NCBI Bookshelf. A service of the National Library of Medicine, National Institutes of Health.
IARC Working Group on the Evaluation of Cancer-Preventive Interventions. Absence of excess body fatness. Lyon (FR): International Agency for Research on Cancer; 2021. (IARC Handbooks of Cancer Prevention, No. 16.)
1.1. Background and definitions
1.1.1. Scientific definitions of obesity
Obesity is the abnormal or excessive accumulation of body fat that results from energy imbalance, i.e. energy intake exceeding energy expenditure, and presents a risk to health. Obesity is both a condition and an important risk factor for other noncommunicable diseases, including diabetes, cardiovascular disease, and many types of cancer.
Overweight and obesity in humans are often classified by the body mass index (BMI), which is obtained by dividing the body weight (in kilograms) by the square of the height (in metres).
In adults, overweight is defined as BMI ≥ 25 kg⁄m2 and obesity as BMI ≥ 30 kg⁄m2 (WHO, 2000). Obesity can be further classified, by level of severity and the corresponding different medical approaches for treatment, into class I (30–34.9 kg⁄m2), class II (35–39.9 kg⁄m2), and class III (≥ 40 kg⁄m2) obesity (Table 1.1). In children younger than 5 years, overweight is defined as a weight-for-height more than 2 standard deviations (SD) above the WHO Child Growth Standards median, and obesity is often defined as a weight-for-height more than 3 SD above the WHO Child Growth Standards median. In children and adolescents from age 5 years to younger than 19 years, overweight and obesity are defined as a BMI-for-age more than 1 SD and more than 2 SD, respectively, above the WHO Growth Reference median (WHO, 2016, 2017a, b).
Table 1.1
Overweight and obesity cut-off values.
1.1.2. Cultural definitions of obesity
Perceptions of overweight and obesity can vary across different settings and populations. People who are overweight or obese may be admired or may be stigmatized, depending on contextual, cultural, spiritual, and other relevant factors.
It is well documented that in many populations, overweight and obese people are discriminated against and stigmatized because of their physical appearance – in interpersonal settings, in the workplace, and in health-care settings (Link & Phelan, 2001; Puhl & Brownell, 2001; Sikorski et al., 2011; Spahlholz et al., 2016). Stigmatization of children is also common, leading to rejection and harassment, especially in educational settings (Puhl & Brownell, 2001).
In contrast, in cultures where overweight and obesity in children are viewed favourably, an obese child may be seen as having a healthy body status (Peña et al., 2012) and as a reflection of good parenting (Brown, 1991). Favourable perceptions of increased body weight can also lead to inaccurate interpretations of a “healthy” body weight when parents assess the weight of their children. In a systematic review based on 13 studies, 13% to 100% of the parents interviewed underestimated the obesity status of their child, and in six studies 70% or more of the parents were unable to identify their child as overweight (Tompkins et al., 2015). In cultures that favour larger body size, women of reproductive age who are overweight may be perceived as having been well cared for and, by extension, will care for their children in a similar manner. In such cultures, increased body weight can be linked to favourable qualities such as self-worth, health, prosperity, and maternity. For men, overweight and obesity can symbolize (in addition to good health) economic success, political power, or social status, and in some societies the power of the elders or leaders (Brown, 1991).
Positive perceptions of large body size in cancer survivors have been reported among cancer care clinicians. Until the past few decades, cancer was frequently diagnosed at a late stage of the disease, in which weight loss was a major diagnostic sign. However, with early detection programmes, many cancer survivors are overweight and obese and suffer obesity-related comorbidities (e.g. diabetes). In a weight-loss feasibility trial in overweight patients with colorectal cancer, Anderson et al. (2010) reported that clinicians describe avoidance of weight loss as desirable and express concern about reported weight loss (even intentional weight loss). In a study of colorectal clinicians in the United Kingdom and Ireland, Anderson et al. (2013) reported that current opinion and practice are influenced by the lack of evidence for the impact of weight management on health, and by a belief that weight gain is good and weight loss is bad in the cancer setting.
1.1.3. Obesity as a public health problem
Until recently, obesity was perceived as being an issue of an individual’s behaviour. As a result, interventions for the prevention and treatment of obesity were focused on the individual level (Caballero, 2007). This perception has changed with the increasing awareness of the influence of external environmental factors on obesity (e.g. the built environment, the marketing of food and beverages), and obesity is now seen as a health problem that demands a social response (Opalinski, 2013); this is recognized both by the public and by health-care professionals (Obesity Society, 2014; see Sections 1.3.6 and 1.3.7).
1.2. Prevalence and trends
1.2.1. Prevalence and trends in adults
Worldwide, in 2014 more than 640 million adults (14% of adults) were obese, 6 times the number in 1975; of those, more than 18% lived in high-income English-speaking countries, and 13.9% lived in the Middle East and North Africa (NCD Risk Factor Collaboration, 2016).
From 1975 to 2014, the average weight of the population increased in all world regions; during those four decades, the global prevalence of underweight decreased and the global prevalence of obesity increased, so that the number of obese adults surpassed the number of underweight adults (NCD Risk Factor Collaboration, 2016). The global average BMI in the adult population (≥ 18 years) in 1975 was 21.7 kg/m2 in men and 22.1 kg/m2 in women, and by 2014 these averages had increased to 24.2 kg/m2 in men and 24.4 kg/m2 in women, according to the latest available estimates (NCD Risk Factor Collaboration, 2016). This means that between 1975 and 2014, the average weight of a man with a height of 170 cm increased by about 7 kg, and that of a woman with a height of 160 cm increased by about 6 kg. This general increase in the average BMI affected both high-income countries and low- and middle-income countries.
The overall increase in the average BMI corresponded to a general increase in the prevalence of obesity. In 2014, the global prevalence of obesity in the adult population was 10.8% in men and 14.9% in women, ranging from less than 1% (Burundi) to almost 50% (Cook Islands and French Polynesia) in men, and from less than 3% (Timor-Leste and Japan) to more than 58% (American Samoa) in women (NCD Risk Factor Collaboration, 2016; Fig. 1.1). In 2014, the prevalence of obesity was lowest in low-income countries, whereas lower-middle-, upper-middle-, and high-income countries were characterized by a high level of heterogeneity; in countries in any of these three income categories, the highest prevalence of obesity was more than 40% in men and about 55% in women. In general, the prevalence of obesity was higher in women than in men, but trends over the four decades suggested a greater increase in the prevalence of obesity in men. However, it should be noted that despite the worldwide increase in the prevalence of obesity and the fact that the number of obese adults is now higher that the number of underweight adults, the proportion of the population that is underweight is still very high (> 20%) in some countries (India and Bangladesh) (NCD Risk Factor Collaboration, 2016).
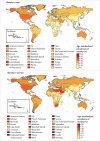
Fig. 1.1
Prevalence of obesity (BMI ≥ 30 kg/m2) by sex and country in 2014.
In light of the obesity epidemic, there is an increasing interest in understanding weight-loss strategies in the population. A study of university students in 22 countries in different regions of the world suggested that the prevalence of trying to lose weight increases with levels of BMI, and is higher in women than in men at any given BMI level (Wardle et al., 2006). Similarly, figures from a survey of European Union citizens aged 15 years and older showed that 34% of people reported changing their eating and drinking behaviour to lose weight, a percentage that increased to 48% among those who perceived their weight as being too high (European Commission, 2006).
With earlier diagnosis and improved cancer treatments, many cancer survivors have similar BMI levels to those of the general population; in addition, some cancer treatments may induce weight gain. Data on BMI distribution by cancer diagnosis are not routinely reported. It was found that 47.3% of patients with breast cancer had a BMI greater than 25 kg/m2 (Nichols et al., 2009), and in a cohort of colorectal cancer survivors, 29.1% were obese (Rohan et al., 2015). Gross et al. (2015) reported that breast cancer survivors gained weight at a higher rate than their cancer-free peers. The implications of high body mass for treatment dosing, subsequent morbidity, and recurrence are not fully understood (see Section 2.4).
1.2.2. Prevalence and trends in children and adolescents
Recent estimates have shown a rapid rise in the prevalence of overweight and obesity in children and adolescents worldwide; however, trend analyses in developed countries have shown a tendency for the prevalence to stabilize starting from mid-2000 (Ng et al., 2014; UNICEF, WHO, and World Bank Group, 2015). The long-term impact of obesity during childhood and adolescence is a higher risk of obesity during adulthood (Guo & Chumlea, 1999; Freedman et al., 2005; Singh et al., 2008).
The WHO European Childhood Obesity Surveillance Initiative (COSI), established in 2007 to monitor changes in the prevalence of overweight (including obesity) in primary schoolchildren, reported that in 2009–2010 the prevalence of overweight ranged from 18% to 57% in boys and from 18% to 50% in girls; 6–31% of boys and 5–21% of girls were obese (Wijnhoven et al., 2014). A recent position statement from the European Association for the Study of Obesity (EASO) Childhood Obesity Task Force (COTF) classified obesity as a chronic disease in children and adolescents (Farpour-Lambert et al., 2015).
In 2013, about 110 million children and adolescents worldwide aged 2–19 years (prevalence of 4.7%) were obese (IHME, 2014), almost twice the number in 1980. In 2013, 24% of boys and 22% of girls living in high-income countries were overweight or obese, whereas the corresponding percentages observed in low- and middle-income countries were about 13% for boys and girls (Ng et al., 2014). The highest prevalence of obesity in children and adolescents was observed in North America (Canada and the USA) for both boys (12.1%) and girls (13.0%). The next-highest prevalences were seen in southern Latin America for boys (~10%) and in North Africa and the Middle East for girls (~10%). The lowest prevalence was observed in South Asia for both sexes. In 2013, the two countries with the highest prevalence of obesity for both boys and girls were Kiribati and Samoa, where 20% of boys and 33% of girls were obese; similar prevalences were observed in Qatar for boys and in the Federated States of Micronesia for girls, whereas prevalences were less than 1.5% for boys and girls in Bangladesh and the Republic of Korea.
Independently of the level of income, sex differences in the prevalence and trends of overweight and obesity were small (Ng et al., 2014). Nevertheless, the prevalence of obesity was higher in boys than in girls in 70% of low-income countries;, and in 63% of high-income countries; the corresponding percentage in lower-middle- and upper-middle-income countries was 33% (IHME, 2014; Ng et al., 2014).
According to estimates from the United Nations Children’s Fund (UNICEF), WHO, and the World Bank Group, the number of overweight children younger than 5 years has been increasing steadily everywhere in the world; in 2014, almost 50% of all overweight children younger than 5 years lived in Asia, and about 25% lived in Africa (UNICEF, WHO, and World Bank Group, 2015).
Similarly to findings for adult cancer survivors, a study of paediatric cancer survivors reported that boys aged 6–11 years were more likely to be overweight than the general population (Nathan et al., 2006).
1.2.3. Prevalence by age and sex
Several studies in the USA and Europe, using cross-sectional data, have shown that BMI increases with age up to the sixth decade of life and then starts to decrease (Flegal et al., 2002; Vasan et al., 2005; Ogden et al., 2006). However, results from cohort studies suggest a more modest decline in BMI at older ages (Grinker et al., 1995). This difference in findings may be due to higher mortality rates at younger ages in the obese population, leading to a lower prevalence of obesity in the surviving older population. Ageing is also associated with a change in body composition, with a decline in fat-free mass, which may cause a decrease in weight, and therefore in the measured obesity (Villareal et al., 2005). [The validity of assessing obesity by BMI is limited in elderly people (see Section 1.4.2).]
The prevalence of obesity and its association with age vary widely across countries, showing both similarities and differences regardless of the socioeconomic level of the country (IHME, 2014). For example, low prevalences of obesity are observed at all ages in China, Ethiopia, India, and Japan (Fig. 1.2), whereas in Colombia, France, the Islamic Republic of Iran, New Zealand, the Russian Federation, and the USA the prevalence increases with age, followed by a decrease later in life.

Fig. 1.2
Prevalence of obese population by age and sex in selected countries.
The difference between the prevalence of obesity in men and in women is only weakly associated with the level of socioeconomic development of the country. The prevalence of obesity is markedly higher in women than in men in Colombia, the Islamic Republic of Iran, and the Russian Federation, whereas a smaller difference or no difference is observed, for example, in China, Ethiopia, France, India, Japan, New Zealand, and the USA.
1.2.4. Prevalence by ethnicity
Several studies have examined the association between ethnicity and obesity, mainly in high-income countries with high levels of immigration (e.g. the United Kingdom and the USA), but very little evidence is available for low- and middle-income countries.
Studies in the USA have clearly shown differences in health between different ethnic groups (Murray et al., 2005; Caprio et al., 2008; Ogden et al., 2014). The prevalence of obesity in the adult population (≥ 20 years) was almost 50% in non-Hispanic Blacks (37.1% in men and 56.6% in women), more than 42% in Hispanics (40.1% in men and 44.4% in women), about 32% in non-Hispanic Whites, and almost 11% in non-Hispanic Asians (Ogden et al., 2014). The prevalence of obesity in children and adolescents (2–19 years) was 22.4% in Hispanics, 20.2% in non-Hispanic Blacks, 14.1% in non-Hispanic Whites, and 8.6% in non-Hispanic Asians (Ogden et al., 2014). Similarly, important ethnic differences have been observed in England (National Obesity Observatory, 2011). According to data from the Health Survey for England 2004, in women the prevalence of obesity was highest for Black African women (38%), followed by Black Caribbean (32%) and Pakistani (28%) women, and lowest in Chinese women (6%). The largest gap between the sexes was observed among the Black African, Pakistani, and Bangladeshi groups, in which the prevalence of obesity in women was 1.9–2.8 times that in men. In addition, data from the National Child Measurement Programme in England in the 2012–2013 school year compared the prevalence of obesity in children aged 4–5 years and 10–11 years. The prevalence of obesity was highest among “Asian or Asian British”, “Black or Black British”, and “Other than White, Chinese, and Mixed” children, with levels of 20–30% (Health and Social Care Information Centre, 2013). [These differences may be partly due to differences in socioeconomic status.]
A study of the ethnic differences in obesity among immigrants to Norway from low- and middle-income countries showed large variability in general adiposity among different ethnicities (Kumar et al., 2006). Whereas 50% of Turkish women were obese, the levels of other anthropometric indicators, such as waist circumference, were higher among the Pakistani and Sri Lankan groups, and these differences persisted after adjustment for sociodemographic, biological, and lifestyle factors.
As a way of avoiding biases when comparing data, it has been suggested to define different obesity cut-off values when comparison between ethnicities is performed (see Section 1.4.2(ii)).
1.2.5. Prevalence by social class and education
The association between socioeconomic status and prevalence of overweight and obesity changes according to the level of economic development of the country (Dinsa et al., 2012). Lower socioeconomic status is protective against obesity in low-income countries, whereas it is a risk factor for obesity in middle- and high-income countries, mostly in women (Monteiro et al., 2004a, b; Dinsa et al., 2012). Similar patterns have been observed in children. Studies in high-income countries have shown an inverse association between socioeconomic status and child obesity (Lamerz et al., 2005; Shrewsbury & Wardle, 2008; Due et al., 2009). For example, data from the National Child Measurement Programme in England in the 2012–2013 school year showed a positive association [increasing deprivation leads to increasing prevalence] between level of deprivation and prevalence of obesity in children aged 4–5 years and 10–11 years (Fig. 1.3). Using the deciles of the Index of Multiple Deprivation 2010 (Department for Communities and Local Government, 2011), children in the most deprived decile had a prevalence of obesity twice that observed in the least deprived decile, with a prevalence of about 10% in children aged 4–5 years and about 20% in children aged 10–11 years (National Obesity Observatory, 2012; Health and Social Care Information Centre, 2013). In contrast, in low-income countries the association is inverted, and children in affluent groups are more likely to be obese (Dinsa et al., 2012; Lissner et al., 2016).

Fig. 1.3
Prevalence of obesity by age and decile of Index of Multiple Deprivation in England.
As is the case for the socioeconomic level, BMI has been shown to be positively associated with education in lower-middle-income countries in women, and for men also in middle-income countries, whereas in high-income countries an inverse association was observed between level of education and BMI in women and no association was seen in men (Di Cesare et al., 2013; Fig. 1.4; see also Section 1.3.7).
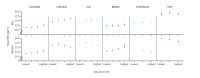
Fig. 1.4
Mean BMI by education level in adults aged 25–64 years in selected countries.
1.3. Risk factors
1.3.1. Regulation of hunger and satiety
The current epidemic of obesity suggests that the processes of satiety and body weight control are not tightly regulated, and physiological systems appear to permit fat storage when humans are exposed to sensory stimulation (e.g. palatability and taste) and opportunities for high energy intakes (hedonic response) (Blundell & Finlayson, 2004). In addition, a range of psychological factors can affect appetite control, including stress, mood, and guilt. Polymorphisms in the fat mass and obesity-associated gene (FTO) are associated with increased food intake, which is thought to be due to the effect of the gene on ghrelin and its role in appetite control (Eissing, 2013; Benedict et al., 2014). Thus, it seems that alterations in appetite control and subsequent food intake (rather than metabolic effects) are important factors for positive energy balance in humans.
The hypothalamus is the main regulatory organ for human appetite; peripheral signals from adipose tissue and the gastrointestinal system are delivered to the hypothalamus to influence short-term food intake (Lean & Malkova, 2015) (see also Sections 4.3.4a and 4.3.6d). Of the several adipokines produced by white adipose tissue, only leptin appears to have a significant effect on appetite suppression (Blundell et al., 2015a, b); in contrast, more than 30 gut-derived peptides, including hormones and neuropeptides, are known to affect appetite (Lean & Malkova, 2015). Meal ingestion results in production of hormones from enteroendocrine cells found at multiple sites in the digestive system (stomach, proximal/distal small intestine, pancreas, and colon). Gut hormones inhibit or stimulate food intake (anorexigenic or orexigenic effects). Key anorexigenic gut peptides include glucagon-like peptide-1 (GLP-1) and peptide YY (PYY), whereas ghrelin is a gut hormone with potent orexigenic effects. Interest in the effect of bariatric surgery on long-term appetite control has highlighted the role of gut hormones (Chakravartty et al., 2015). Lean & Malkova (2015) reported that diet-induced weight loss affects gut hormones and favours increased appetite (and therefore weight regain), whereas physical exercise favours enhanced satiety (supporting maintenance of weight loss). They postulated that the sustained weight loss achieved by bariatric surgery may in part be mediated via favourable changes to gut hormones.
There has been much speculation about the concept of a physiological “set point” that is automatically adjusted to maintain weight. Recent evidence suggests that structural changes in the hypothalamus may lead to a “reset”, and thus maintain an increased body weight.
1.3.2. Weight gain throughout the life-course
The association of noncommunicable diseases in adulthood with nutrient status during pregnancy was proposed in 1990 (Barker, 1990), and there is substantial evidence supporting an early-life origin of susceptibility to obesity in later life (Scientific Advisory Committee on Nutrition, 2011). Both prenatal and postnatal factors, influencing fetal and infant growth, development, birth weight, and programming of metabolism, have been implicated in the later development of obesity. Overall, it is difficult to disentangle the impact of single early-life risk factors in the development of adult obesity.
Maternal obesity is associated with both large-for-gestational-age babies and macrosomia (birth weight > 90th percentile). It has been reported that in women with a pre-pregnancy BMI greater than 30 kg/m2, the likelihood of having a large-for-gestational-age baby is 3.75 times that in women with a BMI less than 30 kg/m2, and that large-for-gestational-age babies have both higher insulin resistance and higher free fatty acid levels (Liu et al., 2013). There is global concern about the number of women in developing countries who enter pregnancy with excess body weight (WHO, 2011; Torloni et al., 2012).
