This book is distributed under the terms of the Creative Commons Attribution-NonCommercial-NoDerivs License which permits noncommercial use and distribution provided the original author(s) and source are credited. (See https://creativecommons.org/licenses/by-nc-nd/4.0/
NCBI Bookshelf. A service of the National Library of Medicine, National Institutes of Health.
Structured Abstract
Background and Objectives:
Diabetic peripheral neuropathy (DPN) is a highly prevalent and costly complication of diabetes that is frequently underdiagnosed and undertreated in primary care settings. Our primary research goal was to facilitate automated monitoring of patient-reported symptoms and medication side effects using interactive voice response telephone calls that systematically fed information to providers via patients' electronic health records. We hypothesized that this technology-enabled communication feedback tool would improve patient quality of life by promoting clinically appropriate treatment changes.
Methods:
Using a cluster (physician level) randomized controlled trial design, we randomized 1834 primary care physicians to the treatment or control condition (usual care plus brief, noninteractive educational telephone message), screened 2203 patients newly treated for DPN symptoms by these providers and recruited 1270 patients (83% of eligible patients) to participate in the intervention. Our primary outcomes were quality of life (EuroQol 5-dimension questionnaire [EQ-5D]) and select DPN symptoms (ie, pain interference—level of functional interference due to pain, sleep disturbance, and lower extremity functioning), which we assessed via telephone interviews with patients approximately 2 to 4 weeks after they initiated DPN medication and 8 months following baseline assessment. Process outcomes included receipt of a therapeutically effective dose of therapy at 8 and 12 months posttreatment start and patients' assessment of the quality of communication with their provider during the prior 12 months. We used generalized estimating equations to evaluate patient-level changes in the primary outcomes, controlling for clustering of patients within physicians. We conducted sensitivity analyses to assess the robustness of our findings to missing data.
Results:
Among the 1270 enrolled patients, 1255 (99%) completed the baseline assessment and 1179 (93%) completed follow-up. We found no effect of the intervention on change in quality of life (EQ-5D = −0.002 [95% CI, −0.01 to 0.01], P = .623); symptoms (pain interference = 0.29 [95% CI, −0.75 to 1.34]; P = .579); sleep disruption (0.34 [95% CI, −0.18 to 0.86]; P = .196); lower extremity functioning (−0.08 [95% CI, −1.27 to 1.11]; P = .896); or depressive symptoms (−0.46 [95% CI, −1.24 to 0.32]). We also found no statistically significant changes in either of our process measures: receipt of a therapeutically effective dose (0.13 [95% CI, −0.11 to 0.38]; P = .289) or patient-provider communication (−0.45 [95% CI, −0.97 to 0.07]; P = .091). Intervention effectiveness did not vary by the degree of perceived shared decision-making. More than 70% of patients in the intervention group reported problems with treatment on the first call, most due to side effects.
Limitations:
Absence of a protocol for physician response to patient reports, possible inclusion of patients who may have been prescribed study medications for another condition, use of a communication measure that extended into the baseline period, and the short time frame of the study (ie, 8 months) limited our ability to observe gradual changes in medication dosing.
Conclusions:
Monitoring of patient-reported symptoms and side effects through automated telephone calls did not significantly improve quality of life, symptoms, dosing, or patient-provider communication among patients newly treated for DPN symptoms. Given the high rates of side effects related to DPN medications reported, automated monitoring may have reinforced more conservative dosing strategies among providers in an attempt to limit treatment-related harms. To be effective in the management of DPN symptoms, automated monitoring may first require accurate identification of those patients most likely to benefit from, or be harmed by, available medications.
Background
An estimated 15 million American adults with type 2 diabetes develop diabetic peripheral neuropathy (DPN),1 a condition that accounts for nearly a third of all diabetes-related health care costs.2 Health care costs for patients with DPN ($12 000 to $30 000 per patient per year) are 2 to 4 times higher than costs for diabetes patients without this complication.1 Total estimated health care costs associated with DPN in the United States are $73.5 billion per year. Untreated DPN symptoms have a dramatic negative impact on patient quality of life, health status, and functioning.3 Although DPN is not curable, progression can be slowed through improved glycemic control, and long-term impact may be reduced through effective treatment of symptoms.4,5 Yet, there is growing evidence of undertreatment of DPN symptoms in primary care settings: Average adherence (proportion of days covered) to DPN treatment is less than 50%, and fewer than 40% of patients receive a therapeutically effective dose of the most common treatment (ie, gabapentin).6,7
Clinical guidelines recommend starting patients on select tricyclics (ie, duloxetine, amitriptyline, nortriptyline) or anticonvulsants (ie, gabapentin, pregabalin) and gradually increasing the dose over time or adding other medications in order to balance symptom relief and side effects.1,8-25 When used appropriately, these treatments have proved clinically effective in reducing pain symptoms by as much as 50%. Other treatments are also available (eg, tricyclic antidepressants: desipramine, imipramine; second-generation antidepressants: citalopram, paroxetine, venlafaxine; other convulsants: valproic acid; opioid analgesics), but evidence is insufficient to support their use as first-line treatments.8,9,25 Finding the right treatment for the right patient can be challenging due to a high degree of variability in patient response to therapy. In addition, side effects, such as nausea, somnolence, and dizziness, are quite common and can be either temporary or long lasting. Ongoing patient-provider communication about symptoms and treatment side effects is critical to optimize dose escalation and medication changes in order to maximize symptom relief.4,7,25
Prior studies suggest that patient factors such as low health literacy, language barriers, and reluctance to discuss pain symptoms,26 as well as limited time during routine primary care27-29 visits, can impede ongoing communication about DPN symptoms and treatment side effects.
In a recent pilot study, we interviewed 42 patients with DPN across 3 health systems about their experiences with DPN symptoms and medications to treat them. Gaps in patient-provider communication emerged as a central theme in these interviews. Patients taking medications to treat painful DPN often failed to tell their physician when a medication was not working. In addition, few recalled their physician following up with them to assess the medication's efficacy or side effects.
Automating the process of collecting information from patients about symptoms and side effects has the potential to bridge these important gaps in patient-provider communication. In particular, using phone-based interactive voice response (IVR) technology to collect patient-reported data between visits has been shown to be effective in facilitating shared decision-making and improving communication, quality of care, and other key outcomes in diabetes care.30-40 In our pilot study, most patients endorsed the idea of reporting symptoms to their primary care physician between visits via automated phone calls. However, such methods have yet to be formally tested to improve management of DPN symptoms and subsequent outcomes in primary care settings.
This study tested the hypothesis that providing prescribing physicians with automated, systematically collected data from patients on their experiences with newly started DPN medications could improve patient quality of life and symptom management by stimulating patient-provider communication, as well as treatment changes in response to patient reports. To the best of our knowledge, this is the first study to test whether using automated symptom and side effect monitoring as an adjunct to usual care can improve outcomes for patients with DPN. We employed a stakeholder-engaged research process involving patients, providers, and health system leaders to align stakeholders and researcher goals and to facilitate the translation of effective strategies into real-world practice.
Stakeholder Engagement Strategies
Throughout the research process, we worked closely with patient partners who had DPN, as well as with clinical and operations leaders. Stakeholders were 3 patient research partners with DPN enrolled at Kaiser Permanente Northern California (KPNC) and 3 primary care physicians (1 of whom is an expert in electronic health record [EHR] physician prompts), a nurse case manager, 2 pharmacists, a health educator, and an endocrinologist who represented health system stakeholders. In addition, we engaged 2 independent neuropathy support groups led by patients in northern California to provide additional patient perspectives on this work outside the KPNC structure.
Our approach to stakeholder engagement was grounded in the PCORI Engagement Rubric, as well as a framework developed by one of the authors (J.S.) on system-based participatory research in health care.43-46 The 4 principles guiding our efforts were (1) partnership and collaboration in all phases of research, (2) building on existing resources and goals, (3) creating and investing in long-term robust partnerships, and (4) engaging in research as a cyclical and iterative process.46
Patient partners and clinician and operations stakeholders were involved at each phase of the study. We held separate orientation meetings with patient partners and clinical and operational stakeholders before the study start in order to establish roles and responsibilities for each research partner. After those initial meetings, we held joint meetings with all stakeholders, including patient partners. All stakeholders were invited to attend weekly and monthly study meetings. We also communicated with all stakeholders via email and phone to address specific study needs.
