professional societies having a strong interest in the topic. For details of the search strategy, see Appendix A.
2.3. Data Extraction (Selecting and Coding)
We used the AI feature of DistillerSR (AI Classifier Manager) as a semi-automated screening tool to conduct this review efficiently at the title and abstract screening stage. A team member screened the title and abstract of each citation based on predefined eligibility criteria (Table 1). The screening responses by team members were used to teach the AI Classifier Manager to serve as a second reviewer of each citation. The AI Classifier Manager generated a ranking score for each citation, based upon a training set of titles and abstracts screened first by team members. The threshold for the AI Classifier Manager to include citations was set at a ranking score of 0.5 or above (scale 0 to 1.0). Discrepancies between team members and the AI Classifier Manager were reviewed and resolved by the team members. The full text of each remaining potentially eligible article was reviewed by a single team investigator to confirm eligibility. Full text articles underwent an additional independent review by a single investigator to determine whether they should be included in the full data abstraction.
We prioritized our efforts by extracting detailed information from the highest quality studies. Given the large number of systematic reviews and studies with strong designs, we focused on extracting detailed information from systematic reviews, randomized controlled trials (RCTs), observational studies with a contemporaneous comparison group, and studies employing time series analyses. We listed otherwise relevant studies having simple pre-post designs in Appendix C, but we did not synthesize them in the text of the results section. If identified primary studies were included in eligible systematic reviews, we made a note of that but did not exclude them from inclusion in the report.
Reviewers extracted available information and organized it according to the review questions and included author, year, study design, frequency and severity of the harms, measures of harm, characteristics of the PSP, rationale for the PSP, outcomes, implementation barriers and facilitators, resources needed for implementation, and description of toolkits. One reviewer completed the data abstraction, and a second reviewer checked all of the first reviewer’s abstraction for completeness and accuracy.
2.4. Risk of Bias (Quality) Assessment
For studies that addressed Review Question 5 about the effectiveness of PSPs, we used the Cochrane Collaboration’s tool for assessing the risk of bias of RCTs or the ROBINS-I tool for assessing the Risk Of Bias In Nonrandomized Studies – of Interventions.14,15 We did not assess the risk of bias in the pre-post studies, recognizing that they have a high risk of bias because of the lack of a contemporaneous comparison group.
For RCTs, we used the items in the Cochrane Collaboration’s tool that cover the domains of selection bias, performance bias, detection bias, attrition bias, reporting bias, and other bias.14 For nonrandomized studies, we used specific items in the ROBINS-I tool that assess bias due to confounding, bias in selection of participants into the study, bias in classification of interventions, bias due to deviations from intended interventions, bias due to missing data, bias in measurement of outcomes, and bias in selection of the reported results. The risk of bias assessment focused on the main outcome of interest in each study.15
For recent eligible systematic reviews, the primary reviewer used the criteria developed by the United States Preventive Services Task Force Methods Workgroup for assessing the quality of systematic reviews.16
- Good - Recent relevant review with comprehensive sources and search strategies; explicit and relevant selection criteria; standard appraisal of included studies; and valid conclusions.
- Fair - Recent relevant review that is not clearly biased but lacks comprehensive sources and search strategies.
- Poor - Outdated, irrelevant, or biased review without systematic search for studies, explicit selection criteria, or standard appraisal of studies.
2.5. Strategy for Data Synthesis
We narratively summarized findings across systematic reviews and across primary studies. We did not conduct a meta-analysis. For Review Question 5 about the effectiveness of PSPs, we recorded information about the context of each primary study and whether the effectiveness of the PSP differed across patient subgroups. We graded the strength of evidence for PSPs with more than one recent primary study of effectiveness using the methods outlined in the AHRQ Effective Health Care Program Methods Guide for Effectiveness and Comparative Effectiveness Reviews.17 We also noted what the included systematic reviews reported about the strength of evidence.
3. Evidence Summary
3.1. Benefits and Harms
- For neonates, systematic reviews and primary studies, reported that sepsis prediction and recognition PSPs improved clinical process measures (low strength of evidence), but there was insufficient evidence for length of stay or mortality outcomes.
- For adults, systematic reviews and primary studies reported that sepsis prediction and recognition PSPs did not demonstrate an effect on clinical process, length of stay, or mortality outcomes. In primary studies of this population, the strength of evidence was insufficient in the pre-hospital setting for mortality, length of stay, and clinical process measures. In the emergency department setting, strength of evidence was low for mortality and clinical process measures and insufficient for length of stay. In ward or hospitalwide settings, strength of evidence was low across all three outcome types.
- The secondary outcome of the performance of the prediction and recognition system (e.g., positive predictive value) could not be meaningfully compared across studies due to diversity in populations.
- Implementation barriers included poor alert system performance, clinician attitudes about clinical interventions and machine learning or artificial intelligence, as well as implementation challenges (e.g., competing sepsis prevention process improvement efforts) and resources required to implement and maintain the system. Facilitators included positive implementation process practices (e.g., using frequent communication, improvement cycles, and clinician engagement throughout the process) and specific sepsis PSP design features (e.g., approaches to training the predictive model, or methods of risk visualization).
- The quality of the sepsis prediction and recognition PSP literature is limited by heterogeneity and inadequate description of intervention details as well as low quality studies employing weak designs.
3.2. Future Research Needs
- Future research efforts should focus on higher quality studies (i.e., larger sample sizes, more rigorous designs) of re-designed sepsis prediction and recognition PSPs.
4. Evidence Base
4.1. Number of Studies
We found 15 studies (7 systematic reviews and 8 primary studies) that met our eligibility criteria (Figure 1). A listing of studies excluded during full-text review is included in Appendix B. Information abstracted from each included study is provided in Appendix C, Evidence Tables.

Figure 1
Results of the search and screening.
4.2. Findings for Review Questions
4.2.1. Question 1. What Are the Frequency and Severity of Harm Associated With Sepsis?
According to the Centers for Disease Control and Prevention (CDC), at least 1.7 million adults in America develop sepsis every year, and one in three people who die in a hospital had sepsis during their hospital stay.4 Between 4 and 12 percent of hospital admissions have a sepsis diagnosis.18–20 Mortality rates for sepsis range from 27% for unspecified sepsis diagnoses to 60% for septic shock diagnoses.3
Timely administration of antibiotics is a critical strategy for managing sepsis, but antibiotic therapy carries risks as well (e.g., medication allergy, organ dysfunction, and infections).21 A recent simulation study estimated that decreasing time to antibiotic treatment did not significantly increase new antibiotic related adverse events.22
4.2.2. Question 2. In PSPs Designed To Improve the Prediction or Recognition of Sepsis, What Patient Safety Measures or Indicators Have Been Used To Examine the Harms Associated With Sepsis?
In 2018, the Centers for Medicare & Medicaid Services (CMS) launched the Severe Sepsis and Septic Shock Early Management Bundle (SEP-1) performance measure. This measure has five elements:
- within three hours of severe sepsis presentation, lactate level was drawn, appropriate antibiotics administered, and blood cultures were drawn;
- within 6 hours of severe sepsis presentation if initial lactate level was elevated, lactate level was redrawn;
- within 3 hours of initial hypotension, appropriate intravenous fluids were given;
- within 6 hours of septic shock presentation if low blood pressure persisted, vasopressors were administered; and
- within 6 hours of septic shock presentation if low blood pressure persisted and lactate was greater than or equal to 4 mmol/l, volume status and tissue perfusion reassessment were performed.
As shown in Table 3, measures used in studies included in this review include the composite SEP-1 measure, its component measures, mortality, and hospital or intensive care unit (ICU) length of stay. In the neonatal population, a different set of clinical measures have been reported in evaluations of sepsis prediction and recognition PSPs. These include outcomes such as reduced overall antibiotic use across all patients (i.e., assessing the positive impact the sepsis prediction and recognition tool can have to reduce unnecessary antibiotics) and reduced lab orders across all patients. These are stewardship outcomes used to demonstrate the reduction in unnecessary care (and associated risks) for patients who are unlikely to develop sepsis.
4.2.3. Question 3. What PSPs Have Been Used To Improve Sepsis Prediction or Recognition and in What Settings Have They Been Used?
Three categories of sepsis prediction and recognition PSPs were included in Making Healthcare Safer (MHS) III: manual screening tools (i.e., electronic or physical checklists to assess a patient’s risk of having or developing sepsis), automated alerting systems (i.e., PSPs driven by patient monitoring systems using physiological monitoring or EHR data), and multicomponent sepsis interventions (i.e., PSPs bundling a prediction and recognition tool with additional interventions such as training, or organizational structures like response teams).11 Sepsis prediction and recognition PSPs included in this report were multicomponent interventions. Individual PSPs included either a manual screening or automated alerting system as well as varying automated communication alerts to different care team members (e.g., physicians, nurses, pharmacists), associated order sets or treatment recommendations, and staff training.
4.2.4. Question 4. What Is the Rationale for Psps Used To Improve Sepsis Prediction or Recognition?
Early detection and treatment of sepsis improves outcomes,5,23,24 so efforts to address sepsis focus heavily on rapid detection and intervention. However, the diagnosis of sepsis is challenging because common sepsis symptoms are nonspecific (e.g., fever, nausea, vomiting, muscle pain), particularly early in the clinical course, and sepsis can have highly variable presentations.
4.2.5. Question 5. What Are the Effectiveness and Unintended Effects of PSPs Designed To Improve the Prediction or Recognition of Sepsis, and What New Evidence Has Been Published Since the Search Was Done for the MHS III Report in 2019?
We identified seven recent SRs and eight recent primary studies. Two of the primary studies were evaluated in the included SRs but we included these primary studies separately as well, given heterogeneity in assessment methods across SRs.25–28 The eight primary studies evaluated PSPs to improve sepsis outcomes.26,28–34 Two studies were randomized controlled trials (RCTs)30,34 and the rest were observational studies with comparison groups.26,28,29,31–33 The study periods varied in length from five months34 to more than two years.26,29 One study had data collection overlapping with the Coronavirus Disease 2019 (COVID-19) pandemic;29 another two studies terminated early in order to expand the intervention to all patients.30,34 Characteristics of the included systematic reviews and primary studies are presented in Tables 2 and 3.
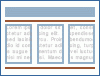
Table 2
Characteristics of the included systematic reviews by population and setting.
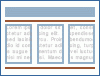
Table 3
Characteristics of the included primary studies by population and setting.
Several types of potential biases were present in the included RCTs and observational studies (Figure 2 and 3). Both RCTs were assessed as having an unclear risk of bias. In one RCT, the randomization technique was unclear;30 the second RCT had unclear blinding of outcome assessors and participants.34 For the observational studies, our assessments revealed concerns for bias in confounding, patient selection, and missing data. Two of the six observational studies had critical risks of bias.29,32

Figure 2
Risk of bias assessments for randomized controlled trials included in this review.

Figure 3
Risk of bias assessments for nonrandomized studies included in this review.
We present the findings by population. We first discuss the evidence from SRs, followed by evidence from primary studies.
4.2.5.1. Neonates
Three SRs35–37 evaluated sepsis PSPs in the neonatal population with two of the SRs35,36 focusing on the use of neonatal early onset sepsis (EOS) calculators versus standard of care. In neonates, sepsis is defined as early- or late-onset based on time of presentation after birth, with cutoffs for EOS that are inconsistent and range between 48 hours and 7 days.41,42 The other SR37 evaluated various clinical decision support algorithms that included non-invasive vital sign measurements versus standard of care. The three SRs included 55 studies cumulatively, two of which were RCTs.35–37
One primary study was a retrospective cohort study in two neonatal ICUs; it enrolled infants who had been admitted within 3 days after birth.29 Researchers compared sepsis-related outcomes in two periods: a period from 2012 to 2014 where C-reactive protein (CRP) was routinely measured in neonates (4,977 infants) and a period between 2018 and 2020, when it was not routinely measured (5,135 infants). A neonatal EOS calculator was also available in the second period.
4.2.5.1.1. Hospital Mortality
Three of the SRs27,36,37 reported impacts on mortality. In the SR by Deshmukh et al., one of six included studies evaluated an EOS calculator, which is a risk-based predictive model using a Bayesian approach developed by Kaiser Permanente North California, and found no change in hospital mortality, although there was only one death reported in both the EOS calculator and control groups.36 Since the only study that reported mortality was not an RCT, Deshmukh et al. concluded that there was low quality evidence for improvement in mortality.36
The SR by Persad et al. found two studies that evaluated the use of clinical decision support algorithms that included non-invasive vital sign measurements.37 One of the included studies showed a decrease in 30-day septicemia-related mortality from 19.6% to 11.8% (p=0.01) and the other showed a decrease from 16.1% to 10%.37 Both studies included the same sample of patients from an RCT and used the same PSP, the Heart Rate Observation (HeRO) monitoring algorithm, which reports a sepsis risk score based on analysis of heart rate variability and heart rate decelerations.37 Given the number of participants and the number of events, Persad et al. concluded that there was a high certainty of evidence that the sepsis PSP reduced 30-day septicemia mortality.37
The SR by van der Vegt et al, included 9 studies that evaluated the impact of predictive machine learning algorithms on mortality, one of which is the study by Schootman et al.27,28 All of the studies showed decreased mortality, however, this finding was only statistically significant in five. Notably, only one study adjusted for differences in characteristics between groups. The SR did not grade the strength of evidence (SOE).
The primary study of neonates compared sepsis-related outcomes in two periods: from 2012 to 2014 where CRP was routinely measured and between 2018 and 2020, when it was not routinely measured.29 Minimal differences in 7-day mortality (0.5% versus 0.6%) and in mortality before discharge (0.8% versus 0.9%, p=.60) were found between the two time periods.
4.2.5.1.2. Hospital or ICU Length of Stay
One primary study of neonates reported on the impact of sepsis PSPs on length of stay between 2 periods: from 2012 to 2014 where CRP was routinely measured and between 2018 and 2020 when it was not routinely measured.29 The hospital length of stay was not significantly different between the two periods.
4.2.5.1.3. Adherence to Clinical Guidelines or SEP-1 Measure
Two SRs evaluated the impact of an EOS calculator on antibiotic use. Deshmukh et al. found six studies that evaluated antibiotic use, all of which showed significantly less antibiotic use in the EOS calculator group compared to the standard of care group (1.4% versus 6%; odds ratio (OR) 0.22 [95% confidence interval (CI): 0.14 to 0.36]).36 Similarly, Achten et al. included thirteen studies, all of which found a lower relative risk of receiving antibiotic therapy in the EOS calculator group.35 Notably, there were larger reductions antibiotic use in studies limited to newborns born to mothers with chorioamnionitis (a group of infants who are at higher risk for EOS) compared to studies not limited to chorioamnionitis.35 Both reviews concluded that there was moderate quality evidence indicating EOS calculators reduce antibiotic use.35,36
Deshmukh et al. found five studies that evaluated the impact of an EOS calculator on lab testing, all of which showed significant reductions in lab testing in the calculator group compared to standard of care (2.5% versus 15.5%; OR, 0.14; 95% CI, 0.08 to 0.27]).36 They also found a significant reduction in admissions to the neonatal ICU (5.4% versus 19%; OR, 0.24; 95% CI, 0.11 to 0.51) and no difference in readmissions to the neonatal ICU (OR, 0.87; 95% CI, 0.57 to 1.33]) based on moderate quality evidence.36
The primary study of neonates that compared sepsis-related outcomes in two periods (routine versus nonroutine CRP measurement) found that fewer infants met SEP-1 measures in the group that did not use routine CRP measurement: there were fewer antibiotics used within the first 3 days of life (65% versus 57%, p < 0.001), fewer blood cultures (75% versus 55%, p < 0.001), and fewer cerebrospinal fluid cultures (8.7% versus 1.2%, p < 0.001).29 Yet, there were minimal differences in 7-day mortality and in-hospital mortality across the two time periods.
4.2.5.2. Adults
Four SRs25,27,38,39 evaluated sepsis PSPs in the adult population. All studies included in the SRs occurred in the hospital setting, but included various sites of implementation (i.e., emergency department [ED], ICU, or acute care floor).25,27, 38,39 Two of the reviews focused on machine learning or artificial intelligence-based sepsis prediction algorithms,27,39 while the other two evaluated automated sepsis alert systems.25,38 Only one of ten studies in Kausch et al. SR,39 one of thirty studies in the van der Vergt et al. SR,27 and all three studies in the Warttig et al. SR38 were RCTs.
We identified seven primary studies of adults, each evaluating use of an electronic health record (EHR)-based alert system directed at clinicians in combination with a treatment intervention, such as provision of order sets.26, 28, 30–34 The alert systems, which triggered treatment, varied across studies. Two studies used the Early Warning System (EWS) or a modified EWS where a score was displayed in the EHR and when the score crossed a threshold, an alert was sent to the clinician.31,34 Three studies used the criteria in the Systemic Inflammatory Response Syndrome (SIRS) definition, where the presence of two or more sepsis criteria elicited a prompt to place a sepsis-related order.26,30,32 One hospital developed their own predictive model with an alert when the sepsis risk exceeded a threshold;28 this was also the only study that included patients in ICUs, general medical wards, and EDs. Three of the studies restricted the evaluation to patients in the EDs (Figure 4).26,33,34
Of the primary studies, two were RCTs. Patients were randomized to a study arm that generated EHR alerts upon crossing a threshold, or to a study arm that did not generate an alert.30,34 Five were observational studies. Three compared outcomes during the intervention period to outcomes drawn from historical data before alert systems were in place; the outcomes were evaluated for differences with time-series analyses.26, 31, 33 Another study compared outcomes in hospitals that implemented an alert system to hospitals that did not, and compared outcomes with a difference-in-difference model.28 One other observational study evaluated the impact of displaying the alert to pre-hospital clinicians (emergency medical services) compared to displaying the alert to clinicians in the ED.32

Figure 4
Overview of strength of evidence from recent primary studies by outcome and setting for studies of adult populations. ICU = intensive care unit; SEP-1 = Severe Sepsis and Septic Shock Management Bundle
4.2.5.2.1. Mortality
Three of the SRs reported the impact of sepsis PSPs on mortality. In the SR by Kausch et al., one RCT showed that a machine learning algorithm, the Insight model, reduced mortality in the ICU setting.39 However, one study evaluating a different machine learning model showed no difference in mortality in the ICU setting.39 The SR did not grade the SOE.
In the SR by Hwang et al., one low quality study on the use of a sepsis alert system showed a reduction in mortality (26.1% versus 36.3%; incidence rate ratio (IRR), 0.64; 95% CI, 0.43 to 0.97). However, another low quality study and two high quality studies, one of which was the study by Austrian et al., showed no difference in mortality with the use of a sepsis alert system.25, 26 The review graded the quality of evidence as low.
In the SR by Warrtig et al., one study found no significant difference in 14-day mortality between groups (20% versus 21%) and another study found no difference in 28-day mortality between groups (14% versus 10%).38 Due to inadequate reporting of sample sizes for these outcomes in both studies, the 95% CIs could not be estimated.38 The SR assessed the evidence for this outcome to be of very low quality.
The seven primary studies all reported on mortality; they demonstrated modest impact of the PSPs.26,28,30–34 Three reported on 30-day (or 28-day) mortality rates.30,33,34
We report the findings from the primary studies, by setting, below.
