Readers may use this article as long as the work is properly cited, the use is educational and not for profit, and the work is not altered. See http://creativecommons.org/licenses/by-nc-nd/3.0 for details.
NCBI Bookshelf. A service of the National Library of Medicine, National Institutes of Health.
The introduction of home blood glucose monitoring (BGM) 40 years ago revolutionized diabetes self-management, providing valuable glucose data that have helped many people with diabetes (PWD) improve their glycemic management. In the early 2000s, personal continuous glucose monitoring (CGM) systems also became available, raising questions regarding the future role of BGM. However, for some PWD, particularly many with type 2 diabetes who do not take medications associated with increased hypoglycemia risk, BGM remains more easily accessible and more affordable than CGM and can adequately meet their needs. In addition, PWD who use CGM still need to periodically use BGM. This publication reviews key issues related to the optimal and most cost-effective use of BGM. The authors address the accuracy of modern glucose meters and the recommended frequency of monitoring for people with different types of diabetes and therapeutic regimens. They suggest strategies for using glycemic data to inform therapy adjustments, as well as ways to overcome common barriers to BGM use. They then review the use of BGM in patients who also use CGM and describe the latest related technological innovations, including Cloud-based data management, mobile applications, insulin calculators, and remote and automated counseling systems. The authors conclude that BGM remains an important tool in diabetes management, even as diabetes management technologies continue to evolve.
[PREFACE]
Connected for Life.
Dear ADA Journal Subscriber:
I am pleased to present to you “The Role of Blood Glucose Monitoring in Diabetes Management,” the latest in the American Diabetes Association’s (ADA’s) Clinical Compendia Series. Produced by ADA and supported by unrestricted educational grants from Ascensia and Lifescan, this valuable resource reviews the continuing importance of blood glucose monitoring (BGM) in diabetes management, even in this age of rapid technological advancements.
In her introduction, lead author Ruth S. Weinstock, MD, PhD, FACE, FACP, of Upstate Medical University in Syracuse, NY, notes that, although home BGM revolutionized diabetes self-management four decades ago, the more recent advent of personal continuous glucose monitoring (CGM) systems has raised questions regarding its role moving forward. She and her team of expert coauthors then set out to answer those questions, explaining why BGM remains a useful and cost-effective source of glycemic data for many people with diabetes.
The authors review key issues related to the optimal use of BGM. They discuss the high level of accuracy of modern glucose meters and how that accuracy is measured, and they review current recommendations regarding the frequency and timing of BGM for people with different types of diabetes and therapeutic regimens. They offer specific strategies for making the best use of BGM to improve glycemic management and therapeutic outcomes, as well as tips for overcoming common barriers. They then position BGM in today’s high-tech diabetes landscape, describing the complementary uses of BGM and CGM, as well as the latest blood glucose–related innovations, including Cloud-based data management, mobile apps, insulin calculators, and remote and automated counseling systems. The authors conclude that BGM is, and will continue to be, an important tool in diabetes management, even as technologies continue to evolve.
Sincerely,
Robert A. Gabbay, MD, PhD
Chief Scientific & Medical Officer
American Diabetes Association
[INTRODUCTION]
The introduction of home blood glucose monitoring (BGM) in the late 1970s and regulatory clearance of the first meter for this purpose in 1980 revolutionized the self-care of people with diabetes (PWD) (1–3). Although often referred to as “self-monitoring of blood (or plasma) glucose,” this form of glucose monitoring will be referred to by the more succinct “BGM” in this compendium. Knowing one’s current glucose reading and being able to examine and better understand glucose patterns enable PWD to adjust their food intake, activity, and medications to achieve their glycemic goals.
Over the past 40 years, glucose meters have become ever smaller, more accurate, and more widely available (3). Today, they require less blood and provide readings more rapidly, and the lancets used to perform fingersticks cause less discomfort than ever before. BGM has provided valuable data that have helped many PWD reach their glycemic targets.