Systematic reviews of studies mainly in developed countries have demonstrated a positive association between birth weight and BMI in later life. The association has been described as strongest in children and young adults and weaker in middle-aged adults. It has been estimated that a 1 kg increment in birth weight is associated with a rise of 0.5–0.7 kg/m2 in the BMI of young adults (Scientific Advisory Committee on Nutrition, 2011). Some studies suggest that both low and high birth weight are associated with subsequent risk of obesity in children and young adults; however, a meta-analysis of 66 studies from 26 countries shows that low birth weight (< 2500 g) is followed by a decreasing long-term risk of overweight, whereas high birth weight predisposes to being overweight in later life (Schellong et al., 2012).
The role of maternal diet (both quantity and quality) during pregnancy is of particular interest with respect to fetal developmental programming (Liu et al., 2013), and it is plausible that epigenetic mechanisms are responsible for the associations between birth weight and risk of overweight later in life (Dominguez-Salas et al., 2014; Tobi et al., 2014).
Weight trajectories during infancy are influenced by breastfeeding and complementary feeding practices, which in turn influence body fatness in childhood and growth patterns (Poskitt & Breda, 2012; Bagci Bosi et al., 2016). There is growing evidence from recent reviews of observational studies that breastfeeding is associated with a decreased risk of becoming overweight later in life (Weng et al., 2012; Horta et al., 2015). Horta et al. (2015) reported an odds ratio (OR) of 0.74 (95% confidence interval [CI], 0.70–0.78) that people who were breastfed as babies were less likely to be classified as overweight or obese at ages 1–9 years, 10–19 years, and 20 years and older.
Feeding choices during infancy and childhood are influenced by parental factors, food availability, and other socioeconomic factors, including cultural norms, peer effects, and the marketing of food and beverages (Boyland et al., 2016). However, current research findings on the type of complementary foods and the age at which complementary foods are introduced into the infant diet and subsequent body fatness are inconsistent (Langley-Evans, 2015).
The development of overweight during childhood appears to track into adult life (Brisbois et al., 2012); however, a systematic review showed that childhood BMI is not a good predictor of the incidence of adult obesity or overweight, because most obese adults were not obese in childhood (Simmonds et al., 2015). Observational studies in industrialized countries have shown a consistent association of poor early growth followed by rapid catch-up in infancy with later obesity in childhood and adulthood (Monasta et al., 2010). An early adiposity rebound (a period of increasing BMI after the early childhood nadir), which usually occurs at ages 4–8 years, is associated with high body weight in later life (Williams & Goulding, 2009; Brisbois et al., 2012). Nutritional stunting (usually caused by chronic undernutrition) during childhood is also positively associated with overweight and obesity in later life (Popkin et al., 1996; Black et al., 2013).
Several studies have reported an association between parental smoking and later obesity in the offspring, with the greatest effect from maternal smoking (Power et al., 2010; Dior et al., 2014; Wang et al., 2014; Han et al., 2015). Prenatal maternal exposure to smoking (including secondhand tobacco smoke) was associated with increased risk of obesity in adolescents, independent of birth weight (Wang et al., 2014).
Throughout adult life, there are further periods or key life events associated with weight gain, including student life, the transition from single status to marriage or cohabitation, diet and activity behaviours during pregnancy, postpartum weight retention, the demands of employment, unemployment and job loss, the demands of parenting, and physiological changes associated with the ageing process (including menopause) (WHO Regional Office for Europe, 2016). Maternal weight gained during pregnancy is also associated with the likelihood of weight retention in the mother in the postpartum period and beyond. It is estimated that in the USA 50–67% of women gain more weight during pregnancy than is considered desirable by the United States Institute of Medicine (now known as the National Academy of Medicine) (Liu et al., 2013). It is likely that the metabolic load of exclusive breastfeeding (~500 kcal per day) can help women in losing the weight gained during pregnancy, although results have been inconsistent (Bobrow et al., 2013; Jarlenski et al., 2014; Neville et al., 2014; Palmer et al., 2015).
Physiological changes associated with the ageing process influence weight gain (see Section 1.2.3). Comorbidities also have the potential to increase weight gain, for example through the action of different treatment modalities (Leslie et al., 2007; Rummel-Kluge et al., 2010; McPheeters et al., 2011; Almandil et al., 2013). Unintentional weight loss has also been reported as a side-effect of several therapeutic agents, and this is usually dose-dependent (Leslie et al., 2007).
1.3.3. Excessive energy intake
(a) Total energy intake
Obesity is determined primarily by increased total energy intake (Vandevijvere et al., 2015), which itself is influenced by portion size, the frequency of consumption, and the energy density of food and drinks consumed (Ello-Martin et al., 2005). Assessing total energy intake is challenging in epidemiological studies, because of measurement error, including selective underreporting by overweight and obese people (Livingstone & Black, 2003) and the inability to quantify energy provided by the colonic microbiome (Rahat-Rozenbloom et al., 2014). Because objective measures of energy intake do not exist (de Jonge et al., 2007), the best long-term indicator (or life-course indicator) of positive energy balance is weight gain (Hill et al., 2012). Weight gain cannot differentiate between the proportions of fat and lean tissues gained, but in most adults it is primarily determined by gain in adipose tissue (Okorodudu et al., 2010). The amount of adipose tissue gained over time depends on the degree of positive energy balance, the duration of the energy surplus, and the body composition before weight gain (Schutz et al., 2014).
(b) Macronutrient composition of diet
(i) Total fat
There has been a lack of consensus about whether the macronutrient composition of the diet is a significant determinant of body fatness (Bray & Popkin, 1998; Willett, 2002). Two systematic reviews of observational studies found no significant evidence for an association between percentage of energy from fat and body weight (Fogelholm et al., 2012; Hooper et al., 2015), whereas two systematic reviews with meta-analyses of randomized controlled trials (RCTs) in people with no intention of losing weight suggest that the percentage of energy from fat is positively associated with body weight (Hooper et al., 2015; Tobias et al., 2015). However, in weight-loss trials, no difference in weight loss was observed between diets with different percentages of energy from fat, whereas diets with a low percentage of energy from carbohydrates led to greater weight loss than those with a low percentage of energy from fat (Tobias et al., 2015). A third meta-analysis of RCTs reported that diets with a low percentage of energy from fat and those with a low percentage of energy from carbohydrates resulted in comparable reductions in body weight (Hu et al., 2012). [It is recognized that the success of any dietary restriction regimen in achieving weight loss is dependent on compliance with that regimen.]
(ii) Sugars
Two systematic reviews and meta-analyses of RCTs in adults found a significant positive association between intake of free sugars (defined as monosaccharides and disaccharides added to foods and beverages by the manufacturer, cook, or consumer, and sugars naturally present in honey, syrups, fruit juices, and fruit juice concentrates; WHO, 2015) and weight gain (Te Morenga et al., 2012) and energy intake (Scientific Advisory Committee on Nutrition, 2015), respectively.
Overconsumption of sugar-sweetened beverages in particular has been linked to weight gain and obesity. Reviews and meta-analyses of prospective cohort studies and RCTs indicate that consumption of sugar-sweetened beverages promotes weight gain and obesity (Hu & Malik, 2010; Te Morenga et al., 2012; Malik et al., 2013; Fardet & Boirie, 2014; Scientific Advisory Committee on Nutrition, 2015). In contrast, there are investigations that consistently show weak or null results for the association between consumption of sugar-sweetened beverages and obesity (Forshee et al., 2008; Gibson, 2008; Kaiser et al., 2013; Trumbo & Rivers, 2014). [Concern has been expressed about potential bias of these studies, because of sources of funding.]
(c) Dietary patterns
Studies using dietary patterns as derived by factor or cluster analysis to examine common eating behaviour in relation to weight control and obesity found that diets characterized by high intakes of sugar-sweetened beverages, refined grains, potatoes, and red and processed meat were related to greater gains in BMI and waist circumference, whereas diets typified by high intakes of fruit, vegetables, whole grains, fish, poultry, and reduced-fat dairy products were associated with smaller weight gains (Newby et al., 2003; Schulze et al., 2006). Similarly, the traditional Mediterranean diet – as characterized by high intakes of fruit, vegetables, legumes, nuts/seeds, and wholegrain cereals; regular consumption of fish and seafood; moderate intakes of dairy products, poultry, and eggs; low consumption of red and processed meat; use of olive oil as the main source of dietary fat; and moderate intake of wine – has been consistently found to be inversely related to the development of weight gain and obesity (Buckland et al., 2008; García-Fernández et al., 2014; Gotsis et al., 2015). It has been suggested that eating patterns, such as eating breakfast, frequency of eating, snacking, and timing of meals, may also modulate the risk of overweight and obesity (van der Heijden et al., 2007; McCrory, 2014; Jiang & Turek, 2017).
(d) Fast food
Fast foods are energy-dense, micronutrient-poor foods that are often high in fatty acids, processed starches, and added sugars (Jaworowska et al., 2013); they have more recently been termed “ultra-processed foods” (Martínez Steele et al., 2016). There is concern about the rising global trend in consumption of fast food and its potential impact on obesity (McCrory et al., 1999; Paeratakul et al., 2003; Louzada et al., 2015).
Most available studies are limited by their cross-sectional designs, non-standardized definitions of fast food, and potential confounding by unmeasured factors. A recent narrative review reported that frequent consumption of fast foods is accompanied by weight gain and obesity (Bahadoran et al., 2016). In one observational cohort study, frequency of consumption of fast food was positively related to changes in body weight. Specifically, more frequent consumers (more than twice per week) gained an extra 4.5 kg of body weight over a 15-year period compared with less frequent consumers (less than once per week) of fast-food products (Pereira et al., 2005).
(e) Alcoholic beverages
Findings on the association between consumption of alcoholic beverages and body weight have been inconsistent, with observational studies showing positive, inverse, or null associations (reviewed in Poppitt, 2015). In addition, evidence linking alcohol consumption to weight gain and obesity was ranked insufficient by international reports (WHO, 2003; WCRF/AICR, 2007).
(f) Sleep
The relationship of sleep duration to adiposity has been examined in numerous epidemiological studies. Results from studies in adults have been mixed, but most investigations support an association of short sleep duration with adiposity, with the association appearing to wane with increasing age (Marshall et al., 2008; Patel & Hu, 2008). By comparison, studies in children showed a clear pattern, with short sleep duration being positively related to obesity (Chen et al., 2008; Marshall et al., 2008; Patel & Hu, 2008). Results from systematic reviews and meta-analyses are in line with these findings (Cappuccio et al., 2008; Magee & Hale, 2012; Capers et al., 2015). [One possible explanation for the divergent results between children and adults is that the association between short sleep duration and weight gain diminishes over time after the transition to chronic short sleep duration (Magee & Hale, 2012).]
Sleep deprivation can lead to enhanced energy intake, possibly through a mechanism involving greater propensity to overeat and altered levels of hormones involved in appetite regulation, such as ghrelin and leptin (Bayon et al., 2014; Kim et al., 2015). By comparison, there is little evidence to support that insufficient sleep leads to decreased energy expenditure (Chaput & Tremblay, 2012). Light exposure during the night decreases melatonin synthesis (Haus & Smolensky, 2013; McFadden et al., 2014), and melatonin deficiency has been shown to exert beneficial effects on obesity and to normalize the expression and secretion patterns of adipokines such as leptin and adiponectin (Szewczyk-Golec et al., 2015).
[Limitations of study designs in the area of short sleep duration and obesity include a failure to account for potential confounding, mediating, and moderating variables, such as sedentary behaviour, and measurement issues such as a paucity of objective measures of sleep duration, lack of repeated assessments of both sleep duration and body weight, and an insufficient number of experimental study designs that manipulate sleep.]
1.3.4. Endocrine disruptors
An endocrine-disrupting chemical (EDC) is an exogenous chemical, or mixture of chemicals, that interferes with any aspect of the regulation of hormone action (Zoeller et al., 2012). EDCs act directly by binding or interfering with receptors, or indirectly by disrupting hormone levels or by altering hormonal transport mechanisms (Heindel et al., 2015). Because endocrine systems exhibit tissue-, cell-, and receptor-specific actions during the life-cycle, EDCs can produce a complex mosaic of effects across the life span (Zoeller et al., 2012).
Some EDCs, referred to as “obesogens” (Janesick & Blumberg, 2016), improperly interfere with lipid homeostasis, and promote adipogenesis by perturbing various endocrine axes (Burgio et al., 2015). The developmental age at which EDC exposures occur is a critical consideration in understanding their effects. Exposure to obesogenic EDCs during fetal life and early childhood appears to have an impact on obesity during childhood and adulthood (Grün & Blumberg, 2009; Heindel et al., 2015).
The EDCs with obesogenic properties that are most prevalently used are presented in Table 1.2 (adapted from Heindel et al., 2015), and the available data are summarized below. [The overall limitations of the data from humans lie in the cross-sectional nature of the majority of the studies and in the use of a single measure of exposure. The inconsistences in results in the small number of birth cohort studies is likely to be related to the small sample size, differences in population characteristics, and differences in levels of exposure and timing of exposure.]
Table 1.2
Endocrine-disrupting chemicals with obesogenic properties.
(a) Bisphenol A
Studies in animals have shown that exposure to bisphenol A can disrupt many metabolic pathways and could alter the hypothalamic energy balance circuitry, resulting in increased susceptibility to developing diet-induced obesity and metabolic impairment (Heindel et al., 2015). Some studies reported an association between urinary concentrations of bisphenol A and increased obesity in children (OR, 2.53; 95% CI, 1.72–3.74, between the highest and lowest quartile of bisphenol A concentration; Trasande et al., 2012) and in adults (OR, 1.50; 95% CI, 1.15–1.97, between the highest and lowest quartile) (Wang et al., 2012), whereas other studies reported no association (Lakind et al., 2014). Also, exposure to bisphenol A during fetal life increased adipokine levels in childhood (Volberg et al., 2013; Ashley-Martin et al., 2014).
(b) Phthalates
In animal models, prenatal exposure to phthalates led to increased body weight, increased number and size of adipocytes, and activation of peroxisome proliferator-activated receptor gamma in male offspring (Heindel et al., 2015). Also, cross-sectional studies suggest an association between exposure to phthalates and weight gain (Kim & Park, 2014). However, data from human birth cohorts are inconsistent (Ashley-Martin et al., 2014; Maresca et al., 2015).
(c) Tributyltin
In animals, prenatal exposure to tributyltin causes lipid accumulation in adipose tissue, with effects persisting into adulthood and into future generations (Heindel et al., 2015). A recent study showed a positive association between placenta tributyltin levels and weight gain in male infants at age 3 months (Rantakokko et al., 2014).
(d) Polychlorinated biphenyls and other persistent organic pollutants
Data from the National Health and Nutrition Examination Survey 1999–2002, in adults and children, showed an association of detectable levels of persistent organic pollutants with waist circumference and BMI (Elobeid et al., 2010). Cohort studies in children have not confirmed the association with weight gain (Cupul-Uicab et al., 2013; Tang-Péronard et al., 2015). However, in a birth cohort in the Faroe Islands, higher prenatal exposure to polychlorinated biphenyls was associated with increased BMI at age 7 years in daughters of overweight mothers (Tang-Péronard et al., 2014). In addition, higher levels of persistent organic pollutants have been observed in visceral fat of obese subjects with larger metabolic dysfunction compared with those without metabolic dysfunction (Pestana et al., 2014).
(e) Polycyclic aromatic hydrocarbons
Studies in animals have shown that the combination of exposure to diesel exhaust in utero and a high-fat diet as an adult increases susceptibility to diet-induced obesity and neuroinflammation in females but not in males (Bolton et al., 2012). In a birth cohort of women who were exposed to air pollutants during pregnancy, higher exposure levels were associated with higher obesity in their children at ages 5 years and 7 years (Rundle et al., 2012).
(f) Other compounds
Very limited data in humans are available for perfluorinated chemicals and flame retardants, and the results are inconclusive (Erkin-Cakmak et al., 2015; Heindel et al., 2015).
1.3.5. Physical activity and sedentary behaviour
Physical activity is considered “any bodily movement produced by skeletal muscles that results in energy expenditure” (Caspersen et al., 1985). The intensity of physical activity refers to the rate of energy expenditure brought about by physical activity; in epidemiological studies, it is commonly expressed in metabolic equivalents (METs) of tasks. Specifically, activities are grouped according to light (1.6–2.9 METs), moderate (3.0–5.9 METs), and vigorous (≥ 6 METs) levels of activity (Ainsworth et al., 2011). Several methods for measuring physical activity are available and have been used in epidemiological studies (Ndahimana & Kim, 2017).
Globally, there have been declines in levels of physical activity and increases in time spent sedentary in adults over the past decades (Ng et al., 2012; Dearth-Wesley et al., 2014). However, experimental evidence from repeated cross-sectional studies since the 1980s with measurements of energy expenditure by the doubly labelled water method indicate that levels of physical activity have not declined over the time period in which the prevalence of obesity has risen (Westerterp & Speakman, 2008). Thus, it seems likely that the recent rise in the average body weight of the population globally is determined largely by increased energy intake rather than by decreased energy expenditure (Swinburn et al., 2009).
(a) Physical activity and prevention of weight gain
Evidence from long-term observational studies (Williamson et al., 1993; Di Pietro et al., 2004; Gordon-Larsen et al., 2009; Lee et al., 2010; Mozaffarian et al., 2011) suggests a positive relationship between self-reported physical activity and prevention of weight gain. Data from RCTs are sparse and are based on short-term interventions and follow-up (Simkin-Silverman et al., 2003). Expert consensus has estimated that the amount of moderate-intensity physical activity associated with prevention of weight gain is 150–250 minutes per week (Donnelly et al., 2009), or 45–60 minutes per day (Saris et al., 2003).
(b) Physical activity and weight loss
In adults, moderate physical activity shows a dose–response association with weight loss. Based on evidence from observational studies of varying durations (spanning time periods of several months to several years), the American College of Sports Medicine concluded that less than 150 minutes per week results in minimal weight loss, more than 150 minutes per week yields weight loss of 2–3 kg, and 225–420 minutes per week leads to weight loss of 5–7.5 kg (Donnelly et al., 2009). Moreover, a positive relationship was reported, for a similar duration, between the intensity of the physical activity and the amount of weight lost (Shaw et al., 2006). A meta-analysis of trials with durations of 12 weeks to 12 months reported that moderate-intensity physical activity is only modestly effective in producing weight loss in overweight and obese adults, showing a small weighted mean difference in body weight of −1.6 kg (Thorogood et al., 2011).
Physical activity may generate a more pronounced effect on weight loss in children and adolescents, as suggested by a meta-analysis of 20 intervention studies reporting that an exercise programme involving approximately 150 minutes per week of physical activity for 13 weeks led to a 3.6% decrease in BMI in overweight and obese children and adolescents (Kelley et al., 2015).
A meta-analysis of 15 RCTs compared the effects of 2.5–6 months of aerobic and resistance exercise on weight loss in overweight and obese subjects and concluded that the combination of aerobic and resistance training may be the most efficacious physical activity regimen for weight loss (Schwingshackl et al., 2013).
Physical activity and energy restriction should yield similar amounts of weight loss if they provide comparable degrees of negative energy balance, and they are both important for achieving optimal weight loss. However, energy restriction combined with physical activity results in the combination of loss of fat mass and maintenance of lean mass, thereby leading to a more desirable effect on body composition (Miller et al., 2013).
(c) Physical activity and prevention of weight regain after weight loss
An early systematic review of RCTs and observational studies reported that people who engaged in physical activity experienced less weight regain than their sedentary counterparts, but confounding by a healthy lifestyle or reverse causation by better exercise adherence among those with less weight regain could not be ruled out (Fogelholm & Kukkonen-Harjula, 2000). In contrast, a more recent meta-analysis of RCTs on long-term maintenance of weight loss found no evidence of effectiveness for interventions involving physical activity only. However, the combination of physical activity and dietary energy restriction resulted in a difference of −1.56 kg in weight regain compared with controls at 12 months (Dombrowski et al., 2014). Taken together, findings from observational studies and RCTs show inconsistent results, and the volume of physical activity needed to prevent weight regain after weight loss remains poorly defined.
Data about the influence of resistance training on prevention of weight gain and weight regain after weight loss are sparse.