4.2.5.2.1.1. Prehospital Setting
A single, small, observational study compared a field-based (pre-hospital) alert to an ED alert and found lower 60-day mortality among patients who were exposed to the ED alert system (16% versus 9.6%, p = 0.07).32 We were unable to draw a conclusion due to lack of evidence.(SOE: Insufficient)
4.2.5.2.1.2. Emergency Department Setting
Three studies evaluated a sepsis alert system used in the ED on mortality.26,33,34 Tarabichi, et al. did not find significantly reduced mortality with display of the alert (mortality rates of 9.9% in the control group versus 6% in the intervention group p = 0.077); this trial included approximately 300 participants per arm.34 Similarly, in-hospital mortality did not differ significantly (8% versus 4.6%; p = 0.086).
A cohort study evaluated adding a navigator bundle to an alert against both a historical control group without an alert and a contemporaneous control group without the navigator bundle. The study found similar rates of 30-day mortality in all three groups of 9.7%, 8.6%, 9.3%, respectively.33
Austrian, et al. conducted a time series study with historical controls and reported the adjusted IRR for death as 0.58 (95% CI, 0.29 to 1.19), which non-significantly favored the intervention period when alerts were in use.26 This study was also included in the SR by Hwang et al.25
Overall, evidence suggests a potentially small benefit, but no statistically significant results were reported in these studies (SOE: Low).
4.2.5.2.1.3. Ward or Hospitalwide Setting
Three studies that tested the intervention in inpatient units or throughout the hospital assessed mortality as an outcome.28,30,31 The trial conducted by Downing et al. did not show a mortality benefit associated with display of the alert in the EHR (OR, 0.9; 95% CI, 0.56 to 1.46).30
The times series analysis of the EWS system found reduced monthly mortality rates after implementation although the difference was not statistically significant at −3.14% per month (95% CI, −8.76 to 2.48).31
One observational study,23 which was also included in the SR by van der Vegt et al., reported on mortality rates across the observation periods.27,28 In the adjusted difference-in-difference analysis using control hospitals that did not have the alert intervention, Schootman and colleagues reported a small, and not significant, difference in mortality rates attributable to the intervention (reduction of 1%, p = 0.17).28
Overall, there was no difference in mortality outcomes in these studies. (SOE: Low)
4.2.5.2.2. Hospital or Intensive Care Unit Length of Stay
Three SRs reported the impact of sepsis prediction and recognition PSPs on length of stay. In the review by Kausch et al., one RCT showed that the use of a machine learning model, the Insight model, reduced overall length of stay.39 However, one study showed no difference in length of stay.39 The review did not grade the SOE.
In the SR by Hwang et al., one high quality study, which is the study by Austrian et al., showed both a decrease in ICU length of stay as well as overall hospital length of stay.25,28 Additionally, a low quality study showed improvement in overall hospital length of stay (OR, 0.66; 95% CI, 0.53 to 0.82).25 The review graded evidence as low quality.
The SR by Warrtig et al. included a study with 560 patients which showed no difference in median length of stay with the use of a sepsis alert system (3.0 days versus 3.0 days, p = 0.22).38 This review assessed the quality of the evidence for this outcome to be low.
There were seven primary studies that reported on hospital or ICU length of stay outcomes.26,28,30–34
We report the results from the primary studies, by settings, below.
4.2.5.2.2.1. Prehospital Setting
In the study comparing the field alert (pre-hospital) to the ED alert, there was no significant difference between groups on hospital length of stay.32 We were unable to draw a conclusion due to lack of evidence.(SOE: Insufficient)
4.2.5.2.2.2. Emergency Department Setting
Two studies evaluated the impact of an alert in the ED on hospital or ICU length of stay.26,34 The trial by Tarabichi, et al, found comparable median hospital and ICU lengths of stay in the intervention and comparison groups, with hospital lengths of stay of 3.2 [interquartile range (IQR) 1.1-6.2] and 4.0 [IQR:1.4 to 7.0]), respectively.34
The observational study by Austrian and colleagues, which was also included in the SR by Hwang et al., demonstrated significantly shortened lengths of stay associated with the alert-based intervention: length of ICU stay after implementation of the alert system in the ED was reduced from 1.8 days (standard deviation [SD] 3.1) to 1.2 days (SD 3.1) (p < 0.001) and total hospital length of stay was reduced from 10.1 days (SD 10.1) to 8.6 days (SD 7.9) (p < 0.001).25,26
The studies conflicted and we are unable to draw conclusions about the impact of sepsis alerts in the ED on hospital or ICU length of stay (SOE: Insufficient).
4.2.5.2.2.3. Ward or Hospitalwide Setting
Three studies conducted in the inpatient setting or hospitalwide assessed hospital or ICU length of stay.28,30,31 The Downing, et al, trial evaluated a binary outcome of length of stay greater or less than 72 hours and found no difference between intervention arms.30 The Horton, et al, interrupted times series analysis of the EWS alert reported a small decrease in median length of stay of 0.63 days (95% CI, −1.28 to 0.03, P=.059).31 The Schootman, et al, observational study, which was also included in the SR by van der Vegt et al., also did not demonstrate a significant impact of the intervention on length of stay.28
Overall, there was no difference in the length of stay (SOE: Low).
4.2.5.2.3. Adherence to Clinical Guidelines or SEP-1 Measure
Two of the SRs reported the impact of a PSP on adherence to aspects of sepsis guidelines or the SEP-1 measure.25,38 In one high quality study, included in the review by Hwang et al., there was no difference in antibiotics being given in the ED with the use of a sepsis alert system, but a different low quality study showed an improvement in the time to antibiotic therapy (29 minutes versus 61.5 minutes, p < 0.001).25 The review graded the evidence as low quality.
All three studies included in the SR by Warrtig et al. included data regarding time to initiation of antibiotic therapy, but due to insufficient information being included in the studies, the data could not be pooled and the impact on antibiotic timing could not be evaluated.38 The largest included study with 680 patients in the review by Warrtig, did show a decrease in median time to initiation of antibiotic therapy (5.6 hours versus 7.8 hours) although statistical significance could not be evaluated due to insufficient data.38 This review assessed the quality of evidence for this outcome to be very low.
Hwang et al, included one high-quality study, which was the study by Austrian et al., that showed improvement in time to first lactate level being measured.25,28 However, the same study showed no difference in lactate level being collected more than 24 hours after ED arrival (90.7% versus 91.3%, p = 0.65) and another high-quality study showed no difference in lactate being collected at any time (OR, 1.7; 95% CI, 0.9 to 3.2]).25 Another study showed increases in overall lactate testing (12.7% versus 5.2%, p < 0.001), but this was deemed to be a low-quality study.
The review by Hwang et al. also included two studies evaluating collection of blood cultures. In one high-quality study, which was the study by Austrian et al., there was no change in blood cultures being drawn prior to antibiotics, but there was an overall increase in blood cultures being collected in another high-quality study (OR, 2.9; 95% CI, 1.1 to 7.7]).25,28
The seven primary studies all reported on at least one SEP-1 measure. The most frequently reported outcome was antibiotic (or anti-infective) use.28,30,32–34
We report the findings from the primary studies by settings below.
4.2.5.2.3.1. Prehospital Setting
In the study comparing the prehospital alert to the ED alert, the pre-hospital alert group had higher rates of antibiotic use within the first hour (59% versus 44%, p = 0.01) and a shorter time to administration of 48 minutes (IQR, 34 to 87) compared to 64 minutes (IQR, 47 to 99).32 This study did not find a difference in fluid bolus use between groups (52% versus 43%, p = 0.5).32 We were unable to draw a conclusion due to lack of evidence (SOE: Insufficient).
4.2.5.2.3.2. Emergency Department
The three ED studies reported on a SEP-1 measure.26,33,34 The RCT, by Tarabachi et al, evaluated any antibiotic utilization and found no important differences in use between the study arms by 28 days (70% and 68%, p = 0.55).34 In this trial, the time to administration was shorter in the intervention group at 2.3 hours (95% CI, 1.4 to 4.7) compared to 3.0 hours (95% CI, 1.6 to 5.5) in the comparison group (p = 0.039).
In the observational study by Schertz, et al, the authors reported that the time to antibiotic delivery was shorter in the intervention group relative to the historical control group by 2.8 hours (−3.5 to −2.0 hours) in adjusted analyses; however, there was not a difference when comparing this intervention to a contemporaneous control using a SIRS alert system, with a difference of 0.01 hours (95% CI, −0.16 to 0.19 hours).33 Those authors also reported results in subgroups based on sepsis severity, with similar findings.
In the observational study by Austrian and colleagues, which was also included in the review by Hwang et al., early blood culture collection was assessed.25, 26 No difference was found in the culture-before-antibiotic rates when the intervention period was compared with the historical control period, with rates of 79% in both groups (IRR, 0.87; 95% CI, 0.56 to 1.4).26 That study also assessed the measurement of lactate levels and did not find a significant difference in completion of lactate measurement (IRR, 1.7; 95% CI, 0.86 to 3.25). However, the time to first lactate measurement was shorter after implementation of the alert system (0.19 days versus 0.16 days (IRR, 0.63; p < 0.001).
The RCT by Tarabichi and colleagues assessed the administration of intravenous fluids, and did not find differences in rates of use of fluid boluses, by 28 days, between the control and intervention groups with rates of 65% and 61%, respectively (p = 0.34).34
Overall, there was no difference in SEP-1 measures with the ED-based sepsis alerts (SOE: Low).
4.2.5.2.3.3. Ward or Hospitalwide Setting
Two studies in ward or hospitalwide setting reported on the SEP-1 measures.28,30,31 The RCT by Downing and colleagues did not find a significant difference in rates of use of antibiotics within 180 minutes in the usual care and active alert arms with rates around 36% in both arms (OR, 0.9; 95% CI, 0.72 to 1.2)30 Schootman, et al,28 evaluated antibiotic administration within 60 minutes of the alert, and this study was also included in the review by van der Vegt et al.27 In the adjusted difference-in-difference analysis, relative to control hospitals, the change from baseline rates were non-significantly greater. Horton and colleagues’ time series analysis of the EWS system found no difference in antibiotic use within 24 hours with implementation of the alert.31
The trial, by Downing et al, also assessed the timely administration of intravenous fluids, finding little difference between groups in fluid administration by 180 minutes (20% versus 24%, OR, 1.3; 95% CI, 0.94 to 1.7) or in large volume fluid administration within 180 minutes (1.5% in both arms, OR, 1.0; 95% CI, 0.37 to 2.7).30
In the Downing, et al, trial, there was not a difference in blood culture orders within 180 minutes across arms (5.1% versus 4.7%, OR= 0.9, p =1.0).30 Similarly, there was no significant difference in lactate measurement within 180 minutes (OR, 1.4; 95% CI, 0.95 to 1.95).30
Overall, there was no difference in SEP-1 measures with implementation of the alert system hospitalwide (SOE: Low).
4.2.5.2.4. Secondary Outcomes
The outcomes that we considered to be secondary were those pertaining to the performance of the alert systems for detecting sepsis in patients suspected of impending sepsis. It is not meaningful to compare the positive predictive values (PPV) across studies as the populations that were exposed to the alert systems varied greatly. For example, the PPV of the alert system in the Downing et al trial was 61%—it was applied broadly on hospital wards and triggered with three or more SIRS criteria.30 The PPV of the alert system in the Tarabichi et al trial was 27%—this study involved application of the EWS system to patients in the ED.34 The PPV associated with measurement of CRP in neonates was very low (less than 3%) regardless of the time of use (within 4 hours, 4-24 hours, or 24-72 hours (Table 4).29
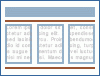
Table 4
Summary of sensitivity and specificity data reported in the primary studies by setting.
4.2.6. Question 6. What Are Common Barriers and Facilitators to Implementing PSPs Targeting the Prediction or Recognition of Sepsis?
Only one systematic review reported both barriers and facilitators to implementation.27 Five primary studies reported barriers to implementation of a sepsis PSP,26,28,30–32 but none reported facilitators to implementation (Table 5).
A commonly described barrier to implementation was suboptimal performance of the predictive model, with poor PPV in particular being a major issue.26,28,30 The poor PPV was found to lead to lower response rates to alerts among clinicians and likely contributed to alert fatigue, limiting the impact of the sepsis PSP on clinical outcomes.26,28,30 Similarly, the review by van der Vegt et al. found that alert fatigue and dismissal of alerts due to no clinical signs of deterioration were among the most common barriers to implementation across included studies.27 Austrian et al. noted that prior studies that demonstrated a significant change in process or clinical outcomes with the use of a sepsis PSP had much higher PPVs, which further supports this concern.26
Downing et al. also observed that in addition to false positives leading to non-response to alerts by clinicians, alerts may lag behind clinical judgment and clinicians often had already diagnosed sepsis and initiated appropriate treatment.30 In the review by Vegt et al. it was noted that such delays in alerting may lead to clinicians believing they are better at diagnosing sepsis than the alert system.27 The reasons for delayed alerts may vary, but Mixon et al. noted that the cumbersome interactions between clinicians and the EHR when manual input of data is required may be an important contributing factor.32
Furthermore, Downing et al. found that certain clinician beliefs and attitudes may limit the impact of a PSP. In their study, there were issues with fluid resuscitation of septic patients due to clinicians’ prior beliefs regarding fluid resuscitation in patients with a diagnosis of heart failure. This highlighted the importance of change management and local culture shifts in addition to the implementation of a sepsis PSP to change behavior and improve outcomes.30
Similarly, beliefs and attitudes regarding machine learning or artificial intelligence may be barriers to successful implementation of sepsis PSPs that use such algorithms. A common barrier noted in the review by van der Vegt was lack of clinician trust in machine learning-based sepsis alert systems, possibly related to lack of machine learning foundational knowledge and experience, as well as performance issues.27
Limitations of the EHR were also found to be a barrier for sepsis alert systems that used EHR data. The review by van der Vegt noted that this may be due to inherent limitations of EHR data, such as missingness, inaccuracies, and changes in practice patterns over time.27 Additionally, there may be data entry delays that lead to delayed predictions.
Another barrier to implementation that was noted by Horton et al. was the concurrent implementation of quality improvement initiatives during the time of the sepsis PSP implementation. This may have affected allocation of resources needed for successful implementation, and limited evaluation of the impact of the sepsis PSP on outcomes.31 The review by van der Veght also noted that the substantial costs for infrastructure, implementation personnel time, and ongoing maintenance were barriers to implementation for machine learning based alert systems.27
The review by van Vegt et al. also identified implementation facilitators. A total of 26 facilitators were noted across studies in the review, although most were only noted in one or two studies. The most commonly reported facilitators were frequent communications to raise awareness of the sepsis PSP during and after clinical trials, conducting improvement cycles, clinician involvement at all stages of development and implementation, identification of clinical champions, and use of test versions of the PSP for training.27 Other less commonly reported facilitators ranged from factors specific to development and design of the sepsis PSP, such as improved training methods and color-coded visual depiction of sepsis risk, to factors related to the implementation strategy, such as staggering deployment across sites and providing clinical end users with “fact sheets” about the PSP.27
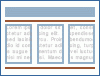
Table 5
Patient safety practice implementation facilitators and barriers.
4.2.7. Question 7. What Resources (e.g., cost, staff, time) Are Required for Implementation?
None of the included primary studies or SRs reported specific information regarding resources that are required for implementation of sepsis PSPs. However, the review by van der Vegt et al. did note that that there may be substantial costs involved for infrastructure, implementation personnel time, and ongoing maintenance.27 Similarly, Horton et al. described how competing resource allocation due to concurrent quality improvement initiatives may impact the effectiveness of a sepsis PSP.31
4.2.8. Question 8. What Toolkits Are Available To Support Implementation of the PSPs?
No primary studies described toolkits used or produced. However, the following toolkits related to sepsis are openly available.
- The Surviving Sepsis Campaign, led by the Society for Critical Care Medicine, is an ongoing effort to maintain international guidelines for sepsis recognition and treatment, and to disseminate tools to support adoption including protocols, apps, checklists, and an implementation guide.43
- The CDC’s Hospital Toolkit for Adult Sepsis Surveillance provides resources for healthcare facility-level monitoring of the incidence and outcomes of sepsis, and the related Hospital Sepsis Program Core Elements guide outlines key features of successful monitoring programs. These CDC toolkits do not focus on sepsis prediction or recognition for individual patient care, but for sepsis monitoring across an organization.44,45
5. Discussion
5.1. Summary and Interpretation of Findings
The Making Healthcare Safer (MHS) III report in 2020 concluded that there was moderate evidence of improvement in process measures in the hospital setting for both sepsis screening and patient monitoring system patient safety practices (PSPs), while there was only sparse evidence regarding process measure improvement in the prehospital setting and mixed findings for clinical outcome measures. All three included studies in the hospital setting for manual screening PSPs showed significant improvement in at least one process measure. PSPs focused on patient monitoring systems for sepsis were also found to improve process measures significantly across five of the six included studies, although only two of five studies found a significant effect on outcome measures.
In the current review, all PSPs in the included studies were multicomponent interventions. Studies occurred in either pediatric or adult populations and in pre-hospital, emergency department (ED), intensive care unit (ICU) and hospital ward settings.
In the neonatal population, we found some evidence of benefit for sepsis prediction and recognition PSPs on clinical outcomes, including reduced lab tests, empirical antibiotics, and ICU admissions for suspected (Early Onset Sepsis) EOS cases. These are not the types of clinical care measures typically considered in evaluating sepsis recognition and prediction PSPs (e.g., Severe Sepsis and Septic Shock Early Management Bundle (SEP-1) components) but do represent improvements in quality of care or patient outcomes. The strength of evidence was insufficient for mortality and length of stay (LOS) due to inconsistency in study findings.
In the adult population, systematic reviews and primary studies reported that sepsis prediction and recognition PSPs did not demonstrate an effect on clinical process, LOS, or mortality outcomes. In primary studies of this population, strength of evidence was insufficient in the pre-hospital setting across the three outcome categories due to there being only one included study. Strength of evidence was low in ED and ward or hospitalwide settings for mortality and clinical process measures. For LOS, strength of evidence was low in ward or hospital settings and insufficient in the ED setting. Reasons for low and insufficient ratings included imprecision, inconsistency of findings, and moderate study limitations. However, the three studies in the ED setting reported a small decrease in mortality with use of sepsis PSPs, though none of the differences were significant.
Other outcomes that were assessed in the systematic reviews but not included in the above results were rates of septic shock and ICU transfer. One study in the review by Kausch et al., showed a decrease in the rates of septic shock after implementation of a machine learning algorithm to predict the onset of sepsis.39 Another study in the review by Hwang et al., which was deemed to be of high-quality, showed a decrease in ICU transfers with the use of a sepsis alert system.25
The secondary outcome of the performance of alerting system (e.g., positive predictive value) could not meaningfully be compared across studies due to diversity in populations and interventions. However, as shown in Table 4, the performance of these predictive systems varies widely. This has been demonstrated more comprehensively in reviews on manual scoring46 and artificial intelligence systems47,48 for predicting sepsis and is a common barrier to implementation.