As previously mentioned, the patient, clinician, and operations stakeholders were engaged at all stages of the research process, including intervention design, participant recruitment and retention methods, specification of outcome measures, data interpretation, report and paper writing, and development of dissemination and communication strategies. In order to increase the generalizability of our research, we also engaged 2 patient-run neuropathy support groups (Walnut Creek and Livermore, California) during the course of the study. We were invited by these groups to present and receive feedback on our study goals and ongoing progress during their regularly scheduled in-person meetings. The groups were especially instrumental in helping us develop and pilot a patient preference instrument as part of a substudy (see below). Their feedback allowed us to redesign this complex tool to facilitate its use by potential participants.
Methods
Design Overview
We conducted a pragmatic cluster randomized controlled trial in primary clinics within the Kaiser Permanente Northern California health system between July 2014 and July 2016.41 The clusters comprised primary care physicians treating patients with diabetes at KPNC. The study was pragmatic in that we designed it to work under usual clinical conditions; minimized exclusions to ensure that eligible patients would be representative of those in usual care; at the request of health system leaders, used an opt out approach for physician recruitment to reduce selection of physicians into the intervention; and employed technologies that are already in use by the health care system as part of usual care (ie, IVR and EHRs) to conduct the intervention.42
We randomized 1834 primary care physicians to either usual care or the intervention, which consisted of usual care plus automated monitoring and feedback of patient-reported symptoms and side effects via IVR. Using the HER, we prospectively identified patients of these physicians who were newly treated for DPN symptoms and contacted them by telephone to provide details about the study and solicit consent to participate. During this call, study staff explained how the automated phone calls would work. Patients were assigned to the treatment or control condition in accordance with their primary care provider's assignment (Figure 1).

Figure 1
Description of Study Groups.
Clustering at the physician level reduced the likelihood of contamination (ie, physicians changing practice for all patients based on the experiences of patients in the intervention group). The Kaiser Permanente Northern California IRB reviewed and approved all research procedures and the study was registered at ClinicalTrials.gov (identifier NCT02056431).
Consent was waived for physicians and written consent was waived for patients because the study involved no more than minimal risk.
Study Setting and Participants
KPNC is a multispecialty health care system with 21 medical centers serving nearly 4 million members in Northern California, including more than 260 000 members with diabetes.47 Usual care for patients with diabetes and related chronic conditions at KPNC involves robust panel management that leverages performance feedback, system-wide efficiencies, disease registries, and evidence-based practice.48 The EHR at KPNC is a critical component of this comprehensive disease management strategy.49,50
All primary care physicians treating at least 1 patient with diabetes enrolled the KPNC Diabetes Registry from July 2013 to July 2014 were eligible for randomization. Patients who were newly treated by these physicians for DPN symptoms were potentially eligible for the study if they were 18 years old at the time they started therapy, were continuously enrolled in KPNC during the 12 months before starting study medications and spoke English or Spanish. We excluded patients who had a diagnosis of gestational diabetes, dementia, or substance abuse disorder during the previous 12 months or who had any opioid use during the 90 days before starting DPN treatment. We excluded these individuals on the advice of our clinician stakeholders because care for these patients may vary substantively from usual care.
Randomization and Patient Recruitment
We undertook a multistage process to roll out the intervention within this large health system. First, the principal investigator (A.A.) presented the IRB-approved protocol to the chief of chief's group for its review and approval. The chief of chief's group agreed to permit recruitment of patients and physicians to the study with the limitation that any physician had the option to withdraw himself or herself or any of his or her patients from the trial at any time. The chiefs were then provided with a 1-page handout providing information about the study that they presented to their staff at a weekly meeting.
We randomized 1834 KPNC primary care physicians treating adult diabetes patients to the intervention or control group on July 11, 2014 (Figure 1). Of those randomized, 1714 physicians had patients who met the initial study inclusion criteria. In October 2014, the principal investigator emailed each individual physician to notify him or her that patients in his or her panels might be approached about participation in a new clinical trial and inviting the physician to opt out any patient due to illness or other factors that would make him or her ineligible for participation.
Among diabetes patients not opted out by their physicians, we used the EHR to prospectively identify patients who had ever had a positive screen for DPN symptoms (according to a brief questionnaire administered during routine primary care visits—85% of diabetes patients in this setting had completed the survey at least once at the time study enrollment began) and who had started a new prescription (ie, no use in the previous 12 months) for 1 of 10 medications commonly used to treat DPN symptoms in this setting (ie, amitriptyline, nortriptyline, imipramine, desipramine, duloxetine, paroxetine, citalopram, pregabalin, venlafaxine, gabapentin) during the previous 2 weeks (total patient enrollment period: September 2014-November 2015).
Study staff sent recruitment letters describing the study and potential risks and benefits of participation to 5716 identified patients. Study interviewers contacted patients by phone approximately 1 week after the letters were sent to obtain phone consent and to conduct a baseline interview. Study staff charged with contacting patients for recruitment and data collection were blind to treatment assignments. Because patients were not randomized individually, patients and providers were also unaware of their treatment assignment at the time they were recruited into the study. Only the main analysts and the individual responsible for scheduling the IVR-enabled calls (see description of intervention below) had access to participants' treatment status during the data collection process. These individuals were not blinded to condition because they were responsible for ensuring that participants received the correct intervention.
Interviewers screened 2203 patients to confirm that the patients had active DPN symptoms and that the prescribed study medications were for DPN and not another condition. In addition, the interviewers excluded patients because of factors that may have impeded participation in the study: The patient did not recall being prescribed a new medication (193), had no symptoms (152), denied having diabetes (2), had only a rotary phone and was thus unable to receive IVR calls (n = 1), was on the no contact registry (n = 1), was no longer a KP member (n = 16), was prescribed a study drug for a condition other than DPN (290), was unable to communicate in English or Spanish (n = 1), or was unreachable or unavailable during the length of the study (n = 3). Some patients were ineligible due to multiple reasons.
For both the treatment and control groups, the first IVR call took place approximately 2 months after the baseline interview. The second IVR call took place approximately 4 months after the initial interview, and the third IVR call took place approximately 6 months after the initial interview. Although the caller ID did not identify the phone number as coming from Kaiser Permanente, participants knew to expect these calls had been given the number earlier. During the baseline assessments, study interviewers explained how the IVR calls worked, what participants should expect, and numbers to call if they were having any difficulties with the IVR calls. Intervention and control calls were offered in English and Spanish.
Intervention
Participants assigned to the intervention group received all usual care for diabetes and DPN, plus three 5-minute IVR calls spaced 2 months apart over the 6-month period following start of DPN treatment (Figure 1 and Appendix A). It is important to note that this study did not influence the initial decision to prescribe medication or the type of medication prescribed. We leveraged access to real-time EHR data to identify new prescriptions for study medications among adult patients with diabetes who had recently reported DPN symptoms.
The intervention calls systematically recorded participant responses to questions about symptoms, medication use, titration, discontinuation, and side effects. Each call was distinct and was based on the stage of the intervention (ie, 2, 4, or 6 months after starting treatment). For a sample IVR script, see Appendix A. A trained research assistant then manually entered the responses into the EHR. To minimize the impact of the intervention on clinic workflow, clinical stakeholders identified 3 types of responses that were potentially actionable and worthy of an additional alert to the physician: dissatisfaction with the level of symptom relief, side effects, and discontinuation of (or failing to start) the medication. We designed the EHR reports so that responses indicating any of these problems would be automatically forwarded directly to the physician via an automated alert system within the EHR to prompt rapid physician response. Responses from patients who reported no major problems with their DPN medication were entered into the health record but not forwarded to the physician since no immediate follow-up was required.
Calls were spaced 2 business days apart, and we made every effort to take into account the time-of-day preferences that participants provided during the baseline interview (these preferences were integrated into the IVR programming). If the participant did not answer the telephone, the IVR system left voicemail messages on the first and third call attempt. The messages included a telephone number so that participants could call back into the system if they preferred. Calls were stopped after the third attempt but were not flagged as complete unless the participant answered the call and confirmed his or her identity by entering his or her medical record number via the touchtone pad or verbally. Once the patients entered their medical record number, they had the opportunity to key ahead—ie, to press touchtone digits before the completion of a voice message. This option allowed participants to quickly navigate through the system without the necessity of listening to all voice prompts.