(d) Sedentary behaviour
Sedentary behaviour is a behaviour with reduced light activity and is distinct from lack of moderate to vigorous activity (Lynch et al., 2010). Time spent in sedentary activities reduces energy expenditure, potentially promoting weight gain over time (Owen et al., 2010). Long-term observational epidemiological studies in adults reported that sedentary behaviour, such as television viewing and computer gaming, is associated with enhanced risk of adiposity (Hu et al., 2003; Thomée et al., 2015). Also, television viewing is associated with enhanced intake of sugar-sweetened beverages and sweets (Lipsky & Iannotti, 2012). Moreover, a meta-analysis of 25 RCTs showed a small but significant decrease in BMI with reducing sedentary behaviour in children and adolescents (Liao et al., 2014). [Potential confounding by energy intake could not be ruled out.] Data on sedentary behaviour and prevention of weight regain after weight loss are sparse.
1.3.6. Built environment
The human body has evolved to transform excess energy into body fat during exceptional times of food abundance for protection during periods of low food availability, but has not adapted to meet the challenge of the supply provided by current food systems (Hill et al., 1998).
The built environment refers to aspects of a person’s surroundings that are human-made (or modified), compared with naturally occurring features (Caballero, 2007; Papas et al., 2007). It is one part of the so-called obesogenic environment, which includes sociocultural, economic, and marketing barriers to the achievement of healthy ways of life.
Food availability has undergone many changes in recent decades, as a result of economic, social, and demographic changes (the nutrition transition) (Brown, 1991; Popkin, 1993), resulting in an increased dependence on processed foods, a strong emphasis on the marketing and promotion of energy-dense foods and beverages, a decreased intake of fruit and vegetables, and an increased intake of foods that are high in fat and sugar (Caballero, 2007). The food environment has changed as the opportunities for consumption have increased through increased diversification of food outlets (e.g. vending and fast-food restaurants) and intensive and creative marketing strategies. In addition, changes in the built environment, including shifts in transportation patterns, labour-saving devices, limited public space for recreational physical activity, and increased public safety concerns, have promoted sedentary lifestyles (Caballero, 2007; Parizkova et al., 2007) (see also Section 1.3.5).
It is important to note that although individual energy balance is strongly predicted by personal behaviours, the factors that influence these behaviours are a response to the stimulus of the obesogenic environment. Thus, societal change that will have an impact both on public health and on the health of the individual is required to counter the current obesity epidemic (Mackenbach et al., 2014).
The ecological approach to obesity research embraces the biological, behavioural, and environmental factors that have an impact on energy balance (Egger & Swinburn, 1997). The built environment is thought to play a key role in promoting both reductions in energy expenditure and increases in energy intake, but it also has the potential to promote and sustain healthy behaviours for significant periods of time throughout the life-course (Ludwig et al., 2011). The built environment affects a person’s ability to comply with interventions, including educational interventions, that are focused on individual behaviour change (Sallis et al., 2012).
Most research has focused on the role of the built environment in providing opportunities for physical activity; with respect to the food environment, most work has focused on food availability within communities.
Several reviews have examined the relationship between the built environment and obesity, but they have had heterogeneous measurement approaches. The lack of valid and reliable measurement tools and analytical approaches may have contributed to inconsistent findings (Feng et al., 2010).
Metrics of the food environment (relevant for energy intake) include measures of the density, proximity, and diversity of and spatial access to food establishments (supermarkets, convenience stores, farmers’ markets, and restaurants). It is difficult to compare studies, because of metrics used and the overall suboptimal quality of the studies (Ding & Gebel, 2012). A review (Cobb et al., 2015) that assessed the relationship of obesity to local food environments reported few significant findings, although a trend towards an inverse association between availability of supermarkets and obesity was noted. Metrics that are relevant for physical activity (and thus energy expenditure) include measures of population density, diversity, connectivity, design of and spatial access to physical activity facilities, walkability, and sprawl (Lopez & Hynes, 2003).
Although a consistent relationship has been seen between physical activity (in the form of active transport and recreational walking) and neighbourhood walkability in adults, these studies have not always reported measures of obesity. Where obesity has been reported, there seems to be a lower than average BMI in neighbourhoods with higher perceived mixed land use, improved walkability, and better access to sports facilities (Black & Macinko, 2008). In a review by Papas et al. (2007), significant associations between some objectively measured aspects of the built environment (e.g. residential density, street connectivity, greenery, and access to destinations) and obesity were observed in 84% of the studies. In a study on environmental attributes and adult weight status in 12 countries, De Bourdeaudhuij et al. (2015) reported that safety from traffic had the most robust correlation with BMI.
Several studies have evaluated accessibility to recreation or exercise spaces and facilities (such as parks, playgrounds, cycle routes, and sports facilities) using adiposity as an outcome. In a review of cross-sectional studies that directly measured body weight in adults or children, two out of three studies in adults observed that shorter distance to (or greater density of) fitness facilities was associated with lower BMI and lower prevalence of overweight (Papas et al., 2007). In their review of population approaches to improving health behaviours, Mozaffarian et al. (2012) concluded that greater access to recreation and exercise spaces and facilities is relatively consistently linked to higher levels of physical activity and lower adiposity or other metabolic risk factors. However, they noted that nearly all the evidence is cross-sectional, which limits inferences about causality.
In a review by Dunton et al. (2009) of childhood obesity, associations between physical environmental variables and obesity differed by sex, age, socioeconomic status, population density, and whether reports were made by the parent or the child.
Research in the USA shows that children and adults living in rural areas are more likely to be obese than their urban counterparts (even after adjustment for individual-level behaviours). A review by Hansen et al. (2015) suggests that limited “active living” built environments are a contributory factor.
1.3.7. Social determinants
Socioeconomic status is an aggregate concept that consists of numerous indicators that reflect a person’s position in society, including education level, occupation, income, wealth, poverty, and deprivation. Because levels of BMI vary according to levels of economic development, sociocultural factors, and characteristics of the health-care system of a country, the relationship between social determinants and obesity cannot be explained by any individual factor; rather, numerous interrelated factors are involved (WHO, 2008).
It has been widely shown that in high-income countries, the prevalence of obesity is higher among people with lower socioeconomic status (WHO, 2014); this inverse association is driven mainly by the fact that socially disadvantaged people experience a more obesogenic environment, which favours access to cheap, energy-dense foods, lower levels of physical activity, and poor education. In contrast, in low-income countries a positive association is seen between socioeconomic status and the prevalence of obesity (Monteiro et al., 2004a, b; Blakely et al., 2005; Dinsa et al., 2012; Pampel et al., 2012). Possible reasons for this are food scarcity among the poor in low-income countries, leading to low or moderate food intake, and greater engagement in manual labour, requiring higher energy expenditure. By comparison, the rich in low-income countries may be particularly susceptible to obesity because of easy access to excess food and lower levels of occupational physical activity. Also, in some low-income countries, a large body size may be perceived as an indicator of socioeconomic status (for additional cultural aspects, see also Section 1.1). Inequalities in childhood obesity generally mirror those seen in adults (Due et al., 2009; Dinsa et al., 2012) (see also Section 1.2.5).
The relationship of socioeconomic status to obesity appears to be affected by the choice of socioeconomic indicator (income vs education level) in approximately 20–30% of studies conducted in low-income countries. This may be due to the weak correlation observed between wealth and education level in some low-income countries where educational investment has not yet translated into a higher income (Dinsa et al., 2012). One literature review of studies from high-income countries examined various indicators of socioeconomic status and found inverse relationships of education level and occupation to weight gain, with less consistent findings when income was used as the measure of socioeconomic status (Ball & Crawford, 2005). Another review of studies conducted in industrialized countries reported that women who changed social class after childhood took on the prevalence of adiposity of the class they joined, a relationship that was not evident in men (Parsons et al., 1999). Data about other social determinants, such as family size, number of parents at home, or availability of childcare and their relationship to obesity are sparse.
Education level has been one of the most frequently used measures of socioeconomic status (Krieger et al., 1997) and has been shown to be associated with obesity. This has led to an emphasis on nutrition education and practical food skills as a route to improving dietary habits. However, recent data from the United Kingdom (Adams & White, 2015) show an inconsistent relationship between sociodemographic variables and all markers of cooking skills, highlighting the need to explore the wider social factors that educational background reflects (see, for example, Lawrence & Barker, 2009).
It is recognized that numerous factors potentially influence food choice (and thus energy intake) across the population, which may have differential health effects according to socioeconomic status (McKinnon et al., 2014). Such factors include perception of sensory attributes, such as a liking for sweetness (Deglaire et al., 2015); psychological factors, such as mood (Singh, 2014); mental health and well-being, including stress (Moore & Cunningham, 2012); and the food marketing environment, notably with marketing of foods high in sugars, fats, and salt being targeted at children (Cairns et al., 2013).
1.3.8. Microbiota and gastrointestinal environment
The adult human gut hosts a complex community of microorganisms (microbiota), including about 1.5 kg of bacteria, most of which belong to four major phyla: Firmicutes, Bacteroidetes, Actinobacteria, and Proteobacteria. The amount, composition, and ratio of these vary according to diet and energy intake, and it is clear that variations in eating habits can lead to selective promotion of particular species. It is estimated that 57% of the bacterial variation in the gut is due to diet, and there are particular concerns that high-fat, high-sugar diets may alter the functionality of the microbiota (Harris et al., 2012). There is accumulating evidence to support a role for the microbiota in obesity and its downstream metabolic sequelae.
For example, the gut microbiota has been found to be significantly altered in animal models of obesity, with a reduction in bacterial diversity as well as a change in bacterial composition. It is generally agreed that a lower diversity of microorganisms and the presence of more tolerant bacteria are also observed in obese people. In many studies, the pattern associated with obesity is a relative decrease in Bacteroidetes (gram-negative) and a corresponding relative increase in Firmicutes (gram-positive), although there is some inconsistency in results in relation to ratios of different phyla (Sanmiguel et al., 2015). Reduction in body weight results in a shift in the composition of the gut microbiota, with a significant relative increase in Bacteroidetes and relative decrease in Firmicutes as weight loss progresses. Exercise alone is also associated with an alteration in the ratio of the major bacteria, with a higher proportion of butyrate-producing bacteria (Bacteroidetes) (Harris et al., 2012).
Both animals and humans experience major changes in the gut microbiota after gastric bypass, when there is a restoration to a normal ratio of Firmicutes to Bacteroidetes. It is postulated that this occurs as a result of major changes in the composition of the diet after surgery, a decrease in production of bile acid, and a raised pH, which encourages the growth of bacteria associated with weight loss (Harris et al., 2012; Sanmiguel et al., 2015). The changes in the microbiota appear to be independent of energy intake, suggesting that weight loss may also be the result of an interplay between the microbiota and host biology.
It is recognized that along with an impact on metabolic state, obesity-associated changes in bacterial diversity, as well as specific shifts in gut bacteria such as Faecalibacterium prausnitzii (phylum Firmicutes), are associated with alterations in the inflammatory state (Le Chatelier, et al., 2013; Marchesi et al., 2016) (see also Section 4.4.1). In studies in rats, it has been demonstrated that inflammation alone can cause weight gain in normal rats and that the absence of inflammation protects rats against weight gain from a high-fat diet (Harris et al., 2012).
Studies in animal models have demonstrated that transfer of the gut microbiota (faecal microbiota transplantation) from obese mice to lean mice results in weight gain despite decreases in food intake. Similarly, the introduction of a “lean” microbiota will result in weight loss in obese animals. However, in humans similar changes in the gut microbiota created by probiotics and prebiotics have not been successful in demonstrating weight loss (Graham et al., 2015; Sanmiguel et al., 2015). It seems likely that in humans, differences in the gut microbiota accompany obesity rather than causing the problem. Further research in experimental systems and in humans is needed to understand the link between the microbiome and obesity and whether variation in the microbiome is a direct cause of obesity or is a consequence of it.
1.3.9. Genetic and epigenetic determinants of obesity
There is growing evidence that gene–environment interactions play a major role in obesity (Reddon et al., 2016). A hereditary component to obesity has long been recognized, but the underlying genetic variants have only recently been identified. The first single nucleotide polymorphism robustly associated with increased BMI was identified in 2007 and mapped to a gene now known as FTO (Frayling et al., 2007). Since then, genome-wide association studies and meta-analyses conducted through large-scale consortium efforts (e.g. the Genetic Investigation of Anthropometric Traits [GIANT] consortium) have identified almost 100 gene variants robustly linked to BMI, including genes involved in appetite regulation, neural networking, glutamate receptor activity, insulin function, and energy metabolism (Speliotes et al., 2010; Locke et al., 2015). In addition, BMI-associated variants in genes linked to immune function, such as TLR4, may confer susceptibility to obesity through interactions with the microbiome. Genome-wide association studies for other anthropometric parameters, such as central adiposity (as determined by waist circumference), have identified additional variants (Heard-Costa et al., 2009). As even larger studies of common genetic variation and studies of rare variants are undertaken, and more sophisticated computational tools become available, it is likely that additional genetic variants associated with obesity will be identified. Nevertheless, the variants identified to date, combined, explain only a modest fraction (~3%) of the variation in BMI (Locke et al., 2015).
Epigenetic mechanisms, such as DNA methylation and histone modifications, may also play a role in obesity and represent an integrated measure of both genetic and environmental factors (see also Section 4.2.3). Emerging data from population-based studies indicate that specific regions of the genome are differentially methylated in obese people compared with those of normal weight (Dick et al., 2014; Aslibekyan et al., 2015). These studies demonstrate that increased adiposity is associated with specific epigenetic changes and may provide clues about the biology of obesity. Maternal exposure to environmental risk factors during pregnancy can alter the metabolic phenotype of offspring (e.g. “body size”) by means of epigenetic regulation of specific genes, and the epigenetic modifications could be passed on to future generations (Cutfield et al., 2007; Chamorro-García & Blumberg, 2014). Although developmental programming of body weight regulation occurs in humans, it is still unclear whether it occurs via epigenetic mechanisms (Institute of Medicine, 2015).
1.4. Assessment of anthropometric measures and body composition
The assessment of body composition is essential in obesity research, and several approaches have been proposed. The simpler and less expensive approach is to use weight and height – the most commonly used measures of nutritional status in epidemiological studies – and waist circumferences as a measure of abdominal obesity. More technologically advanced methods include bioelectrical impedance analysis (BIA), dual-energy X-ray absorptiometry (DXA), computed tomography (CT), and magnetic resonance imaging (MRI). These methods provide more precise and accurate estimates of body composition and fat distribution, but their use is limited by the lack of practicality and the cost; they are mostly used to validate simpler methods or in small clinical and epidemiological studies (Willett & Hu, 2013).
1.4.1. Weight and height
Standardized methods are available to measure weight, height, and other anthropometric variables (Gibson, 2005). These are currently used in nutritional surveys (CDC, 2016) and analytical epidemiological studies. These measurements should be obtained by trained health technicians, with routine calibration of equipment and strict quality control. Body weight is measured in kilograms (to the second decimal place) using a self-zeroing digital scale, while the subject is wearing light clothing and without shoes, preferably in the fasting state. Height is measured to the nearest millimetre with a stadiometer, while the subject is without shoes, with the back square against a metal wall tape, and with the eyes looking straight ahead and a set square resting on the scalp (Willett & Hu, 2013).
Among various anthropometric variables, weight and height are measured with the highest precision (reproducibility) and accuracy (little deviance from the true value) and with the least amount of technical error (Ulijaszek & Kerr, 1999). In many large cohort studies, weight and height are based on self-reports. Self-reported measures are more feasible for large samples and are necessary when considering past weight (Ulijaszek & Kerr, 1999). When self-reported measures are validated with objective measures, it has been shown that subjects tend to understate weight and overstate height (Krul et al., 2011); in general, the achieved degree of accuracy is sufficient to rank individuals in epidemiological studies, but use of self-reported measures will lead to an underestimation of the prevalence of obesity (Willett & Hu, 2013). Self-reported weight and height obtained by telephone interviews have been shown to be less reliable than those obtained by in-person interviews (Ezzati et al., 2006).
Adult height is a complex variable, determined primarily by genetics but also by nutritional factors, especially intakes of energy and proteins during the pre-adult period (Cole, 2000). Thus, height may reflect energy balance during childhood and adolescence (Willett & Hu, 2013).
Recalled weight from many years in the past appears to be valid, although the error is greater than for self-reported current weight. In women, the correlation between weight, height, and BMI measured at age 18 years and reported at ages 71–76 years was found to be 0.84, 0.92, and 0.83, respectively (Must et al., 1993).
In addition, useful information can be obtained by asking subjects to describe their body profile (body silhouette) currently and at different earlier ages using pictograms that range from very thin to massively obese (Sørensen et al., 1983). Recalled body silhouette at ages 20, 15, and 10 years has been found to correlate well with measured BMI at ages 71–76 years (Pearson correlation ranging from 0.53 to 0.75 in men and women) (Must et al., 1993). The use of these pictograms is particularly helpful in populations in which it is challenging to obtain accurate anthropometric measurements and to obtain information about body fatness at earlier ages (Romieu et al., 2012). Similar pictograms have been used to study personal perception of current body silhouette (Thompson & Gray, 1995) and perception of body silhouette in relation to age and health (Han et al., 1999).
Self-reported birth weight also appears to be reasonably valid. In the Nurses’ Health Study II cohort, the correlation between recorded birth weight and self-reported birth weight was 0.74 (Troy et al., 1996).
1.4.2. Indexes of adiposity
(a) BMI
The most commonly used index of obesity is the BMI (see Section 1.1). The cut-off value for underweight (18.5 kg/m2) is based largely on health-related problems associated with malnutrition in developing countries (IARC, 2002).
BMI is easy to assess and has high precision and accuracy. It has been shown to correlate strongly with both absolute body fat and body fat percentage in different age, sex, and racial groups when compared with more sophisticated measures of fat distribution (DXA) (Gallagher et al., 1996; Blew et al., 2002; Evans et al., 2006). BMI is a useful indicator of body fatness, but it is an imperfect measure of obesity, because it does not differentiate between lean mass and fat mass, the relative proportions of which vary between individuals and with age, sex, and race/ethnicity (Garn et al., 1986; Prentice & Jebb, 2001).
(i) Age and sex differences
For biological reasons, women have higher body fat percentages than men for the same BMI (Gallagher et al., 1996). For children, BMI centiles and Z-scores [measures of standard deviation] should be used to determine BMI status for ages up to 18 years, because these indicators are age- and sex-specific in children and adolescents (Cole, 2002; WHO 2016, 2017a, b). Among elderly people, the validity of BMI as a measure of body fatness appears to be reduced because of changes in body composition, such as loss of lean mass (sarcopenia) and increase in abdominal fat mass associated with ageing (Gallagher et al., 1996: Hu, 2008). This lower validity of BMI in elderly people may explain why the relationship of BMI with mortality is less pronounced in elderly people than in younger adults; in elderly people, waist circumference is a better marker of adiposity, in particular of abdominal fatness (Seidell & Visscher, 2000, Janssen et al., 2005).
(ii) Ethnic differences
The interpretation of BMI in epidemiological studies is further complicated by the ethnic variation in body composition (Hu, 2008). For the same BMI, Blacks have a lower adiposity and body fat percentage than Whites, on average, whereas Asians have a higher body fat percentage than Whites (Wagner & Heyward, 2000; Rush et al., 2009; Liu et al., 2011). On the basis of these observations, lower cut-off values have been proposed for overweight (23 kg/m2 to 27.4 kg/m2) and obesity (≥ 27.5 kg/m2) in Asian populations (WHO Expert Consultation, 2004). However, the available data do not allow a clear cut-off value to be established for all Asians, given the heterogeneity in the observed risks related to BMI in different Asian populations (WHO Expert Consultation, 2004).