Implementation barriers included poor performance of alerting systems, which included false positives as well as the timing of alerts lagging clinical judgement and flagging patients who already had a diagnosis of sepsis. Pre-existing attitudes and beliefs of clinicians about both clinical practices (e.g., fluid resuscitation for patients with heart failure) and machine learning or artificial intelligence were noted to impact use of sepsis PSPs. Poor electronic health record (EHR) data quality was cited as a contributing factor to poor system performance and implementation success. Finally, quality improvement interventions concurrent with sepsis PSP implementation may affect resource allocation and program evaluation. One review identified 26 implementation facilitators.27 Many of them were common best practices for implementation (e.g., frequent communication, improvement cycles, clinician engagement throughout the process) and others were more specific to sepsis PSPs (e.g., model training methods, risk visualization). No articles included in this review reported specific information about resources required; however, one review noted that technical infrastructure and personnel costs involved in implementation and maintenance of these systems were substantial and potential barriers to implementation.27
These many challenges described as implementation barriers provide possible reasons why sepsis prediction and recognition PSPs are not more effective. Additionally, two aspects of the rationale for the effectiveness of the specific PSPs evaluated in the included studies have been challenged. First, the degree to which sepsis-associated mortality is preventable has been questioned in a recent retrospective cohort study which found that out of 300 sepsis-associated deaths, only 11 (3.7%) were definitely or moderately likely to have been preventable, and 25 (8.3%) were possibly preventable.49 In this study, chronic comorbidities were the most common underlying cause of death. However, assessing preventability of harm is highly subjective. Second, a large retrospective study demonstrated that delays in time to antibiotics were significant for patients with septic shock (with each hour adding additional risk for mortality), but not for patients with sepsis without shock.50 For the latter group, only delays greater than six hours were associated with higher mortality. Timeliness of care matters, but the impact depends on disease acuity. Finally, for sepsis prediction and recognition PSPs employing machine learning models or any statistical prediction model, the general phenomenon of model quality degradation over time may be a concern.51 Simulation studies have demonstrated several forms of temporal phenomenon (i.e., changes in the distribution of predictors, changes in the relationship between predictors and targets, and the impact of major events) commonly referred to as model or data drift do impact the quality of sepsis prediction models.52
5.2. Limitations
We discuss both limitations of this rapid review and of the sepsis prediction and recognition PSP literature. Sepsis prevention has been an ongoing effort for over twenty years, with the international Surviving Sepsis Campaign having been launched in 2002.53–55 Additionally, more recent implementation of mandatory SEP-1 reporting in 2018 has further increased awareness of the clinical interventions and organizational efforts to improve sepsis management.56 These concurrent trends may complicate detecting effects, and create confounders for observational and pre-post study designs, which are common in this literature.
A major limitation of the literature is heterogeneity of the PSPs. In MHS III, PSPs were reported in three broad categories. The literature reviewed here were all multi-component interventions, indicating that perhaps the field has matured in how sepsis prediction and recognition PSPs are developed and implemented. However, this creates challenges synthesizing evidence, which is further complicated by lack of detail in intervention descriptions in many studies. It is notable that the strongest evidence of benefit was found in systematic reviews of a single PSP (the EOS Calculator).
A second major limitation of the literature is study quality. Few RCTs were found, and studies often used pre-post study designs and small sample sizes.
Our review is also limited in that we restricted eligible publications to recent primary studies performed in the United States or reviews that included primary studies in the United States. Sepsis prevention, generally, and sepsis prediction and recognition PSPs are of interest globally and potential advances from outside the United States were not included in this review.
5.3. Implications for Clinical Practice and Future Research
Future research efforts should focus on higher quality studies of redesigned sepsis prediction and recognition PSPs, and address issues in disparities of care. The overall quality of research was low and dominated by observational studies. Many of the studies included here were probably underpowered; they were small and identified non-significant differences often favoring the sepsis prediction and recognition PSPs. Higher quality trials would increase the strength of evidence; however, given the findings of this review, the field may require redesign of PSPs before larger trials are conducted. It is increasingly clear that these PSPs are not generating the benefits identified in earlier MHS reports but it is unclear why. Many barriers have been identified in the literature, including those attributable to the interventions themselves (poor predictive performance), how they are implemented within the larger health IT ecosystem (poor data quality) and workflow (timing of alerts relative to clinician judgement). Future research should address the sizable disparities in sepsis-related mortality, with Black, Hispanic and Native American patients being at elevated risk compared to Whites.53
6. References
- 1.
- Rosen M, Dy SM, Stewart CM, et al. Final Report on Prioritization of Patient Safety Practices for a New Rapid Review or Rapid Response. Making Healthcare Safer IV. (Prepared by the Johns Hopkins, ECRI, and Southern California Evidence-based Practice Centers under Contract No. 75Q80120D00003). AHRQ Publication No. 23-EHC019-1 Agency for Healthcare Research and Quality. Rockville, MD: 2023. https:
​//effectivehealthcare ​.ahrq.gov/products ​/prioritization-patient-safety-practices - 2.
- Global report on the epidemiology and burden of sepsis: current evidence, identifying gaps and future directions World Health Organization. Geneva: 2020.
- 3.
- Buchman TG, Simpson SQ, Sciarretta KL, et al. Sepsis Among Medicare Beneficiaries: 1. The Burdens of Sepsis, 2012-2018. Crit Care Med. 2020 Mar;48(3):276–88. doi: 10.1097/ccm.0000000000004224. PMID: 32058366. [PMC free article: PMC7017943] [PubMed: 32058366] [CrossRef]
- 4.
- What is Sepsis? Centers for Disease Control and Prevention; 2023. https://www
​.cdc.gov/sepsis ​/what-is-sepsis.html. Accessed on November 14, 2023. - 5.
- Seymour CW, Gesten F, Prescott HC, et al. Time to Treatment and Mortality during Mandated Emergency Care for Sepsis. N Engl J Med. 2017 Jun 8;376(23):2235–44. doi: 10.1056/NEJMoa1703058. PMID: 28528569. [PMC free article: PMC5538258] [PubMed: 28528569] [CrossRef]
- 6.
- Kumar A, Roberts D, Wood KE, et al. Duration of hypotension before initiation of effective antimicrobial therapy is the critical determinant of survival in human septic shock. Crit Care Med. 2006 Jun;34(6):1589–96. doi: 10.1097/01.ccm.0000217961.75225.e9. PMID: 16625125. [PubMed: 16625125] [CrossRef]
- 7.
- Evans L, Rhodes A, Alhazzani W, et al. Surviving sepsis campaign: international guidelines for management of sepsis and septic shock 2021. Intensive Care Med. 2021 Nov;47(11):1181–247. doi: 10.1007/s00134-021-06506-y. PMID: 34599691. [PMC free article: PMC8486643] [PubMed: 34599691] [CrossRef]
- 8.
- Rhee C, Yu T, Wang R, et al. Association Between Implementation of the Severe Sepsis and Septic Shock Early Management Bundle Performance Measure and Outcomes in Patients With Suspected Sepsis in US Hospitals. JAMA Netw Open. 2021 Dec 1;4(12):e2138596. doi: 10.1001/jamanetworkopen.2021.38596. PMID: 34928358. [PMC free article: PMC8689388] [PubMed: 34928358] [CrossRef]
- 9.
- Townsend SR, Phillips GS, Duseja R, et al. Effects of Compliance With the Early Management Bundle (SEP-1) on Mortality Changes Among Medicare Beneficiaries With Sepsis: A Propensity Score Matched Cohort Study. Chest. 2022 Feb;161(2):392–406. doi: 10.1016/j.chest.2021.07.2167. PMID: 34364867. [PubMed: 34364867] [CrossRef]
- 10.
- Gesten F, Evans L. SEP-1-Taking the Measure of a Measure. JAMA Netw Open. 2021 Dec 1;4(12):e2138823. doi: 10.1001/jamanetworkopen.2021.38823. PMID: 34928364. [PubMed: 34928364] [CrossRef]
- 11.
- Hall KK, Shoemaker-Hunt S, Hoffman L, et al. Making Healthcare Safer III: A Critical Analysis of Existing and Emerging Patient Safety Practices. Rockville (MD): Agency for Healthcare Research and Quality (US); 2020. [PubMed: 32255576]
- 12.
- Promoting Patient Safety. Rockville, MD: Agency for Healthcare Research and Quality. https:/psnet
​.ahrq.gov. Accessed on November 14, 2023. - 13.
- Tools. Rockville, MD: Agency for Healthcare Research and Quality. https://www
​.ahrq.gov/tools/index ​.html?search ​_api_views_fulltext ​=&field_toolkit_topics ​=14170&sort_by ​=title&sort ​_order=ASC. Accessed on November 14, 2023. - 14.
- Higgins JP, Altman DG, Gøtzsche PC, et al. The Cochrane Collaboration’s tool for assessing risk of bias in randomised trials. Bmj. 2011 Oct 18;343:d5928. doi: 10.1136/bmj.d5928. PMID: 22008217. [PMC free article: PMC3196245] [PubMed: 22008217] [CrossRef]
- 15.
- Sterne JA, Hernán MA, Reeves BC, et al. ROBINS-I: a tool for assessing risk of bias in non-randomised studies of interventions. Bmj. 2016 Oct 12;355:i4919. doi: 10.1136/bmj.i4919 10.1136/bmj.i4919. PMID: 27733354. [PMC free article: PMC5062054] [PubMed: 27733354] [CrossRef]
- 16.
- Procedure Manual Appendix VI. Criteria for Assessing Internal Validity of Individual Studies. Rockville, MD: U.S. Preventive Services Task Force; 2017. https://www
​.uspreventiveservicestaskforce ​.org/uspstf/about-uspstf ​/methods-and-processes ​/procedure-manual ​/procedure-manual-appendix-vi-criteria-assessing-internal-validity-individual-studies. Accessed on June 29, 2023. - 17.
- Methods Guide for Effectiveness and Comparative Effectiveness Reviews. Rockville, MD: Agency for Healthcare Research and Quality; 2022. https:
​//effectivehealthcare ​.ahrq.gov/products ​/collections/cer-methods-guide. Accessed on July 31, 2023. [PubMed: 21433403] - 18.
- Chan HK, Khose S, Chavez S, et al. Updated estimates of sepsis hospitalizations at United States academic medical centers. J Am Coll Emerg Physicians Open. 2022 Aug;3(4):e12782. doi: 10.1002/emp2.12782. PMID: 35859855. [PMC free article: PMC9288236] [PubMed: 35859855] [CrossRef]
- 19.
- Dantes RB, Kaur H, Bouwkamp BA, et al. Sepsis Program Activities in Acute Care Hospitals - National Healthcare Safety Network, United States, 2022. MMWR Morb Mortal Wkly Rep. 2023 Aug 25;72(34):907–11. doi: 10.15585/mmwr.mm7234a2. PMID: 37616184. [PMC free article: PMC10468219] [PubMed: 37616184] [CrossRef]
- 20.
- Rhee C, Wang R, Zhang Z, et al. Epidemiology of Hospital-Onset Versus Community-Onset Sepsis in U.S. Hospitals and Association With Mortality: A Retrospective Analysis Using Electronic Clinical Data. Crit Care Med. 2019 Sep;47(9):1169–76. doi: 10.1097/ccm.0000000000003817. PMID: 31135503. [PMC free article: PMC6697188] [PubMed: 31135503] [CrossRef]
- 21.
- Tamma PD, Avdic E, Li DX, et al. Association of Adverse Events With Antibiotic Use in Hospitalized Patients. JAMA Intern Med. 2017 Sep 1;177(9):1308–15. doi: 10.1001/jamainternmed.2017.1938. PMID: 28604925. [PMC free article: PMC5710569] [PubMed: 28604925] [CrossRef]
- 22.
- Donnelly JP, Seelye SM, Kipnis P, et al. Impact of Reducing Time-to-Antibiotics on Sepsis Mortality, Antibiotic Use, and Adverse Events. Ann Am Thorac Soc. 2023 Nov 7doi: 10.1513/AnnalsATS.202306-505OC. PMID: 37934602. [PMC free article: PMC10867916] [PubMed: 37934602] [CrossRef]
- 23.
- Liu VX, Fielding-Singh V, Greene JD, et al. The Timing of Early Antibiotics and Hospital Mortality in Sepsis. Am J Respir Crit Care Med. 2017 Oct 1;196(7):856–63. doi: 10.1164/rccm.201609-1848OC. PMID: 28345952. [PMC free article: PMC5649973] [PubMed: 28345952] [CrossRef]
- 24.
- Peltan ID, Mitchell KH, Rudd KE, et al. Prehospital Care and Emergency Department Door-to-Antibiotic Time in Sepsis. Ann Am Thorac Soc. 2018 Dec;15(12):1443–50. doi: 10.1513/AnnalsATS.201803-199OC. PMID: 30153044. [PMC free article: PMC6322022] [PubMed: 30153044] [CrossRef]
- 25.
- Hwang MI, Bond WF, Powell ES. Sepsis Alerts in Emergency Departments: A Systematic Review of Accuracy and Quality Measure Impact. West J Emerg Med. 2020 Aug 24;21(5):1201–10. doi: 10.5811/westjem.2020.5.46010. PMID: 32970576. [PMC free article: PMC7514413] [PubMed: 32970576] [CrossRef]
- 26.
- Austrian JS, Jamin CT, Doty GR, et al. Impact of an emergency department electronic sepsis surveillance system on patient mortality and length of stay. J Am Med Inform Assoc. 2018 May 1;25(5):523–9. doi: 10.1093/jamia/ocx072. PMID: 29025165. [PMC free article: PMC7646853] [PubMed: 29025165] [CrossRef]
- 27.
- van der Vegt AH, Scott IA, Dermawan K, et al. Deployment of machine learning algorithms to predict sepsis: systematic review and application of the SALIENT clinical AI implementation framework. J Am Med Inform Assoc. 2023 Jun 20;30(7):1349–61. doi: 10.1093/jamia/ocad075. PMID: 37172264. [PMC free article: PMC10280361] [PubMed: 37172264] [CrossRef]
- 28.
- Schootman M, Wiskow C, Loux T, et al. Evaluation of the effectiveness of an automated sepsis predictive tool on patient outcomes. J Crit Care. 2022 Oct;71:154061. doi: 10.1016/j.jcrc.2022.154061. PMID: 35598533. [PubMed: 35598533] [CrossRef]
- 29.
- Dhudasia MB, Benitz WE, Flannery DD, et al. Diagnostic Performance and Patient Outcomes With C-Reactive Protein Use in Early-Onset Sepsis Evaluations. J Pediatr. 2023 May;256:98–104.e6. doi: 10.1016/j.jpeds.2022.12.007. PMID: 36529283. [PMC free article: PMC10164676] [PubMed: 36529283] [CrossRef]
- 30.
- Downing NL, Rolnick J, Poole SF, et al. Electronic health record-based clinical decision support alert for severe sepsis: a randomised evaluation. BMJ Qual Saf. 2019 Sep;28(9):762–8. doi: 10.1136/bmjqs-2018-008765. PMID: 30872387. [PMC free article: PMC6860967] [PubMed: 30872387] [CrossRef]
- 31.
- Horton DJ, Graves KK, Kukhareva PV, et al. Modified early warning score-based clinical decision support: cost impact and clinical outcomes in sepsis. JAMIA Open. 2020 Jul;3(2):261–8. doi: 10.1093/jamiaopen/ooaa014. PMID: 32734167. [PMC free article: PMC7382614] [PubMed: 32734167] [CrossRef]
- 32.
- Mixon M, Dietrich S, Floren M, et al. Time to antibiotic administration: Sepsis alerts called in emergency department versus in the field via emergency medical services. Am J Emerg Med. 2021 Jun;44:291–5. doi: 10.1016/j.ajem.2020.04.008. PMID: 32321681. [PubMed: 32321681] [CrossRef]
- 33.
- Schertz AR, Smith SA, Lenoir KM, et al. Clinical Impact of a Sepsis Alert System Plus Electronic Sepsis Navigator Using the Epic Sepsis Prediction Model in the Emergency Department. J Emerg Med. 2023 May;64(5):584–95. doi: 10.1016/j.jemermed.2023.02.025. PMID: 37045722. [PubMed: 37045722] [CrossRef]
- 34.
- Tarabichi Y, Cheng A, Bar-Shain D, et al. Improving Timeliness of Antibiotic Administration Using a Provider and Pharmacist Facing Sepsis Early Warning System in the Emergency Department Setting: A Randomized Controlled Quality Improvement Initiative. Crit Care Med. 2022 Mar 1;50(3):418–27. doi: 10.1097/ccm.0000000000005267. PMID: 34415866. [PubMed: 34415866] [CrossRef]
- 35.
- Achten NB, Klingenberg C, Benitz WE, et al. Association of Use of the Neonatal Early-Onset Sepsis Calculator With Reduction in Antibiotic Therapy and Safety: A Systematic Review and Meta-analysis. JAMA Pediatr. 2019 Nov 1;173(11):1032–40. doi: 10.1001/jamapediatrics.2019.2825. PMID: 31479103. [PMC free article: PMC6724419] [PubMed: 31479103] [CrossRef]
- 36.
- Deshmukh M, Mehta S, Patole S. Sepsis calculator for neonatal early onset sepsis - a systematic review and meta-analysis. J Matern Fetal Neonatal Med. 2021 Jun;34(11):1832–40. doi: 10.1080/14767058.2019.1649650. PMID: 31352846. [PubMed: 31352846] [CrossRef]
- 37.
- Persad E, Jost K, Honoré A, et al. Neonatal sepsis prediction through clinical decision support algorithms: A systematic review. Acta Paediatr. 2021 Dec;110(12):3201–26. doi: 10.1111/apa.16083. PMID: 34432903. [PubMed: 34432903] [CrossRef]
- 38.
- Warttig S, Alderson P, Evans DJ, et al. Automated monitoring compared to standard care for the early detection of sepsis in critically ill patients. Cochrane Database Syst Rev. 2018 Jun 25;6(6):Cd012404. doi: 10.1002/14651858.CD012404.pub2. PMID: 29938790. [PMC free article: PMC6353245] [PubMed: 29938790] [CrossRef]
- 39.
- Kausch SL, Moorman JR, Lake DE, et al. Physiological machine learning models for prediction of sepsis in hospitalized adults: An integrative review. Intensive Crit Care Nurs. 2021 Aug;65:103035. doi: 10.1016/j.iccn.2021.103035. PMID: 33875337. [PubMed: 33875337] [CrossRef]
- 40.
- robvis (visualization tool). 2023. https://www
​.riskofbias ​.info/welcome/robvis-visualization-tool. Accessed on January 31, 2024. - 41.
- Fleischmann-Struzek C, Goldfarb DM, Schlattmann P, et al. The global burden of paediatric and neonatal sepsis: a systematic review. Lancet Respir Med. 2018 Mar;6(3):223–30. doi: 10.1016/s2213-2600(18)30063-8. PMID: 29508706. [PubMed: 29508706] [CrossRef]
- 42.
- Hayes R, Hartnett J, Semova G, et al. Neonatal sepsis definitions from randomised clinical trials. Pediatr Res. 2023 Apr;93(5):1141–8. doi: 10.1038/s41390-021-01749-3. PMID: 34743180. [PMC free article: PMC10132965] [PubMed: 34743180] [CrossRef]
- 43.
- The Surviving Sepsis Campaign Mount Prospect, IL: Society of Critical Care Medicine. https://www
​.sccm.org ​/SurvivingSepsisCampaign/Home. Accessed on November 14, 2023. - 44.
- Hospital toolkit for adult sepsis surveillance Centers for Disease Control and Prevention. Atlanta, GA: 2018. https://www
​.cdc.gov/sepsis ​/pdfs/sepsis-surveillance-toolkit-mar-2018_508.pdf - 45.