Touchtone errors occurred when participants either responded incorrectly to a prompt or failed to respond to a prompt within a predetermined time frame. When participants made an invalid entry, an error message was played indicating that the entry was invalid. When participants made no response, an error message was played indicating that no response was detected. Participants were given 3 opportunities to enter valid input. When they exceeded the allowed number of errors, a failure message notified them they could call into the IVR or they could call the help number if they would like to try again. We stored all data, including voice files played and participant responses, in an SQL database.
Each week during the intervention, a trained research assistant manually entered patient responses to the IVR calls into the EHR using an electronic form to minimize data entry errors. The stakeholder requested that no specific protocols be imposed on physicians to allow for individual physician flexibility in responding to the patient reports.
Control Group
Participants assigned to the control group received all usual care for diabetes and DPN, plus 3 noninteractive, automated telephone calls spaced 2 months apart over the 6 months following start of a DPN treatment. The 2-minute automated telephone calls consisted of 3 distinct prerecorded educational messages on health-promoting behaviors for patients with diabetes (ie, diet on the first call, exercise on the second call, and foot care on the third call), and did not allow any input from the patients. The goal of providing these noninteractive calls was to provide all patients with a diabetes-related telephone call in order to assess the marginal benefit of receiving the interactive call in the intervention group.
Outcomes and Follow-up
Trained interviewers collected all patient-reported outcomes during 20-minute phone interviews at approximately 2 months (baseline) and again at 8 months (follow-up) after treatment start. The primary outcome for this study was quality of life, as measured by the EuroQol 5-dimension questionnaire (EQ-5D), a previously validated measure of global quality of life. We calculated this measure from the Global Health Scale, a 10-item PROMIS measure developed and previously validated in patients with neuropathy as part of the Quality of Life in Neurological Disorders measurement system.51 We converted the Global Health Scale into the EQ-5D using previously validated methods to ensure comparability with other studies. The PROMIS measure included questions about quality of life, mood, physical and social functioning, and pain severity. We chose quality of life (assessed as overall health status from worst to best possible health) as our primary outcome of interest because it reflects the overall impact of the condition on patients' lives and is generally underexplored as an outcome in clinical trials of DPN treatment.3,52 We hypothesized that automated monitoring and feedback of information on patient symptoms and side effects to prescribing physicians via the EHR would result in clinically significant improvements in patient quality of life (minimal meaningful difference in EQ-5D = 0.074).53
Outcomes |
---|
Primary outcomes
|
Process outcomes
|
Abbreviations: CAHPS, Consumer Assessment of Healthcare Providers and Systems; ED-5Q, EuroQol 5-dimension questionnaire; max, maximum; min, minimum.
In addition, we evaluated changes in DPN symptoms, including pain, sleep disruption, lower extremity functioning, and depressive symptoms, using previously validated instruments development by the NeuroQOL measurement system.51 Finally, we evaluated 2 process measures to assess changes in the quality of care. First, we assessed patient-perceived changes in communication between patients and their doctors using the 6-item Doctor Communication Composite from the Consumer Assessment of Healthcare Providers and Systems (CAHPS) Health Plan Survey.54 We then used EHR data to calculate whether the participant ever received the minimum effective dose of the treatment he or she was prescribed based on prior clinical trial evidence.2,6,8,9 Specifically, for each medication, we assessed whether the maximum daily dose of the prescribed medication ever reached or surpassed the dose found to be therapeutically effective in clinical trials.6 We assessed this measure for the 12 months following the first observed prescription. All participants who completed the follow-up interview were mailed a $30 gift card along with a letter thanking them for their participation in the study.
Subgroup Analyses
Variation in Treatment Effect by Patient Perception of Shared Decision-Making
Our protocol included a preplanned analysis of differences in effect by the level of participants' perception of the degree to which clinical decisions are shared between patients and their providers. We hypothesized that the intervention would be more effective in participants who perceived a high level of shared decision-making relative to those who perceived a lower level of shared decision-making at baseline. We measured shared decision-making at baseline using the 9-item Shared Decision-Making Questionnaire.55 This questionnaire consists of 9 statements rated on a 6-point scale, ranging from “completely disagree” (0) to “completely agree” (5). A high score indicates that the participant perceives a high level of shared decision-making between himself or herself and his or her physician, and a low score indicates that the participant perceives a low level of shared decision-making (ie, the physician makes most treatment decisions with little input from the participant).
Among participants in the treatment group, we also examined medication-taking behavior and symptom changes over time. We did not test a specific hypothesis, but examined frequencies of specific behaviors (eg, self-titration, discontinuation) and demographic characteristics that could be associated with medication-taking behavior. To our knowledge, only 1 other study has explored the natural history of medication-taking behavior among patients newly prescribed treatment for DPN symptoms in a real-world setting.6
Statistical Analysis
Using the GENMOD procedure in SAS, we used generalized estimating equations with an exchangeable correlation structure to estimate intervention effects for each of the outcomes of interest.56 We hypothesized that the intervention would be associated with improvements in patient-reported outcomes (eg, quality of life, symptoms) through changes in processes of care, including receiving a therapeutically effective dose and patient-provider communication. The clustering unit was the primary care physician, and we included no additional covariates in the models due to the high degree of similarity between the treatment and control groups with respect to baseline characteristics following randomization. We used an intent-to-treat approach,57 including all participants in the analysis with complete baseline and follow-up data regardless of whether they completed all 3 intervention calls.
All participants were required to complete the quality-of-life and sleep disruption surveys. However, to minimize patient burden, we administered instruments related to pain, depressive symptoms, or lower extremity functioning only to patients who indicated problems with these symptoms in the quality-of-life survey. For the statistical analysis, we imputed missing symptom data as follows: pain interference: minimum observed value − 0.1 = 40.6; lower extremity function: maximum observed value + 0.1 = 58.7; and depressive symptoms: minimum observed value − 0.1 = 38.1. This strategy directly accounted for skipping patterns by setting outcomes for the symptom level measures to the best possible value for those who did not indicate these symptoms on the quality-of-life survey.
Due to the prospective nature of patient recruitment in this study, we calculated power by estimating the number of potentially eligible patients per month and using that number to determine the minimum detectable effect. We then compared the minimum detectible effect with the smallest clinically meaningful difference for the primary outcome. Specifically, we estimated that 118 patients would be newly started on DPN treatment each month and that the average number of patients per physician would be 1. Assuming a response rate (willingness to participate) of 40% and an 18-month enrollment period, we aimed to enroll a total of 850 patients, or 425 per group. With a cluster size of 1, power calculations for a cluster randomized controlled trial are equivalent to standard power calculations for experiments with simple randomization (n = 2(zα/2 + zβ)2σ2/d2).58
Based on the large size of the KPNC diabetes population, we estimated that the minimum detectable mean score difference between the 2 groups with α = .05, β = .2, and an outcome (EQ-5D) with an SD of 0.143 was 0.017. Therefore, we anticipated having more than 80% power to detect differences in the change of the primary outcome between the treated and control groups that were smaller than the clinically meaningful difference in EQ-5D of 0.074, as reported in a prior study.53,59 However, during the study, we identified more patients than anticipated and completed enrollment in 15 months instead of 18.
Sensitivity Analysis
Missing data are a common challenge in clinical research, and our study was at risk for bias due to patients dropping out of the study over time. Specifically, in analyzing only data from patients who completed the study, we may have introduced bias if those who dropped out did so for systematic reasons (eg, dissatisfaction with the intervention). In our proposal, we suggested addressing systematic differences in characteristics between those who completed the study and those who dropped out by using inverse probability weighting to adjust for missing outcome or covariate data.60
We did not adjust for missing outcome or covariate data, however, for the following 3 reasons: (1) Only 6% of patient outcome data were missing, (2) outcome missingness was not associated with being in the treatment or control group (see Appendix B), and (3) outcome missingness was not associated with baseline covariates. Based on these results, we did not expect that inferences from this trial would change due to an inverse probability weighting sensitivity analysis to address missing data.