(b) Waist circumference and hip circumference
There is increasing recognition that body fat distribution contributes to obesity-related disease risk, independently of overall adiposity (Eckel et al., 2005). Distribution of body fat has been used to delineate two body shapes: gynecoid (or pear shape), with fat accumulation in the lower part of the body, such as the hips and the thighs), and android (or apple shape), with fat accumulation in the upper part of the body, such as in the abdomen. Consistent evidence has linked android obesity with more metabolic alterations than gynecoid obesity (Hu, 2008).
Waist circumference is widely used as an indirect measure of abdominal and central obesity in epidemiological studies. Waist circumference is measured at the natural waist (midway between the lowest rib margin and the iliac crest) at the level of the umbilicus (Hu, 2008). Because a “natural waist” may be difficult to locate for obese subjects, the umbilicus site is preferred, although it may introduce substantial variations in defining the measurement site for very obese patients. Therefore, waist circumference is a less standardized metric than BMI. Correlation of waist circumference with predicted total abdominal fat and abdominal visceral fat using CT scan was 0.87–0.93 and 0.84–0.93, respectively, in men and women, and waist circumference performed as well as DXA (Clasey et al., 1999). However, in non-obese women, DXA appears to be superior to waist circumference (Kamel et al., 1999). Despite the fact that waist circumference has greater accuracy than BMI for measuring abdominal fat, it still does not differentiate the subcutaneous fat from the visceral fat.
Hip circumference is typically measured at the maximal circumference over the buttocks (Hu, 2008). Hip circumference is more difficult to interpret than waist circumference, because it can reflect more accumulation of subcutaneous fat, greater gluteal muscle mass, or larger bone structure (pelvic width) (Willett, 1998).
Waist-to-hip ratio is used as an indirect measure of abdominal and central obesity, but there is some evidence that waist circumference may be superior to waist-to-hip ratio as a surrogate marker of central obesity, in particular among elderly people, for whom waist-to-hip ratio may be an indicator of visceral obesity combined with muscle loss (Clasey et al., 1999; Snijder et al., 2006).
Waist circumference-to-height ratio has been used as an alternative to waist-to-hip ratio as a measure of central obesity, but it does not seem to be a better predictor of total mortality than waist circumference or waist-to-hip ratio (Hu, 2008).
Other more complex measures, such as sagittal abdominal diameter (i.e. the horizontal distance between the anterior and the posterior of the abdomen), waist-to-thigh ratio, or conicity index (waist circumference (m)/{0.109 × square root of weight (kg)/height (m)}) have been proposed; however, the difference with other measures is small (IARC, 2002).
Waist circumference has been recommended as a measure of central obesity over waist-to-hip ratio (NHLBI Obesity Education Initiative, 2000). Recommended cut-off values for waist circumference were 102 cm for men and 88 cm for women (Table 1.3), and for waist-to-hip ratio were 0.95 for men and 0.88 for women (Hu, 2008). However, as for BMI, these cut-off values are arbitrary, given that metabolic risk appears to increase linearly. In addition, the association of central obesity with chronic disease varies across different ages, sexes, and ethnicities. Recently, the International Diabetes Federation proposed a new definition of metabolic syndrome diagnosis that includes central obesity measured by waist circumference (Zimmet et al., 2005; IDF, 2006). Table 1.4 presents the recommended waist circumference cut-off values for Caucasian and Asian populations.
Table 1.3
Recommendations of body mass index and waist circumference cut-off values made for overweight or obesity.
Table 1.4
International Diabetes Federation criteria for waist circumference cut-off by ethnicity.
In large cohort studies, waist circumference and hip circumference are self-reported. Although obese participants tend to underestimate waist circumference, validation studies have shown high correlations between self-reported and technician-measured waist circumferences (r = 0.95 for men and 0.89 for women), whereas for waist-to-hip ratio the correlation was slightly lower (r = 0.69 for men and 0.70 for women) (Hu, 2008). [It is important to note that the validation studies on self-reported height, weight, waist circumference, and hip circumference have been done in educated populations in the USA and that, depending on the cultural and social context, self-reported anthropometric measures may have less validity.]
1.4.3. Other measures of adiposity or body composition
(a) Skinfold thickness
Skinfold thickness measurements are used as an indirect assessment of body fat distribution using a special caliper (skinfold caliper) to measure the thickness of a double layer of skin and the fat beneath it at predetermined sites, such as the triceps, the biceps, the subscapular region, the abdomen, and the thigh. The measurements are repeated most commonly at four body sites that are high in fat mass (usually the chest, arm, abdomen, and thigh), and in some cases at seven body sites. Skinfold measurement requires great expertise, is prone to inter-observer variations, and is less reproducible than other anthropometric methods. Skinfold thickness measurements and the use of standardized equations have been validated as a good measure of body fat percentage in adults and in children, but this is highly dependent on the validity of the prediction equation, which is population-specific (Hu, 2008; Horan et al., 2015). However, because skinfold thickness measurements are measures of subcutaneous fat, they are unable to quantify intra-abdominal or visceral fat (Steinberger et al., 2005).
(b) Bioelectrical impedance (BIA)
BIA estimates body composition by measuring the impedance or resistance to a small electrical current (typically 800 µA; 50 kHz) passing across the body tissues. A simple version is foot-to-foot BIA assessment, where an individual steps onto scales with electrode foot plates; this is particularly advantageous for children. The BIA method is based on the principle that resistance to an applied alternating electrical current is a function of tissue composition; because fat is a non-conductor of electricity, the greater the lean mass or water content of a person, the faster the current will pass through, and the greater the fatty tissue content, the greater the resistance to the current (Hu, 2008). BIA measures total body water content and enables the calculation of lean mass and fat mass (Kyle et al., 2004). Numerous prediction equations have been used to determine fat-free mass and body fat percentage. Models typically involved height, age, sex, race, weight, and other anthropometric measures. As with other prediction equations for body fat, those for BIA tend to be population-specific. [The limitations of this method include the hydration levels of the individual, the composition of the latest meal, whether a workout took place before the measurement, and the time of the day when the measurement took place.]
Comparison of estimates of body fat percentage has shown a good correlation between BIA and DXA in subjects within a normal range of body fat (r = 0.88 for the whole population; 0.78 for men and 0.85 for women). However, BIA tended to overestimate body fat percentage in lean subjects and to underestimate it in overweight and obese subjects (by 3–4%) (Sun et al., 2005).
Because BIA equipment is portable, it can be used in large surveys or epidemiological studies (Kyle et al., 2003).
(c) Ultrasound
Ultrasound, or ultrasonography, involves exposure of the body to high-frequency sound waves which reflect off the structures and tissues of the body and are detected by transducers. This method is non-invasive, portable, and quick, and ultrasound is a readily available technique in the clinical setting. It can also distinguish between visceral and subcutaneous fat (Wagner, 2013). However, interpretation of ultrasound images requires technical skill and practice. Ultrasound measures subcutaneous and pre-peritoneal fat, which give a good approximation of visceral fat in adults. Among children, results are conflicting (Horan et al., 2015).
(d) Dual-energy X-ray absorptiometry (DXA)
DXA involves the use of X-ray beams with different photon energies to determine body composition. High-density material (i.e. bone) attenuates the X-ray beam the most, whereas lower-density material allows more photons to pass through. DXA enables the determination of three components of the whole body (fat-free mass, fat mass, and bone mineral density) as well as of specific regions, such as the arms, legs, and trunk (Hu, 2008). The procedure is relatively simple, and the measurements are highly reproducible and accurate for lean mass and fat mass. DXA has the inconvenience of involving ionizing radiation, but the exposure is very low.
DXA measures of body composition have shown high reproducibility over several months (Cordero-MacIntyre et al., 2002) and high validity (Lohman & Chen, 2005). DXA estimates of trunk and abdominal fat mass were strongly correlated with CT scan estimates of total abdominal fat (correlations, 0.94–0.97) and abdominal visceral fat (correlations, 0.86–0.90) (Clasey et al., 1999; Glickman et al., 2004).
Although the DXA instrument is expensive and immobile, it has been used in several epidemiological studies either as a references method or in an association study with metabolic disorders or chronic outcomes. DXA has been used as a reference method to determine the body fat percentage corresponding to BMI cut-off values stratified by age, sex, and race/ethnicity in a large sample from the United States population (n = 12 906) (Heo et al., 2012). The oldest age group had the highest cut-off values of body fat percentage. Non-Hispanic Blacks had lower cut-off values, whereas Mexicans had the highest. Cut-off values of body fat percentage were higher in women than in men.
DXA is becoming a frequent measure of human body composition in small clinical and epidemiological studies to obtain a reliable estimate of total body fat mass and fat distribution, alone or together with ultrasound.
(e) Computed tomography (CT) and magnetic resonance imaging (MRI)
CT and MRI are considered to be the most accurate methods for assessing body composition and ascertaining fat distribution at the levels of tissues and organs. Both CT and MRI provide high-resolution cross-sectional scans of selected tissues or organs and can be used to measure the volume and distribution of subcutaneous versus visceral fat, muscle mass, and organ composition. Unlike CT, MRI does not expose subjects to ionizing radiation and can be used in children and pregnant women (Hu, 2008). The measurements are highly reproducible and accurate (Ross & Janssen, 2005). These methods are expensive and not readily accessible and are used mostly for calibrating or validating simpler and less expensive methods for measuring body fat distribution. CT and MRI have been used in small clinical and epidemiological studies to measure total adipose tissue, subcutaneous adipose tissue, visceral adipose tissue, and hepatic and intramuscular triglyceride content. Significant correlations have been found between visceral adipose tissue (but not subcutaneous adipose tissue), insulin resistance, and metabolic syndrome (Lebovitz & Banerji, 2005).
Recent epidemiological studies have implemented CT or MRI measurements on large subsamples to investigate biomarkers of fat distribution (Shah et al., 2016) or to validate simpler anthropometric indices (Neamat-Allah et al., 2014). Measured BMI, waist circumference, and hip circumference correlated well with MRI measures of total body volume, total adipose tissue, and subcutaneous adipose tissue in a cohort of German men (n = 598) and women (n = 594) aged 51–81 years (see Table 1.5).
1.4.4. Change in weight
Change in weight is also of interest when considering the association between weight and risk of cancer, and change in weight has been widely used in epidemiological studies as both exposure and outcome. Several definitions have been used across studies, such as the highest attained weight or current weight, weight gain, weight loss, weight cycling (repeated loss and regain of body weight over time), or body weight variability. Body weight gain during the period from young adulthood (18–20 years) to middle age (30–55 years) is of great interest.
Change in weight may rely on recall of previous weights by participants. The Nurses’ Health Study found a high correlation between recalled weight at age 18 years and measured weight from medical records, and this finding has been confirmed in additional studies (Troy et al., 1995). When considering weight loss, it is important to distinguish between intentional and unintentional weight loss, because unintentional weight loss is associated with increased morbidity and mortality (Wannamethee et al., 2005).
When epidemiological evidence is evaluated for a relationship between weight change and risk of cancer, the timing of weight change measurements must be considered, because evaluation of weight change at certain life stages may not be adequate to fully address potential associations.
Analysis to relate weight change to cancer risk should control for baseline BMI. As demonstrated in cardiovascular disease, to assess the contribution of waist circumference and waist-to-hip ratio separately from BMI, it is helpful to present results in strata of baseline BMI (Wormser et al., 2011). In particular, the initial value of BMI before the weight gain should be included in the model to directly assess the independent effect of the subsequent weight gain on risk; then, the subsequent weight change is predominantly change in adiposity. At present, analysis and reporting of weight gain is inconsistent in the cancer epidemiology literature.
1.4.5. Assessment of paediatric adiposity
The main anthropometric indicators of adiposity in children are BMI and waist circumference, which provide sufficient information to enable classification of overweight or obesity when growth centile charts and ratios are used (Horan et al., 2015). There is little evidence that alternative measures, including skinfold thickness and foot-to-foot BIA assessment, provide better estimates than BMI when measurements of weight and height are taken accurately and compared with appropriate growth charts and/or cut-off values. Indirect proxy reporting, such as parental reports, should only be used with caution (Himes, 2009; Van Cauwenberghe et al., 2014). In adolescents, self-reported height and weight have been shown to underestimate the prevalence of overweight, with a 25–50% underdiagnosis of overweight (Sherry et al., 2007).
References
- Adams J, White M (2015). Characterisation of UK diets according to degree of food processing and associations with socio-demographics and obesity: cross-sectional analysis of UK National Diet and Nutrition Survey (2008-12). Int J Behav Nutr Phys Act. 12(1):160. 10.1186/s12966-015-0317-y [PMC free article: PMC4683717] [PubMed: 26684833] [CrossRef]
- Ainsworth BE, Haskell WL, Herrmann SD, Meckes N, Bassett DR Jr, Tudor-Locke C, et al. (2011). 2011 Compendium of Physical Activities: a second update of codes and MET values. Med Sci Sports Exerc. 43(8):1575–81. 10.1249/MSS.0b013e31821ece12 [PubMed: 21681120] [CrossRef]
- Almandil NB, Liu Y, Murray ML, Besag FM, Aitchison KJ, Wong IC (2013). Weight gain and other metabolic adverse effects associated with atypical antipsychotic treatment of children and adolescents: a systematic review and meta-analysis. Paediatr Drugs. 15(2):139–50. 10.1007/s40272-013-0016-6 [PubMed: 23519708] [CrossRef]
- Anderson AS, Caswell S, MacAskill S, Steele RJC, Wells M (2010). LiveWell, a feasibility study of a personalized lifestyle programme for colorectal cancer survivors. Support Care Cancer. 18(4):409–15. 10.1007/s00520-009-0677-4 [PubMed: 19554354] [CrossRef]
- Anderson AS, Caswell S, Wells M, Steele RJC (2013). Obesity and lifestyle advice in colorectal cancer survivors – how well are clinicians prepared? Colorectal Dis. 15(8):949–57. 10.1111/codi.12203 [PubMed: 23480570] [CrossRef]
- Ashley-Martin J, Dodds L, Arbuckle TE, Ettinger AS, Shapiro GD, Fisher M, et al. (2014). A birth cohort study to investigate the association between prenatal phthalate and bisphenol A exposures and fetal markers of metabolic dysfunction. Environ Health. 13(84):84. 10.1186/1476-069X-13-84 [PMC free article: PMC4271497] [PubMed: 25336252] [CrossRef]
- Aslibekyan S, Demerath EW, Mendelson M, Zhi D, Guan W, Liang L, et al. (2015). Epigenome-wide study identifies novel methylation loci associated with body mass index and waist circumference. Obesity (Silver Spring). 23(7):1493–501. 10.1002/oby.21111 [PMC free article: PMC4482015] [PubMed: 26110892] [CrossRef]
- Bagci Bosi AT, Eriksen KG, Sobko T, Wijnhoven TM, Breda J (2016). Breastfeeding practices and policies in WHO European Region Member States. Public Health Nutr. 19(4):753–64. 10.1017/S1368980015001767 [PMC free article: PMC4754616] [PubMed: 26096540] [CrossRef]
- Bahadoran Z, Mirmiran P, Azizi F (2016). Fast food pattern and cardiometabolic disorders: a review of current studies. Health Promot Perspect. 5(4):231–40. 10.15171/hpp.2015.028 [PMC free article: PMC4772793] [PubMed: 26933642] [CrossRef]
- Ball K, Crawford D (2005). Socioeconomic status and weight change in adults: a review. Soc Sci Med. 60(9):1987–2010. 10.1016/j.socscimed.2004.08.056 [PubMed: 15743649] [CrossRef]
- Barker DJ (1990). The fetal and infant origins of adult disease. BMJ. 301(6761):1111. 10.1136/bmj.301.6761.1111 [PMC free article: PMC1664286] [PubMed: 2252919] [CrossRef]