- Hospital Sepsis Program Core Elements. Centers for Disease Control and Prevention; 2023. https://www
​.cdc.gov/sepsis ​/core-elements.html. Accessed on November 14, 2023. - 46.
- Usman OA, Usman AA, Ward MA. Comparison of SIRS, qSOFA, and NEWS for the early identification of sepsis in the Emergency Department. Am J Emerg Med. 2019 Aug;37(8):1490–7. doi: 10.1016/j.ajem.2018.10.058. PMID: 30470600. [PubMed: 30470600] [CrossRef]
- 47.
- Islam MM, Nasrin T, Walther BA, et al. Prediction of sepsis patients using machine learning approach: A meta-analysis. Comput Methods Programs Biomed. 2019 Mar;170:1–9. doi: 10.1016/j.cmpb.2018.12.027. PMID: 30712598. [PubMed: 30712598] [CrossRef]
- 48.
- Fleuren LM, Klausch TLT, Zwager CL, et al. Machine learning for the prediction of sepsis: a systematic review and meta-analysis of diagnostic test accuracy. Intensive Care Med. 2020 Mar;46(3):383–400. doi: 10.1007/s00134-019-05872-y. PMID: 31965266. [PMC free article: PMC7067741] [PubMed: 31965266] [CrossRef]
- 49.
- Rhee C, Jones TM, Hamad Y, et al. Prevalence, Underlying Causes, and Preventability of Sepsis-Associated Mortality in US Acute Care Hospitals. JAMA Netw Open. 2019 Feb 1;2(2):e187571. doi: 10.1001/jamanetworkopen.2018.7571. PMID: 30768188. [PMC free article: PMC6484603] [PubMed: 30768188] [CrossRef]
- 50.
- Pak TR, Young J, McKenna CS, et al. Risk of Misleading Conclusions in Observational Studies of Time-to-Antibiotics and Mortality in Suspected Sepsis. Clin Infect Dis. 2023 Aug 2doi: 10.1093/cid/ciad450. PMID: 37531612. [PMC free article: PMC10686960] [PubMed: 37531612] [CrossRef]
- 51.
- Vela D, Sharp A, Zhang R, et al. Temporal quality degradation in AI models. Sci Rep. 2022 Jul 8;12(1):11654. doi: 10.1038/s41598-022-15245-z. PMID: 35803963. [PMC free article: PMC9270447] [PubMed: 35803963] [CrossRef]
- 52.
- Rahmani K, Thapa R, Tsou P, et al. Assessing the effects of data drift on the performance of machine learning models used in clinical sepsis prediction. Int J Med Inform. 2023 May;173:104930. doi: 10.1016/j.ijmedinf.2022.104930. PMID: 36893656. [PubMed: 36893656] [CrossRef]
- 53.
- Prest J, Sathananthan M, Jeganathan N. Current Trends in Sepsis-Related Mortality in the United States. Crit Care Med. 2021 Aug 1;49(8):1276–84. doi: 10.1097/ccm.0000000000005017. PMID: 34261926. [PubMed: 34261926] [CrossRef]
- 54.
- Luhr R, Cao Y, Söderquist B, et al. Trends in sepsis mortality over time in randomised sepsis trials: a systematic literature review and meta-analysis of mortality in the control arm, 2002-2016. Crit Care. 2019 Jul 3;23(1):241. doi: 10.1186/s13054-019-2528-0. PMID: 31269976. [PMC free article: PMC6610784] [PubMed: 31269976] [CrossRef]
- 55.
- Stevenson EK, Rubenstein AR, Radin GT, et al. Two decades of mortality trends among patients with severe sepsis: a comparative meta-analysis*. Crit Care Med. 2014 Mar;42(3):625–31. doi: 10.1097/ccm.0000000000000026. PMID: 24201173. [PMC free article: PMC4313930] [PubMed: 24201173] [CrossRef]
- 56.
- Bolte TB, Swanson MB, Kaldjian AM, et al. Hospitals That Report Severe Sepsis and Septic Shock Bundle Compliance Have More Structured Sepsis Performance Improvement. J Patient Saf. 2022 Dec 1;18(8):e1231–e6. doi: 10.1097/pts.0000000000001062. PMID: 35858483. [PMC free article: PMC9722504] [PubMed: 35858483] [CrossRef]
- 57.
- Roney JK, Whitley BE, Long JD. Implementation of a MEWS-Sepsis screening tool: Transformational outcomes of a nurse-led evidence-based practice project. Nurs Forum. 2020 Apr;55(2):144–8. doi: 10.1111/nuf.12408. PMID: 31705549. [PubMed: 31705549] [CrossRef]
- 58.
- Davis C, Faruki A, Breyer D, et al. The Case for Virtual Sepsis Surveillance and Intervention. Telemed J E Health. 2022 Jan;28(1):102–6. doi: 10.1089/tmj.2020.0513. PMID: 33826409. [PubMed: 33826409] [CrossRef]
- 59.
- Simon EL, Truss K, Smalley CM, et al. Improved hospital mortality rates after the implementation of emergency department sepsis teams. Am J Emerg Med. 2022 Jan;51:218–22. doi: 10.1016/j.ajem.2021.10.035. PMID: 34775195. [PubMed: 34775195] [CrossRef]
- 60.
- MacMillan A, Rudinsky D, Han G, et al. Multidisciplinary Approach to Improve Sepsis Outcomes. J Healthc Qual. 2019 Jul/Aug;41(4):220–7. doi: 10.1097/jhq.0000000000000166. PMID: 30418300. [PubMed: 30418300] [CrossRef]
- 61.
- Gibbs KD, Shi Y, Sanders N, et al. Evaluation of a Sepsis Alert in the Pediatric Acute Care Setting. Appl Clin Inform. 2021 May;12(3):469–78. doi: 10.1055/s-0041-1730027. PMID: 34041734. [PMC free article: PMC8154346] [PubMed: 34041734] [CrossRef]
- 62.
- Eisenberg MA, Freiman E, Capraro A, et al. Outcomes of Patients with Sepsis in a Pediatric Emergency Department after Automated Sepsis Screening. J Pediatr. 2021 Aug;235:239–45.e4. doi: 10.1016/j.jpeds.2021.03.053. PMID: 33798508. [PubMed: 33798508] [CrossRef]
- 63.
- Vidrine R, Zackoff M, Paff Z, et al. Improving Timely Recognition and Treatment of Sepsis in the Pediatric ICU. Jt Comm J Qual Patient Saf. 2020 May;46(5):299–307. doi: 10.1016/j.jcjq.2020.02.005. PMID: 32201121. [PubMed: 32201121] [CrossRef]
- 64.
- Borrelli G, Koch E, Sterk E, et al. Early recognition of sepsis through emergency medical services pre-hospital screening. Am J Emerg Med. 2019 Aug;37(8):1428–32. doi: 10.1016/j.ajem.2018.10.036. PMID: 30366742. [PubMed: 30366742] [CrossRef]
- 65.
- Huff S, Stephens K, Whiteman K, et al. Implementation of a Vital Sign Alert System to Improve Outcomes. J Nurs Care Qual. 2019 Oct/Dec;34(4):346–51. doi: 10.1097/ncq.0000000000000384. PMID: 30585982. [PubMed: 30585982] [CrossRef]
- 66.
- Lipatov K, Daniels CE, Park JG, et al. Implementation and evaluation of sepsis surveillance and decision support in medical ICU and emergency department. Am J Emerg Med. 2022 Jan;51:378–83. doi: 10.1016/j.ajem.2021.09.086. PMID: 34823194. [PubMed: 34823194] [CrossRef]
- 67.
- Baker AH, Monuteaux MC, Madden K, et al. Effect of a Sepsis Screening Algorithm on Care of Children with False-Positive Sepsis Alerts. J Pediatr. 2021 Apr;231:193–9.e1. doi: 10.1016/j.jpeds.2020.12.034. PMID: 33358842. [PubMed: 33358842] [CrossRef]
- 68.
- Miller H, Tseng A, Lowerre T, et al. Improving Time to Stat Intravenous Antibiotic Administration: An 8-Year Quality Initiative. Hosp Pediatr. 2023 Jan 1;13(1):88–94. doi: 10.1542/hpeds.2021-006422. PMID: 36545766. [PubMed: 36545766] [CrossRef]
- 69.
- Mittal Y, Sankar J, Dhochak N, et al. Decreasing the Time to Administration of First Dose of Antibiotics in Children With Severe Sepsis. J Healthc Qual. 2019 Jan/Feb;41(1):32–8. doi: 10.1097/jhq.0000000000000141. PMID: 30247207. [PubMed: 30247207] [CrossRef]
- 70.
- Oddiri U, Propper G, Brill P, et al. Early Identification of Severe Sepsis in Pediatric Patients Using an Electronic Alert System. Hosp Pediatr. 2023 Feb 1;13(2):174–82. doi: 10.1542/hpeds.2022-006587. PMID: 36695040. [PubMed: 36695040] [CrossRef]
- 71.
- Threatt DL. Improving Sepsis Bundle Implementation Times: A Nursing Process Improvement Approach. J Nurs Care Qual. 2020 Apr/Jun;35(2):135–9. doi: 10.1097/ncq.0000000000000430. PMID: 31290779. [PubMed: 31290779] [CrossRef]
- 72.
- Toews JR, Leonard JC, Shi J, et al. Implementation of an Automated Sepsis Screening Tool in a Children’s Hospital Emergency Department: A Cost Analysis. J Pediatr. 2022 Nov;250:38–44.e1. doi: 10.1016/j.jpeds.2022.06.026. PMID: 35772510. [PubMed: 35772510] [CrossRef]
- 73.
- Afshar M, Arain E, Ye C, et al. Patient Outcomes and Cost-Effectiveness of a Sepsis Care Quality Improvement Program in a Health System. Crit Care Med. 2019 Oct;47(10):1371–9. doi: 10.1097/ccm.0000000000003919. PMID: 31306176. [PMC free article: PMC7195842] [PubMed: 31306176] [CrossRef]
- 74.
- Jung AD, Baker J, Droege CA, et al. Sooner is better: use of a real-time automated bedside dashboard improves sepsis care. J Surg Res. 2018 Nov;231:373–9. doi: 10.1016/j.jss.2018.05.078. PMID: 30278956. [PubMed: 30278956] [CrossRef]
- 75.
- Moore WR, Vermuelen A, Taylor R, et al. Improving 3-Hour Sepsis Bundled Care Outcomes: Implementation of a Nurse-Driven Sepsis Protocol in the Emergency Department. J Emerg Nurs. 2019 Nov;45(6):690–8. doi: 10.1016/j.jen.2019.05.005. PMID: 31235077. [PubMed: 31235077] [CrossRef]
- 76.
- Dewan M, Vidrine R, Zackoff M, et al. Design, Implementation, and Validation of a Pediatric ICU Sepsis Prediction Tool as Clinical Decision Support. Appl Clin Inform. 2020 Mar;11(2):218–25. doi: 10.1055/s-0040-1705107. PMID: 32215893. [PMC free article: PMC7096320] [PubMed: 32215893] [CrossRef]
- 77.
- Alnababteh MH, Huang SS, Ryan A, et al. A Multimodal Sepsis Quality-Improvement Initiative Including 24/7 Screening and a Dedicated Sepsis Response Team-Reduced Readmissions and Mortality. Crit Care Explor. 2020 Dec;2(12):e0251. doi: 10.1097/cce.0000000000000251. PMID: 33251514. [PMC free article: PMC7688252] [PubMed: 33251514] [CrossRef]
- 78.
- Burdick H, Pino E, Gabel-Comeau D, et al. Effect of a sepsis prediction algorithm on patient mortality, length of stay and readmission: a prospective multicentre clinical outcomes evaluation of real-world patient data from US hospitals. BMJ Health Care Inform. 2020 Apr;27(1)doi: 10.1136/bmjhci-2019-100109. PMID: 32354696. [PMC free article: PMC7245419] [PubMed: 32354696] [CrossRef]
- 79.
- Delaveris SL, Cichetti JR, Edleblute E. 2019 John M. Eisenberg Patient Safety and Quality Awards: A Model Cell for Transformational Redesign of Sepsis Identification and Treatment: Aligning Digital Tools with Innovative Workflows. Jt Comm J Qual Patient Saf. 2020 Jul;46(7):392–9. doi: 10.1016/j.jcjq.2020.04.001. PMID: 32598282. [PubMed: 32598282] [CrossRef]
- 80.
- Perlin JB, Jackson E, Hall C, et al. 2019 John M. Eisenberg Patient Safety and Quality Awards: SPOTting Sepsis to Save Lives: A Nationwide Computer Algorithm for Early Detection of Sepsis: Innovation in Patient Safety and Quality at the National Level (Eisenberg Award). Jt Comm J Qual Patient Saf. 2020 Jul;46(7):381–91. doi: 10.1016/j.jcjq.2020.04.006. PMID: 32598281. [PubMed: 32598281] [CrossRef]
- 81.
- Mitzkewich M. Sepsis Screening in Triage to Decrease Door-to-Antibiotic Time. J Emerg Nurs. 2019 May;45(3):254–6. doi: 10.1016/j.jen.2018.08.002. PMID: 30195863. [PubMed: 30195863] [CrossRef]
- 82.
- Shah T, Sterk E, Rech MA. Emergency department sepsis screening tool decreases time to antibiotics in patients with sepsis. Am J Emerg Med. 2018 Oct;36(10):1745–8. doi: 10.1016/j.ajem.2018.01.060. PMID: 29395762. [PubMed: 29395762] [CrossRef]
- 83.
- Ahmed AM, Macapili E, Brenner MJ, et al. Accelerating Detection and Intervention for Sepsis in Skilled Nursing Facilities Using a Sepsis Pathway. J Nurs Care Qual. 2023 Jun 22doi: 10.1097/ncq.0000000000000729. PMID: 37350588. [PubMed: 37350588] [CrossRef]
- 84.
- Colorafi KJ, Ferrell K, D’Andrea A, et al. Influencing outcomes with automated time zero for sepsis through statistical validation and process improvement. Mhealth. 2019;5:36. doi: 10.21037/mhealth.2019.09.04. PMID: 31620463. [PMC free article: PMC6789198] [PubMed: 31620463] [CrossRef]
- 85.
- Iannello J, Maltese N. Improving Unadjusted and Adjusted Mortality With an Early Warning Sepsis System in the Emergency Department and Inpatient Wards. Fed Pract. 2021 Nov;38(11):508–15b. doi: 10.12788/fp.0194. PMID: 35136335. [PMC free article: PMC8815614] [PubMed: 35136335] [CrossRef]
- 86.
- Gaieski DF, Carr B, Toolan M, et al. Can an End-to-End Telesepsis Solution Improve the Severe Sepsis and Septic Shock Management Bundle-1 Metrics for Sepsis Patients Admitted From the Emergency Department to the Hospital? Crit Care Explor. 2022 Oct;4(10):e0767. doi: 10.1097/cce.0000000000000767. PMID: 36248316. [PMC free article: PMC9553400] [PubMed: 36248316] [CrossRef]
- 87.
- Cull J, Brevetta R, Gerac J, et al. Epic Sepsis Model Inpatient Predictive Analytic Tool: A Validation Study. Crit Care Explor. 2023 Jul;5(7):e0941. doi: 10.1097/cce.0000000000000941. PMID: 37405252. [PMC free article: PMC10317482] [PubMed: 37405252] [CrossRef]
- 88.
- Stellpflug C, Pierson L, Roloff D, et al. Continuous Physiological Monitoring Improves Patient Outcomes. Am J Nurs. 2021 Apr 1;121(4):40–6. doi: 10.1097/01.NAJ.0000742504.44428.c9. PMID: 33755624. [PubMed: 33755624] [CrossRef]
- 89.
- Polito CC, Bloom I, Dunn C, et al. Implementation of an EMS protocol to improve prehospital sepsis recognition. Am J Emerg Med. 2022 Jul;57:34–8. doi: 10.1016/j.ajem.2022.04.035. PMID: 35500527. [PubMed: 35500527] [CrossRef]
- 90.
- Giannini HM, Ginestra JC, Chivers C, et al. A Machine Learning Algorithm to Predict Severe Sepsis and Septic Shock: Development, Implementation, and Impact on Clinical Practice. Crit Care Med. 2019 Nov;47(11):1485–92. doi: 10.1097/ccm.0000000000003891. PMID: 31389839. [PMC free article: PMC8635476] [PubMed: 31389839] [CrossRef]
- 91.
- Forget A, Adegboye C, Alfieri M, et al. A sepsis trigger tool reduces time to antibiotic administration in the NICU. J Perinatol. 2023 Jun;43(6):806–12. doi: 10.1038/s41372-023-01636-1. PMID: 36813901. [PubMed: 36813901] [CrossRef]
- 92.
- Gaieski DF, Carr B, Toolan M, et al. End-to-End Sepsis Solution Incorporating Expert Telemedicine Consultation. Telemed J E Health. 2023 Apr 10doi: 10.1089/tmj.2022.0369. PMID: 37036813. [PubMed: 37036813] [CrossRef]
Acknowledgments
The authors would like to thank Ellen S. Deutsch, M.D., M.S., Medical Officer with AHRQ’s Division of General Patient Safety, for her review and input on the report.
Peer Reviewers
The list of Peer Reviewers follows:
- Mitchell Levy, M.D., M.C.C.M., F.C.C.P.Professor of MedicineChief of the Division of Critical Care, Pulmonary, and Sleep MedicineThe Warren Alpert Medical School of Brown UniversityProvidence, RI
- Hallie Prescott, M.D., M.Sc.Associate Professor in Pulmonary & Critical Care Medicine, University of MichiganStaff Physician, VA Ann Arbor Healthcare SystemAnn Arbor, MI
Afterword
Recognized for excellence in conducting comprehensive systematic reviews, the Agency for Healthcare Research and Quality (AHRQ) Evidence-based Practice Center (EPC) Program is developing a range of rapid evidence products to assist end-users in making specific decisions in a limited timeframe. AHRQ recognizes that people are struggling with urgent questions on how to make healthcare safer. AHRQ is using this rapid format for the fourth edition of its Making Healthcare Safer series of reports, produced by the EPC Program and the General Patient Safety Program. To shorten timelines, reviewers make strategic choices about which processes to abridge. However, the adaptations made for expediency may limit the certainty and generalizability of the findings from the review, particularly in areas with a large literature base. Transparent reporting of the methods used and the resulting limitations of the evidence synthesis are extremely important.
AHRQ expects that these rapid evidence products will be helpful to health plans, providers, purchasers, government programs, and the healthcare system as a whole. Transparency and stakeholder input are essential to AHRQ. If you have comments related to this report, they may be sent by mail to the Task Order Officer named below at: Agency for Healthcare Research and Quality, 5600 Fishers Lane, Rockville, MD 20857, or by email to vog.shh.qrha@SHM.