Another threat to the internal validity of our study was the potential for physicians to learn their treatment assignment status following receipt of the first IVR report for their first patient. To mitigate concerns that physicians could opt out subsequent patients from the trial, we conducted sensitivity analyses in which only data from the first patient of each physician contributed to the statistical analyses. We also conducted 2 additional sensitivity analyses based on the potential outcome framework (ie, causal analytic perspective). For analyses, we defined a unique aggregate outcome for each physician enrolled in the trial defined as the average of his or her patient's outcomes for physicians with more than 1 enrolled patient. In the first approach, we set this outcome to missing if at least 1 of the physician's patients had a missing outcome. In the second approach, the physician's outcome was missing only if all of his or her patients had a missing outcome. In these 2 approaches, we then contrasted outcomes between the 2 groups using a simple linear regression (no repeated measurements).
Contextual Considerations
As this was a pragmatic trial,61 it was important to gather information from providers regarding the feasibility and utility of the intervention for clinical care. Therefore, at the end of the study, we conducted a brief, limited survey of physicians who were in the intervention group. We included the following 5 survey topics: (1) physician awareness of study, (2) physician use of study-provided data, (3) effect of study on patient management, (4) physician feedback/critiques of study protocols, and (5) relevancy of protocol for other conditions. All questions were closed-ended, multiple choice questions, or simply yes/no, and physicians had the ability to add open-ended comments. This survey was not part of the original study protocol, and we did not intend for it to have statistical power and be generalizable to the broader population of physicians. Rather, we conceptualized this survey as a way to obtain some preliminary data to identify issues that were important to at least some participating physicians.
We randomly selected 186 physicians in the intervention group who received at least 1 alert during the study to receive an email from the principal investigator asking them to complete the survey (by phone or email) regarding their experience with the intervention. We sent emails in batches of 50 until we identified 30 physicians who were willing to participate in the survey. Physicians who did not respond directly to the email received a recruitment phone call from a research assistant 1 week later.
We did not formally analyze the survey results, but rather examined frequencies of response to questions about physician recall and experience with automated monitoring reports.
Substudy 1: Best–Worst Scaling Experiment
Design Overview
In addition to the subgroup analyses described above, we had planned to assess whether the intervention was more effective among patients whose preferences for specific treatment characteristics (eg, 1 pill per day, costs less than $20) were a match with their prescribed treatment using best–worst scaling methods to assess patient preferences.62 However, feedback from patient stakeholders and trained interviewers indicated that patients would have had difficulty completing the proposed preference questionnaire via phone. Therefore, we designed and conducted a separate substudy to assess patient preference for DPN medication among patients who were potentially eligible for, but were not invited to participate in, the intervention study due to our inability to contact them before the recruitment window ended. We conducted this substudy to determine whether this methodology to assess patient preferences about DPN treatment would be feasible for future studies.
We designed an object case best–worst scaling preference instrument using data from the in-depth pilot interviews with 42 patients with DPN served by Kaiser Permanente in the Mid-Atlantic, Colorado, and Northern California regions that were conducted before the outset of this trial. In these interviews, factors most associated with DPN treatment decisions included symptom relief, side effects, provider support, medication costs, and family concerns. The in-depth interviews resulted in the identification of 9 key attributes associated with DPN treatment decisions.
We used Sawtooth Software63 to design an experiment that balanced the number of times each treatment attribute (eg, costs more than $20) appeared as a choice to patients, as well as the number of times attributes appeared in the same choice set (eg, when taking it, I can do the things I usually do vs costs more than $20 per month). We chose a block design in which patients compared 5 different attributes at a time, choosing the attribute that was most and least important to them when deciding to take a DPN medication, and in which the maximum number of comparisons presented to any 1 patient was 9. An example of the final instrument is included in Appendix B.
Participants
We conducted 2 rounds of pilot testing. First, the 3 patient stakeholders on the research team, 2 clinician stakeholder partners, and the study interviewers completed the best–worst survey task as if they were the participants and then debriefed with the full team regarding their experience with the instrument. We then fielded the revised questionnaire with a subgroup of 19 patients who were members of a neuropathy support group and who provided feedback on the instrument to the study team. Patients who were potentially eligible for the main study, but who were not contacted about the main study due to our inability to reach them before the time window for recruitment closed (ie, 2-4 weeks since treatment start), were eligible for inclusion in the best–worst scaling substudy. We selected a randomized sample of 586 patients stratified by race or ethnicity (ie, African American, Asian, Hispanic, and White) and randomly selected 12 to 15 participants from each subgroup. We oversampled Asian and Hispanic patients due to their underrepresentation in the main study. Interviewers contacted 517 of the 586 patients to identify 100 who consented to participate. Interviewers could not reach 64 patients despite repeated attempts. Of those who received a recruitment call, 205 patients were determined to be ineligible. Of those eligible to participate, common reasons for nonparticipation were unwillingness or inability to participate or failure to appear for the in-person interview.
Intervention
The best–worst scaling instrument (Appendix B) was administered as a 15-page pen-and-paper survey provided to substudy participants during one-on-one in-person interviews with trained interviewers at the KPNC Division of Research in downtown Oakland, California, and at local medical centers 20 to 30 miles from Oakland. Substudy participants were asked to review each choice set independently and to choose 1 attribute that was most important and 1 that was least important to them in deciding whether to try a hypothetical medication for treatment of DPN symptoms. The interviewer was available to assist substudy participants in completing the questionnaire. The survey took approximately 10 to 15 minutes to complete. Substudy participants provided written consent before completing the survey and were mailed a $50 gift card along with a letter thanking them for their participation in the survey.
Results of the Main Study
Trained interviewers identified 1535 patients who were eligible for participation in the main study, and of these 83% (n = 1270) consented to participate. Reasons for declining consent included lack of interest (n = 173), being too busy (n = 44), and being too sick to participate (n = 52).
Patients could indicate more than 1 reason to decline consent. Among those who consented, 1252 completed the baseline assessment and 1179 (619 control group, 560 intervention group) completed the follow-up interview, resulting in an overall retention rate of 93% among consented participants and in both the control and treatment groups. A flow diagram of patient recruitment and retention is presented in Figure 2 and a comparison of characteristics between participants who did and did not complete the follow-up interview is provided in Appendix C. Although there were no statistically significant differences in characteristics between participants with and without complete follow-up data, there were a few differences of note. A higher proportion of participants with complete data than those with incomplete data were female (54.2% of those with complete data, 42.5% of those with incomplete data; P = .054) and perceived that their physician made decisions on their behalf according to the shared decision-making scale (13.1% of those with complete data, 6.9% of those with incomplete data; P = .064). In addition, the patients with complete data (mean ± SD, EQ-5D, 0.7 ± 0.10) had slightly higher (better) scores on quality-of-life measures than those who dropped out (0.60 ± 0.10; P = .051) Characteristics of participants who completed the baseline interview are presented in Table 2.

Figure 2
Clinical Trial Flowchart.
Table 2
Results of Primary and Secondary Outcome Measures.
Table 1
Baseline Characteristics of 1252 Patients With Complete Baseline Data.
Most patients were older than 65 years (mean age, 67 years; SD, 11.7 years) and female (53.5%). The population was racially and ethnically diverse (56.6% White, 8.2% Asian, 13.3% Black, 19.7% Hispanic) and 21.3% were living in economically depressed areas as evidenced by the neighborhood deprivation index. Of participants, 15% had an A1c above 9%, and 37.5% reported having calf pain before the baseline interview. There were no statistically significant differences in baseline characteristics between the intervention and control groups.
The intervention did not lead to clinically significant changes in quality of life (−0.002 [95% CI, −0.01 to 0.01]) or DPN symptoms (Table 2). The intervention was not associated with changes in the processes of care, including the likelihood of receiving the minimum effective dose at 12 months (treatment = 52%; control = 48%; differences = 0.03 percentage points [95% CI, −0.08 to 0.02]) or patient satisfaction with patient-provider communication (difference = −0.45 [95% CI, −0.97 to 0.07]). In the subgroup analyses, we observed no significant interactions related to shared decision-making (Appendix D).