- Bayon V, Leger D, Gomez-Merino D, Vecchierini MF, Chennaoui M (2014). Sleep debt and obesity. Ann Med. 46(5):264–72. 10.3109/07853890.2014.931103 [PubMed: 25012962] [CrossRef]
- Benedict C, Axelsson T, Söderberg S, Larsson A, Ingelsson E, Lind L, et al. (2014). Fat mass and obesity-associated gene (FTO) is linked to higher plasma levels of the hunger hormone ghrelin and lower serum levels of the satiety hormone leptin in older adults. Diabetes. 63(11):3955–9. 10.2337/db14-0470 [PubMed: 24898142] [CrossRef]
- Black JL, Macinko J (2008). Neighborhoods and obesity. Nutr Rev. 66(1):2–20. 10.1111/j.1753-4887.2007.00001.x [PubMed: 18254880] [CrossRef]
- Black RE, Victora CG, Walker SP, Bhutta ZA, Christian P, de Onis M, et al.; Maternal and Child Nutrition Study Group (2013). Maternal and child undernutrition and overweight in low-income and middle-income countries. Lancet. 382(9890):427–51. 10.1016/S0140-6736(13)60937-X [PubMed: 23746772] [CrossRef]
- Blakely T, Hales S, Kieft C, Wilson N, Woodward A (2005). The global distribution of risk factors by poverty level. Bull World Health Organ. 83(2):118–26. [PMC free article: PMC2623808] [PubMed: 15744404]
- Blew RM, Sardinha LB, Milliken LA, Teixeira PJ, Going SB, Ferreira DL, et al. (2002). Assessing the validity of body mass index standards in early postmenopausal women. Obes Res. 10(8):799–808. 10.1038/oby.2002.108 [PubMed: 12181389] [CrossRef]
- Blundell JE, Finlayson G (2004). Is susceptibility to weight gain characterized by homeostatic or hedonic risk factors for overconsumption? Physiol Behav. 82(1):21–5. 10.1016/j.physbeh.2004.04.021 [PubMed: 15234585] [CrossRef]
- Blundell JE, Finlayson G, Gibbons C, Caudwell P, Hopkins M (2015b). The biology of appetite control: do resting metabolic rate and fat-free mass drive energy intake? Physiol Behav. 152(Pt B):473–8. 10.1016/j.physbeh.2015.05.031 [PubMed: 26037633] [CrossRef]
- Blundell JE, Gibbons C, Caudwell P, Finlayson G, Hopkins M (2015a). Appetite control and energy balance: impact of exercise. Obes Rev. 16(Suppl 1):67–76. 10.1111/obr.12257 [PubMed: 25614205] [CrossRef]
- Bobrow KL, Quigley MA, Green J, Reeves GK, Beral V; Million Women Study Collaborators (2013). Persistent effects of women’s parity and breastfeeding patterns on their body mass index: results from the Million Women Study. Int J Obes (Lond). 37(5):712–7. 10.1038/ijo.2012.76 [PMC free article: PMC3647235] [PubMed: 22777544] [CrossRef]
- Bolton JL, Smith SH, Huff NC, Gilmour MI, Foster WM, Auten RL, et al. (2012). Prenatal air pollution exposure induces neuroinflammation and predisposes offspring to weight gain in adulthood in a sex-specific manner. FASEB J. 26(11):4743–54. 10.1096/fj.12-210989 [PubMed: 22815382] [CrossRef]
- Boyland EJ, Nolan S, Kelly B, Tudur-Smith C, Jones A, Halford JC, et al. (2016). Advertising as a cue to consume: a systematic review and meta-analysis of the effects of acute exposure to unhealthy food and nonalcoholic beverage advertising on intake in children and adults. Am J Clin Nutr. 103(2):519–33. 10.3945/ajcn.115.120022 [PubMed: 26791177] [CrossRef]
- Bray GA, Popkin BM (1998). Dietary fat intake does affect obesity! Am J Clin Nutr. 68(6):1157–73. [PubMed: 9846842]
- Brisbois TD, Farmer AP, McCargar LJ (2012). Early markers of adult obesity: a review. Obes Rev. 13(4):347–67. 10.1111/j.1467-789X.2011.00965.x [PMC free article: PMC3531624] [PubMed: 22171945] [CrossRef]
- Brown PJ (1991). Culture and the evolution of obesity. Hum Nat. 2(1):31–57. 10.1007/BF02692180 [PubMed: 24222189] [CrossRef]
- Buckland G, Bach A, Serra-Majem L (2008). Obesity and the Mediterranean diet: a systematic review of observational and intervention studies. Obes Rev. 9(6):582–93. 10.1111/j.1467-789X.2008.00503.x [PubMed: 18547378] [CrossRef]
- Burgio E, Lopomo A, Migliore L (2015). Obesity and diabetes: from genetics to epigenetics. Mol Biol Rep. 42(4):799–818. 10.1007/s11033-014-3751-z [PubMed: 25253098] [CrossRef]
- Caballero B (2007). The global epidemic of obesity: an overview. Epidemiol Rev. 29(1):1–5. 10.1093/epirev/mxm012 [PubMed: 17569676] [CrossRef]
- Cairns G, Angus K, Hastings G, Caraher M (2013). Systematic reviews of the evidence on the nature, extent and effects of food marketing to children. A retrospective summary. Appetite. 62:209–15. 10.1016/j.appet.2012.04.017 [PubMed: 22561190] [CrossRef]
- Capers PL, Fobian AD, Kaiser KA, Borah R, Allison DB (2015). A systematic review and meta-analysis of randomized controlled trials of the impact of sleep duration on adiposity and components of energy balance. Obes Rev. 16(9):771–82. 10.1111/obr.12296 [PMC free article: PMC4532553] [PubMed: 26098388] [CrossRef]
- Cappuccio FP, Taggart FM, Kandala NB, Currie A, Peile E, Stranges S, et al. (2008). Meta-analysis of short sleep duration and obesity in children and adults. Sleep. 31(5):619–26. [PMC free article: PMC2398753] [PubMed: 18517032]
- Caprio S, Daniels SR, Drewnowski A, Kaufman FR, Palinkas LA, Rosenbloom AL, et al. (2008). Influence of race, ethnicity, and culture on childhood obesity: implications for prevention and treatment: a consensus statement of Shaping America’s Health and the Obesity Society. Diabetes Care. 31(11):2211–21. 10.2337/dc08-9024 [PMC free article: PMC2571048] [PubMed: 18955718] [CrossRef]
- Caspersen CJ, Powell KE, Christenson GM (1985). Physical activity, exercise, and physical fitness: definitions and distinctions for health-related research. Public Health Rep. 100(2):126–31. [PMC free article: PMC1424733] [PubMed: 3920711]
- CDC (2016). NHANES III standardized anthropometric procedures, videos. Atlanta (GA), USA: Centers for Disease Control and Prevention. Available from: https://wwwn
.cdc.gov /nchs/nhanes/nhanes3 /anthropometricvideos.aspx. - Chakravartty S, Tassinari D, Salerno A, Giorgakis E, Rubino F (2015). What is the mechanism behind weight loss maintenance with gastric bypass? Curr Obes Rep. 4(2):262–8. 10.1007/s13679-015-0158-7 [PubMed: 26627220] [CrossRef]
- Chamorro-García R, Blumberg B (2014). Transgenerational effects of obesogens and the obesity epidemic. Curr Opin Pharmacol. 19:153–8. 10.1016/j.coph.2014.10.010 [PMC free article: PMC4297575] [PubMed: 25460228] [CrossRef]
- Chaput J-P, Tremblay A (2012). Insufficient sleep as a contributor to weight gain: an update. Curr Obes Rep. 1(4):245–56. 10.1007/s13679-012-0026-7 [CrossRef]
- Chen X, Beydoun MA, Wang Y (2008). Is sleep duration associated with childhood obesity? A systematic review and meta-analysis. Obesity (Silver Spring). 16(2):265–74. 10.1038/oby.2007.63 [PubMed: 18239632] [CrossRef]
- Clasey JL, Bouchard C, Teates CD, Riblett JE, Thorner MO, Hartman ML, et al. (1999). The use of anthropometric and dual-energy X-ray absorptiometry (DXA) measures to estimate total abdominal and abdominal visceral fat in men and women. Obes Res. 7(3):256–64. 10.1002/j.1550-8528.1999.tb00404.x [PubMed: 10348496] [CrossRef]
- Cobb LK, Appel LJ, Franco M, Jones-Smith JC, Nur A, Anderson CA (2015). The relationship of the local food environment with obesity: a systematic review of methods, study quality, and results. Obesity (Silver Spring). 23(7):1331–44. 10.1002/oby.21118 [PMC free article: PMC4482774] [PubMed: 26096983] [CrossRef]
- Cole TJ (2000). Secular trends in growth. Proc Nutr Soc. 59(2):317–24. 10.1017/S0029665100000355 [PubMed: 10946801] [CrossRef]
- Cole TJ (2002). A chart to link child centiles of body mass index, weight and height. Eur J Clin Nutr. 56(12):1194–9. 10.1038/sj.ejcn.1601473 [PubMed: 12494304] [CrossRef]
- Cordero-MacIntyre ZR, Peters W, Libanati CR, España RC, Abila SO, Howell WH, et al. (2002). Reproducibility of DXA in obese women. J Clin Densitom. 5(1):35–44. 10.1385/JCD:5:1:035 [PubMed: 11940727] [CrossRef]
- Cupul-Uicab LA, Klebanoff MA, Brock JW, Longnecker MP (2013). Prenatal exposure to persistent organochlorines and childhood obesity in the US Collaborative Perinatal Project. Environ Health Perspect. 121(9):1103–9. [PMC free article: PMC3764072] [PubMed: 23799652]
- Cutfield WS, Hofman PL, Mitchell M, Morison IM (2007). Could epigenetics play a role in the developmental origins of health and disease? Pediatr Res. 61(5 Pt 2):68R–75R. 10.1203/pdr.0b013e318045764c [PubMed: 17413843] [CrossRef]
- De Bourdeaudhuij I, Van Dyck D, Salvo D, Davey R, Reis RS, Schofield G, et al. (2015). International study of perceived neighbourhood environmental attributes and body mass index: IPEN Adult study in 12 countries. Int J Behav Nutr Phys Act. 12(1):62. 10.1186/s12966-015-0228-y [PMC free article: PMC4440250] [PubMed: 25982214] [CrossRef]
- Department for Communities and Local Government (2011). The English Indices of Deprivation 2010. Available from: https://www
.gov.uk/government /uploads/system /uploads/attachment_data /file/6871/1871208.pdf. - de Jonge L, DeLany JP, Nguyen T, Howard J, Hadley EC, Redman LM, et al. (2007). Validation study of energy expenditure and intake during calorie restriction using doubly labeled water and changes in body composition. Am J Clin Nutr. 85(1):73–9. [PMC free article: PMC2662402] [PubMed: 17209180]
- Dearth-Wesley T, Popkin BM, Ng SW (2014). Estimated and forecasted trends in domain specific time-use and energy expenditure among adults in Russia. Int J Behav Nutr Phys Act. 11(1):11. 10.1186/1479-5868-11-11 [PMC free article: PMC3931326] [PubMed: 24475868] [CrossRef]
- Deglaire A, Méjean C, Castetbon K, Kesse-Guyot E, Hercberg S, Schlich P (2015). Associations between weight status and liking scores for sweet, salt and fat according to the gender in adults (the Nutrinet-Santé study). Eur J Clin Nutr. 69(1):40–6. 10.1038/ejcn.2014.139 [PubMed: 25074389] [CrossRef]
- Di Cesare M, Khang YH, Asaria P, Blakely T, Cowan MJ, Farzadfar F, et al.; Lancet NCD Action Group (2013). Inequalities in non-communicable diseases and effective responses. Lancet. 381(9866):585–97. 10.1016/S0140-6736(12)61851-0 [PubMed: 23410608] [CrossRef]
- Di Pietro L, Dziura J, Blair SN (2004). Estimated change in physical activity level (PAL) and prediction of 5-year weight change in men: the Aerobics Center Longitudinal Study. Int J Obes Relat Metab Disord. 28(12):1541–7. 10.1038/sj.ijo.0802821 [PubMed: 15543159] [CrossRef]
- Dick KJ, Nelson CP, Tsaprouni L, Sandling JK, Aïssi D, Wahl S, et al. (2014). DNA methylation and body-mass index: a genome-wide analysis. Lancet. 383(9933):1990–8. 10.1016/S0140-6736(13)62674-4 [PubMed: 24630777] [CrossRef]
- Ding D, Gebel K (2012). Built environment, physical activity, and obesity: what have we learned from reviewing the literature? Health Place. 18(1):100–5. 10.1016/j.healthplace.2011.08.021 [PubMed: 21983062] [CrossRef]
- Dinsa GD, Goryakin Y, Fumagalli E, Suhrcke M (2012). Obesity and socioeconomic status in developing countries: a systematic review. Obes Rev. 13(11):1067–79. 10.1111/j.1467-789X.2012.01017.x [PMC free article: PMC3798095] [PubMed: 22764734] [CrossRef]
- Dior UP, Lawrence GM, Sitlani C, Enquobahrie D, Manor O, Siscovick DS, et al. (2014). Parental smoking during pregnancy and offspring cardio-metabolic risk factors at ages 17 and 32. Atherosclerosis. 235(2):430–7. 10.1016/j.atherosclerosis.2014.05.937 [PMC free article: PMC4123626] [PubMed: 24937467] [CrossRef]
- Dombrowski SU, Knittle K, Avenell A, Araújo-Soares V, Sniehotta FF (2014). Long term maintenance of weight loss with non-surgical interventions in obese adults: systematic review and meta-analyses of randomised controlled trials. BMJ. 348:g2646. 10.1136/bmj.g2646 [PMC free article: PMC4020585] [PubMed: 25134100] [CrossRef]
- Dominguez-Salas P, Moore SE, Baker MS, Bergen AW, Cox SE, Dyer RA, et al. (2014). Maternal nutrition at conception modulates DNA methylation of human metastable epialleles. Nat Commun. 5:3746. 10.1038/ncomms4746 [PMC free article: PMC4015319] [PubMed: 24781383] [CrossRef]
- Donnelly JE, Blair SN, Jakicic JM, Manore MM, Rankin JW, Smith BK; American College of Sports Medicine (2009). American College of Sports Medicine Position Stand. Appropriate physical activity intervention strategies for weight loss and prevention of weight regain for adults. Med Sci Sports Exerc. 41(2):459–71. 10.1249/MSS.0b013e3181949333 [PubMed: 19127177] [CrossRef]
- Due P, Damsgaard MT, Rasmussen M, Holstein BE, Wardle J, Merlo J, et al.; HBSC obesity writing group (2009). Socioeconomic position, macroeconomic environment and overweight among adolescents in 35 countries. Int J Obes. 33(10):1084–93. 10.1038/ijo.2009.128 [PMC free article: PMC3421462] [PubMed: 19621018] [CrossRef]
- Dunton GF, Kaplan J, Wolch J, Jerrett M, Reynolds KD (2009). Physical environmental correlates of childhood obesity: a systematic review. Obes Rev. 10(4):393–402. 10.1111/j.1467-789X.2009.00572.x [PMC free article: PMC3833101] [PubMed: 19389058] [CrossRef]
- Eckel RH, Grundy SM, Zimmet PZ (2005). The metabolic syndrome. Lancet. 365(9468):1415–28. 10.1016/S0140-6736(05)66378-7 [PubMed: 15836891] [CrossRef]
- Egger G, Swinburn B (1997). An “ecological” approach to the obesity pandemic. BMJ. 315(7106):477–80. 10.1136/bmj.315.7106.477 [PMC free article: PMC2127317] [PubMed: 9284671] [CrossRef]
- Eissing L (2013). Metabolism: FTO-associated obesity risk is linked to brain food responses via modulation of ghrelin levels. Nat Rev Endocrinol. 9(10):564. 10.1038/nrendo.2013.155 [PubMed: 23917583] [CrossRef]
- Ello-Martin JA, Ledikwe JH, Rolls BJ (2005). The influence of food portion size and energy density on energy intake: implications for weight management. Am J Clin Nutr. 82(1 Suppl):236S–41S. [PubMed: 16002828]
- Elobeid MA, Padilla MA, Brock DW, Ruden DM, Allison DB (2010). Endocrine disruptors and obesity: an examination of selected persistent organic pollutants in the NHANES 1999–2002 data. Int J Environ Res Public Health. 7(7):2988–3005. 10.3390/ijerph7072988 [PMC free article: PMC2922741] [PubMed: 20717554] [CrossRef]
- Erkin-Cakmak A, Harley KG, Chevrier J, Bradman A, Kogut K, Huen K, et al. (2015). In utero and childhood polybrominated diphenyl ether exposures and body mass at age 7 years: the CHAMACOS study. Environ Health Perspect. 123(6):636–42. [PMC free article: PMC4455588] [PubMed: 25738596]
- European Commission (2006). Health and food. Special Eurobarometer 246/Wave 64.3 – TNS Opinion & Social. Available from: http://ec
.europa.eu/public_opinion /archives/ebs/ebs_246_en .pdf. - Evans EM, Rowe DA, Racette SB, Ross KM, McAuley E (2006). Is the current BMI obesity classification appropriate for black and white postmenopausal women? Int J Obes (Lond). 30(5):837–43. 10.1038/sj.ijo.0803208 [PubMed: 16418761] [CrossRef]
- Ezzati M, Martin H, Skjold S, Vander Hoorn S, Murray CJ (2006). Trends in national and state-level obesity in the USA after correction for self-report bias: analysis of health surveys. J R Soc Med. 99(5):250–7. 10.1258/jrsm.99.5.250 [PMC free article: PMC1457748] [PubMed: 16672759] [CrossRef]
- Fardet A, Boirie Y (2014). Associations between food and beverage groups and major diet-related chronic diseases: an exhaustive review of pooled/meta-analyses and systematic reviews. Nutr Rev. 72(12):741–62. 10.1111/nure.12153 [PubMed: 25406801] [CrossRef]
- Farpour-Lambert NJ, Baker JL, Hassapidou M, Holm JC, Nowicka P, O’Malley G, et al. (2015). Childhood obesity is a chronic disease demanding specific health care – a position statement from the Childhood Obesity Task Force (COTF) of the European Association for the Study of Obesity (EASO). Obes Facts. 8(5):342–9. 10.1159/000441483 [PMC free article: PMC5644867] [PubMed: 26469067] [CrossRef]
- Feng J, Glass TA, Curriero FC, Stewart WF, Schwartz BS (2010). The built environment and obesity: a systematic review of the epidemiologic evidence. Health Place. 16(2):175–90. 10.1016/j.healthplace.2009.09.008 [PubMed: 19880341] [CrossRef]
- Flegal KM, Carroll MD, Ogden CL, Johnson CL (2002). Prevalence and trends in obesity among US adults, 1999–2000. JAMA. 288(14):1723–7. 10.1001/jama.288.14.1723 [PubMed: 12365955] [CrossRef]
- Fogelholm M, Anderssen S, Gunnarsdottir I, Lahti-Koski M (2012). Dietary macronutrients and food consumption as determinants of long-term weight change in adult populations: a systematic literature review. Food Nutr Res. 56(0). 10.3402/fnr.v56i0.19103 [PMC free article: PMC3418611] [PubMed: 22893781] [CrossRef]
- Fogelholm M, Kukkonen-Harjula K (2000). Does physical activity prevent weight gain – a systematic review. Obes Rev. 1(2):95–111. 10.1046/j.1467-789x.2000.00016.x [PubMed: 12119991] [CrossRef]
- Forshee RA, Anderson PA, Storey ML (2008). Sugar-sweetened beverages and body mass index in children and adolescents: a meta-analysis. Am J Clin Nutr. 87(6):1662–71. [PubMed: 18541554]
- Frayling TM, Timpson NJ, Weedon MN, Zeggini E, Freathy RM, Lindgren CM, et al. (2007). A common variant in the FTO gene is associated with body mass index and predisposes to childhood and adult obesity. Science. 316(5826):889–94. 10.1126/science.1141634 [PMC free article: PMC2646098] [PubMed: 17434869] [CrossRef]
- Freedman DS, Khan LK, Serdula MK, Dietz WH, Srinivasan SR, Berenson GS (2005). The relation of childhood BMI to adult adiposity: the Bogalusa Heart Study. Pediatrics. 115(1):22–7. [PubMed: 15629977]
- Gallagher D, Visser M, Sepúlveda D, Pierson RN, Harris T, Heymsfield SB (1996). How useful is body mass index for comparison of body fatness across age, sex, and ethnic groups? Am J Epidemiol. 143(3):228–39. 10.1093/oxfordjournals.aje.a008733 [PubMed: 8561156] [CrossRef]
- García-Fernández E, Rico-Cabanas L, Rosgaard N, Estruch R, Bach-Faig A (2014). Mediterranean diet and cardiodiabesity: a review. Nutrients. 6(9):3474–500. 10.3390/nu6093474 [PMC free article: PMC4179172] [PubMed: 25192027] [CrossRef]
- Garn SM, Leonard WR, Hawthorne VM (1986). Three limitations of the body mass index. Am J Clin Nutr. 44(6):996–7. [PubMed: 3788846]
- Gibson RS (2005). Principles of nutritional assessment. 2nd edition. Oxford, UK: Oxford University Press.