- Robert Otto Valdez, Ph.D., M.H.S.A.DirectorAgency for Healthcare Research and Quality
- Therese Miller, D.P.H.DirectorCenter for Evidence and Practice ImprovementAgency for Healthcare Research and Quality
- Christine Chang, M.D., M.P.H.DirectorEvidence-based Practice Center DivisionCenter for Evidence and Practice ImprovementAgency for Healthcare Research and Quality
- David W. Niebuhr, M.D., M.P.H., M.Sc.Evidence-based Practice Center Division LiaisonCenter for Evidence and Practice ImprovementAgency for Healthcare Research and Quality
- Craig A. Umscheid, M.D., M.S.DirectorCenter for Quality Improvement and Patient SafetyAgency for Healthcare Research and Quality
- Margie Shofer, B.S.N., M.B.A.Director, General Patient Safety ProgramCenter for Quality Improvement and Patient SafetyAgency for Healthcare Research and Quality
- Jennifer EskandariTask Order OfficerCenter for Quality Improvement and Patient SafetyAgency for Healthcare Research and Quality
- Farzana Samad, Pharm.D., FISMP, CPPSHealth Scientist AdministratorCenter for Quality Improvement and Patient SafetyAgency for Healthcare Research and Quality
Appendixes
Appendix A. Methods
Search Strategies for Published Literature
Table A-1PubMed search strategy
# | Concept | Search Terms |
---|---|---|
1 | Sepsis | “sepsis prediction”[tiab:~2] OR “Sepsis onset”[tiab] OR “sepsis identification”[tiab:~1] OR “sepsis detection”[tiab:~1] OR “Sepsis development”[tiab:~1] OR “sepsis recognize”[tiab:~1] OR “sepsis diagnosis”[tiab:~1] OR “sepsis screening”[tiab:~1] OR “sepsis monitoring”[tiab:~1] OR “sepsis surveillance”[tiab:~1] OR “sepsis prognosis” [tiab:~1] OR “sepsis progress” [tiab:~1] OR “sepsis recognition” [tiab:~1] OR “sepsis treatment” [tiab:~0] OR “sepsis alert”[tiab:~0] OR “sepsis alerts”[tiab:~0] OR “severe sepsis” [ti:~0] |
2 | Sepsis #2 | sepsis[tiab] OR sepsis [mh] AND (“Triage Tool”[tiab] OR “Early Warning”[tiab] OR “clinical decision Support”[tiab] OR “prediction model*”[tiab] OR “prediction algorithm”[tiab] OR “predictive system”[tiab]) |
3 | # 1 OR #2 | |
4 | Patient safety and Harms | “patient safety”[mh] OR “patient safety” [tiab] OR “Patient Harm”[mh] OR “Patient Harm*”[tiab] OR “patient risk*”[tiab] OR “quality care” [tiab] OR “adverse event*”[tiab] OR “undesired event*”[tiab] OR “medical errors”[mh] OR “medical error*”[tiab] OR “Diagnostic Errors” [mh] OR “diagnostic error*”[tiab] OR “diagnostic mistake*”[tiab] OR “health care error*”[tiab] OR “healthcare error*”[tiab] OR “medical fault*”[tiab] OR “medical mistake*”[tiab] OR “erroneous diagnos*”[tiab] OR “failure to diagnose”[tiab] OR “false diagnos*”[tiab] OR “faulty diagnos*”[tiab] OR misdiagnos*[tiab] OR “mistaken diagnos*”[tiab] OR “wrong diagnos*”[tiab] OR “risk assessment”[Title/Abstract] OR “length of stay”[Title/Abstract] OR “Quality Improvement” [tiab] OR “Quality Improvement” [mh] OR mortality [tiab] OR mortality [mh:noexp] OR “hospital mortality”[mh:noexp] OR performance [tiab] OR compliance [tiab] OR bundle [tiab] OR “patient outcomes” [tiab:~1] OR “appropriate management”[tiab] OR adherence [tiab] OR “time to” [tiab:~0] OR “Costs and Cost Analysis”[Mesh] OR Cost*[Tiab] OR economic*[tiab] OR “economics”[Subheading] OR “health care costs” [tiab:~0] OR “hospital costs” [tiab] OR “healthcare costs” [tiab:~0] |
5 | Exclude | (“Animals”[Mesh] NOT “Humans”[Mesh]) OR address[pt] OR “autobiography”[pt] OR “bibliography”[pt] OR “biography”[pt] OR congress[pt] OR “dictionary”[pt] OR “directory”[pt] OR “festschrift”[pt] OR “historical article”[pt] OR lecture[pt] OR “legal case”[pt] OR “legislation”[pt] OR “periodical index”[pt] OR Comment[pt] OR Letter[pt] OR Editorial[pt] OR “news”[pt] OR “newspaper article”[pt] OR “patient education handout”[pt] OR “periodical index”[pt] OR “study guide”[pt] OR “Study protocol” [ti] OR “trial protocol” [ti] OR “review protocol” [ti] OR editorial[pt] OR letter[pt] OR “case reports”[pt]OR rats[tw] OR cow[tw] OR cows[tw] OR chicken[tw] OR chickens[tw] OR horse[tw] OR horses[tw] OR mice[tw] OR mouse[tw] OR bovine[tw] OR sheep[tw] OR ovine [tw] OR murine[tw] OR “environmental scan”[ti] |
6 | #5 NOT #6 | |
7 | 8. PubMed filter [ publication date January 2018 – August 17th, 2023, | |
8 | 6. PubMed filter [English] |
Table A-2Cochrane search strategy
# | Concept | Search Terms |
---|---|---|
1 | Sepsis | (“sepsis prediction” OR “Sepsis onset” OR “sepsis identification” OR “sepsis detection” OR “Sepsis development” OR “sepsis recognize” OR “sepsis diagnosis” OR “sepsis screening” OR “sepsis monitoring” OR “sepsis surveillance” OR “sepsis prognosis” OR “sepsis progress” OR “sepsis recognition” OR “sepsis treatment” OR “sepsis alert” OR “sepsis alerts” OR “severe sepsis”):ti OR (“sepsis prediction” OR “Sepsis onset” OR “sepsis identification” OR “sepsis detection” OR “Sepsis development” OR “sepsis recognize” OR “sepsis diagnosis” OR “sepsis screening” OR “sepsis monitoring” OR “sepsis surveillance” OR “sepsis prognosis” OR “sepsis progress” OR “sepsis recognition” OR “sepsis treatment” OR “sepsis alert” OR “sepsis alerts”):ab |
2 | Sepsis #2 | (Sepsis:ti OR Sepsis:ab OR sepsis [mh]) AND ((“Triage Tool” OR “Early Warning” OR “clinical decision Support” OR “prediction model*” OR “prediction algorithm” OR “predictive system”):ti OR (“Triage Tool” OR “Early Warning” OR “clinical decision Support” OR “prediction model*” OR “prediction algorithm” OR “predictive system”):ab) |
3 | # 1 OR #2 | |
4. |
4 Patient safety and Harms [additional terms added to the standard search string are highlighted] | (“patient safety” OR “patient harm” OR “patient risk” OR “quality care” OR “adverse event” OR “undesired event” OR “medical error” OR “diagnostic error” OR “diagnostic mistake” OR “health care error” OR “healthcare error” OR “medical fault” OR “medical mistake” OR “erroneous diagnose” OR “erroneous diagnoses” OR “failure to diagnose” OR “false diagnose” OR “false diagnoses” OR “faulty diagnose” OR “faulty diagnoses” OR “mistaken diagnose” OR “mistaken diagnoses” OR “wrong diagnose” OR “wrong diagnoses” OR “risk assessment” OR “length of stay” OR “quality improvement” OR “patient outcomes” OR “appropriate management” OR “time to” OR “health care costs” OR “hospital costs” OR “healthcare costs” OR misdiagnose OR misdiagnoses OR misdiagnosis OR mortality OR performance OR compliance OR bundle OR adherence OR cost OR economic):ti OR (“patient safety” OR “patient harm” OR “patient risk” OR “quality care” OR “adverse event” OR “undesired event” OR “medical error” OR “diagnostic error” OR “diagnostic mistake” OR “health care error” OR “healthcare error” OR “medical fault” OR “medical mistake” OR “erroneous diagnose” OR “erroneous diagnoses” OR “failure to diagnose” OR “false diagnose” OR “false diagnoses” OR “faulty diagnose” OR “faulty diagnoses” OR “mistaken diagnose” OR “mistaken diagnoses” OR “wrong diagnose” OR “wrong diagnoses” OR “risk assessment” OR “length of stay” OR “quality improvement” OR “patient outcomes” OR “appropriate management” OR “time to” OR “health care costs” OR “hospital costs” OR “healthcare costs” OR misdiagnose OR misdiagnoses OR misdiagnosis OR mortality OR performance OR compliance OR bundle OR adherence OR cost OR economic):ab OR “patient safety”[mh] OR “Patient Harm”[mh] OR “medical errors”[mh] OR “Diagnostic Errors” [mh] OR “Quality Improvement” [mh] OR mortality [mh:noexp] OR “hospital mortality”[mh:noexp] OR “Costs and Cost Analysis”[mh] OR “economics”[mh] |
5 | 5 | #3 AND #4 |
6 | 6 [ Exclude] | (address OR “autobiography” OR “bibliography” OR “biography” OR congress OR “dictionary” OR “directory” OR “festschrift” OR “historical article” OR lecture OR “legal case” OR “legislation” OR “periodical index” OR Comment OR Letter OR Editorial OR “news” OR “newspaper article” OR “patient education handout” OR “periodical index” OR “study guide” OR editorial OR letter OR “case reports”):pt OR (“Study protocol” OR “trial protocol” OR “review protocol” OR “environmental scan”):ti OR (rats OR cow OR cows OR chicken OR chickens OR horse OR horses OR mice OR mouse OR bovine OR sheep OR ovine OR murine):kw |
#7 | 7 | #5 NOT #6 |
#8 | Cochrane filter [ publication date 2018 – August 17th, 2023 | |
#9 | Cochrane filter [English] and HUMANS only |
Appendix B. List of Excluded Studies Upon Full-Text Review
- 1.
- Achten NB, Dorigo-Zetsma JW, van der Linden PD, et al. Sepsis calculator implementation reduces empiric antibiotics for suspected early-onset sepsis. Eur J Pediatr. 2018 May;177(5):741–6. doi: 10.1007/s00431-018-3113-2. PMID: 29455368. - Non-USA based study or does not report data separately for USA [PubMed: 29455368] [CrossRef]
- 2.
- Ackermann K, Baker J, Festa M, et al. Computerized Clinical Decision Support Systems for the Early Detection of Sepsis Among Pediatric, Neonatal, and Maternal Inpatients: Scoping Review. JMIR Med Inform. 2022 May 6;10(5):e35061. doi: 10.2196/35061. PMID: 35522467. - Other: Scoping review [PMC free article: PMC9123549] [PubMed: 35522467] [CrossRef]
- 3.
- Ackermann K, Baker J, Green M, et al. Computerized Clinical Decision Support Systems for the Early Detection of Sepsis Among Adult Inpatients: Scoping Review. J Med Internet Res. 2022 Feb 23;24(2):e31083. doi: 10.2196/31083. PMID: 35195528. - Other: Scoping review [PMC free article: PMC8908200] [PubMed: 35195528] [CrossRef]
- 4.
- Adams R, Henry KE, Sridharan A, et al. Prospective, multi-site study of patient outcomes after implementation of the TREWS machine learning-based early warning system for sepsis. Nat Med. 2022 Jul;28(7):1455–60. doi: 10.1038/s41591-022-01894-0. PMID: 35864252. - Comparison group does not meet inclusion criteria [PubMed: 35864252] [CrossRef]
- 5.
- Aguirre U, Urrechaga E. Diagnostic performance of machine learning models using cell population data for the detection of sepsis: a comparative study. Clin Chem Lab Med. 2023 Jan 27;61(2):356–65. doi: 10.1515/cclm-2022-0713. PMID: 36351434. - Intervention does not include a prediction or recognition component [PubMed: 36351434] [CrossRef]
- 6.
- Alessi LJ, Warmus HR, Schaffner EK, et al. A Computable Definition of Sepsis Facilitates Screening and Performance Improvement Tracking. Pediatr Qual Saf. 2018 Mar;3(2):e067. doi: 10.1097/pq9.0000000000000067. PMID: 29732457. - Conference, meeting abstract, or poster [PMC free article: PMC5916476] [PubMed: 29732457] [CrossRef]
- 7.
- Almutary A, Althunayyan S, Alenazi K, et al. National Early Warning Score (NEWS) as Prognostic Triage Tool for Septic Patients. Infect Drug Resist. 2020;13:3843–51. doi: 10.2147/idr.s275390. PMID: 33149629. - Non-USA based study or does not report data separately for USA [PMC free article: PMC7602891] [PubMed: 33149629] [CrossRef]
- 8.
- Alturki A, Al-Eyadhy A, Alfayez A, et al. Impact of an electronic alert system for pediatric sepsis screening a tertiary hospital experience. Sci Rep. 2022 Jul 20;12(1):12436. doi: 10.1038/s41598-022-16632-2. PMID: 35859000. - Non-USA based study or does not report data separately for USA [PMC free article: PMC9300636] [PubMed: 35859000] [CrossRef]
- 9.
- Ashana DC, Anesi GL, Liu VX, et al. Equitably Allocating Resources during Crises: Racial Differences in Mortality Prediction Models. Am J Respir Crit Care Med. 2021 Jul 15;204(2):178–86. doi: 10.1164/rccm.202012-4383OC. PMID: 33751910. - No appropriate outcomes [PMC free article: PMC8759151] [PubMed: 33751910] [CrossRef]
- 10.
- Bader MZ, Obaid AT, Al-Khateb HM, et al. Developing Adult Sepsis Protocol to Reduce the Time to Initial Antibiotic Dose and Improve Outcomes among Patients with Cancer in Emergency Department. Asia Pac J Oncol Nurs. 2020 Oct–Dec;7(4):355–60. doi: 10.4103/apjon.apjon_32_20. PMID: 33062830. - Non-USA based study or does not report data separately for USA [PMC free article: PMC7529030] [PubMed: 33062830] [CrossRef]
- 11.
- Ballester L, Martínez R, Méndez J, et al. Differences in Hypotensive vs. Non-Hypotensive Sepsis Management in the Emergency Department: Door-to-Antibiotic Time Impact on Sepsis Survival. Med Sci (Basel). 2018 Oct 10;6(4)doi: 10.3390/medsci6040091. PMID: 30309044. - Non-USA based study or does not report data separately for USA [PMC free article: PMC6313793] [PubMed: 30309044] [CrossRef]
- 12.
- Baniasadi A, Rezaeirad S, Zare H, et al. Two-Step Imputation and AdaBoost-Based Classification for Early Prediction of Sepsis on Imbalanced Clinical Data. Crit Care Med. 2021 Jan 1;49(1):e91–e7. doi: 10.1097/ccm.0000000000004705. PMID: 33156121. - Intervention does not include a prediction or recognition component [PubMed: 33156121] [CrossRef]
- 13.
- Bansal V, Festić E, Mangi MA, et al. Early Machine-Human Interface around Sepsis Severity Identification: From Diagnosis to Improved Management? Acta Med Acad. 2018 May;47(1):27–38. doi: 10.5644/ama2006-124.212. PMID: 29957969. - No appropriate outcomes [PubMed: 29957969] [CrossRef]
- 14.
- Barbara P, Graziano C, Caputo W, et al. The quick sequential organ failure assessment (qSOFA) identifies septic patients in the out-of-hospital setting. Am J Emerg Med. 2018 Jun;36(6):1022–6. doi: 10.1016/j.ajem.2018.01.073. PMID: 29426799. - No appropriate outcomes [PubMed: 29426799] [CrossRef]
- 15.
- Barbash IJ, Davis BS, Yabes JG, et al. Treatment Patterns and Clinical Outcomes After the Introduction of the Medicare Sepsis Performance Measure (SEP-1). Ann Intern Med. 2021 Jul;174(7):927–35. doi: 10.7326/m20-5043. PMID: 33872042. - Intervention does not include a prediction or recognition component [PMC free article: PMC8844885] [PubMed: 33872042] [CrossRef]
- 16.
- Barton C, Chettipally U, Zhou Y, et al. Evaluation of a machine learning algorithm for up to 48-hour advance prediction of sepsis using six vital signs. Comput Biol Med. 2019 Jun;109:79–84. doi: 10.1016/j.compbiomed.2019.04.027. PMID: 31035074. - Intervention does not include a prediction or recognition component [PMC free article: PMC6556419] [PubMed: 31035074] [CrossRef]
- 17.
- Barton C, Shimabakuru D, Feldman M, et al. Effect of a machine learning-based severe sepsis prediction algorithm on patient survival. Critical care medicine. 2018;46:699. doi: 10.1097/01.ccm.0000529432.50757.3c. PMID: CN-01452229. - Conference, meeting abstract, or poster [CrossRef]
- 18.
- Beneyto-Ripoll C, Palazón-Bru A, Llópez-Espinós P, et al. A critical appraisal of the prognostic predictive models for patients with sepsis: Which model can be applied in clinical practice? Int J Clin Pract. 2021 Aug;75(8):e14044. doi: 10.1111/ijcp.14044. PMID: 33492724. - No appropriate outcomes [PubMed: 33492724] [CrossRef]
- 19.
- Bolte TB, Swanson MB, Kaldjian AM, et al. Hospitals That Report Severe Sepsis and Septic Shock Bundle Compliance Have More Structured Sepsis Performance Improvement. J Patient Saf. 2022 Dec 1;18(8):e1231–e6. doi: 10.1097/pts.0000000000001062. PMID: 35858483. - Intervention does not include a prediction or recognition component [PMC free article: PMC9722504] [PubMed: 35858483] [CrossRef]
- 20.
- Boskabadi H, Zakerihamidi M. Evaluate the diagnosis of neonatal sepsis by measuring interleukins: A systematic review. Pediatr Neonatol. 2018 Aug;59(4):329–38. doi: 10.1016/j.pedneo.2017.10.004. PMID: 29239828. - Intervention does not include a prediction or recognition component [PubMed: 29239828] [CrossRef]
- 21.
- Calderon K, Van Landingham E, Purcell S, et al. Identifying and treating sepsis in older people: a quality improvement project in hospitals and nursing homes in Texas. Nurs Older People. 2021 Jun 1;33(3):36–41. doi: 10.7748/nop.2021.e1308. PMID: 33565283. - Intervention does not include a prediction or recognition component [PubMed: 33565283] [CrossRef]
- 22.
- Carbó M, Fresco L, Osorio G, et al. Predictors of mortality in emergency department patients with sepsis scored 2 or 3 on the Quick Sequential Organ Failure Assessment scale. Emergencias. 2020 Jun;32(3):169–76. PMID: 32395924. - Non-USA based study or does not report data separately for USA [PubMed: 32395924]
- 23.
- Champagne HA, Garabedian MJ. Routine Screening for Sepsis in an Obstetric Population: Evaluation of an Improvement Project. Perm J. 2020 Nov;24:1–10. doi: 10.7812/tpp/19.232. PMID: 33482959. - No intervention [PMC free article: PMC7849262] [PubMed: 33482959] [CrossRef]
- 24.
- Chen KF, Tsai MY, Wu CC, et al. Effectiveness of Treatments and Diagnostic Tools and Declining Mortality in Patients With Severe Sepsis: A 12-Year Population-Based Cohort Study. J Intensive Care Med. 2020 Dec;35(12):1418–25. doi: 10.1177/0885066619827270. PMID: 30700200. - Non-USA based study or does not report data separately for USA [PubMed: 30700200] [CrossRef]
- 25.