We also analyzed data collected from patients in the intervention group via the IVR. Among participants in the intervention group, most patients (74%) reported side effects, dissatisfaction with treatment, or medication discontinuation during the first IVR call, which triggered a physician alert in the EHR. We observed no differences in demographic characteristics among participants who reported these problems on the first call compared with those who did not. Side effects were the most common problem reported and occurred for more than 50% of participants. Among participants who were actively using the medication at the time of the first call, more than 95% reported taking it once a day and 20% reported having increased the dose. Among those completing their third and final call (approximately 6 months after starting medication), only 11% had discontinued use, 16% had increased the dose, and 20% had added another medication since the second call (data not shown).
Sensitivity Analyses
The sensitivity analyses produced consistent findings whether they were analyzed at the physician or patient level and whether all participants were included or only the first enrolled participant for each physician was included.
Contextual Considerations
In follow-up surveys with 31 physicians in the intervention group, 13 reported being aware or very aware of the study, and only 7 recalled seeing the electronic symptom and side effect reports. Of the physicians, 23 reported that the automated monitoring strategy could be useful for other conditions and medications, including diabetes, hypertension, chronic pain, depression, migraines, warfarin, and antihypertensive agents. However, several noted that the limited effectiveness and tolerability of available DPN treatments might limit the utility of ongoing monitoring for these patients.
Quotes: physicians receiving alerts |
---|
“[It could be helpful], but only when answers are black and white.” “[It] might be good for blood thinners like Coumadin, and for other medications that have serious side effects.” |
Preference (Best–Worst Scaling) Substudy
We conducted preference assessments with 100 patients (mean age, 61 years). Most of the participants were female (52%) and 34% were White, 33% were Black, 16% were Asian, 14% were Hispanic, and 3% did not report race or ethnicity. The factor substudy participants identified as being most important to treatment decision-making was whether the medication allowed them to “do the things that I usually do” (Figure 3). This is consistent with our prior in-depth interviews, indicating that for patients, improving quality of life is the highest priority for treatment. The second most important factor was whether “my doctor and I discuss my concerns about this medication.” The factor substudy participants identified as being least important was the medication cost, followed by whether they had to take more than 1 pill each day.
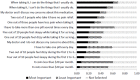
Figure 3
Results of Best-Worst Scaling Substudy (n-100): Aggregate Proportion of Times Each Item Was Selected as Most Important, Least Important, or Was Not Selected.
Discussion
Context for Study Results
We conducted a pragmatic cluster randomized trial testing the use of telephone-automated patient-reported data collection and feedback via the EHR to help physicians more effectively manage DPN medications. Although the sample was large and response and retention rates were high, we were unable to demonstrate a clinically significant improvement in DPN symptoms because of the intervention. Despite high rates of self-reported adherence to medications throughout the follow-up period in the intervention group, half of enrolled patients never received the minimally effective dose of DPN medication.
Generalizability of Findings
Generalizability of the key findings may be somewhat limited by the fact that this study was conducted in an integrated health care system with a comprehensive diabetes care program. However, the demographic profile of patients who receive care in Kaiser Permanente Northern California closely matches that of the region's racial, ethnic, and socioeconomic diversity.
Table 3
Detailed Summary of Call Completion Rates.
Implementation of Study Results
Our negative research findings are in conflict with prior studies demonstrating positive effects of technology-based monitoring of patient-reported treatment outcomes in diabetes and other conditions.30-34 One possible explanation for this difference is that relative to prior interventions, our brief intervention was not sufficiently intensive to influence care or outcomes. For example, we did not dictate a specific medication protocol for physicians to follow once they received an alert via the EHR. In addition, the vast majority of participants in the main study met our criteria for physician alert, which may have diminished the urgency of the alerts, particularly in light of “alert fatigue” experienced by primary care physicians.27,28 The limited number of participants per physician in the study may have further minimized the likelihood that physicians would dramatically change how they treated these patients relative to other patients with diabetes.
Also, our intent-to-treat approach meant that participants who did not complete the intervention calls were included in the treatment group and may have biased our estimates toward the null. The absence of a significant treatment effect in this study may also reflect the choice of DPN as the target condition due to the lack of highly effective treatment for DPN symptoms and, consequently, the lack of options for physician response in light of patient reports. In particular, the primary treatment for patients following the failure with first-line therapy is opioid analgesics.4 Therefore, our failure to change prescribing behavior may reflect the presence of omission bias or the tendency of physicians to undertreat out of concern that treatment will cause further harm.64 This tendency toward undertreatment may be particularly strong when outcomes related to the natural progression of disease (ie, DPN symptoms) are considered more acceptable than potential side effects associated with treatment. The development of new treatments targeting the type and source of DPN pain may enhance the value of automated monitoring for patients with DPN.12,24 In addition, there is promising evidence from the Department of Veterans Affairs regarding cognitive behavioral therapy as an adjunct to first-line pharmacologic treatment.65
Our finding of a slight, nonsignificant decline in patient-provider communication in the intervention group may indicate increased expectations for engagement among patients in this group. This effect may be due in part to our decision to maximize physician acceptance of the intervention by allowing each physician to decide how to respond to patient reports. In this case, our effort to maximize the acceptability of the intervention may have limited its impact on clinical care.
Key lessons learned |
---|
|
Abbreviation: DPN, diabetic peripheral neuropathy.
Subpopulation Considerations
We conducted subgroup analyses based on the patient's perception of the degree to which he or she participated in clinical decisions with his or her provider (ie, shared decision-making) in accordance with our protocol. However, we observed no subgroup differences in effect.
Study Limitations
Our study had several limitations that deserve consideration. At the request of our clinical stakeholder, this study did not include a formal protocol for physicians, which may have severely limited the potential for this intervention to change practice. In addition, we could not systematically measure or track whether physicians read the forwarded alerts regarding patient IVR responses. In our convenience sample poststudy survey of 31 physicians, only 7 reported having seen the patient alerts. Our findings may not extend to patient subgroups that we explicitly excluded from participation (eg, those with dementia). However, we endeavored to include a diverse cohort of patients, and the consented patients were quite similar to the overall diabetes population. Despite our efforts to assess patient eligibility using both clinical data and patient self-reports, we may have included some patients who were taking study medications for conditions other than DPN. This possibility is unlikely to have biased our findings, but may have diluted the impact of the intervention, which focused on DPN treatment specifically. In addition, our measure of patient-provider communication assessed patient perception of the quality of communication over the prior 12 months, which was longer than our follow-up period of 8 months. As a result, this measure may capture aspects of patient–physician interactions that are not specific to DPN management. Finally, medications are dispensed for an average of 100 days in this setting and the intervention took place over 6 months. As a consequence, it was difficult to assess smaller changes in medication dosing below the threshold for the minimum effective dose.
Conclusions
In contrast with our hypothesis and prior studies using similar technologies,30-34 our intervention was not effective at improving patient quality of life or symptoms. The lack of effect of this particular intervention may be due to the relatively low-intensity nature of the intervention, including the absence of a specific protocol for the prescribing physician, and the limited efficacy of available treatments for DPN symptoms. Certainly, more effective and tolerable pharmacotherapy and nonpharmacotherapy treatments for DPN are needed. As one of the first studies to employ automated monitoring with the intent of improving outcomes for patients with DPN, this study demonstrated the feasibility of instituting this type of system for symptom and side effect monitoring of patients with EHR feedback. IVR technology is widely available within health systems, can be used to monitor patients outside health care settings, and has demonstrated efficacy for improving diabetes adherence and control.31,35 However, for complex conditions like DPN for which concerns about treatment side effects may contribute to intentional undertreatment,6,7 automated monitoring of symptoms and treatment side effects may simply reinforce more conservative dosing strategies (ie, first do no harm).64 Therefore, for patients with DPN, automated monitoring with IVR may be more effective if it focused on patients who are most likely to benefit from, rather than be harmed by, available treatments.
Accurate identification of patients most likely to benefit from treatment may override clinician defaults and promote more guideline-consistent prescribing. Given the high costs of undertreatment of DPN symptoms for patients and health systems,2 inaction is not an option. Evidence-based strategies are needed to help patients and clinicians balance the potential benefits and harms of treatment.
References
- 1.
- Tesfaye S, Selvarajah D. Advances in the epidemiology, pathogenesis and management of diabetic peripheral neuropathy. Diabetes Metab Res Rev. 2012;28(suppl 1):8-14. doi:10.1002/dmrr.2239 [PubMed: 22271716] [CrossRef]
- 2.
