NCBI Bookshelf. A service of the National Library of Medicine, National Institutes of Health.
Hempel S, Suttorp MJ, Miles JNV, et al. Empirical Evidence of Associations Between Trial Quality and Effect Size [Internet]. Rockville (MD): Agency for Healthcare Research and Quality (US); 2011 Jun.
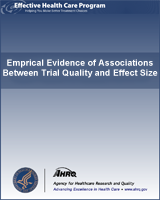
Empirical Evidence of Associations Between Trial Quality and Effect Size [Internet].
Show detailsBackground
Trial design and execution factors are widely believed to be associated with bias. Bias is typically defined as a systematic deviation of an estimate, such as the estimated treatment effect from the true value. More factors have been proposed as associated with bias than have actually been empirically confirmed by systematic examination. There are some conflicting results regarding the association of quality features and effect sizes. Little is known about moderators and confounders that might predict when quality features (or the lack thereof) influence results of research studies.
Objective
The objective of this project was to examine the empirical evidence for associations between a set of proposed quality criteria and estimates of effect sizes in randomized controlled trials using multiple datasets representing a variety of clinical fields and to explore variables potentially influencing the association.
Methods
We applied a set of proposed quality criteria to three large datasets of studies included in a variety of systematic reviews covering a wide range of clinical fields. The first dataset was derived from all Cochrane Back Review Group reviews of nonsurgical treatment for nonspecific low back pain in the Cochrane Library 2005, issue 3; the set included 216 individual trials. For the second dataset we searched prior systematic reviews and meta-analyses conducted by Agency for Healthcare Research and Quality-funded Evidence-based Practice Centers with the goal of assembling a set with a wide range of clinical topics and interventions; this dataset included 165 trials. The third dataset was obtained by replicating a selection of trials used in a published meta-epidemiological study demonstrating associations of quality with the size of treatment effects; this set included 100 trials (79 percent of the original dataset).
The proposed set of 11 quality features comprised the following:
- Generation of the randomization sequence
- Concealment of treatment allocation
- Similarity of baseline values
- Blinding of outcome assessors
- Blinding of care providers
- Blinding of patients
- Acceptable dropout rate and stated reasons for withdrawals
- Intention-to-treat analysis
- Similarity of cointerventions
- Acceptable compliance
- Similar timing of outcome assessment.
In addition we applied the Jadad components and scale, and criteria suggested by Schulz, including allocation concealment, to one of the datasets. The inter-item relationships of the proposed quality criteria were explored using psychometric methods. A multiple indicator multiple cause (MIMIC) factor analysis explored inter-item correlations as well as associations of quality features with reported effect sizes.
We assessed the relationship between quality and effect sizes for individual criteria as well as summary scores. In particular, the use of total quality scores per study with each item adding to a sum score, factor-analytically derived broad quality domains, and the application of different cutoffs for a total quality score was further explored.
We investigated moderators and confounders that affect the association between quality measures and the size of the treatment effect across datasets. In particular, we investigated whether (1) the overall size of the treatment effect of the intervention observed in datasets, (2) the condition being treated, (3) the investigated type of outcome, and (4) the variance in effect sizes across studies moderates or confounds the association between quality and effect sizes.
Results
The average quality levels varied across datasets. Many studies did not report sufficient information to judge the quality of the feature (although quality of reporting increased after the introduction of the Consolidated Standards of Reporting Trials statement). Some individual quality features were substantially intercorrelated, but a total score did not show high overall internal consistency of the 11 quality features (α's = 0.55 to 0.61). A MIMIC factor-analytic model suggested three distinct quality domains; randomization sequence generation and allocation concealment constituted the first factor, the blinding items constituted a second factor, and the third factor was primarily derived from the acceptable dropout rate item.
Allocation concealment was consistently associated with a slightly smaller treatment effect across all three datasets: Effect size differences were −0.08 (95% CI: −0.23, 0.07) in dataset 1 and −0.06 (95% CI: −0.22, 0.11) in dataset 2. The ratio of odds ratios was 0.91 (0.72, 1.14) in the third dataset where only categorical outcome measures were included; hence, we computed odds ratios rather than effect sizes. Other individual criteria results varied across datasets. In dataset 1 the 11 individual quality criteria were consistently associated with a lower effect size, indicating that low-quality studies overestimated treatment effects. Results in dataset 2 showed unexpected results: Higher quality studies reported larger effect sizes in this sample. The third dataset showed some variation across quality criteria.
There was no statistically significant linear association of a summary quality score (derived by equally weighing all 11 quality items) and effect sizes, which would have indicated that the effect size decreased linearly with increased quality. There was also no consistent linear association across datasets for the factor scores.
Applying a cutoff of 5 or 6 quality criteria met (out of a possible 11) differentiated high- and low-quality studies best. Effect size differences were −0.20 in dataset 1. In the third dataset, the ratio of odds ratios were 0.79 (cutoff at 5; 95% CI: 0.63, 0.95) and 0.77 (cutoff at 6; 95% CI: 0.63, 0.99). These associations indicated that low-quality trials tended to overestimate treatment effects. This effect could not be replicated in dataset 2, suggesting the influence of confounders and moderators of the association.
The specific moderators and confounders that were investigated in this report did not sufficiently explain the variation in associations across datasets. When controlling for the mean treatment effect obtained in each included meta-analysis, the differences across datasets in observed associations between quality and effect sizes remained. A stratified analysis for the condition being treated also failed to explain the contrary results observed in dataset 2 compared to the other two datasets; the clinical condition did not appear to confound the underlying association between quality and effect sizes for individual quality criteria, and the interaction effect of condition with total quality score was also not statistically significant. When categorizing the different measures used to show a treatment effect into objective versus more subjective outcomes, the type of outcome did not show statistically significant interaction effects. The variance in effect sizes within datasets varied across the three datasets and may potentially explain differences observed in the association between quality and effect sizes across datasets; this finding should be investigated systematically. Several assumptions can be tested in meta-epidemiological datasets that may help determine when and which quality features lead to biased effect sizes.
Conclusions
The associations between quality features and effect sizes are complex. Effect sizes of individual studies depend on many factors. In two datasets, individual quality items and summary scores of items were associated with differences in effect sizes. This relationship was not found in the remaining dataset. Despite several exploratory analyses, we were not able to explain these differences. The conditions under which quality features and which features lead to biased effect sizes warrant further exploration and factors such as the variance in quality scores and effect sizes will be investigated in a subsequent project.
- Executive Summary - Empirical Evidence of Associations Between Trial Quality and...Executive Summary - Empirical Evidence of Associations Between Trial Quality and Effect Size
- LOC118568795 [Mus musculus]LOC118568795 [Mus musculus]Gene ID:118568795Gene
- FOXI2 [Microcebus murinus]FOXI2 [Microcebus murinus]Gene ID:105880319Gene
- ENSMICG00000012926 AND (alive[prop]) (1)Gene
- LOC100643361 [Bombus terrestris]LOC100643361 [Bombus terrestris]Gene ID:100643361Gene
Your browsing activity is empty.
Activity recording is turned off.
See more...