- Chertoff J, Stevenson P, Alnuaimat H. Sepsis Mortality in the U.S. Correctional System: An Underappreciated Disparity. J Correct Health Care. 2018 Oct;24(4):337–41. doi: 10.1177/1078345818792235. PMID: 30126314. - Intervention does not include a prediction or recognition component [PubMed: 30126314] [CrossRef]
- 26.
- Chimenti C, Sears G, McIntyre J. Sepsis in Home Health Care: Screening, Education, and Rapid Triage. J Nurs Care Qual. 2021 Jul–Sep 01;36(3):210–6. doi: 10.1097/ncq.0000000000000525. PMID: 33079820. - No appropriate outcomes [PubMed: 33079820] [CrossRef]
- 27.
- Clar J, Oltra MR, Benavent R, et al. Prognostic value of diagnostic scales in community-acquired sepsis mortality at an emergency service. Prognosis in community-adquired sepsis. BMC Emerg Med. 2021 Dec 18;21(1):161. doi: 10.1186/s12873-021-00532-1. PMID: 34922448. - Non-USA based study or does not report data separately for USA [PMC free article: PMC8684687] [PubMed: 34922448] [CrossRef]
- 28.
- Crilly J, Robinson J, Sharman V, et al. Recognition, response and outcomes of sepsis: A dual site retrospective observational study. Int Emerg Nurs. 2019 Sep;46:100782. doi: 10.1016/j.ienj.2019.06.005. PMID: 31324537. - Non-USA based study or does not report data separately for USA [PubMed: 31324537] [CrossRef]
- 29.
- Desai MD, Tootooni MS, Bobay KL. Can Prehospital Data Improve Early Identification of Sepsis in Emergency Department? An Integrative Review of Machine Learning Approaches. Appl Clin Inform. 2022 Jan;13(1):189–202. doi: 10.1055/s-0042-1742369. PMID: 35108741. - No intervention [PMC free article: PMC8810268] [PubMed: 35108741] [CrossRef]
- 30.
- Downey C, Randell R, Brown J, et al. Continuous Versus Intermittent Vital Signs Monitoring Using a Wearable, Wireless Patch in Patients Admitted to Surgical Wards: Pilot Cluster Randomized Controlled Trial. J Med Internet Res. 2018 Dec 11;20(12):e10802. doi: 10.2196/10802. PMID: 30538086. - Non-USA based study or does not report data separately for USA [PMC free article: PMC6305881] [PubMed: 30538086] [CrossRef]
- 31.
- Downey CL, Croft J, Ainsworth G, et al. Trial of remote continuous versus intermittent NEWS monitoring after major surgery (TRaCINg): a feasibility randomised controlled trial. Pilot Feasibility Stud. 2020 Nov 23;6(1):183. doi: 10.1186/s40814-020-00709-8. PMID: 33292669. - Non-USA based study or does not report data separately for USA [PMC free article: PMC7684886] [PubMed: 33292669] [CrossRef]
- 32.
- Eisenberg M, Freiman E, Capraro A, et al. Comparison of Manual and Automated Sepsis Screening Tools in a Pediatric Emergency Department. Pediatrics. 2021 Feb;147(2)doi: 10.1542/peds.2020-022590. PMID: 33472987. - No appropriate outcomes [PubMed: 33472987] [CrossRef]
- 33.
- Eisenberg M, Madden K, Christianson JR, et al. Performance of an Automated Screening Algorithm for Early Detection of Pediatric Severe Sepsis. Pediatr Crit Care Med. 2019 Dec;20(12):e516–e23. doi: 10.1097/pcc.0000000000002101. PMID: 31567896. - No appropriate outcomes [PubMed: 31567896] [CrossRef]
- 34.
- Emmanuel J, Torres A. The Impact of Automated Electronic Surveillance of Electronic Medical Records on Pediatric Inpatient Care. Cureus. 2018 Oct 1;10(10):e3395. doi: 10.7759/cureus.3395. PMID: 30533330. - No appropriate outcomes [PMC free article: PMC6278995] [PubMed: 30533330] [CrossRef]
- 35.
- Evans IVR, Phillips GS, Alpern ER, et al. Association Between the New York Sepsis Care Mandate and In-Hospital Mortality for Pediatric Sepsis. Jama. 2018 Jul 24;320(4):358–67. doi: 10.1001/jama.2018.9071. PMID: 30043064. - Intervention does not include a prediction or recognition component [PMC free article: PMC6500448] [PubMed: 30043064] [CrossRef]
- 36.
- Fagerström J, Bång M, Wilhelms D, et al. LiSep LSTM: A Machine Learning Algorithm for Early Detection of Septic Shock. Sci Rep. 2019 Oct 22;9(1):15132. doi: 10.1038/s41598-019-51219-4. PMID: 31641162. - No appropriate outcomes [PMC free article: PMC6805937] [PubMed: 31641162] [CrossRef]
- 37.
- Falk GE, Rogers J, Lu L, et al. Sepsis, Septic Shock, and Differences in Cardiovascular Event Occurrence. J Intensive Care Med. 2022 Nov;37(11):1528–34. doi: 10.1177/08850666221083644. PMID: 35236176. - Intervention does not include a prediction or recognition component [PubMed: 35236176] [CrossRef]
- 38.
- Feinstein Y, Kogan S, Dreiher J, et al. Implementing a screening algorithm for early recognition of sepsis in hospitalized children: a quality improvement project. Int J Qual Health Care. 2023 Feb 22;35(1)doi: 10.1093/intqhc/mzad006. PMID: 36715271. - Non-USA based study or does not report data separately for USA [PubMed: 36715271] [CrossRef]
- 39.
- Ferguson A, Coates DE, Osborn S, et al. Early, Nurse-Directed Sepsis Care. Am J Nurs. 2019 Jan;119(1):52–8. doi: 10.1097/01.NAJ.0000552614.89028.d6. PMID: 30589710. - Intervention does not include a prediction or recognition component [PubMed: 30589710] [CrossRef]
- 40.
- Ferrer R, Martínez ML, Gomà G, et al. Improved empirical antibiotic treatment of sepsis after an educational intervention: the ABISS-Edusepsis study. Crit Care. 2018 Jun 22;22(1):167. doi: 10.1186/s13054-018-2091-0. PMID: 29933756. - Non-USA based study or does not report data separately for USA [PMC free article: PMC6013897] [PubMed: 29933756] [CrossRef]
- 41.
- Fesnak S, Abbadessa MK, Hayes K, et al. Sepsis in Complex Patients in the Emergency Department: Time to Recognition and Therapy in Pediatric Patients With High-Risk Conditions. Pediatr Emerg Care. 2020 Feb;36(2):63–5. doi: 10.1097/pec.0000000000002038. PMID: 31929394. - No appropriate outcomes [PubMed: 31929394] [CrossRef]
- 42.
- Fleuren LM, Klausch TLT, Zwager CL, et al. Machine learning for the prediction of sepsis: a systematic review and meta-analysis of diagnostic test accuracy. Intensive Care Med. 2020 Mar;46(3):383–400. doi: 10.1007/s00134-019-05872-y. PMID: 31965266. - No appropriate outcomes [PMC free article: PMC7067741] [PubMed: 31965266] [CrossRef]
- 43.
- Floer M, Ziegler M, Lenkewitz B, et al. Out-of-hospital sepsis recognition by paramedics improves the course of disease and mortality: A single center retrospective study. Adv Clin Exp Med. 2021 Nov;30(11):1115–25. doi: 10.17219/acem/140357. PMID: 34418337. - Non-USA based study or does not report data separately for USA [PubMed: 34418337] [CrossRef]
- 44.
- Forner J, Schupp T, Weidner K, et al. Cardiac Troponin I Reveals Diagnostic and Prognostic Superiority to Aminoterminal Pro-B-Type Natriuretic Peptide in Sepsis and Septic Shock. J Clin Med. 2022 Nov 7;11(21)doi: 10.3390/jcm11216592. PMID: 36362826. - No appropriate outcomes [PMC free article: PMC9658155] [PubMed: 36362826] [CrossRef]
- 45.
- Gale BM, Hall KK. The Use of Patient Monitoring Systems to Improve Sepsis Recognition and Outcomes: A Systematic Review. J Patient Saf. 2020 Sep;16(3S Suppl 1):S8–s11. doi: 10.1097/pts.0000000000000750. PMID: 32809995. - Other: Article based on Making Healthcare Safer III report [PMC free article: PMC7447166] [PubMed: 32809995] [CrossRef]
- 46.
- Gigli KH, Davis BS, Yabes JG, et al. Pediatric Outcomes After Regulatory Mandates for Sepsis Care. Pediatrics. 2020 Jul;146(1)doi: 10.1542/peds.2019-3353. PMID: 32605994. - Intervention does not include a prediction or recognition component [PMC free article: PMC7329251] [PubMed: 32605994] [CrossRef]
- 47.
- Gonçalves LS, Amaro MLM, Schamne FK, et al. Nursing Documentation and Activities when Using a Clinical Decision Support System for Sepsis and Clinical Deterioration Management. Stud Health Technol Inform. 2022 May 25;294:153–4. doi: 10.3233/shti220426. PMID: 35612046. - Non-USA based study or does not report data separately for USA [PubMed: 35612046] [CrossRef]
- 48.
- Grandbois van Ravenhorst C, Schluep M, Endeman H, et al. Prognostic models for outcome prediction following in-hospital cardiac arrest using pre-arrest factors: a systematic review, meta-analysis and critical appraisal. Crit Care. 2023 Jan 20;27(1):32. doi: 10.1186/s13054-023-04306-y. PMID: 36670450. - Not about sepsis [PMC free article: PMC9862512] [PubMed: 36670450] [CrossRef]
- 49.
- Gripp L, Raffoul M, Milner KA. Implementation of the Surviving Sepsis Campaign one-hour bundle in a short stay unit: A quality improvement project. Intensive Crit Care Nurs. 2021 Apr;63:103004. doi: 10.1016/j.iccn.2020.103004. PMID: 33358134. - Other: No comparison group [PubMed: 33358134] [CrossRef]
- 50.
- Guan G, Lee CMY, Begg S, et al. The use of early warning system scores in prehospital and emergency department settings to predict clinical deterioration: A systematic review and meta-analysis. PLoS One. 2022;17(3):e0265559. doi: 10.1371/journal.pone.0265559. PMID: 35298560. - No appropriate outcomes [PMC free article: PMC8929648] [PubMed: 35298560] [CrossRef]
- 51.
- Guirgis FW, Puskarich MA, Smotherman C, et al. Development of a Simple Sequential Organ Failure Assessment Score for Risk Assessment of Emergency Department Patients With Sepsis. J Intensive Care Med. 2020 Mar;35(3):270–8. doi: 10.1177/0885066617741284. PMID: 29141524. - Intervention does not include a prediction or recognition component [PMC free article: PMC5955789] [PubMed: 29141524] [CrossRef]
- 52.
- Guo S, Guo Z, Ren Q, et al. A prediction model for sepsis in infected patients: EASE (Early Assessment of Sepsis Engagement). Shock. 2023 Jul 12doi: 10.1097/shk.0000000000002170. PMID: 37477387. - Non-USA based study or does not report data separately for USA [PMC free article: PMC10476592] [PubMed: 37477387] [CrossRef]
- 53.
- Hamilton F, Arnold D, Baird A, et al. Early Warning Scores do not accurately predict mortality in sepsis: A meta-analysis and systematic review of the literature. J Infect. 2018 Mar;76(3):241–8. doi: 10.1016/j.jinf.2018.01.002. PMID: 29337035. - No appropriate outcomes [PubMed: 29337035] [CrossRef]
- 54.
- Han X, Dou Q, Zhu Y, et al. Heparin-binding protein-enhanced quick SOFA score improves mortality prediction in sepsis patients. Front Med (Lausanne). 2022;9:926798. doi: 10.3389/fmed.2022.926798. PMID: 36035420. - Non-USA based study or does not report data separately for USA [PMC free article: PMC9402998] [PubMed: 36035420] [CrossRef]
- 55.
- Harpenau TL, Bhatti SN, Hoffman BM, et al. Impact of extended emergency department stay on antibiotic re-dosing delays and outcomes in sepsis. Am J Emerg Med. 2022 May;55:32–7. doi: 10.1016/j.ajem.2022.02.028. PMID: 35255455. - No intervention [PubMed: 35255455] [CrossRef]
- 56.
- Hashem HE, El Masry SA, Mokhtar AM, et al. Valuable Role of Neutrophil CD64 and Highly Sensitive CRP Biomarkers for Diagnostic, Monitoring, and Prognostic Evaluations of Sepsis Patients in Neonatal ICUs. Biomed Res Int. 2020;2020:6214363. doi: 10.1155/2020/6214363. PMID: 32832553. - No appropriate outcomes [PMC free article: PMC7429763] [PubMed: 32832553] [CrossRef]
- 57.
- Hassan N, Slight R, Weiand D, et al. Preventing sepsis; how can artificial intelligence inform the clinical decision-making process? A systematic review. Int J Med Inform. 2021 Jun;150:104457. doi: 10.1016/j.ijmedinf.2021.104457. PMID: 33878596. - No appropriate outcomes [PubMed: 33878596] [CrossRef]
- 58.
- Henry KE, Adams R, Parent C, et al. Factors driving provider adoption of the TREWS machine learning-based early warning system and its effects on sepsis treatment timing. Nat Med. 2022 Jul;28(7):1447–54. doi: 10.1038/s41591-022-01895-z. PMID: 35864251. - Other: No comparison group [PubMed: 35864251] [CrossRef]
- 59.
- Herd SH, Allen PL, Reed LJ, et al. Time to embrace sepsis pathways and antibiotic prescribing decision support in the emergency department: Observations from a retrospective single site clinical audit. Emerg Med Australas. 2023 Apr 11doi: 10.1111/1742-6723.14206. PMID: 37038917. - Non-USA based study or does not report data separately for USA [PubMed: 37038917] [CrossRef]
- 60.
- Herrán-Monge R, Muriel-Bombín A, García-García MM, et al. Epidemiology and Changes in Mortality of Sepsis After the Implementation of Surviving Sepsis Campaign Guidelines. J Intensive Care Med. 2019 Sep;34(9):740–50. doi: 10.1177/0885066617711882. PMID: 28651474. - Intervention does not include a prediction or recognition component [PubMed: 28651474] [CrossRef]
- 61.
- Hester J, Youn TS, Trifilio E, et al. The Modified Early Warning Score: A Useful Marker of Neurological Worsening but Unreliable Predictor of Sepsis in the Neurocritically Ill-A Retrospective Cohort Study. Crit Care Explor. 2021 May;3(5):e0386. doi: 10.1097/cce.0000000000000386. PMID: 34036267. - Intervention does not include a prediction or recognition component [PMC free article: PMC8133042] [PubMed: 34036267] [CrossRef]
- 62.
- Hollenbeak CS, Henning DJ, Geeting GK, et al. Costs and Consequences of a Novel Emergency Department Sepsis Diagnostic Test: The IntelliSep Index. Crit Care Explor. 2023 Jul;5(7):e0942. doi: 10.1097/cce.0000000000000942. PMID: 37465702. - No appropriate outcomes [PMC free article: PMC10351935] [PubMed: 37465702] [CrossRef]
- 63.
- Hu JR, Yo CH, Lee HY, et al. Risk-standardized sepsis mortality map of the United States. Digit Health. 2022 Jan–Dec;8:20552076211072400. doi: 10.1177/20552076211072400. PMID: 35096409. - Intervention does not include a prediction or recognition component [PMC free article: PMC8796072] [PubMed: 35096409] [CrossRef]
- 64.
- Hu W, Chen H, Wang H, et al. Identifying high-risk phenotypes and associated harms of delayed time-to-antibiotics in patients with ICU onset sepsis: A retrospective cohort study. J Crit Care. 2023 Apr;74:154221. doi: 10.1016/j.jcrc.2022.154221. PMID: 36565649. - Intervention does not include a prediction or recognition component [PubMed: 36565649] [CrossRef]
- 65.
- Hunter CL, Silvestri S, Stone A, et al. Prehospital sepsis alert notification decreases time to initiation of CMS sepsis core measures. Am J Emerg Med. 2019 Jan;37(1):114–7. doi: 10.1016/j.ajem.2018.09.034. PMID: 30269999. - Intervention does not include a prediction or recognition component [PubMed: 30269999] [CrossRef]
- 66.
- Islam MM, Nasrin T, Walther BA, et al. Prediction of sepsis patients using machine learning approach: A meta-analysis. Comput Methods Programs Biomed. 2019 Mar;170:1–9. doi: 10.1016/j.cmpb.2018.12.027. PMID: 30712598. - No appropriate outcomes [PubMed: 30712598] [CrossRef]
- 67.
- Jayasundera R, Neilly M, Smith TO, et al. Are Early Warning Scores Useful Predictors for Mortality and Morbidity in Hospitalised Acutely Unwell Older Patients? A Systematic Review. J Clin Med. 2018 Sep 28;7(10)doi: 10.3390/jcm7100309. PMID: 30274205. - No intervention [PMC free article: PMC6210896] [PubMed: 30274205] [CrossRef]
- 68.
- Jiang S, Zhang W, Lu Y. Development and validation of novel inflammatory response-related gene signature for sepsis prognosis. J Zhejiang Univ Sci B. 2022 Dec 15;23(12):1028–41. doi: 10.1631/jzus.B2200285. PMID: 36518055. - Intervention does not include a prediction or recognition component [PMC free article: PMC9758714] [PubMed: 36518055] [CrossRef]
- 69.
- Jones J, Allen S, Davies J, et al. Randomised feasibility study of prehospital recognition and antibiotics for emergency patients with sepsis (PhRASe). Sci Rep. 2021 Sep 20;11(1):18586. doi: 10.1038/s41598-021-97979-w. PMID: 34545117. - Non-USA based study or does not report data separately for USA [PMC free article: PMC8452688] [PubMed: 34545117] [CrossRef]
- 70.
- Karakike E, Kyriazopoulou E, Tsangaris I, et al. The early change of SOFA score as a prognostic marker of 28-day sepsis mortality: analysis through a derivation and a validation cohort. Crit Care. 2019 Nov 29;23(1):387. doi: 10.1186/s13054-019-2665-5. PMID: 31783881. - Non-USA based study or does not report data separately for USA [PMC free article: PMC6884794] [PubMed: 31783881] [CrossRef]
- 71.
- Kerns EK, Staggs VS, Fouquet SD, et al. Estimating the impact of deploying an electronic clinical decision support tool as part of a national practice improvement project. J Am Med Inform Assoc. 2019 Jul 1;26(7):630–6. doi: 10.1093/jamia/ocz011. PMID: 30925592. - Not about sepsis [PMC free article: PMC6562155] [PubMed: 30925592] [CrossRef]
- 72.
- Keul R, Rodriguez J, Coleman A, et al. A Quality Improvement Project Focused on Improving First Dose Antibiotic Timeliness for Pediatric Intensive Care Unit Patients with Severe Sepsis. Pediatr Qual Saf. 2020 Jan–Feb;5(1):e250. doi: 10.1097/pq9.0000000000000250. PMID: 32190795. - Not about sepsis [PMC free article: PMC7056285] [PubMed: 32190795] [CrossRef]
- 73.