- Gibson S (2008). Sugar-sweetened soft drinks and obesity: a systematic review of the evidence from observational studies and interventions. Nutr Res Rev. 21(2):134–47. 10.1017/S0954422408110976 [PubMed: 19087367] [CrossRef]
- Glickman SG, Marn CS, Supiano MA, Dengel DR (2004). Validity and reliability of dual-energy X-ray absorptiometry for the assessment of abdominal adiposity. J Appl Physiol (1985). 97(2):509–14. 10.1152/japplphysiol.01234.2003 [PubMed: 15075304] [CrossRef]
- Gordon-Larsen P, Hou N, Sidney S, Sternfeld B, Lewis CE, Jacobs DR Jr, et al. (2009). Fifteen-year longitudinal trends in walking patterns and their impact on weight change. Am J Clin Nutr. 89(1):19–26. 10.3945/ajcn.2008.26147 [PMC free article: PMC2715291] [PubMed: 19056560] [CrossRef]
- Gotsis E, Anagnostis P, Mariolis A, Vlachou A, Katsiki N, Karagiannis A (2015). Health benefits of the Mediterranean Diet: an update of research over the last 5 years. Angiology. 66(4):304–18. 10.1177/0003319714532169 [PubMed: 24778424] [CrossRef]
- Graham C, Mullen A, Whelan K (2015). Obesity and the gastrointestinal microbiota: a review of associations and mechanisms. Nutr Rev. 73(6):376–85. 10.1093/nutrit/nuv004 [PubMed: 26011912] [CrossRef]
- Grinker JA, Tucker K, Vokonas PS, Rush D (1995). Body habitus changes among adult males from the normative aging study: relations to aging, smoking history and alcohol intake. Obes Res. 3(5):435–46. 10.1002/j.1550-8528.1995.tb00173.x [PubMed: 8521163] [CrossRef]
- Gross AL, May BJ, Axilbund JE, Armstrong DK, Roden RB, Visvanathan K (2015). Weight change in breast cancer survivors compared to cancer-free women: a prospective study in women at familial risk of breast cancer. Cancer Epidemiol Biomarkers Prev. 24(8):1262–9. 10.1158/1055-9965.EPI-15-0212 [PMC free article: PMC4531263] [PubMed: 26178712] [CrossRef]
- Grün F, Blumberg B (2009). Endocrine disrupters as obesogens. Mol Cell Endocrinol. 304(1–2):19–29. 10.1016/j.mce.2009.02.018 [PMC free article: PMC2713042] [PubMed: 19433244] [CrossRef]
- Guo SS, Chumlea WC (1999). Tracking of body mass index in children in relation to overweight in adulthood. Am J Clin Nutr. 70:145S–8S. [PubMed: 10419418]
- Han TS, Hart CL, Haig C, Logue J, Upton MN, Watt GC, et al. (2015). Contribution of maternal and paternal adiposity and smoking to adult offspring adiposity and CVD risk. BMJ Open. 5(11):e007682. 10.1136/bmjopen-2015-007682 [PMC free article: PMC4636631] [PubMed: 26525718] [CrossRef]
- Han TS, Morrison CE, Lean ME (1999). Age and health indications assessed by silhouette photographs. Eur J Clin Nutr. 53(8):606–11. 10.1038/sj.ejcn.1600816 [PubMed: 10477246] [CrossRef]
- Hansen AY, Umstattd Meyer MR, Lenardson JD, Hartley D (2015). Built environments and active living in rural and remote areas: a review of the literature. Curr Obes Rep. 4(4):484–93. 10.1007/s13679-015-0180-9 [PubMed: 26364307] [CrossRef]
- Harris K, Kassis A, Major G, Chou CJ (2012). Is the gut microbiota a new factor contributing to obesity and its metabolic disorders? J Obes. 2012:879151. 10.1155/2012/879151 [PMC free article: PMC3270440] [PubMed: 22315672] [CrossRef]
- Haus EL, Smolensky MH (2013). Shift work and cancer risk: potential mechanistic roles of circadian disruption, light at night, and sleep deprivation. Sleep Med Rev. 17(4):273–84. 10.1016/j.smrv.2012.08.003 [PubMed: 23137527] [CrossRef]
- Health and Social Care Information Centre (2013). National Child Measurement Programme: England, 2012-13 school year. Available from: http://content
.digital .nhs.uk/catalogue/PUB13115 /nati-chil-meas-prog-eng-2012-2013-rep.pdf. - Heard-Costa NL, Zillikens MC, Monda KL, Johansson A, Harris TB, Fu M, et al. (2009). NRXN3 is a novel locus for waist circumference: a genome-wide association study from the CHARGE Consortium. PLoS Genet. 5(6):e1000539. 10.1371/journal.pgen.1000539 [PMC free article: PMC2695005] [PubMed: 19557197] [CrossRef]
- Heindel JJ, Newbold R, Schug TT (2015). Endocrine disruptors and obesity. Nat Rev Endocrinol. 11(11):653–61. 10.1038/nrendo.2015.163 [PubMed: 26391979] [CrossRef]
- Heo M, Faith MS, Pietrobelli A, Heymsfield SB (2012). Percentage of body fat cutoffs by sex, age, and race-ethnicity in the US adult population from NHANES 1999–2004. Am J Clin Nutr. 95(3):594–602. 10.3945/ajcn.111.025171 [PubMed: 22301924] [CrossRef]
- Hill JO, Peters JC, Peters JC (1998). Environmental contributions to the obesity epidemic. Science. 280(5368):1371–4. 10.1126/science.280.5368.1371 [PubMed: 9603719] [CrossRef]
- Hill JO, Wyatt HR, Peters JC (2012). Energy balance and obesity. Circulation. 126(1):126–32. 10.1161/CIRCULATIONAHA.111.087213 [PMC free article: PMC3401553] [PubMed: 22753534] [CrossRef]
- Himes JH (2009). Challenges of accurately measuring and using BMI and other indicators of obesity in children. Pediatrics. 124(Suppl 1):S3–22. 10.1542/peds.2008-3586D [PubMed: 19720665] [CrossRef]
- Hooper L, Abdelhamid A, Bunn D, Brown T, Summerbell CD, Skeaff CM (2015). Effects of total fat intake on body weight. Cochrane Database Syst Rev. 8:CD011834. 10.1002/14651858.CD011834 [PMC free article: PMC10403157] [PubMed: 26250104] [CrossRef]
- Horan M, Gibney E, Molloy E, McAuliffe F (2015). Methodologies to assess paediatric adiposity. Ir J Med Sci. 184(1):53–68. 10.1007/s11845-014-1124-1 [PubMed: 24791970] [CrossRef]
- Horta BL, Loret de Mola C, Victora CG (2015). Long-term consequences of breastfeeding on cholesterol, obesity, systolic blood pressure and type 2 diabetes: a systematic review and meta-analysis. Acta Paediatr. 104(467):30–7. 10.1111/apa.13133 [PubMed: 26192560] [CrossRef]
- Hu FB (2008). Measurements of adiposity and body composition. In: Hu FB, editor. Obesity epidemiology. Oxford, UK: Oxford University Press. 10.1093/acprof:oso/9780195312911.003.0005 [CrossRef]
- Hu FB, Li TY, Colditz GA, Willett WC, Manson JE (2003). Television watching and other sedentary behaviors in relation to risk of obesity and type 2 diabetes mellitus in women. JAMA. 289(14):1785–91. 10.1001/jama.289.14.1785 [PubMed: 12684356] [CrossRef]
- Hu FB, Malik VS (2010). Sugar-sweetened beverages and risk of obesity and type 2 diabetes: epidemiologic evidence. Physiol Behav. 100(1):47–54. 10.1016/j.physbeh.2010.01.036 [PMC free article: PMC2862460] [PubMed: 20138901] [CrossRef]
- Hu T, Mills KT, Yao L, Demanelis K, Eloustaz M, Yancy WS Jr, et al. (2012). Effects of low-carbohydrate diets versus low-fat diets on metabolic risk factors: a meta-analysis of randomized controlled clinical trials. Am J Epidemiol. 176(Suppl 7):S44–54. 10.1093/aje/kws264 [PMC free article: PMC3530364] [PubMed: 23035144] [CrossRef]
- IARC (2002). Weight control and physical activity. Lyon, France: IARC Press (IARC Handbooks of Cancer Prevention, Vol. 6). Available from: http://publications
.iarc.fr/376. - IDF (2006). The IDF consensus worldwide definition of the metabolic syndrome. Brussels, Belgium: International Diabetes Federation. Available from: https://www
.idf.org/e-library /consensus-statements /60-idfconsensus-worldwide-definitionof-the-metabolic-syndrome. - IHME (2014). Overweight and Obesity Viz. Seattle (WA), USA: Institute for Health Metrics and Evaluation. Available from: http://www
.healthdata .org/data-visualization /overweight-and-obesity-viz. - Institute of Medicine (2015). Examining a developmental approach to childhood obesity: the fetal and early childhood years. Workshop summary. Washington (DC), USA: National Academies Press. Available from: https://www
.nap.edu/catalog/21782. [PubMed: 26512393] - Janesick AS, Blumberg B (2016). Obesogens: an emerging threat to public health. Am J Obstet Gynecol. 214(5):559–65. 10.1016/j.ajog.2016.01.182 [PMC free article: PMC4851574] [PubMed: 26829510] [CrossRef]
- Janssen I, Katzmarzyk PT, Ross R (2005). Body mass index is inversely related to mortality in older people after adjustment for waist circumference. J Am Geriatr Soc. 53(12):2112–8. 10.1111/j.1532-5415.2005.00505.x [PubMed: 16398895] [CrossRef]
- Jarlenski MP, Bennett WL, Bleich SN, Barry CL, Stuart EA (2014). Effects of breastfeeding on postpartum weight loss among U.S. women. Prev Med. 69:146–50. 10.1016/j.ypmed.2014.09.018 [PMC free article: PMC4312189] [PubMed: 25284261] [CrossRef]
- Jaworowska A, Blackham T, Davies IG, Stevenson L (2013). Nutritional challenges and health implications of takeaway and fast food. Nutr Rev. 71(5):310–8. 10.1111/nure.12031 [PubMed: 23590707] [CrossRef]
- Jiang P, Turek FW (2017). Timing of meals: when is as critical as what and how much. Am J Physiol Endocrinol Metab. 312(5):E369–80. 10.1152/ajpendo.00295.2016 [PMC free article: PMC6105931] [PubMed: 28143856] [CrossRef]
- Kaiser KA, Shikany JM, Keating KD, Allison DB (2013). Will reducing sugar-sweetened beverage consumption reduce obesity? Evidence supporting conjecture is strong, but evidence when testing effect is weak. Obes Rev. 14(8):620–33. 10.1111/obr.12048 [PMC free article: PMC3929296] [PubMed: 23742715] [CrossRef]
- Kamel EG, McNeill G, Han TS, Smith FW, Avenell A, Davidson L, et al. (1999). Measurement of abdominal fat by magnetic resonance imaging, dual-energy X-ray absorptiometry and anthropometry in non-obese men and women. Int J Obes Relat Metab Disord. 23(7):686–92. 10.1038/sj.ijo.0800904 [PubMed: 10454101] [CrossRef]
- Kelley GA, Kelley KS, Pate RR (2015). Exercise and BMI in overweight and obese children and adolescents: a systematic review and trial sequential meta-analysis. Biomed Res Int. 2015:704539. 10.1155/2015/704539 [PMC free article: PMC4633529] [PubMed: 26579538] [CrossRef]
- Kim SH, Park MJ (2014). Phthalate exposure and childhood obesity. Ann Pediatr Endocrinol Metab. 19(2):69–75. 10.6065/apem.2014.19.2.69 [PMC free article: PMC4114051] [PubMed: 25077088] [CrossRef]
- Kim TW, Jeong JH, Hong SC (2015). The impact of sleep and circadian disturbance on hormones and metabolism. Int J Endocrinol. 2015:591729. 10.1155/2015/591729 [PMC free article: PMC4377487] [PubMed: 25861266] [CrossRef]
- Krieger N, Williams DR, Moss NE (1997). Measuring social class in US public health research: concepts, methodologies, and guidelines. Annu Rev Public Health. 18(1):341–78. 10.1146/annurev.publhealth.18.1.341 [PubMed: 9143723] [CrossRef]
- Krul AJ, Daanen HA, Choi H (2011). Self-reported and measured weight, height and body mass index (BMI) in Italy, the Netherlands and North America. Eur J Public Health. 21(4):414–9. 10.1093/eurpub/ckp228 [PubMed: 20089678] [CrossRef]
- Kumar BN, Meyer HE, Wandel M, Dalen I, Holmboe-Ottesen G (2006). Ethnic differences in obesity among immigrants from developing countries, in Oslo, Norway. Int J Obes (Lond). 30(4):684–90. 10.1038/sj.ijo.0803051 [PubMed: 16130029] [CrossRef]
- Kyle UG, Bosaeus I, De Lorenzo AD, Deurenberg P, Elia M, Gómez JM, et al.; Composition of the ESPEN Working Group (2004). Bioelectrical impedance analysis – part I: review of principles and methods. Clin Nutr. 23(5):1226–43. 10.1016/j.clnu.2004.06.004 [PubMed: 15380917] [CrossRef]
- Kyle UG, Pirlich M, Schuetz T, Luebke HJ, Lochs H, Pichard C (2003). Prevalence of malnutrition in 1760 patients at hospital admission: a controlled population study of body composition. Clin Nutr. 22(5):473–81. 10.1016/S0261-5614(03)00049-9 [PubMed: 14512035] [CrossRef]
- Lakind JS, Goodman M, Mattison DR (2014). Bisphenol A and indicators of obesity, glucose metabolism/type 2 diabetes and cardiovascular disease: a systematic review of epidemiologic research. Crit Rev Toxicol. 44(2):121–50. 10.3109/10408444.2013.860075 [PubMed: 24392816] [CrossRef]
- Lamerz A, Kuepper-Nybelen J, Wehle C, Bruning N, Trost-Brinkhues G, Brenner H, et al. (2005). Social class, parental education, and obesity prevalence in a study of six-year-old children in Germany. Int J Obes (Lond). 29(4):373–80. 10.1038/sj.ijo.0802914 [PubMed: 15768043] [CrossRef]
- Langley-Evans SC (2015). Nutrition in early life and the programming of adult disease: a review. J Hum Nutr Diet. 28(Suppl 1):1–14. 10.1111/jhn.12212 [PubMed: 24479490] [CrossRef]
- Lawrence W, Barker M (2009). A review of factors affecting the food choices of disadvantaged women. Proc Nutr Soc. 68(2):189–94. 10.1017/S0029665109001013 [PubMed: 19208268] [CrossRef]
- Le Chatelier E, Nielsen T, Qin J, Prifti E, Hildebrand F, Falony G, et al.; MetaHIT consortium (2013). Richness of human gut microbiome correlates with metabolic markers. Nature. 500(7464):541–6. 10.1038/nature12506 [PubMed: 23985870] [CrossRef]
- Lean MEJ, Malkova D (2015). Altered gut and adipose tissue hormones in overweight and obese individuals: cause or consequence? Int J Obes (Lond). 1:1–10. [PMC free article: PMC4827002] [PubMed: 26499438]
- Lebovitz HE, Banerji MA (2005). Point: visceral adiposity is causally related to insulin resistance. Diabetes Care. 28(9):2322–5. 10.2337/diacare.28.9.2322 [PubMed: 16123512] [CrossRef]
- Lee IM, Djoussé L, Sesso HD, Wang L, Buring JE (2010). Physical activity and weight gain prevention. JAMA. 303(12):1173–9. 10.1001/jama.2010.312 [PMC free article: PMC2846540] [PubMed: 20332403] [CrossRef]
- Leslie WS, Hankey CR, Lean ME (2007). Weight gain as an adverse effect of some commonly prescribed drugs: a systematic review. QJM. 100(7):395–404. 10.1093/qjmed/hcm044 [PubMed: 17566010] [CrossRef]
- Liao Y, Liao J, Durand CP, Dunton GF (2014). Which type of sedentary behaviour intervention is more effective at reducing body mass index in children? A meta-analytic review. Obes Rev. 15(3):159–68. 10.1111/obr.12112 [PMC free article: PMC4131845] [PubMed: 24588966] [CrossRef]
- Link BG, Phelan JC (2001). Conceptualizing stigma. Annu Rev Sociol. 27(1):363–85. 10.1146/annurev.soc.27.1.363 [CrossRef]
- Lipsky LM, Iannotti RJ (2012). Associations of television viewing with eating behaviors in the 2009 Health Behaviour in School-aged Children Study. Arch Pediatr Adolesc Med. 166(5):465–72. 10.1001/archpediatrics.2011.1407 [PMC free article: PMC4733642] [PubMed: 22566548] [CrossRef]
- Lissner L, Wijnhoven TMA, Mehlig K, Sjöberg A, Kunesova M, Yngve A, et al. (2016). Socioeconomic inequalities in childhood overweight: heterogeneity across five countries in the WHO European Childhood Obesity Surveillance Initiative (COSI-2008). Int J Obes (Lond). 40(5):796–802. 10.1038/ijo.2016.12 [PMC free article: PMC4856730] [PubMed: 27136760] [CrossRef]
- Liu A, Byrne NM, Kagawa M, Ma G, Poh BK, Ismail MN, et al. (2011). Ethnic differences in the relationship between body mass index and percentage body fat among Asian children from different backgrounds. Br J Nutr. 106(9):1390–7. 10.1017/S0007114511001681 [PubMed: 21736824] [CrossRef]
- Liu KY, Chow JM, Sherry C (2013). Early life obesity and diabetes: origins in pregnancy. Open J Endocr Metab Dis. 3:1–12. 10.1007/s11154-013-9238-8 [CrossRef]
- Livingstone MB, Black AE (2003). Markers of the validity of reported energy intake. J Nutr. 133(Suppl 3):895S–920S. [PubMed: 12612176]
- Locke AE, Kahali B, Berndt SI, Justice AE, Pers TH, Day FR, et al.; LifeLines Cohort Study; ADIPOGen Consortium; AGEN-BMI Working Group; CARDIOGRAMplusC4D Consortium; CKDGen Consortium; GLGC; ICBP; MAGIC Investigators; MuTHER Consortium; MIGen Consortium; PAGE Consortium; ReproGen Consortium; GENIE Consortium; International Endogene Consortium (2015). Genetic studies of body mass index yield new insights for obesity biology. Nature. 518(7538):197–206. 10.1038/nature14177 [PMC free article: PMC4382211] [PubMed: 25673413] [CrossRef]
- Lohman TG, Chen Z (2005). Dual-energy x-ray absorptiometry. In: Heymsfield SB, Lohman TG, Wang Z, Going SB, editors. Human body composition. 2nd edition. Champaign (IL), USA; Leeds, UK: Human Kinetics Press; pp. 63–77.