- American Diabetes Association. Economic costs of diabetes in the U.S. in 2012. Diabetes Care. 2013;36(4):1033-1046. doi:10.2337/dc12-2625 [PMC free article: PMC3609540] [PubMed: 23468086] [CrossRef]
- 3.
- Sadosky A, Schaefer C, Mann R, et al. Burden of illness associated with painful diabetic peripheral neuropathy among adults seeking treatment in the US: results from a retrospective chart review and cross-sectional survey. Diabetes Metab Syndr Obes. 2013;6:79-92. doi:10.2147/dmso.s37415 [PMC free article: PMC3569051] [PubMed: 23403729] [CrossRef]
- 4.
- American Diabetes Association. Standards of medical care in diabetes—2015 abridged for primary care providers. Clin Diabetes. 2015;33(2):97-111. doi:10.2337/diaclin.33.2.97 [PMC free article: PMC4398006] [PubMed: 25897193] [CrossRef]
- 5.
- The Diabetes Control and Complications Trial Research Group. The effect of intensive diabetes therapy on the development and progression of neuropathy. Ann Intern Med. 1995;122(8):561-568. [PubMed: 7887548]
- 6.
- Yang M, Qian C, Liu Y. Suboptimal treatment of diabetic peripheral neuropathic pain in the United States. Pain Med. 2015;16(11):2075-2083. doi:10.1111/pme.12845 [PubMed: 26118704] [CrossRef]
- 7.
- Sobhy T. The need for improved management of painful diabetic neuropathy in primary care. Pain Res Manag. 2016;2016:1974863. doi:10.1155/2016/1974863 [PMC free article: PMC4904618] [PubMed: 27445600] [CrossRef]
- 8.
- Callaghan BC, Cheng HT, Stables CL, Smith AL, Feldman EL. Diabetic neuropathy: clinical manifestations and current treatments. Lancet Neurol. 2012;11(6):521-534. doi:10.1016/s1474-4422(12)70065-0 [PMC free article: PMC4254767] [PubMed: 22608666] [CrossRef]
- 9.
- Bril V, England J, Franklin GM, et al. Evidence-based guideline: treatment of painful diabetic neuropathy: report of the American Academy of Neurology, the American Association of Neuromuscular and Electrodiagnostic Medicine, and the American Academy of Physical Medicine and Rehabilitation. Neurology. 2011;76(20):1758-1765. doi:10.1212/WNL.0b013e3182166ebe [PMC free article: PMC3100130] [PubMed: 21482920] [CrossRef]
- 10.
- Griebeler ML, Morey-Vargas OL, Brito JP, et al. Pharmacologic interventions for painful diabetic neuropathy: an umbrella systematic review and comparative effectiveness network meta-analysis. Ann Intern Med. 2014;161(9):639-649. doi:10.7326/m14-0511 [PubMed: 25364885] [CrossRef]
- 11.
- Marchettini P, Wilhelm S, Petto H, et al. Are there different predictors of analgesic response between antidepressants and anticonvulsants in painful diabetic neuropathy? Eur J Pain. 2016;20(3):472-482. doi:10.1002/ejp.763 [PubMed: 26311228] [CrossRef]
- 12.
- Snyder MJ, Gibbs LM, Lindsay TJ. Treating painful diabetic peripheral neuropathy: an update. Am Fam Physician. 2016;94(3):227-234. [PubMed: 27479625]
- 13.
- Moon DE, Lee DI, Lee SC, et al. Efficacy and tolerability of pregabalin using a flexible, optimized dose schedule in Korean patients with peripheral neuropathic pain: a 10-week, randomized, double-blind, placebo-controlled, multicenter study. Clin Ther. 2010;32(14):2370-2385. doi:10.1016/j.clinthera.2011.01.014 [PubMed: 21353106] [CrossRef]
- 14.
- Perez C, Navarro A, Saldana MT, Masramon X, Rejas J. Pregabalin and gabapentin in matched patients with peripheral neuropathic pain in routine medical practice in a primary care setting: findings from a cost-consequences analysis in a nested case-control study. Clin Ther. 2010;32(7):1357-1370. doi:10.1016/j.clinthera.2010.07.014 [PubMed: 20678683] [CrossRef]
- 15.
- Satoh J, Yagihashi S, Baba M, et al. Efficacy and safety of pregabalin for treating neuropathic pain associated with diabetic peripheral neuropathy: a 14 week, randomized, double-blind, placebo-controlled trial. Diabet Med. 2011;28(1):109-116. doi:10.1111/j.1464-5491.2010.03152.x [PubMed: 21166852] [CrossRef]
- 16.
- Armstrong DG, Chappell AS, Le TK, et al. Duloxetine for the management of diabetic peripheral neuropathic pain: evaluation of functional outcomes. Pain Med. 2007;8(5):410-418. doi:10.1111/j.1526-4637.2007.00276.x [PubMed: 17661854] [CrossRef]
- 17.
- Goldstein DJ, Lu Y, Detke MJ, Lee TC, Iyengar S. Duloxetine vs. placebo in patients with painful diabetic neuropathy. Pain. 2005;116(1-2):109-118. doi:10.1016/j.pain.2005.03.029 [PubMed: 15927394] [CrossRef]
- 18.
- Vinik AI, Bril V, Kempler P, et al. Treatment of symptomatic diabetic peripheral neuropathy with the protein kinase C beta-inhibitor ruboxistaurin mesylate during a 1-year, randomized, placebo-controlled, double-blind clinical trial. Clin Ther. 2005;27(8):1164-1180. doi:10.1016/j.clinthera.2005.08.001 [PubMed: 16199243] [CrossRef]
- 19.
- Raskin J, Pritchett YL, Wang F, et al. A double-blind, randomized multicenter trial comparing duloxetine with placebo in the management of diabetic peripheral neuropathic pain. Pain Med. 2005;6(5):346-356. doi:10.1111/j.1526-4637.2005.00061.x [PubMed: 16266355] [CrossRef]
- 20.
- Quilici S, Chancellor J, Lothgren M, et al. Meta-analysis of duloxetine vs. pregabalin and gabapentin in the treatment of diabetic peripheral neuropathic pain. BMC Neurol. 2009;9:6. doi:10.1186/1471-2377-9-6 [PMC free article: PMC2663537] [PubMed: 19208243] [CrossRef]
- 21.
- Tanenberg RJ, Irving GA, Risser RC, et al. Duloxetine, pregabalin, and duloxetine plus gabapentin for diabetic peripheral neuropathic pain management in patients with inadequate pain response to gabapentin: an open-label, randomized, noninferiority comparison. Mayo Clin Proc. 2011;86(7):615-626. doi:10.4065/mcp.2010.0681 [PMC free article: PMC3127557] [PubMed: 21719618] [CrossRef]
- 22.
- Hanna M, O'Brien C, Wilson MC. Prolonged-release oxycodone enhances the effects of existing gabapentin therapy in painful diabetic neuropathy patients. Eur J Pain. 2008;12(6):804-813. doi:10.1016/j.ejpain.2007.12.010 [PubMed: 18262450] [CrossRef]
- 23.
- Gilron I, Bailey JM, Tu D, Holden RR, Jackson AC, Houlden RL. Nortriptyline and gabapentin, alone and in combination for neuropathic pain: a double-blind, randomised controlled crossover trial. Lancet. 2009;374(9697):1252-1261. doi:10.1016/s0140-6736(09)61081-3 [PubMed: 19796802] [CrossRef]
- 24.
- Waldfogel JM, Nesbit SA, Dy SM, et al. Pharmacotherapy for diabetic peripheral neuropathy pain and quality of life: a systematic review. Neurology. 2017;88(20):1958-1967. doi:10.1212/wnl.0000000000003882 [PubMed: 28341643] [CrossRef]
- 25.
- Pop-Busui R, Boulton AJ, Feldman EL, et al. Diabetic neuropathy: a position statement by the American Diabetes Association. Diabetes Care. 2017;40(1):136-154. doi:10.2337/dc16-2042 [PMC free article: PMC6977405] [PubMed: 27999003] [CrossRef]
- 26.