- Khojandi A, Tansakul V, Li X, et al. Prediction of Sepsis and In-Hospital Mortality Using Electronic Health Records. Methods Inf Med. 2018 Sep;57(4):185–93. doi: 10.3414/me18-01-0014. PMID: 30248708. - No intervention [PubMed: 30248708] [CrossRef]
- 74.
- Kondo Y, Umemura Y, Hayashida K, et al. Diagnostic value of procalcitonin and presepsin for sepsis in critically ill adult patients: a systematic review and meta-analysis. J Intensive Care. 2019;7:22. doi: 10.1186/s40560-019-0374-4. PMID: 31016020. - No appropriate outcomes [PMC free article: PMC6466719] [PubMed: 31016020] [CrossRef]
- 75.
- Kong L, Wu Q, Liu B. The impact of selenium administration on severe sepsis or septic shock: a meta-analysis of randomized controlled trials. Afr Health Sci. 2021 Mar;21(1):277–85. doi: 10.4314/ahs.v21i1.36. PMID: 34394308. - Non-USA based study or does not report data separately for USA [PMC free article: PMC8356584] [PubMed: 34394308] [CrossRef]
- 76.
- Koppens HJ, Onland W, Visser DH, et al. Heart Rate Characteristics Monitoring for Late-Onset Sepsis in Preterm Infants: A Systematic Review. Neonatology. 2023 Jun 28:1–10. doi: 10.1159/000531118. PMID: 37379804. - Non-USA based study or does not report data separately for USA [PMC free article: PMC10614451] [PubMed: 37379804] [CrossRef]
- 77.
- Kumar A, Abbenbroek B, Delaney A, et al. Sepsis triggers and tools to support early identification in healthcare settings: An integrative review. Aust Crit Care. 2023 Feb 20doi: 10.1016/j.aucc.2023.01.001. PMID: 36813654. - No appropriate outcomes [PubMed: 36813654] [CrossRef]
- 78.
- Kyriazopoulou E, Poulakou G, Giamarellos-Bourboulis EJ. Biomarkers in sepsis: can they help improve patient outcome? Curr Opin Infect Dis. 2021 Apr 1;34(2):126–34. doi: 10.1097/qco.0000000000000707. PMID: 33534419. - Narrative review [PubMed: 33534419] [CrossRef]
- 79.
- LaFave J, Levy D, Gekle R, et al. Incidence of Clostridium difficile Infection After Sepsis Protocol Antibiotics. West J Emerg Med. 2019 Oct 24;20(6):977–81. doi: 10.5811/westjem.2019.10.42070. PMID: 31738730. - Intervention does not include a prediction or recognition component [PMC free article: PMC6860382] [PubMed: 31738730] [CrossRef]
- 80.
- Lafon T, Baisse A, Karam HH, et al. Sepsis Unit in the Emergency Department: impact on management and outcome of septic patients. Shock. 2023 Jun 15doi: 10.1097/shk.0000000000002155. PMID: 37314202. - Non-USA based study or does not report data separately for USA [PubMed: 37314202] [CrossRef]
- 81.
- Lauritsen SM, Kalør ME, Kongsgaard EL, et al. Early detection of sepsis utilizing deep learning on electronic health record event sequences. Artif Intell Med. 2020 Apr;104:101820. doi: 10.1016/j.artmed.2020.101820. PMID: 32498999. - Non-USA based study or does not report data separately for USA [PubMed: 32498999] [CrossRef]
- 82.
- Lauritsen SM, Kristensen M, Olsen MV, et al. Explainable artificial intelligence model to predict acute critical illness from electronic health records. Nat Commun. 2020 Jul 31;11(1):3852. doi: 10.1038/s41467-020-17431-x. PMID: 32737308. - Non-USA based study or does not report data separately for USA [PMC free article: PMC7395744] [PubMed: 32737308] [CrossRef]
- 83.
- Lauritsen SM, Thiesson B, Jørgensen MJ, et al. The Framing of machine learning risk prediction models illustrated by evaluation of sepsis in general wards. NPJ Digit Med. 2021 Nov 15;4(1):158. doi: 10.1038/s41746-021-00529-x. PMID: 34782696. - Non-USA based study or does not report data separately for USA [PMC free article: PMC8593052] [PubMed: 34782696] [CrossRef]
- 84.
- Laux L, Campbell T, Latouf KM, et al. Emergency Department Initiative to Improve Sepsis Core Measure Compliance: A Hospital Network Approach. Crit Care Nurs Q. 2022 Jan–Mar 01;45(1):25–34. doi: 10.1097/cnq.0000000000000385. PMID: 34818295. - Qualitative studies without quantitative data [PubMed: 34818295] [CrossRef]
- 85.
- Lee AH, Aaronson E, Hibbert KA, et al. Design and Implementation of a Real-time Monitoring Platform for Optimal Sepsis Care in an Emergency Department: Observational Cohort Study. J Med Internet Res. 2021 Jun 24;23(6):e26946. doi: 10.2196/26946. PMID: 34185009. - Other: No comparison group [PMC free article: PMC8277370] [PubMed: 34185009] [CrossRef]
- 86.
- Lee TC, Shah NU, Haack A, et al. Clinical Implementation of Predictive Models Embedded within Electronic Health Record Systems: A Systematic Review. Informatics (MDPI). 2020 Sep;7(3)doi: 10.3390/informatics7030025. PMID: 33274178. - No appropriate outcomes [PMC free article: PMC7710328] [PubMed: 33274178] [CrossRef]
- 87.
- Levin N, Horton D, Sanford M, et al. Failure of vital sign normalization is more strongly associated than single measures with mortality and outcomes. Am J Emerg Med. 2020 Dec;38(12):2516–23. doi: 10.1016/j.ajem.2019.12.024. PMID: 31864869. - Intervention does not include a prediction or recognition component [PMC free article: PMC6938586] [PubMed: 31864869] [CrossRef]
- 88.
- Li P, Wang C, Pang S. The diagnostic accuracy of mid-regional pro-adrenomedullin for sepsis: a systematic review and meta-analysis. Minerva Anestesiol. 2021 Oct;87(10):1117–27. doi: 10.23736/s0375-9393.21.15585-3. PMID: 34134460. - No appropriate outcomes [PubMed: 34134460] [CrossRef]
- 89.
- Liu R, Greenstein JL, Fackler JC, et al. Prediction of Impending Septic Shock in Children With Sepsis. Crit Care Explor. 2021 Jun;3(6):e0442. doi: 10.1097/cce.0000000000000442. PMID: 34151278. - Intervention does not include a prediction or recognition component [PMC free article: PMC8208392] [PubMed: 34151278] [CrossRef]
- 90.
- Loritz M, Busch HJ, Helbing T, et al. Prospective evaluation of the quickSOFA score as a screening for sepsis in the emergency department. Intern Emerg Med. 2020 Jun;15(4):685–93. doi: 10.1007/s11739-019-02258-2. PMID: 32036543. - No appropriate outcomes [PubMed: 32036543] [CrossRef]
- 91.
- Loughlin L, Knowles S, Twomey A, et al. The Neonatal Early Onset Sepsis Calculator; in Clinical Practice. Ir Med J. 2020 Apr 3;113(4):57. PMID: 32268050. - Non-USA based study or does not report data separately for USA [PubMed: 32268050]
- 92.
- Lu Y, Zhang H, Teng F, et al. Early Goal-Directed Therapy in Severe Sepsis and Septic Shock: A Meta-Analysis and Trial Sequential Analysis of Randomized Controlled Trials. J Intensive Care Med. 2018 May;33(5):296–309. doi: 10.1177/0885066616671710. PMID: 27756870. - Intervention does not include a prediction or recognition component [PubMed: 27756870] [CrossRef]
- 93.
- Maciver M. Pre-hospital use of early warning scores to improve detection and outcomes of sepsis. Br J Community Nurs. 2021 Mar 2;26(3):122–9. doi: 10.12968/bjcn.2021.26.3.122. PMID: 33719557. - No appropriate outcomes [PubMed: 33719557] [CrossRef]
- 94.
- Malinovska A, Hinson JS, Badaki-Makun O, et al. Monocyte distribution width as part of a broad pragmatic sepsis screen in the emergency department. J Am Coll Emerg Physicians Open. 2022 Apr;3(2):e12679. doi: 10.1002/emp2.12679. PMID: 35252973. - No appropriate outcomes [PMC free article: PMC8886187] [PubMed: 35252973] [CrossRef]
- 95.
- Medic G, Kosaner Kließ M, Atallah L, et al. Evidence-based Clinical Decision Support Systems for the prediction and detection of three disease states in critical care: A systematic literature review. F1000Res. 2019;8:1728. doi: 10.12688/f1000research.20498.2. PMID: 31824670. - No appropriate outcomes [PMC free article: PMC6894361] [PubMed: 31824670] [CrossRef]
- 96.
- Michelson KA, Bachur RG, Cruz AT, et al. Multicenter evaluation of a method to identify delayed diagnosis of diabetic ketoacidosis and sepsis in administrative data. Diagnosis (Berl). 2023 Jun 22doi: 10.1515/dx-2023-0019. PMID: 37340621. - Intervention does not include a prediction or recognition component [PMC free article: PMC10679849] [PubMed: 37340621] [CrossRef]
- 97.
- Misra D, Avula V, Wolk DM, et al. Early Detection of Septic Shock Onset Using Interpretable Machine Learners. J Clin Med. 2021 Jan 15;10(2)doi: 10.3390/jcm10020301. PMID: 33467539. - No appropriate outcomes [PMC free article: PMC7830968] [PubMed: 33467539] [CrossRef]
- 98.
- Moor M, Rieck B, Horn M, et al. Early Prediction of Sepsis in the ICU Using Machine Learning: A Systematic Review. Front Med (Lausanne). 2021;8:607952. doi: 10.3389/fmed.2021.607952. PMID: 34124082. - No appropriate outcomes [PMC free article: PMC8193357] [PubMed: 34124082] [CrossRef]
- 99.
- Moorthy GS, Pung JS, Subramanian N, et al. Causal Association of Physician-in-Triage with Improved Pediatric Sepsis Care: A Single-Center, Emergency Department Experience. Pediatr Qual Saf. 2023 May–Jun;8(3):e651. doi: 10.1097/pq9.0000000000000651. PMID: 37250616. - Intervention does not include a prediction or recognition component [PMC free article: PMC10219727] [PubMed: 37250616] [CrossRef]
- 100.
- Niemantsverdriet MSA, Varkila MRJ, Vromen-Wijsman JLP, et al. Transportability and Implementation Challenges of Early Warning Scores for Septic Shock in the ICU: A Perspective on the TREWScore. Front Med (Lausanne). 2021;8:793815. doi: 10.3389/fmed.2021.793815. PMID: 35211485. - No appropriate outcomes [PMC free article: PMC8860834] [PubMed: 35211485] [CrossRef]
- 101.
- Nomura O, Ihara T, Morikawa Y, et al. Predictor of Early Administration of Antibiotics and a Volume Resuscitation for Young Infants with Septic Shock. Antibiotics (Basel). 2021 Nov 19;10(11)doi: 10.3390/antibiotics10111414. PMID: 34827352. - Non-USA based study or does not report data separately for USA [PMC free article: PMC8615069] [PubMed: 34827352] [CrossRef]
- 102.
- Nuss KE, Kunar JS, Ahrens EA. Plan-Do-Study-Act Methodology: Refining an Inpatient Pediatric Sepsis Screening Process. Pediatr Qual Saf. 2020 Sep–Oct;5(5):e338. doi: 10.1097/pq9.0000000000000338. PMID: 33062902. - No appropriate outcomes [PMC free article: PMC7470006] [PubMed: 33062902] [CrossRef]
- 103.
- Patnaik R, Azim A, Agarwal V. Neutrophil CD64 a Diagnostic and Prognostic Marker of Sepsis in Adult Critically Ill Patients: A Brief Review. Indian J Crit Care Med. 2020 Dec;24(12):1242–50. doi: 10.5005/jp-journals-10071-23558. PMID: 33446980. - No appropriate outcomes [PMC free article: PMC7775945] [PubMed: 33446980] [CrossRef]
- 104.
- Pepper DJ, Jaswal D, Sun J, et al. Evidence Underpinning the Centers for Medicare & Medicaid Services’ Severe Sepsis and Septic Shock Management Bundle (SEP-1): A Systematic Review. Ann Intern Med. 2018 Apr 17;168(8):558–68. doi: 10.7326/m17-2947. PMID: 29459977. - Intervention does not include a prediction or recognition component [PubMed: 29459977] [CrossRef]
- 105.
- Porter TK, Turner KM, McMillian-Bohler J, et al. Improving Care of Skilled Nursing Patients: Implementation of Early Sepsis Recognition. J Gerontol Nurs. 2021 Aug;47(8):37–44. doi: 10.3928/00989134-20210624-02. PMID: 34309448. - Intervention does not include a prediction or recognition component [PubMed: 34309448] [CrossRef]
- 106.
- Pouryahya P, Guiney N, Meyer A, et al. Evaluating the implementation and outcomes of a sepsis pathway in the emergency department. N Z Med J. 2020 Aug 21;133(1520):35–49. PMID: 32994592. - Non-USA based study or does not report data separately for USA [PubMed: 32994592]
- 107.
- Prasad PA, Fang MC, Abe-Jones Y, et al. Time to Recognition of Sepsis in the Emergency Department Using Electronic Health Record Data: A Comparative Analysis of Systemic Inflammatory Response Syndrome, Sequential Organ Failure Assessment, and Quick Sequential Organ Failure Assessment. Crit Care Med. 2020 Feb;48(2):200–9. doi: 10.1097/ccm.0000000000004132. PMID: 31939788. - Intervention does not include a prediction or recognition component [PMC free article: PMC7494056] [PubMed: 31939788] [CrossRef]
- 108.
- Proffitt RD, Hooper G. Evaluation of the (qSOFA) Tool in the Emergency Department Setting: Nurse Perception and the Impact on Patient Care. Adv Emerg Nurs J. 2020 Jan/Mar;42(1):54–62. doi: 10.1097/tme.0000000000000281. PMID: 32000191. - No appropriate outcomes [PubMed: 32000191] [CrossRef]
- 109.
- Pullyblank A, Tavaré A, Little H, et al. Implementation of the National Early Warning Score in patients with suspicion of sepsis: evaluation of a system-wide quality improvement project. Br J Gen Pract. 2020 Jun;70(695):e381–e8. doi: 10.3399/bjgp20X709349. PMID: 32269043. - Non-USA based study or does not report data separately for USA [PMC free article: PMC7147668] [PubMed: 32269043] [CrossRef]
- 110.
- Qiu X, Lei YP, Zhou RX. SIRS, SOFA, qSOFA, and NEWS in the diagnosis of sepsis and prediction of adverse outcomes: a systematic review and meta-analysis. Expert Rev Anti Infect Ther. 2023 Jul–Dec;21(8):891–900. doi: 10.1080/14787210.2023.2237192. PMID: 37450490. - No appropriate outcomes [PubMed: 37450490] [CrossRef]
- 111.
- Rafiei A, Rezaee A, Hajati F, et al. SSP: Early prediction of sepsis using fully connected LSTM-CNN model. Comput Biol Med. 2021 Jan;128:104110. doi: 10.1016/j.compbiomed.2020.104110. PMID: 33227577. - Non-USA based study or does not report data separately for USA [PubMed: 33227577] [CrossRef]
- 112.
- Raman J, Johnson TJ, Hayes K, et al. Racial Differences in Sepsis Recognition in the Emergency Department. Pediatrics. 2019 Oct;144(4)doi: 10.1542/peds.2019-0348. PMID: 31519793. - No intervention [PubMed: 31519793] [CrossRef]
- 113.
- Rhee C, Wang R, Song Y, et al. Risk adjustment for sepsis mortality to facilitate hospital comparisons using routine EHR data. Critical care medicine. 2019;47(1) PMID: CN-01998595. - Conference, meeting abstract, or poster [PMC free article: PMC7063887] [PubMed: 32166230]
- 114.
- Robert Boter N, Steinherr Zazo A, Rocamora Blanch G, et al. Sepsis Code in emergency department: Performance of q-SOFA score compared to SIRS score. Med Clin (Barc). 2022 Mar 25;158(6):260–4. doi: 10.1016/j.medcli.2021.03.039. PMID: 34256935. - Non-USA based study or does not report data separately for USA [PubMed: 34256935] [CrossRef]
- 115.
- Rodrigues-Santos G, de Magalhães-Barbosa MC, Raymundo CE, et al. Improvement of 1st-hour bundle compliance and sepsis mortality in pediatrics after the implementation of the surviving sepsis campaign guidelines. J Pediatr (Rio J). 2021 Jul–Aug;97(4):459–67. doi: 10.1016/j.jped.2020.09.005. PMID: 33121929. - Non-USA based study or does not report data separately for USA [PMC free article: PMC9432151] [PubMed: 33121929] [CrossRef]
- 116.
- Rodríguez A, Mendoza D, Ascuntar J, et al. Supervised classification techniques for prediction of mortality in adult patients with sepsis. Am J Emerg Med. 2021 Jul;45:392–7. doi: 10.1016/j.ajem.2020.09.013. PMID: 33036848. - Non-USA based study or does not report data separately for USA [PubMed: 33036848] [CrossRef]
- 117.
- Rogers P, Boussina AE, Shashikumar SP, et al. Optimizing the Implementation of Clinical Predictive Models to Minimize National Costs: Sepsis Case Study. J Med Internet Res. 2023 Feb 13;25:e43486. doi: 10.2196/43486. PMID: 36780203. - Other: This is theoretical study with modeling to estimate cost saving based on different changes to hypothetical sepsis prediction algorithms. [PMC free article: PMC9972209] [PubMed: 36780203] [CrossRef]
- 118.
- Roman CP, Dooley M, Nevill A, et al. Introduction of an emergency medicine pharmacist-led sepsis alert response system in the emergency department: A cohort study. Emerg Med Australas. 2023 Aug;35(4):564–71. doi: 10.1111/1742-6723.14168. PMID: 36634917. - Non-USA based study or does not report data separately for USA [PubMed: 36634917] [CrossRef]
- 119.
- Rosenqvist M, Bengtsson-Toni M, Tham J, et al. Improved Outcomes After Regional Implementation of Sepsis Alert: A Novel Triage Model. Crit Care Med. 2020 Apr;48(4):484–90. doi: 10.1097/ccm.0000000000004179. PMID: 32205594. - Non-USA based study or does not report data separately for USA [PubMed: 32205594] [CrossRef]
- 120.
- Rotter J, Dowlati E, Cobourn K, et al. A cross-sectional analysis of variables associated with morbidity and mortality in postoperative neurosurgical patients diagnosed with sepsis. Acta Neurochir (Wien). 2020 Nov;162(11):2837–48. doi: 10.1007/s00701-020-04586-1. PMID: 32959343. - Intervention does not include a prediction or recognition component [PubMed: 32959343] [CrossRef]
- 121.
- Sahu P, Raj Stanly EA, Simon Lewis LE, et al. Prediction modelling in the early detection of neonatal sepsis. World J Pediatr. 2022 Mar;18(3):160–75. doi: 10.1007/s12519-021-00505-1. PMID: 34984642. - No appropriate outcomes [PMC free article: PMC8898244] [PubMed: 34984642] [CrossRef]
- 122.