- Lopez R, Hynes HP (2003). Sprawl in the 1990s: measurement, distribution, and trends. Urban Aff Rev Thousand Oaks Calif. 38(3):325–55. 10.1177/1078087402238805 [PMC free article: PMC1570399] [PubMed: 16990927] [CrossRef]
- Louzada ML, Baraldi LG, Steele EM, Martins AP, Canella DS, Moubarac JC, et al. (2015). Consumption of ultra-processed foods and obesity in Brazilian adolescents and adults. Prev Med. 81:9–15. 10.1016/j.ypmed.2015.07.018 [PubMed: 26231112] [CrossRef]
- Ludwig J, Sanbonmatsu L, Gennetian L, Adam E, Duncan GJ, Katz LF, et al. (2011). Neighborhoods, obesity, and diabetes – a randomized social experiment. N Engl J Med. 365(16):1509–19. 10.1056/NEJMsa1103216 [PMC free article: PMC3410541] [PubMed: 22010917] [CrossRef]
- Lynch BM, Healy GN, Dunstan DW, Owen N (2010). Sedentary versus inactive: distinctions for disease prevention. Nat Rev Cardiol. 7(11):68c1, author reply, 68-c2. 10.1038/nrcardio.2010.68-c1 [PubMed: 21080592] [CrossRef]
- Mackenbach JD, Rutter H, Compernolle S, Glonti K, Oppert JM, Charreire H, et al. (2014). Obesogenic environments: a systematic review of the association between the physical environment and adult weight status, the SPOTLIGHT project. BMC Public Health. 14(1):233. 10.1186/1471-2458-14-233 [PMC free article: PMC4015813] [PubMed: 24602291] [CrossRef]
- Magee L, Hale L (2012). Longitudinal associations between sleep duration and subsequent weight gain: a systematic review. Sleep Med Rev. 16(3):231–41. 10.1016/j.smrv.2011.05.005 [PMC free article: PMC3202683] [PubMed: 21784678] [CrossRef]
- Malik VS, Pan A, Willett WC, Hu FB (2013). Sugar-sweetened beverages and weight gain in children and adults: a systematic review and meta-analysis. Am J Clin Nutr. 98(4):1084–102. 10.3945/ajcn.113.058362 [PMC free article: PMC3778861] [PubMed: 23966427] [CrossRef]
- Marchesi JR, Adams DH, Fava F, Hermes GD, Hirschfield GM, Hold G, et al. (2016). The gut microbiota and host health: a new clinical frontier. Gut. 65(2):330–9. 10.1136/gutjnl-2015-309990 [PMC free article: PMC4752653] [PubMed: 26338727] [CrossRef]
- Maresca MM, Hoepner LA, Hassoun A, Oberfield SE, Mooney SJ, Calafat AM, et al. (2015). Prenatal exposure to phthalates and childhood body size in an urban cohort. Environ Health Perspect. 124(4). 10.1289/ehp.1408750 [PMC free article: PMC4829975] [PubMed: 26069025] [CrossRef]
- Marshall NS, Glozier N, Grunstein RR (2008). Is sleep duration related to obesity? A critical review of the epidemiological evidence. Sleep Med Rev. 12(4):289–98. 10.1016/j.smrv.2008.03.001 [PubMed: 18485764] [CrossRef]
- Martínez Steele E, Baraldi LG, Louzada ML, Moubarac JC, Mozaffarian D, Monteiro CA (2016). Ultra-processed foods and added sugars in the US diet: evidence from a nationally representative cross-sectional study. BMJ Open. 6(3):e009892. 10.1136/bmjopen-2015-009892 [PMC free article: PMC4785287] [PubMed: 26962035] [CrossRef]
- McCrory MA (2014). Meal skipping and variables related to energy balance in adults: a brief review, with emphasis on the breakfast meal. Physiol Behav. 134:51–4. 10.1016/j.physbeh.2014.05.005 [PubMed: 24825781] [CrossRef]
- McCrory MA, Fuss PJ, Hays NP, Vinken AG, Greenberg AS, Roberts SB (1999). Overeating in America: association between restaurant food consumption and body fatness in healthy adult men and women ages 19 to 80. Obes Res. 7(6):564–71. 10.1002/j.1550-8528.1999.tb00715.x [PubMed: 10574515] [CrossRef]
- McFadden E, Jones ME, Schoemaker MJ, Ashworth A, Swerdlow AJ (2014). The relationship between obesity and exposure to light at night: cross-sectional analyses of over 100,000 women in the Breakthrough Generations Study. Am J Epidemiol. 180(3):245–50. 10.1093/aje/kwu117 [PubMed: 24875371] [CrossRef]
- McKinnon L, Giskes K, Turrell G (2014). The contribution of three components of nutrition knowledge to socio-economic differences in food purchasing choices. Public Health Nutr. 17(8):1814–24. 10.1017/S1368980013002036 [PMC free article: PMC10282305] [PubMed: 23920283] [CrossRef]
- McPheeters ML, Warren Z, Sathe N, Bruzek JL, Krishnaswami S, Jerome RN, et al. (2011). A systematic review of medical treatments for children with autism spectrum disorders. Pediatrics. 127(5):e1312–21. 10.1542/peds.2011-0427 [PubMed: 21464191] [CrossRef]
- Miller CT, Fraser SF, Levinger I, Straznicky NE, Dixon JB, Reynolds J, et al. (2013). The effects of exercise training in addition to energy restriction on functional capacities and body composition in obese adults during weight loss: a systematic review. PLoS One. 8(11):e81692. 10.1371/journal.pone.0081692 [PMC free article: PMC3884087] [PubMed: 24409219] [CrossRef]
- Monasta L, Batty GD, Cattaneo A, Lutje V, Ronfani L, Van Lenthe FJ, et al. (2010). Early-life determinants of overweight and obesity: a review of systematic reviews. Obes Rev. 11(10):695–708. 10.1111/j.1467-789X.2010.00735.x [PubMed: 20331509] [CrossRef]
- Monteiro CA, Conde WL, Lu B, Popkin BM (2004a). Obesity and inequities in health in the developing world. Int J Obes Relat Metab Disord. 28(9):1181–6. 10.1038/sj.ijo.0802716 [PubMed: 15211362] [CrossRef]
- Monteiro CA, Moura EC, Conde WL, Popkin BM (2004b). Socioeconomic status and obesity in adult populations of developing countries: a review. Bull World Health Organ. 82(12):940–6. [PMC free article: PMC2623095] [PubMed: 15654409]
- Moore CJ, Cunningham SA (2012). Social position, psychological stress, and obesity: a systematic review. J Acad Nutr Diet. 112(4):518–26. 10.1016/j.jand.2011.12.001 [PubMed: 22709702] [CrossRef]
- Mozaffarian D, Afshin A, Benowitz NL, Bittner V, Daniels SR, Franch HA, et al.; American Heart Association Council on Epidemiology and Prevention, Council on Nutrition, Physical Activity and Metabolism, Council on Clinical Cardiology, Council on Cardiovascular Disease in the Young, Council on the Kidney in Cardiovascular Disease, Council on Peripheral Vascular Disease, and the Advocacy Coordinating Committee (2012). Population approaches to improve diet, physical activity, and smoking habits: a scientific statement from the American Heart Association. Circulation. 126(12):1514–63. 10.1161/CIR.0b013e318260a20b [PMC free article: PMC3881293] [PubMed: 22907934] [CrossRef]
- Mozaffarian D, Hao T, Rimm EB, Willett WC, Hu FB (2011). Changes in diet and lifestyle and long-term weight gain in women and men. N Engl J Med. 364(25):2392–404. 10.1056/NEJMoa1014296 [PMC free article: PMC3151731] [PubMed: 21696306] [CrossRef]
- Murray CJ, Kulkarni S, Ezzati M (2005). Eight Americas: new perspectives on U.S. health disparities. Am J Prev Med. 29(5 Suppl 1):4–10. 10.1016/j.amepre.2005.07.031 [PubMed: 16389119] [CrossRef]
- Must A, Willett WC, Dietz WH (1993). Remote recall of childhood height, weight, and body build by elderly subjects. Am J Epidemiol. 138(1):56–64. [PubMed: 8333427]
- Nathan PC, Jovcevska V, Ness KK, Mammone D’Agostino N, Staneland P, Urbach SL, et al. (2006). The prevalence of overweight and obesity in pediatric survivors of cancer. J Pediatr. 149(4):518–25. 10.1016/j.jpeds.2006.06.039 [PubMed: 17011325] [CrossRef]
- National Obesity Observatory (2011). Obesity and ethnicity. Available from: http://webarchive
.nationalarchives .gov.uk /20160805121933/http://www .noo.org.uk/uploads /doc/vid_9851_Obesity_ethnicity .pdf. - National Obesity Observatory (2012). NOO data factsheet: child obesity and socioeconomic status. Available from: http://webarchive
.nationalarchives .gov.uk /20160805121933/http://www .noo.org.uk/uploads /doc/vid_16967 _ChildSocioeconSep2012.pdf. - NCD Risk Factor Collaboration (2016). Trends in adult body-mass index in 200 countries from 1975 to 2014: a pooled analysis of 1698 population-based measurement studies with 19.2 million participants. Lancet. 387(10026):1377–96. 10.1016/S0140-6736(16)30054-X [PMC free article: PMC7615134] [PubMed: 27115820] [CrossRef]
- Ndahimana D, Kim EK (2017). Measurement methods for physical activity and energy expenditure: a review. Clin Nutr Res. 6(2):68–80. 10.7762/cnr.2017.6.2.68 [PMC free article: PMC5426207] [PubMed: 28503503] [CrossRef]
- Neamat-Allah J, Wald D, Hüsing A, Teucher B, Wendt A, Delorme S, et al. (2014). Validation of anthropometric indices of adiposity against whole-body magnetic resonance imaging – a study within the German European Prospective Investigation into Cancer and Nutrition (EPIC) cohorts. PLoS One. 9(3):e91586. 10.1371/journal.pone.0091586 [PMC free article: PMC3953447] [PubMed: 24626110] [CrossRef]
- Neville CE, McKinley MC, Holmes VA, Spence D, Woodside JV (2014). The relationship between breastfeeding and postpartum weight change – a systematic review and critical evaluation. Int J Obes (Lond). 38(4):577–90. 10.1038/ijo.2013.132 [PubMed: 23892523] [CrossRef]
- Newby PK, Muller D, Hallfrisch J, Qiao N, Andres R, Tucker KL (2003). Dietary patterns and changes in body mass index and waist circumference in adults. Am J Clin Nutr. 77(6):1417–25. [PubMed: 12791618]
- Ng M, Fleming T, Robinson M, Thomson B, Graetz N, Margono C, et al. (2014). Global, regional, and national prevalence of overweight and obesity in children and adults during 1980–2013: a systematic analysis for the Global Burden of Disease Study 2013. Lancet. 384(9945):766–81. 10.1016/S0140-6736(14)60460-8 [PMC free article: PMC4624264] [PubMed: 24880830] [CrossRef]
- Ng SW, Popkin BM, Popkin BM (2012). Time use and physical activity: a shift away from movement across the globe. Obes Rev. 13(8):659–80. 10.1111/j.1467-789X.2011.00982.x [PMC free article: PMC3401184] [PubMed: 22694051] [CrossRef]
- NHLBI Obesity Education Initiative (2000). The practical guide: identification, evaluation, and treatment of overweight and obesity in adults. Bethesda (MD), USA: National Heart, Lung, and Blood Institute, National Institutes of Health. Available from: https://www
.nhlbi.nih .gov/files/docs/guidelines/prctgd_c.pdf. - Nichols HB, Trentham-Dietz A, Egan KM, Titus-Ernstoff L, Holmes MD, Bersch AJ, et al. (2009). Body mass index before and after breast cancer diagnosis: associations with all-cause, breast cancer, and cardiovascular disease mortality. Cancer Epidemiol Biomarkers Prev. 18(5):1403–9. 10.1158/1055-9965.EPI-08-1094 [PMC free article: PMC2715918] [PubMed: 19366908] [CrossRef]
- Obesity Society (2014). Americans’ view on obesity is changing: fewer adults see it as a personal problem of bad choices. Science Daily, 5 November 2014. Available from: https://www
.sciencedaily .com/releases/2014/11/141105112514 .htm. - Ogden CL, Carroll MD, Curtin LR, McDowell MA, Tabak CJ, Flegal KM (2006). Prevalence of overweight and obesity in the United States, 1999–2004. JAMA. 295(13):1549–55. 10.1001/jama.295.13.1549 [PubMed: 16595758] [CrossRef]
- Ogden CL, Carroll MD, Kit BK, Flegal KM (2014). Prevalence of childhood and adult obesity in the United States, 2011–2012. JAMA. 311(8):806–14. 10.1001/jama.2014.732 [PMC free article: PMC4770258] [PubMed: 24570244] [CrossRef]
- Okorodudu DO, Jumean MF, Montori VM, Romero-Corral A, Somers VK, Erwin PJ, et al. (2010). Diagnostic performance of body mass index to identify obesity as defined by body adiposity: a systematic review and meta-analysis. Int J Obes. 34(5):791–9. 10.1038/ijo.2010.5 [PubMed: 20125098] [CrossRef]
- Opalinski A (2013). Cultural perspectives of childhood obesity in Haitan and Hispanic children. J Nurse Pract. 9(3):149–54. 10.1016/j.nurpra.2012.10.009 [CrossRef]
- Owen N, Sparling PB, Healy GN, Dunstan DW, Matthews CE (2010). Sedentary behavior: emerging evidence for a new health risk. Mayo Clin Proc. 85(12):1138–41. 10.4065/mcp.2010.0444 [PMC free article: PMC2996155] [PubMed: 21123641] [CrossRef]
- Paeratakul S, Ferdinand DP, Champagne CM, Ryan DH, Bray GA (2003). Fast-food consumption among US adults and children: dietary and nutrient intake profile. J Am Diet Assoc. 103(10):1332–8. 10.1016/S0002-8223(03)01086-1 [PubMed: 14520253] [CrossRef]
- Palmer JR, Kipping-Ruane K, Wise LA, Yu J, Rosenberg L (2015). Lactation in relation to long-term maternal weight gain in African-American women. Am J Epidemiol. 181(12):932–9. 10.1093/aje/kwv027 [PMC free article: PMC4462338] [PubMed: 25944887] [CrossRef]
- Pampel FC, Denney JT, Krueger PM (2012). Obesity, SES, and economic development: a test of the reversal hypothesis. Soc Sci Med. 74(7):1073–81. 10.1016/j.socscimed.2011.12.028 [PMC free article: PMC3298576] [PubMed: 22341204] [CrossRef]
- Papas MA, Alberg AJ, Ewing R, Helzlsouer KJ, Gary TL, Klassen AC (2007). The built environment and obesity. Epidemiol Rev. 29(1):129–43. 10.1093/epirev/mxm009 [PubMed: 17533172] [CrossRef]
- Parizkova J, Chin MK, Chia M, Yang J (2007). An international perspective on obesity, health and physical activity: current trends and challenges in China and Asia. J Exerc Sci Fit. 5(1):7–23.
- Parsons TJ, Power C, Logan S, Summerbell CD (1999). Childhood predictors of adult obesity: a systematic review. Int J Obes Relat Metab Disord. 23(Suppl 8):S1–107. [PubMed: 10641588]
- Patel SR, Hu FB (2008). Short sleep duration and weight gain: a systematic review. Obesity (Silver Spring). 16(3):643–53. 10.1038/oby.2007.118 [PMC free article: PMC2723045] [PubMed: 18239586] [CrossRef]
- Peña MM, Dixon B, Taveras EM (2012). Are you talking to ME? The importance of ethnicity and culture in childhood obesity prevention and management. Child Obes. 8(1):23–7. [PMC free article: PMC3647541] [PubMed: 22799474]
- Pereira MA, Kartashov AI, Ebbeling CB, Van Horn L, Slattery ML, Jacobs DR Jr, et al. (2005). Fast-food habits, weight gain, and insulin resistance (the CARDIA study): 15-year prospective analysis. Lancet. 365(9453):36–42. 10.1016/S0140-6736(04)17663-0 [PubMed: 15639678] [CrossRef]
- Pestana D, Faria G, Sá C, Fernandes VC, Teixeira D, Norberto S, et al. (2014). Persistent organic pollutant levels in human visceral and subcutaneous adipose tissue in obese individuals – depot differences and dysmetabolism implications. Environ Res. 133:170–7. 10.1016/j.envres.2014.05.026 [PubMed: 24949816] [CrossRef]
- Popkin BM (1993). Nutritional patterns and transitions. Popul Dev Rev. 19(1):138–57. 10.2307/2938388 [CrossRef]
- Popkin BM, Richards MK, Montiero CA (1996). Stunting is associated with overweight in children of four nations that are undergoing the nutrition transition. J Nutr. 126(12):3009–16. [PubMed: 9001368]
- Poppitt SD (2015). Beverage consumption: are alcoholic and sugary drinks tipping the balance towards overweight and obesity? Nutrients. 7(8):6700–18. 10.3390/nu7085304 [PMC free article: PMC4555143] [PubMed: 26270675] [CrossRef]
- Poskitt EME, Breda J (2012). Complementary feeding and non communicable diseases: current knowledge and future research needs. Nutr Metab Cardiovasc Dis. 22(10):819–22. 10.1016/j.numecd.2012.08.005 [PubMed: 22917600] [CrossRef]
- Power C, Atherton K, Thomas C (2010). Maternal smoking in pregnancy, adult adiposity and other risk factors for cardiovascular disease. Atherosclerosis. 211(2):643–8. 10.1016/j.atherosclerosis.2010.03.015 [PubMed: 20400081] [CrossRef]
- Prentice AM, Jebb SA (2001). Beyond body mass index. Obes Rev. 2(3):141–7. 10.1046/j.1467-789x.2001.00031.x [PubMed: 12120099] [CrossRef]
- Puhl R, Brownell KD (2001). Bias, discrimination, and obesity. Obes Res. 9(12):788–805. 10.1038/oby.2001.108 [PubMed: 11743063] [CrossRef]
- Rahat-Rozenbloom S, Fernandes J, Gloor GB, Wolever TM (2014). Evidence for greater production of colonic short-chain fatty acids in overweight than lean humans. Int J Obes. 38(12):1525–31. 10.1038/ijo.2014.46 [PMC free article: PMC3970979] [PubMed: 24642959] [CrossRef]
- Rantakokko P, Main KM, Wohlfart-Veje C, Kiviranta H, Airaksinen R, Vartiainen T, et al. (2014). Association of placenta organotin concentrations with growth and ponderal index in 110 newborn boys from Finland during the first 18 months of life: a cohort study. Environ Health. 13(1):45. 10.1186/1476-069X-13-45 [PMC free article: PMC4061538] [PubMed: 24899383] [CrossRef]
- Reddon H, Guéant JL, Meyre D (2016). The importance of gene-environment interactions in human obesity. Clin Sci (Lond). 130(18):1571–97. 10.1042/CS20160221 [PMC free article: PMC6381863] [PubMed: 27503943] [CrossRef]
- Rohan EA, Townsend JS, Fairley TL, Stewart SL (2015). Health behaviors and quality of life among colorectal cancer survivors. J Natl Compr Canc Netw. 13(3):297–302. [PMC free article: PMC4597894] [PubMed: 25736006]
- Romieu I, Escamilla-Núñez MC, Sánchez-Zamorano LM, Lopez-Ridaura R, Torres-Mejía G, Yunes EM, et al. (2012). The association between body shape silhouette and dietary pattern among Mexican women. Public Health Nutr. 15(1):116–25. 10.1017/S1368980011001182 [PubMed: 21875454] [CrossRef]
- Ross R, Janssen I (2005). Computed tomography and magnetic resonance imaging. In: Heymsfield SB, Lohman TG, Wang Z, Going SB, editors. Human body composition. 2nd edition. Champaign (IL), USA; Leeds, UK: Human Kinetics Press; pp. 89–108.