- Adams AS, Parker MM, Moffet HH, et al. Communication barriers and the clinical recognition of diabetic peripheral neuropathy in a diverse cohort of adults: the DISTANCE study. J Health Commun. 2016;21(5):544-553. doi:10.1080/10810730.2015.1103335 [PMC free article: PMC4920056] [PubMed: 27116591] [CrossRef]
- 27.
- Grant RW, Adams AS, Bayliss EA, Heisler M. Establishing visit priorities for complex patients: a summary of the literature and conceptual model to guide innovative interventions. Healthc (Amst). 2013;1(3-4):117-122. doi:10.1016/j.hjdsi.2013.07.008 [PMC free article: PMC4058767] [PubMed: 24944911] [CrossRef]
- 28.
- Bodenheimer T, Pham HH. Primary care: current problems and proposed solutions. Health Aff (Millwood). 2010;29(5):799-805. doi:10.1377/hlthaff.2010.0026 [PubMed: 20439864] [CrossRef]
- 29.
- Adams AS, Callaghan B, Grant RW. Overcoming barriers to diabetic polyneuropathy management in primary care. Healthc (Amst). 2016. doi:10.1016/j.hjdsi.2016.10.003 [PMC free article: PMC7265436] [PubMed: 27939169] [CrossRef]
- 30.
- Piette JD, Krein SL, Striplin D, et al. Patient-centered pain care using artificial intelligence and mobile health tools: protocol for a randomized study funded by the US Department of Veterans Affairs Health Services Research and Development Program. JMIR Res Protoc. 2016;5(2):e53. doi:10.2196/resprot.4995 [PMC free article: PMC4856067] [PubMed: 27056770] [CrossRef]
- 31.
- Glasgow RE, Bull SS, Piette JD, Steiner JF. Interactive behavior change technology. a partial solution to the competing demands of primary care. Am J Prev Med. 2004;27(suppl 2):80-87. doi:10.1016/j.amepre.2004.04.026 [PubMed: 15275676] [CrossRef]
- 32.
- Lieberman G, Naylor MR. Interactive voice response technology for symptom monitoring and as an adjunct to the treatment of chronic pain. Transl Behav Med. 2012;2(1):93-101. doi:10.1007/s13142-012-0115-x [PMC free article: PMC3291819] [PubMed: 22448205] [CrossRef]
- 33.
- Basch E, Deal AM, Dueck AC, et al. Overall survival results of a trial assessing patient-reported outcomes for symptom monitoring during routine cancer treatment. JAMA. 2017;318(2):197-198. doi:10.1001/jama.2017.7156 [PMC free article: PMC5817466] [PubMed: 28586821] [CrossRef]
- 34.
- Martinez LS, Schwartz JS, Freres D, Fraze T, Hornik RC. Patient-clinician information engagement increases treatment decision satisfaction among cancer patients through feeling of being informed. Patient Educ Couns. 2009;77(3):384-390. doi:10.1016/j.pec.2009.09.008 [PMC free article: PMC3279194] [PubMed: 19815365] [CrossRef]
- 35.
- Piette JD. Interactive voice response systems in the diagnosis and management of chronic disease. Am J Manag Care. 2000;6(7):817-827. [PubMed: 11067378]
- 36.
- Tulsky JA. Interventions to enhance communication among patients, providers, and families. J Palliat Med. 2005;8(suppl 1):S95-S102. doi:10.1089/jpm.2005.8.s-95 [PubMed: 16499474] [CrossRef]
- 37.
- Piette JD, Rosland AM, Marinec NS, Striplin D, Bernstein SJ, Silveira MJ. Engagement with automated patient monitoring and self-management support calls: experience with a thousand chronically ill patients. Med Care. 2013;51(3):216-223. doi:10.1097/MLR.0b013e318277ebf8 [PMC free article: PMC3711084] [PubMed: 23222527] [CrossRef]
- 38.
- Willig JH, Krawitz M, Panjamapirom A, et al. Closing the feedback loop: an interactive voice response system to provide follow-up and feedback in primary care settings. J Med Syst. 2013;37(2):9905. doi:10.1007/s10916-012-9905-4 [PMC free article: PMC3662799] [PubMed: 23340825] [CrossRef]
- 39.
- Estabrooks PA, Smith-Ray RL. Piloting a behavioral intervention delivered through interactive voice response telephone messages to promote weight loss in a pre-diabetic population. Patient Educ Couns. 2008;72(1):34-41. doi:10.1016/j.pec.2008.01.007 [PubMed: 18282679] [CrossRef]
- 40.
- Mundt JC. Interactive voice response systems in clinical research and treatment. Psychiatr Serv. 1997;48(5):611-612. doi:10.1176/ps.48.5.611 [PubMed: 9144814] [CrossRef]
- 41.
- Adams AS, Bayliss EA, Schmittdiel JA, et al. The Diabetes Telephone Study: design and challenges of a pragmatic cluster randomized trial to improve diabetic peripheral neuropathy treatment. Clin Trials. 2016;13(3):286-293. doi:10.1177/1740774516631530 [PMC free article: PMC7261503] [PubMed: 27034455] [CrossRef]
- 42.
- Loudon K, Treweek S, Sullivan F, Donnan P, Thorpe KE, Zwarenstein M. The PRECIS-2 tool: designing trials that are fit for purpose. BMJ. 2015;350:h2147. doi:10.1136/bmj.h2147 [PubMed: 25956159] [CrossRef]
- 43.
- Frank L, Basch E, Selby JV. The PCORI perspective on patient-centered outcomes research. JAMA. 2014;312(15):1513-1514. doi:10.1001/jama.2014.11100 [PubMed: 25167382] [CrossRef]
- 44.
- Fleurence RL, Forsythe LP, Lauer M, et al. Engaging patients and stakeholders in research proposal review: the Patient-Centered Outcomes Research Institute. Ann Intern Med. 2014;161(2):122-130. doi:10.7326/m13-2412 [PubMed: 25023251] [CrossRef]
- 45.
- Fleurence RL, Beal AC, Sheridan SE, Johnson LB, Selby JV. Patient-powered research networks aim to improve patient care and health research. Health Aff (Millwood). 2014;33(7):1212-1219. doi:10.1377/hlthaff.2014.0113 [PubMed: 25006148] [CrossRef]
- 46.
- Schmittdiel JA, Grumbach K, Selby JV. System-based participatory research in health care: an approach for sustainable translational research and quality improvement. Ann Fam Med. 2010;8(3):256-259. doi:10.1370/afm.1117 [PMC free article: PMC2866724] [PubMed: 20458110] [CrossRef]
- 47.
- Karter AJ, Schillinger D, Adams AS, et al. Elevated rates of diabetes in Pacific Islanders and Asian subgroups: the Diabetes Study of Northern California (DISTANCE). Diabetes Care. 2013;36(3):574-579. doi:10.2337/dc12-0722 [PMC free article: PMC3579366] [PubMed: 23069837] [CrossRef]
- 48.
- Jaffe MG, Lee GA, Young JD, Sidney S, Go AS. Improved blood pressure control associated with a large-scale hypertension program. JAMA. 2013;310(7):699-705. doi:10.1001/jama.2013.108769 [PMC free article: PMC4270203] [PubMed: 23989679] [CrossRef]
- 49.
- Reed M, Huang J, Graetz I, et al. Outpatient electronic health records and the clinical care and outcomes of patients with diabetes mellitus. Ann Intern Med. 2012;157(7):482-489. doi:10.7326/0003-4819-157-7-201210020-00004 [PMC free article: PMC3603566] [PubMed: 23027319] [CrossRef]
- 50.
- Reed M, Huang J, Brand R, et al. Implementation of an outpatient electronic health record and emergency department visits, hospitalizations, and office visits among patients with diabetes. JAMA. 2013;310(10):1060-1065. doi:10.1001/jama.2013.276733 [PMC free article: PMC4503235] [PubMed: 24026601] [CrossRef]
- 51.
- National Institute of Neurological Disorders and Stroke (NINDS). User Manual for the Quality of Life in Neurological Disorders (Neuro-QOL) Measures, Version 1.0. March 2015. http://www
.healthmeasures .net/images/neuro_qol /Neuro-QOL_User _Manual_v2_24Mar2015.pdf - 52.