- Samsudin MI, Liu N, Prabhakar SM, et al. A novel heart rate variability based risk prediction model for septic patients presenting to the emergency department. Medicine (Baltimore). 2018 Jun;97(23):e10866. doi: 10.1097/md.0000000000010866. PMID: 29879021. - Intervention does not include a prediction or recognition component [PMC free article: PMC5999455] [PubMed: 29879021] [CrossRef]
- 123.
- Scherer JS, Pereira JS, Debastiani MS, et al. Beyond technology: Can artificial intelligence support clinical decisions in the prediction of sepsis? Rev Bras Enferm. 2022;75(5):e20210586. doi: 10.1590/0034-7167-2021-0586. PMID: 35584427. - Non-USA based study or does not report data separately for USA [PubMed: 35584427] [CrossRef]
- 124.
- Schinkel M, Paranjape K, Nannan Panday RS, et al. Clinical applications of artificial intelligence in sepsis: A narrative review. Comput Biol Med. 2019 Dec;115:103488. doi: 10.1016/j.compbiomed.2019.103488. PMID: 31634699. - No appropriate outcomes [PubMed: 31634699] [CrossRef]
- 125.
- Schneider JE, Dick K, Cooper JT, et al. Pancreatic stone protein point-of-care testing can reduce healthcare expenditure in sepsis. Health Econ Rev. 2022 Jul 22;12(1):39. doi: 10.1186/s13561-022-00381-z. PMID: 35867213. - No appropriate outcomes [PMC free article: PMC9306195] [PubMed: 35867213] [CrossRef]
- 126.
- Schwarzkopf D, Matthaeus-Kraemer CT, Thomas-Rüddel DO, et al. A multifaceted educational intervention improved anti-infectious measures but had no effect on mortality in patients with severe sepsis. Sci Rep. 2022 Mar 10;12(1):3925. doi: 10.1038/s41598-022-07915-9. PMID: 35273276. - Non-USA based study or does not report data separately for USA [PMC free article: PMC8913650] [PubMed: 35273276] [CrossRef]
- 127.
- Sen P, Demirdal T, Nemli SA, et al. Diagnostic and prognostic value of new bioscore in critically ill septic patients. Arch Physiol Biochem. 2022 Apr;128(2):300–5. doi: 10.1080/13813455.2019.1677720. PMID: 31687850. - Intervention does not include a prediction or recognition component [PubMed: 31687850] [CrossRef]
- 128.
- Sendak MP, Ratliff W, Sarro D, et al. Real-World Integration of a Sepsis Deep Learning Technology Into Routine Clinical Care: Implementation Study. JMIR Med Inform. 2020 Jul 15;8(7):e15182. doi: 10.2196/15182. PMID: 32673244. - No appropriate outcomes [PMC free article: PMC7391165] [PubMed: 32673244] [CrossRef]
- 129.
- Serna E, Parra-Llorca A, Panadero J, et al. miRNomic Signature in Very Low Birth-Weight Neonates Discriminates Late-Onset Gram-Positive Sepsis from Controls. Diagnostics (Basel). 2021 Jul 31;11(8)doi: 10.3390/diagnostics11081389. PMID: 34441323. - Non-USA based study or does not report data separately for USA [PMC free article: PMC8391178] [PubMed: 34441323] [CrossRef]
- 130.
- Shashikumar SP, Wardi G, Malhotra A, et al. Artificial intelligence sepsis prediction algorithm learns to say “I don’t know”. NPJ Digit Med. 2021 Sep 9;4(1):134. doi: 10.1038/s41746-021-00504-6. PMID: 34504260. - No appropriate outcomes [PMC free article: PMC8429719] [PubMed: 34504260] [CrossRef]
- 131.
- Shojaee M, Safari S, Sabzghabaei A, et al. Pro-BNP versus MEDS Score in Determining the Prognosis of Sepsis Patients; a Diagnostic Accuracy Study. Emerg (Tehran). 2018;6(1):e4. PMID: 29503829. - Non-USA based study or does not report data separately for USA [PMC free article: PMC5827050] [PubMed: 29503829]
- 132.
- Shu E, Ives Tallman C, Frye W, et al. Pre-hospital qSOFA as a predictor of sepsis and mortality. Am J Emerg Med. 2019 Jul;37(7):1273–8. doi: 10.1016/j.ajem.2018.09.025. PMID: 30322666. - Intervention does not include a prediction or recognition component [PubMed: 30322666] [CrossRef]
- 133.
- Sloan SNB, Rodriguez N, Seward T, et al. Compliance with SEP-1 guidelines is associated with improved outcomes for septic shock but not for severe sepsis. J Intensive Med. 2022 Jul;2(3):167–72. doi: 10.1016/j.jointm.2022.03.003. PMID: 36789014. - Intervention does not include a prediction or recognition component [PMC free article: PMC9924005] [PubMed: 36789014] [CrossRef]
- 134.
- Smith V, Kenny LC, Sandall J, et al. Physiological track-and-trigger/early warning systems for use in maternity care. Cochrane Database Syst Rev. 2021 Sep 13;9(9):Cd013276. doi: 10.1002/14651858.CD013276.pub2. PMID: 34515991. - No appropriate outcomes [PMC free article: PMC8436732] [PubMed: 34515991] [CrossRef]
- 135.
- Soleimani H, Henry KE, Zhan A, et al. Early intervention to reduce need for mechanical ventilation in sepsis patients with respiratory failure in the emergency department. Diagnosis. 2018;5(4):eA70–eA1. doi: 10.1515/dx-2018-0095. PMID: CN-01788306. - Conference, meeting abstract, or poster [CrossRef]
- 136.
- Spoto S, Nobile E, Carnà EPR, et al. Best diagnostic accuracy of sepsis combining SIRS criteria or qSOFA score with Procalcitonin and Mid-Regional pro-Adrenomedullin outside ICU. Sci Rep. 2020 Oct 6;10(1):16605. doi: 10.1038/s41598-020-73676-y. PMID: 33024218. - Intervention does not include a prediction or recognition component [PMC free article: PMC7538435] [PubMed: 33024218] [CrossRef]
- 137.
- Sreeramoju P, Voy-Hatter K, White C, et al. Results and lessons from a hospital-wide initiative incentivised by delivery system reform to improve infection prevention and sepsis care. BMJ Open Qual. 2021 Feb;10(1)doi: 10.1136/bmjoq-2020-001189. PMID: 33547154. - Intervention does not include a prediction or recognition component [PMC free article: PMC7871234] [PubMed: 33547154] [CrossRef]
- 138.
- Stinson HR, Viteri S, Koetter P, et al. Early Experience with a Novel Strategy for Assessment of Sepsis Risk: The Shock Huddle. Pediatr Qual Saf. 2019 Jul–Aug;4(4):e197. doi: 10.1097/pq9.0000000000000197. PMID: 31572898. - Other: No comparison group [PMC free article: PMC6708645] [PubMed: 31572898] [CrossRef]
- 139.
- Sullivan BA, Hochheimer CJ, Chernyavskiy P, et al. Impact of race on heart rate characteristics monitoring in very low birth weight infants. Pediatr Res. 2023 Aug;94(2):575–80. doi: 10.1038/s41390-023-02470-z. PMID: 36650306. - No appropriate outcomes [PMC free article: PMC10350468] [PubMed: 36650306] [CrossRef]
- 140.
- Suttapanit K, Dangprasert K, Sanguanwit P, et al. The Ramathibodi early warning score as a sepsis screening tool does not reduce the timing of antibiotic administration. Int J Emerg Med. 2022 May 10;15(1):18. doi: 10.1186/s12245-022-00420-w. PMID: 35538415. - Non-USA based study or does not report data separately for USA [PMC free article: PMC9087922] [PubMed: 35538415] [CrossRef]
- 141.
- Taj M, Brenner M, Sulaiman Z, et al. Sepsis protocols to reduce mortality in resource-restricted settings: A systematic review. Intensive Crit Care Nurs. 2022 Oct;72:103255. doi: 10.1016/j.iccn.2022.103255. PMID: 35688752. - Non-USA based study or does not report data separately for USA [PubMed: 35688752] [CrossRef]
- 142.
- Tchou MJ, Andersen H, Robinette E, et al. Accelerating Initiation of Adequate Antimicrobial Therapy Using Real-Time Decision Support and Microarray Testing. Pediatr Qual Saf. 2019 Jul–Aug;4(4):e191. doi: 10.1097/pq9.0000000000000191. PMID: 31572892. - Intervention does not include a prediction or recognition component [PMC free article: PMC6708656] [PubMed: 31572892] [CrossRef]
- 143.
- Thodphetch M, Chenthanakij B, Wittayachamnankul B, et al. A comparison of scoring systems for predicting mortality and sepsis in the emergency department patients with a suspected infection. Clin Exp Emerg Med. 2021 Dec;8(4):289–95. doi: 10.15441/ceem.20.145. PMID: 35000356. - Non-USA based study or does not report data separately for USA [PMC free article: PMC8743683] [PubMed: 35000356] [CrossRef]
- 144.
- Tian R, Wang X, Pan T, et al. Plasma PTX3, MCP1 and Ang2 are early biomarkers to evaluate the severity of sepsis and septic shock. Scand J Immunol. 2019 Dec;90(6):e12823. doi: 10.1111/sji.12823. PMID: 31489646. - No appropriate outcomes [PMC free article: PMC6900011] [PubMed: 31489646] [CrossRef]
- 145.
- Topiwala R, Patel K, Twigg J, et al. Retrospective Observational Study of the Clinical Performance Characteristics of a Machine Learning Approach to Early Sepsis Identification. Crit Care Explor. 2019 Sep;1(9):e0046. doi: 10.1097/cce.0000000000000046. PMID: 32166288. - Intervention does not include a prediction or recognition component [PMC free article: PMC7063939] [PubMed: 32166288] [CrossRef]
- 146.
- Troncoso R, Jr., Garfinkel EM, Hinson JS, et al. Do prehospital sepsis alerts decrease time to complete CMS sepsis measures? Am J Emerg Med. 2023 Sep;71:81–5. doi: 10.1016/j.ajem.2023.06.024. PMID: 37354893. - Intervention does not include a prediction or recognition component [PubMed: 37354893] [CrossRef]
- 147.
- Tullo G, Candelli M, Gasparrini I, et al. Ultrasound in Sepsis and Septic Shock-From Diagnosis to Treatment. J Clin Med. 2023 Feb 2;12(3)doi: 10.3390/jcm12031185. PMID: 36769833. - Narrative review [PMC free article: PMC9918257] [PubMed: 36769833] [CrossRef]
- 148.
- Tuttle E, Wang X, Modrykamien A. Sepsis mortality and ICU length of stay after the implementation of an intensive care team in the emergency department. Intern Emerg Med. 2023 Apr 19:1–8. doi: 10.1007/s11739-023-03265-0. PMID: 37074499. - Intervention does not include a prediction or recognition component [PMC free article: PMC10113981] [PubMed: 37074499] [CrossRef]
- 149.
- Uffen JW, Oosterheert JJ, Schweitzer VA, et al. Interventions for rapid recognition and treatment of sepsis in the emergency department: a narrative review. Clin Microbiol Infect. 2021 Feb;27(2):192–203. doi: 10.1016/j.cmi.2020.02.022. PMID: 32120030. - Non-USA based study or does not report data separately for USA [PubMed: 32120030] [CrossRef]
- 150.
- Valik JK, Ward L, Tanushi H, et al. Predicting sepsis onset using a machine learned causal probabilistic network algorithm based on electronic health records data. Sci Rep. 2023 Jul 20;13(1):11760. doi: 10.1038/s41598-023-38858-4. PMID: 37474597. - Non-USA based study or does not report data separately for USA [PMC free article: PMC10359402] [PubMed: 37474597] [CrossRef]
- 151.
- Villegas N, Moore LJ. Sepsis Screening: Current Evidence and Available Tools. Surg Infect (Larchmt). 2018 Feb/Mar;19(2):126–30. doi: 10.1089/sur.2017.250. PMID: 29315023. - No appropriate outcomes [PubMed: 29315023] [CrossRef]
- 152.
- Wang W, Qiang Y, Tao Z, et al. Serum Cystatin C Level Monitoring for Intervention Opportunity of CBP in Children with Severe Sepsis. Evid Based Complement Alternat Med. 2022;2022:8571203. doi: 10.1155/2022/8571203. PMID: 35815274. - Other: Article retracted [PMC free article: PMC9259228] [PubMed: 35815274] [CrossRef]
- 153.
- Warstadt NM, Caldwell JR, Tang N, et al. Quality initiative to improve emergency department sepsis bundle compliance through utilisation of an electronic health record tool. BMJ Open Qual. 2022 Jan;11(1)doi: 10.1136/bmjoq-2021-001624. PMID: 34992053. - Intervention does not include a prediction or recognition component [PMC free article: PMC8739442] [PubMed: 34992053] [CrossRef]
- 154.
- Wayne MT, Seelye S, Molling D, et al. Temporal Trends and Hospital Variation in Time-to-Antibiotics Among Veterans Hospitalized With Sepsis. JAMA Netw Open. 2021 Sep 1;4(9):e2123950. doi: 10.1001/jamanetworkopen.2021.23950. PMID: 34491351. - Intervention does not include a prediction or recognition component [PMC free article: PMC8424480] [PubMed: 34491351] [CrossRef]
- 155.
- Westphal GA, Pereira AB, Fachin SM, et al. An electronic warning system helps reduce the time to diagnosis of sepsis. Rev Bras Ter Intensiva. 2018 Oct–Dec;30(4):414–22. doi: 10.5935/0103-507x.20180059. PMID: 30570029. - Non-USA based study or does not report data separately for USA [PMC free article: PMC6334482] [PubMed: 30570029] [CrossRef]
- 156.
- Wolf TA, Wimalawansa SJ, Razzaque MS. Procalcitonin as a biomarker for critically ill patients with sepsis: Effects of vitamin D supplementation. J Steroid Biochem Mol Biol. 2019 Oct;193:105428. doi: 10.1016/j.jsbmb.2019.105428. PMID: 31323346. - No original data [PubMed: 31323346] [CrossRef]
- 157.
- Wu M, Du X, Gu R, et al. Artificial Intelligence for Clinical Decision Support in Sepsis. Front Med (Lausanne). 2021;8:665464. doi: 10.3389/fmed.2021.665464. PMID: 34055839. - No appropriate outcomes [PMC free article: PMC8155362] [PubMed: 34055839] [CrossRef]
- 158.
- Yu SC, Gupta A, Betthauser KD, et al. Sepsis Prediction for the General Ward Setting. Front Digit Health. 2022;4:848599. doi: 10.3389/fdgth.2022.848599. PMID: 35350226. - Intervention does not include a prediction or recognition component [PMC free article: PMC8957791] [PubMed: 35350226] [CrossRef]
- 159.
- Yu Y, Wu W, Dong Y, et al. C-Reactive Protein-to-Albumin Ratio Predicts Sepsis and Prognosis in Patients with Severe Burn Injury. Mediators Inflamm. 2021;2021:6621101. doi: 10.1155/2021/6621101. PMID: 33833617. - Non-USA based study or does not report data separately for USA [PMC free article: PMC8016580] [PubMed: 33833617] [CrossRef]
- 160.
- Yun H, Park JH, Choi DH, et al. Enhancement in Performance of Septic Shock Prediction Using National Early Warning Score, Initial Triage Information, and Machine Learning Analysis. J Emerg Med. 2021 Jul;61(1):1–11. doi: 10.1016/j.jemermed.2021.01.038. PMID: 33812727. - No appropriate outcomes [PubMed: 33812727] [CrossRef]
- 161.
- Zheng X, Zhang Y, Lin S, et al. Diagnostic significance of microRNAs in sepsis. PLoS One. 2023;18(2):e0279726. doi: 10.1371/journal.pone.0279726. PMID: 36812225. - No appropriate outcomes [PMC free article: PMC9946237] [PubMed: 36812225] [CrossRef]
Appendix C. Evidence Tables
Evidence Table C-1. Included systematic review characteristics addressing effectiveness of sepsis prediction, recognition, and treatment (MS Word, 397K)
Evidence Table C-2. Included systematic review outcomes addressing effectiveness of sepsis prediction, recognition, and treatment (MS Word, 395K)
Evidence Table C-5. Study characteristics of included primary studies addressing effectiveness of sepsis prediction, recognition, and treatment (MS Word, 398K)
Evidence Table C-18. Pre-post studies addressing effectiveness of sepsis prediction, recognition, and treatment in an adult population (MS Word, 434K)
Evidence Table C-19. Strength of evidence for included primary studies (MS Word, 434K)
- Disclaimers: This report is based on research conducted by the Johns Hopkins University under contract to the Agency for Healthcare Research and Quality (AHRQ), Rockville, MD (Contract No. 75Q80120D00003). The findings and conclusions in this document are those of the authors, who are responsible for its contents; the findings and conclusions do not necessarily represent the views of AHRQ. Therefore, no statement in this report should be construed as an official position of AHRQ or of the U.S. Department of Health and Human Services.None of the investigators have any affiliations or financial involvement that conflicts with the material presented in this report.The information in this report is intended to help healthcare decision makers—patients and clinicians, health system leaders, and policymakers, among others—make well-informed decisions and thereby improve the quality of healthcare services. This report is not intended to be a substitute for the application of clinical judgment. Anyone who makes decisions concerning the provision of clinical care should consider this report in the same way as any medical reference and in conjunction with all other pertinent information, i.e., in the context of available resources and circumstances presented by individual patients.This report is made available to the public under the terms of a licensing agreement between the author and the Agency for Healthcare Research and Quality. Most AHRQ documents are publicly available to use for noncommercial purposes (research, clinical or patient education, quality improvement projects) in the United States and do not need specific permission to be reprinted and used unless they contain material that is copyrighted by others. Specific written permission is needed for commercial use (reprinting for sale, incorporation into software, incorporation into for-profit training courses) or for use outside of the United States. If organizational policies require permission to adapt or use these materials, AHRQ will provide such permission in writing.AHRQ or U.S. Department of Health and Human Services endorsement of any derivative products that may be developed from this report, such as clinical practice guidelines, other quality enhancement tools, or reimbursement or coverage policies, may not be stated or implied.A representative from AHRQ served as a Contracting Officer’s Representative and reviewed the contract deliverables for adherence to contract requirements and quality. AHRQ did not directly participate in the literature search, determination of study eligibility criteria, data analysis, interpretation of data, or preparation or drafting of this report.AHRQ appreciates appropriate acknowledgment and citation of its work. Suggested language for acknowledgment: This work was based on an evidence report, Patient Safety Practices Focused on Sepsis Prediction and Recognition, by the Evidence-based Practice Center Program at the Agency for Healthcare Research and Quality (AHRQ).
- Cherian J, Segal J, Sharma R, Zhang A, Bass E, Rosen M. Patient Safety Practices Focused on Sepsis Prediction and Recognition. (Prepared by the Johns Hopkins University Evidence-based Practice Center under Contract No. 75Q80120D00003). AHRQ Publication No. 23(24)-EHC019-13. Rockville, MD: Agency for Healthcare Research and Quality. April 2024. DOI: https://doi.org/10.23970/AHRQEPC_MHS4SEPSIS. Posted final reports are located on the Effective Health Care Program search page.
- Patient Safety Practices Focused on Sepsis Prediction and Recognition - Making H...Patient Safety Practices Focused on Sepsis Prediction and Recognition - Making Healthcare Safer IV
Your browsing activity is empty.
Activity recording is turned off.
See more...