- Rummel-Kluge C, Komossa K, Schwarz S, Hunger H, Schmid F, Lobos CA, et al. (2010). Head-to-head comparisons of metabolic side effects of second generation antipsychotics in the treatment of schizophrenia: a systematic review and meta-analysis. Schizophr Res. 123(2–3):225–33. 10.1016/j.schres.2010.07.012 [PMC free article: PMC2957510] [PubMed: 20692814] [CrossRef]
- Rundle A, Hoepner L, Hassoun A, Oberfield S, Freyer G, Holmes D, et al. (2012). Association of childhood obesity with maternal exposure to ambient air polycyclic aromatic hydrocarbons during pregnancy. Am J Epidemiol. 175(11):1163–72. 10.1093/aje/kwr455 [PMC free article: PMC3491973] [PubMed: 22505764] [CrossRef]
- Rush EC, Freitas I, Plank LD (2009). Body size, body composition and fat distribution: comparative analysis of European, Maori, Pacific Island and Asian Indian adults. Br J Nutr. 102(4):632–41. 10.1017/S0007114508207221 [PubMed: 19203416] [CrossRef]
- Scientific Advisory Committee on Nutrition (2011). The influence of maternal, fetal and child nutrition on the development of chronic disease later in life. London, UK: TSO. Available from: https://www
.gov.uk/government /publications /sacn-early-life-nutrition-report. - Scientific Advisory Committee on Nutrition (2015). Carbohydrates and health. London, UK: TSO. Available from: https://www
.gov.uk/government /uploads/system /uploads/attachment_data /file/445503 /SACN_Carbohydrates_and_Health.pdf. - Sallis JF, Floyd MF, Rodríguez DA, Saelens BE (2012). Role of built environments in physical activity, obesity, and cardiovascular disease. Circulation. 125(5):729–37. 10.1161/CIRCULATIONAHA.110.969022 [PMC free article: PMC3315587] [PubMed: 22311885] [CrossRef]
- Sanmiguel C, Gupta A, Mayer EA (2015). Gut microbiome and obesity: a plausible explanation for obesity. Curr Obes Rep. 4(2):250–61. 10.1007/s13679-015-0152-0 [PMC free article: PMC4443745] [PubMed: 26029487] [CrossRef]
- Saris WH, Blair SN, van Baak MA, Eaton SB, Davies PS, Di Pietro L, et al. (2003). How much physical activity is enough to prevent unhealthy weight gain? Outcome of the IASO 1st Stock Conference and consensus statement. Obes Rev. 4(2):101–14. 10.1046/j.1467-789X.2003.00101.x [PubMed: 12760445] [CrossRef]
- Schellong K, Schulz S, Harder T, Plagemann A (2012). Birth weight and long-term overweight risk: systematic review and a meta-analysis including 643,902 persons from 66 studies and 26 countries globally. PLoS One. 7(10):e47776. 10.1371/journal.pone.0047776 [PMC free article: PMC3474767] [PubMed: 23082214] [CrossRef]
- Schulze MB, Fung TT, Manson JE, Willett WC, Hu FB (2006). Dietary patterns and changes in body weight in women. Obesity (Silver Spring). 14(8):1444–53. 10.1038/oby.2006.164 [PubMed: 16988088] [CrossRef]
- Schutz Y, Byrne NM, Dulloo A, Hills AP (2014). Energy gap in the aetiology of body weight gain and obesity: a challenging concept with a complex evaluation and pitfalls. Obes Facts. 7(1):15–25. 10.1159/000357846 [PMC free article: PMC5644816] [PubMed: 24457473] [CrossRef]
- Schwingshackl L, Dias S, Strasser B, Hoffmann G (2013). Impact of different training modalities on anthropometric and metabolic characteristics in overweight/obese subjects: a systematic review and network meta-analysis. PLoS One. 8(12):e82853. 10.1371/journal.pone.0082853 [PMC free article: PMC3866267] [PubMed: 24358230] [CrossRef]
- Seidell JC, Visscher TL (2000). Body weight and weight change and their health implications for the elderly. Eur J Clin Nutr. 54(Suppl 3):S33–9. 10.1038/sj.ejcn.1601023 [PubMed: 11041073] [CrossRef]
- Shah RV, Allison MA, Lima JA, Abbasi SA, Eisman A, Lai C, et al. (2016). Abdominal fat radiodensity, quantity and cardiometabolic risk: the Multi-Ethnic Study of Atherosclerosis. Nutr Metab Cardiovasc Dis. 26(2):114–22. [PMC free article: PMC4775418] [PubMed: 26817938]
- Shaw K, Gennat H, O’Rourke P, Del Mar C (2006). Exercise for overweight or obesity. Cochrane Database Syst Rev. (4):CD003817. [PMC free article: PMC9017288] [PubMed: 17054187]
- Sherry B, Jefferds ME, Grummer-Strawn LM (2007). Accuracy of adolescent self-report of height and weight in assessing overweight status: a literature review. Arch Pediatr Adolesc Med. 161(12):1154–61. 10.1001/archpedi.161.12.1154 [PubMed: 18056560] [CrossRef]
- Shrewsbury V, Wardle J (2008). Socioeconomic status and adiposity in childhood: a systematic review of cross-sectional studies 1990–2005. Obesity (Silver Spring). 16(2):275–84. 10.1038/oby.2007.35 [PubMed: 18239633] [CrossRef]
- Sikorski C, Luppa M, Kaiser M, Glaesmer H, Schomerus G, König HH, et al. (2011). The stigma of obesity in the general public and its implications for public health – a systematic review. BMC Public Health. 11(1):661. 10.1186/1471-2458-11-661 [PMC free article: PMC3175190] [PubMed: 21859493] [CrossRef]
- Simkin-Silverman LR, Wing RR, Boraz MA, Kuller LH (2003). Lifestyle intervention can prevent weight gain during menopause: results from a 5-year randomized clinical trial. Ann Behav Med. 26(3):212–20. 10.1207/S15324796ABM2603_06 [PubMed: 14644697] [CrossRef]
- Simmonds M, Burch J, Llewellyn A, Griffiths C, Yang H, Owen C, et al. (2015). The use of measures of obesity in childhood for predicting obesity and the development of obesity-related diseases in adulthood: a systematic review and meta-analysis. Health Technol Assess. 19(43):1–336. 10.3310/hta19430 [PMC free article: PMC4781104] [PubMed: 26108433] [CrossRef]
- Singh AS, Mulder C, Twisk JW, van Mechelen W, Chinapaw MJ (2008). Tracking of childhood overweight into adulthood: a systematic review of the literature. Obes Rev. 9(5):474–88. 10.1111/j.1467-789X.2008.00475.x [PubMed: 18331423] [CrossRef]
- Singh M (2014). Mood, food, and obesity. Front Psychol. 5:925. 10.3389/fpsyg.2014.00925 [PMC free article: PMC4150387] [PubMed: 25225489] [CrossRef]
- Snijder MB, van Dam RM, Visser M, Seidell JC (2006). What aspects of body fat are particularly hazardous and how do we measure them? Int J Epidemiol. 35(1):83–92. 10.1093/ije/dyi253 [PubMed: 16339600] [CrossRef]
- Sørensen TI, Stunkard AJ, Teasdale TW, Higgins MW (1983). The accuracy of reports of weight: children’s recall of their parents’ weights 15 years earlier. Int J Obes. 7(2):115–22. [PubMed: 6862758]
- Spahlholz J, Baer N, König HH, Riedel-Heller SG, Luck-Sikorski C (2016). Obesity and discrimination – a systematic review and meta-analysis of observational studies. Obes Rev. 17(1):43–55. 10.1111/obr.12343 [PubMed: 26596238] [CrossRef]
- Speliotes EK, Willer CJ, Berndt SI, Monda KL, Thorleifsson G, Jackson AU, et al.; MAGIC; Procardis Consortium (2010). Association analyses of 249,796 individuals reveal 18 new loci associated with body mass index. Nat Genet. 42(11):937–48. 10.1038/ng.686 [PMC free article: PMC3014648] [PubMed: 20935630] [CrossRef]
- Steinberger J, Jacobs DR, Raatz S, Moran A, Hong CP, Sinaiko AR (2005). Comparison of body fatness measurements by BMI and skinfolds vs dual energy X-ray absorptiometry and their relation to cardiovascular risk factors in adolescents. Int J Obes (Lond). 29(11):1346–52. 10.1038/sj.ijo.0803026 [PubMed: 16044176] [CrossRef]
- Sun G, French CR, Martin GR, Younghusband B, Green RC, Xie YG, et al. (2005). Comparison of multifrequency bioelectrical impedance analysis with dual-energy X-ray absorptiometry for assessment of percentage body fat in a large, healthy population. Am J Clin Nutr. 81(1):74–8. [PubMed: 15640463]
- Swinburn B, Sacks G, Ravussin E (2009). Increased food energy supply is more than sufficient to explain the US epidemic of obesity. Am J Clin Nutr. 90(6):1453–6. 10.3945/ajcn.2009.28595 [PubMed: 19828708] [CrossRef]
- Szewczyk-Golec K, Woźniak A, Reiter RJ (2015). Inter-relationships of the chronobiotic, melatonin, with leptin and adiponectin: implications for obesity. J Pineal Res. 59(3):277–91. 10.1111/jpi.12257 [PubMed: 26103557] [CrossRef]
- Tang-Péronard JL, Heitmann BL, Andersen HR, Steuerwald U, Grandjean P, Weihe P, et al. (2014). Association between prenatal polychlorinated biphenyl exposure and obesity development at ages 5 and 7 y: a prospective cohort study of 656 children from the Faroe Islands. Am J Clin Nutr. 99(1):5–13. 10.3945/ajcn.113.066720 [PMC free article: PMC3862459] [PubMed: 24153349] [CrossRef]
- Tang-Péronard JL, Jensen TK, Andersen HR, Ried-Larsen M, Grøntved A, Andersen LB, et al. (2015). Associations between exposure to persistent organic pollutants in childhood and overweight up to 12 years later in a low exposed Danish population. Obes Facts. 8(4):282–92. 10.1159/000438834 [PMC free article: PMC5644795] [PubMed: 26228100] [CrossRef]
- Te Morenga L, Mallard S, Mann J (2012). Dietary sugars and body weight: systematic review and meta-analyses of randomised controlled trials and cohort studies. BMJ. 346:e7492. 10.1136/bmj.e7492 [PubMed: 23321486] [CrossRef]
- Thomée S, Lissner L, Hagberg M, Grimby-Ekman A (2015). Leisure time computer use and overweight development in young adults – a prospective study. BMC Public Health. 15(1):839. 10.1186/s12889-015-2131-5 [PMC free article: PMC4556216] [PubMed: 26329006] [CrossRef]
- Thompson MA, Gray JJ (1995). Development and validation of a new body-image assessment scale. J Pers Assess. 64(2):258–69. 10.1207/s15327752jpa6402_6 [PubMed: 7722852] [CrossRef]
- Thorogood A, Mottillo S, Shimony A, Filion KB, Joseph L, Genest J, et al. (2011). Isolated aerobic exercise and weight loss: a systematic review and meta-analysis of randomized controlled trials. Am J Med. 124(8):747–55. 10.1016/j.amjmed.2011.02.037 [PubMed: 21787904] [CrossRef]
- Tobi EW, Goeman JJ, Monajemi R, Gu H, Putter H, Zhang Y, et al. (2014). DNA methylation signatures link prenatal famine exposure to growth and metabolism. Nat Commun. 5:5592. 10.1038/ncomms6592 [PMC free article: PMC4246417] [PubMed: 25424739] [CrossRef]
- Tobias DK, Chen M, Manson JE, Ludwig DS, Willett W, Hu FB (2015). Effect of low-fat diet interventions versus other diet interventions on long-term weight change in adults: a systematic review and meta-analysis. Lancet Diabetes Endocrinol. 3(12):968–79. 10.1016/S2213-8587(15)00367-8 [PMC free article: PMC4667723] [PubMed: 26527511] [CrossRef]
- Tompkins CL, Seablom M, Brock DW (2015). Parental perception of child’s body weight: a systematic review. J Child Fam Stud. 24(5):1384–91. 10.1007/s10826-014-9945-0 [CrossRef]
- Torloni MR, Betrán AP, Merialdi M (2012). Demography of obesity. In: Gillman MW, Poston L, editors. Maternal obesity. Cambridge, UK: Cambridge University Press; pp. 1–7.
- Trasande L, Attina TM, Blustein J (2012). Association between urinary bisphenol A concentration and obesity prevalence in children and adolescents. JAMA. 308(11):1113–21. 10.1001/2012.jama.11461 [PubMed: 22990270] [CrossRef]
- Troy LM, Hunter DJ, Manson JE, Colditz GA, Stampfer MJ, Willett WC (1995). The validity of recalled weight among younger women. Int J Obes Relat Metab Disord. 19(8):570–2. [PubMed: 7489028]
- Troy LM, Michels KB, Hunter DJ, Spiegelman D, Manson JE, Colditz GA, et al. (1996). Self-reported birthweight and history of having been breastfed among younger women: an assessment of validity. Int J Epidemiol. 25(1):122–7. 10.1093/ije/25.1.122 [PubMed: 8666479] [CrossRef]
- Trumbo PR, Rivers CR (2014). Systematic review of the evidence for an association between sugar-sweetened beverage consumption and risk of obesity. Nutr Rev. 72(9):566–74. 10.1111/nure.12128 [PubMed: 25091794] [CrossRef]
- Ulijaszek SJ, Kerr DA (1999). Anthropometric measurement error and the assessment of nutritional status. Br J Nutr. 82(3):165–77. 10.1017/S0007114599001348 [PubMed: 10655963] [CrossRef]
- UNICEF, WHO, and World Bank Group (2015). Levels and trends in child malnutrition: UNICEF – WHO – World Bank Group joint child malnutrition estimates: key findings of the 2015 edition. New York, USA: United Nations Children’s Fund; Geneva, Switzerland: World Health Organization; Washington (DC), USA: The World Bank. Available from: http://www
.who.int/nutgrowthdb /jme_brochure2015.pdf?ua=1. - Van Cauwenberghe J, Delvaux I, Michels N, Den Hond E, Schoeters G, Nelen V, et al. (2014). Validity of parentally reported versus measured weight, length and waist in 7- to 9-year-old children for use in follow-up studies. Eur J Pediatr. 173(7):921–8. 10.1007/s00431-014-2274-x [PubMed: 24497184] [CrossRef]
- van der Heijden AA, Hu FB, Rimm EB, van Dam RM (2007). A prospective study of breakfast consumption and weight gain among U.S. men. Obesity (Silver Spring). 15(10):2463–9. 10.1038/oby.2007.292 [PubMed: 17925472] [CrossRef]
- Vandevijvere S, Chow CC, Hall KD, Umali E, Swinburn BA (2015). Increased food energy supply as a major driver of the obesity epidemic: a global analysis. Bull World Health Organ. 93(7):446–56. 10.2471/BLT.14.150565 [PMC free article: PMC4490816] [PubMed: 26170502] [CrossRef]
- Vasan RS, Pencina MJ, Cobain M, Freiberg MS, D’Agostino RB (2005). Estimated risks for developing obesity in the Framingham Heart Study. Ann Intern Med. 143(7):473–80. 10.7326/0003-4819-143-7-200510040-00005 [PubMed: 16204159] [CrossRef]
- Villareal DT, Apovian CM, Kushner RF, Klein S; American Society for Nutrition; NAASO, The Obesity Society (2005). Obesity in older adults: technical review and position statement of the American Society for Nutrition and NAASO, The Obesity Society. Am J Clin Nutr. 82(5):923–34. [PubMed: 16280421]
- Volberg V, Harley K, Calafat AM, Davé V, McFadden J, Eskenazi B, et al. (2013). Maternal bisphenol A exposure during pregnancy and its association with adipokines in Mexican-American children. Environ Mol Mutagen. 54(8):621–8. 10.1002/em.21803 [PMC free article: PMC4422176] [PubMed: 23908009] [CrossRef]
- Wagner DR (2013). Ultrasound as a tool to assess body fat. J Obes. 2013:1-9 10.1155/2013/280713 [PMC free article: PMC3770049] [PubMed: 24062944] [CrossRef]
- Wagner DR, Heyward VH (2000). Measures of body composition in blacks and whites: a comparative review. Am J Clin Nutr. 71(6):1392–402. [PubMed: 10837277]
- Wang L, Mamudu HM, Alamian A, Anderson JL, Brooks B (2014). Independent and joint effects of prenatal maternal smoking and maternal exposure to second-hand smoke on the development of adolescent obesity: a longitudinal study. J Paediatr Child Health. 50(11):908–15. 10.1111/jpc.12667 [PubMed: 24920104] [CrossRef]
- Wang T, Li M, Chen B, Xu M, Xu Y, Huang Y, et al. (2012). Urinary bisphenol A (BPA) concentration associates with obesity and insulin resistance. J Clin Endocrinol Metab. 97(2):E223–7. 10.1210/jc.2011-1989 [PubMed: 22090277] [CrossRef]
- Wannamethee SG, Shaper AG, Lennon L (2005). Reasons for intentional weight loss, unintentional weight loss, and mortality in older men. Arch Intern Med. 165(9):1035–40. 10.1001/archinte.165.9.1035 [PubMed: 15883243] [CrossRef]
- Wardle J, Haase AM, Steptoe A (2006). Body image and weight control in young adults: international comparisons in university students from 22 countries. Int J Obes. 30(4):644–51. Available from: http://www
.nature.com /ijo/journal/v30/n4/pdf/0803050a.pdf. 10.1038/sj.ijo.0803050 [PubMed: 16151414] [CrossRef] - WCRF/AICR (2007). Food, nutrition, physical activity, and the prevention of cancer: a global perspective. Washington (DC), USA: American Institute for Cancer Research. Available from: www
.aicr.org/assets/docs /pdf/reports/Second_Expert_Report .pdf. - Weng SF, Redsell SA, Swift JA, Yang M, Glazebrook CP (2012). Systematic review and meta-analyses of risk factors for childhood overweight identifiable during infancy. Arch Dis Child. 97(12):1019–26. 10.1136/archdischild-2012-302263 [PMC free article: PMC3512440] [PubMed: 23109090] [CrossRef]
- Westerterp KR, Speakman JR (2008). Physical activity energy expenditure has not declined since the 1980s and matches energy expenditures of wild mammals. Int J Obes. 32(8):1256–63. 10.1038/ijo.2008.74 [PubMed: 18504442] [CrossRef]
- WHO (2000). Obesity: preventing and managing the global epidemic: report of a WHO Consultation. Geneva, Switzerland: World Health Organization (WHO Technical Report Series, No. 894). Available from: http://www
.who.int/nutrition /publications /obesity/WHO_TRS_894/en/. [PubMed: 11234459] - WHO (2003). Diet, nutrition and the prevention of chronic diseases. Report of a joint WHO/FAO Expert Consultation. Geneva, Switzerland: World Health Organization (WHO Technical Report Series, No. 916). Available from: http://www
.who.int/dietphysicalactivity /publications /trs916/en/. [PubMed: 12768890] - WHO (2008). Closing the gap in a generation: health equity through action on the social determinants of health. Final report of the Commission on Social Determinants of Health. Geneva, Switzerland: World Health Organization. Available from: http://www
.who.int/social_determinants /thecommission /finalreport/en/. - WHO (2011). Global database on body mass index: an interactive surveillance tool for monitoring nutrition transition. Available from: www
.who.int/bmi/index.jsp. - WHO (2014). Obesity and inequities: guidance for addressing inequities in overweight and obesity. Available from: http://www
.euro.who.int /en/publications/abstracts /obesity-and-inequities .-guidance-for-addressing-inequities-in-overweight-and-obesity-2014. - WHO (2015). Information note about intake of sugars recommended in the WHO guideline for adults and children. Available from: http://www
.who.int/nutrition /publications /guidelines/sugar_intake _information_note_en.pdf. - WHO (2016). Obesity and overweight: fact sheet. Available from: http://www
.who.int/mediacentre /factsheets/fs311/en/. - WHO (2017a). WHO child growth standards. Geneva, Switzerland: World Health Organization. Available from: http://www
.who.int/childgrowth /standards/Technical_report .pdf. - WHO (2017b). WHO growth reference data for 5–19 years. Geneva, Switzerland: World Health Organization. Available from: http://www
.who.int/nutrition /publications /growthref_who_bulletin/en/. - WHO Expert Consultation (2004). Appropriate body-mass index for Asian populations and its implications for policy and intervention strategies. Lancet. 363(9403):157–63.10.1016/S0140-6736(03)15268-3 [PubMed: 14726171] [CrossRef]
- WHO Regional Office for Europe (2016). Good maternal nutrition: the best start in life. Copenhagen, Denmark: World Health Organization Regional Office for Europe. Available from: http://www
.euro.who.int /en/health-topics /disease-prevention/nutrition /publications /2016/good-maternal-nutrition .-the-best-start-in-life-2016. - Wijnhoven TM, van Raaij JM, Spinelli A, Starc G, Hassapidou M, Spiroski I, et al. (2014). WHO European Childhood Obesity Surveillance Initiative: body mass index and level of overweight among 6-9-year-old children from school year 2007/2008 to school year 2009/2010. BMC Public Health. 14(1):806. 10.1186/1471-2458-14-806 [PMC free article: PMC4289284] [PubMed: 25099430] [CrossRef]
- Willett W (1998). Nutritional epidemiology. 2nd edition. Oxford, UK: Oxford University Press. 10.1093/acprof:oso/9780195122978.001.0001 [CrossRef]
- Willett W, Hu F (2013). Anthropometric measures and body composition. In: Willett W, editor. Nutritional epidemiology. 3rd edition. New York (NY), USA: Oxford University Press; pp. 213–40.
- Willett WC (2002). Dietary fat plays a major role in obesity: no. Obes Rev. 3(2):59–68. 10.1046/j.1467-789X.2002.00060.x [PubMed: 12120421] [CrossRef]
- Williams SM, Goulding A (2009). Patterns of growth associated with the timing of adiposity rebound. Obesity (Silver Spring). 17(2):335–41. 10.1038/oby.2008.547 [PubMed: 19057527] [CrossRef]
- Williamson DF, Madans J, Anda RF, Kleinman JC, Kahn HS, Byers T (1993). Recreational physical activity and ten-year weight change in a US national cohort. Int J Obes Relat Metab Disord. 17(5):279–86. [PubMed: 8389337]
- Wormser D, Kaptoge S, Di Angelantonio E, Wood AM, Pennells L, Thompson A, et al.; Emerging Risk Factors Collaboration (2011). Separate and combined associations of body-mass index and abdominal adiposity with cardiovascular disease: collaborative analysis of 58 prospective studies. Lancet. 377(9771):1085–95. 10.1016/S0140-6736(11)60105-0 [PMC free article: PMC3145074] [PubMed: 21397319] [CrossRef]
- Zimmet P, Magliano D, Matsuzawa Y, Alberti G, Shaw J (2005). The metabolic syndrome: a global public health problem and a new definition. J Atheroscler Thromb. 12(6):295–300. 10.5551/jat.12.295 [PubMed: 16394610] [CrossRef]
- Zoeller RT, Brown TR, Doan LL, Gore AC, Skakkebaek NE, Soto AM, et al. (2012). Endocrine-disrupting chemicals and public health protection: a statement of principles from The Endocrine Society. Endocrinology. 153(9):4097–110. 10.1210/en.2012-1422 [PMC free article: PMC3423612] [PubMed: 22733974] [CrossRef]
- Body fatness - Absence of excess body fatnessBody fatness - Absence of excess body fatness
- myelodysplasia/myeloid leukemia factor 2 [Homo sapiens]myelodysplasia/myeloid leukemia factor 2 [Homo sapiens]gi|1399745|gb|AAB08988.1|Protein
- Homologene neighbors for GEO Profiles (Select 131499527) (0)GEO Profiles
- Homologene neighbors for GEO Profiles (Select 131484411) (0)GEO Profiles
- Profile neighbors for GEO Profiles (Select 131484411) (200)GEO Profiles
Your browsing activity is empty.
Activity recording is turned off.
See more...