- Bredfeldt C, Altschuler A, Adams AS, Portz JD, Bayliss EA. Patient reported outcomes for diabetic peripheral neuropathy. J Diabetes Complications. 2015;29(8):1112-1118. doi:10.1016/j.jdiacomp.2015.08.015 [PubMed: 26385309] [CrossRef]
- 53.
- Revicki DA, Kawata AK, Harnam N, Chen WH, Hays RD, Cella D. Predicting EuroQol (EQ-5D) scores from the Patient-Reported Outcomes Measurement Information System (PROMIS) global items and domain item banks in a United States sample. Qual Life Res. 2009;18(6):783-791. doi:10.1007/s11136-009-9489-8 [PMC free article: PMC2704290] [PubMed: 19472072] [CrossRef]
- 54.
- Hargraves JL, Hays RD, Cleary PD. Psychometric properties of the Consumer Assessment of Health Plans Study (CAHPS) 2.0 adult core survey. Health Serv Res. 2003;38(6 Pt 1):1509-1527. [PMC free article: PMC1360961] [PubMed: 14727785]
- 55.
- Kriston L, Scholl I, Holzel L, Simon D, Loh A, Harter M. The 9-item Shared Decision-Making Questionnaire (SDM-Q-9). Development and psychometric properties in a primary care sample. Patient Educ Couns. 2010;80(1):94-99. doi:10.1016/j.pec.2009.09.034 [PubMed: 19879711] [CrossRef]
- 56.
- SAS OnlineDoc. Version 9. SAS Institute, Inc; 2002-2006.
- 57.
- Liao JM, Stack CB, Griswold ME, Localio AR. Annals understanding clinical research: intention-to-treat analysis. Ann Intern Med. 2017;166(9):662-664. doi:10.7326/m17-0196 [PubMed: 28288485] [CrossRef]
- 58.
- Kerry SM, Bland JM. Sample size in cluster randomisation. BMJ. 1998;316(7130):549. [PMC free article: PMC2665662] [PubMed: 9501723]
- 59.
- Walters SJ, Brazier JE. Comparison of the minimally important difference for two health state utility measures: EQ-5D and SF-6D. Qual Life Res. 2005;14(6):1523-1532. [PubMed: 16110932]
- 60.
- Seaman SR, White IR. Review of inverse probability weighting for dealing with missing data. Stat Methods Med Res. 2013;22(3):278-295. doi:10.1177/0962280210395740 [PubMed: 21220355] [CrossRef]
- 61.
- Roland M, Torgerson DJ. What are pragmatic trials? BMJ. 1998;316(7127):285. [PMC free article: PMC2665488] [PubMed: 9472515]
- 62.
- Flynn TN, Louviere JJ, Peters TJ, Coast J. Best-worst scaling: what it can do for health care research and how to do it. J Health Econ. 2007;26(1):171-189. doi:10.1016/j.jhealeco.2006.04.002 [PubMed: 16707175] [CrossRef]
- 63.
- Max-diff Technical Paper. Version 8. Sawtooth Software; 2013.
- 64.
- Mamede S, Schmidt HG. The twin traps of overtreatment and therapeutic nihilism in clinical practice. Med Educ. 2014;48(1):34-43. doi:10.1111/medu.12264 [PubMed: 24330115] [CrossRef]
- 65.
- Otis JD, Sanderson K, Hardway C, Pincus M, Tun C, Soumekh S. A randomized controlled pilot study of a cognitive-behavioral therapy approach for painful diabetic peripheral neuropathy. J Pain. 2013;14(5):475-482. doi:10.1016/j.jpain.2012.12.013 [PubMed: 23452825] [CrossRef]
Acknowledgments
Funding for this work was supported through a Patient-centered Outcomes Research Institute Assessment of Prevention, Diagnosis, and Treatment Options Program Award (CE-1304-7250). Drs Adams and Schmittdiel are also supported by a grant from the Division of Diabetes Translation, Centers for Disease Control and Prevention (U58 DP002641). Dr Grant is supported by the National Institute for Diabetes and Digestive and Kidney Diseases (R01 DK099108); National Heart, Lung, and Blood Institute (R01 HL117939); and PCORI (SC14-1403-11992).
Research reported in this report was [partially] funded through a Patient-Centered Outcomes Research Institute® (PCORI®) Award (#CE-1304-7250) Further information available at: https://www.pcori.org/research-results/2013/automated-tracking-symptoms-and-treatment-side-effects-help-patients-starting
Appendices
Appendix A.
DPN-Intervention Call Scripts (PDF, 254K)
Appendix B.
Sample Preference Survey (PDF, 96K)
Appendix C.
Suggested citation:
Adams A, Altschuler A, Bayliss E, et al. (2019). Automated Tracking of Symptoms and Treatment Side Effects to Help Patients Starting New Medicines for Diabetes-Related Nerve Pain. Patient-Centered Outcomes Research Institute (PCORI). https://doi.org/10.25302/5.2019/CE.13047250
Disclaimer
The [views, statements, opinions] presented in this report are solely the responsibility of the author(s) and do not necessarily represent the views of the Patient-Centered Outcomes Research Institute® (PCORI®), its Board of Governors or Methodology Committee.
- NLM CatalogRelated NLM Catalog Entries
- PMCPubMed Central citations
- PubMedLinks to PubMed
- Folic acid supplementation and malaria susceptibility and severity among people taking antifolate antimalarial drugs in endemic areas.[Cochrane Database Syst Rev. 2022]Folic acid supplementation and malaria susceptibility and severity among people taking antifolate antimalarial drugs in endemic areas.Crider K, Williams J, Qi YP, Gutman J, Yeung L, Mai C, Finkelstain J, Mehta S, Pons-Duran C, Menéndez C, et al. Cochrane Database Syst Rev. 2022 Feb 1; 2(2022). Epub 2022 Feb 1.
- Automated symptom and treatment side effect monitoring for improved quality of life among adults with diabetic peripheral neuropathy in primary care: a pragmatic, cluster, randomized, controlled trial.[Diabet Med. 2019]Automated symptom and treatment side effect monitoring for improved quality of life among adults with diabetic peripheral neuropathy in primary care: a pragmatic, cluster, randomized, controlled trial.Adams AS, Schmittdiel JA, Altschuler A, Bayliss EA, Neugebauer R, Ma L, Dyer W, Clark J, Cook B, Willyoung D, et al. Diabet Med. 2019 Jan; 36(1):52-61. Epub 2018 Nov 7.
- The Diabetes Telephone Study: Design and challenges of a pragmatic cluster randomized trial to improve diabetic peripheral neuropathy treatment.[Clin Trials. 2016]The Diabetes Telephone Study: Design and challenges of a pragmatic cluster randomized trial to improve diabetic peripheral neuropathy treatment.Adams AS, Bayliss EA, Schmittdiel JA, Altschuler A, Dyer W, Neugebauer R, Jaffe M, Young JD, Kim E, Grant RW, et al. Clin Trials. 2016 Jun; 13(3):286-93. Epub 2016 Mar 31.
- Review Behavioral and Pharmacotherapy Weight Loss Interventions to Prevent Obesity-Related Morbidity and Mortality in Adults: An Updated Systematic Review for the U.S. Preventive Services Task Force[ 2018]Review Behavioral and Pharmacotherapy Weight Loss Interventions to Prevent Obesity-Related Morbidity and Mortality in Adults: An Updated Systematic Review for the U.S. Preventive Services Task ForceLeBlanc EL, Patnode CD, Webber EM, Redmond N, Rushkin M, O’Connor EA. 2018 Sep
- Review Interactive telemedicine: effects on professional practice and health care outcomes.[Cochrane Database Syst Rev. 2015]Review Interactive telemedicine: effects on professional practice and health care outcomes.Flodgren G, Rachas A, Farmer AJ, Inzitari M, Shepperd S. Cochrane Database Syst Rev. 2015 Sep 7; 2015(9):CD002098. Epub 2015 Sep 7.
- Automated Tracking of Symptoms and Treatment Side Effects to Help Patients Start...Automated Tracking of Symptoms and Treatment Side Effects to Help Patients Starting New Medicines for Diabetes-Related Nerve Pain
Your browsing activity is empty.
Activity recording is turned off.
See more...