In the early 2000s, personal continuous glucose monitoring (CGM) devices became available and are now being used more commonly, especially
For most PWD, CGM is more expensive than BGM. For PWD who do not require frequent daily glucose monitoring to achieve their glycemic goals, particularly those with type 2 diabetes who are not taking medications that commonly cause hypoglycemia, BGM remains more easily accessible and more affordable than CGM and, for many, can fulfill their glucose self-monitoring needs. In addition, PWD who use CGM still need to periodically use BGM, as will be discussed.
In this monograph, we review issues that are crucial to understand to ensure the optimal and most cost-effective use of BGM. These issues include the accuracy of available glucose meters; the recommended frequency of BGM for PWD who have different types of diabetes and whose diabetes management follows different therapeutic approaches; strategies for using BGM to reach glycemic targets; barriers to BGM use and ways to overcome them; the use of BGM in PWD who also use CGM; and technological innovations such as Cloud-based glucose data management systems, mobile applications (apps), insulin calculators, and remote and automated counseling systems that incorporate BGM to improve care.
Overall, BGM remains an important tool for PWD, even as diabetes management technologies continue to evolve. It is our hope that the information presented in this compendium will assist health care providers (HCPs) in guiding their patients on the most appropriate and cost-effective use of BGM.
BGM ACCURACY
Introduced in the 1970s (1,2), capillary BGM replaced urine glucose monitoring and in-office glucose checking, which were neither timely nor actionable. Frequent BGM was the crucial intervention, along with more frequent insulin dosing, of intensive treatment in the landmark Diabetes Control and Complications Trial (4). Capillary glucose measurements gave people with diabetes and those caring for them data to understand variations in glucose levels, with the potential to optimize basal and prandial insulin dosing.
Initial glucose meters were cumbersome and imprecise, and expectations were largely aspirational (5). However, over the years, both accuracy and usability have greatly improved (3). Here, we describe the key measures of accuracy, review the factors contributing to it, and discuss its impact on various stakeholders.
How Accuracy Is Measured
Accuracy is a concept comprising both trueness and precision. Trueness is defined as “closeness of agreement between the average of an infinite number of replicate measured quantity values and a reference quantity value,” whereas precision is defined as “closeness of agreement between indications or measured quantity values obtained by replicate measurements” (6). A device with high degrees of both trueness and precision would be said to be highly accurate because it would likely produce individual results that would be consistently in close agreement with an accepted reference method.
The error grid is a longstanding tool in the evaluation of BGM accuracy. First developed by Clarke et al. (7), it has subsequently been modified by Parkes et al. (8) and others. In this approach, BGM results are plotted against reference results, with grid regions defined to reflect the potential risk severity of incorrect treatment triggered by the measurement error (Figure 1). Device performance is typically reported as the percentage of glucose values in lower-risk zone A or zones A and B. Higher percentages indicate better performance. Because most current meters attain nearly 100% of results in these lower-risk zones, this method has become less useful for comparing glucose meters.

FIGURE 1
Sample error grid.
Guidance from the U.S. Food and Drug Administration (FDA) and the International Organization for Standardizations has been used to set minimum standards for regulatory clearance of glucose meters. These guidelines have become increasingly strict. In 2016, the FDA published criteria for meters for personal use, stating that 95% of results should be within ±15% of the comparator method, and 99% of results should be within ±20% of the comparator across the entire claimed measuring range (9). Devices for point-of-care professional use should achieve 95% of results within ±12% of the comparator method for blood glucose levels >75 mg/dL and within ±12 mg/dL for levels <75 mg/dL; they should also achieve 98% of values within ±15% of the comparator method for blood glucose levels >75 mg/dL and ±15 mg/dL for levels <75 mg/dL across the entire claimed measuring range (10).
Testing within-run and intermediate precision is required to show that, among multiple meters and glucose strip lots measuring a single sample, over time and with multiple devices, results are consistent. These results are best expressed as coefficient of variation, which is calculated from mean and standard deviation (SD). The effects of potential interfering substances and other factors, such as hematocrit and altitude, are expressed as bias (in mg/dL and percentages).
Accuracy data are reported to and reviewed by the FDA before a meter is cleared. However, there is no post-marketing surveillance other than monitoring of reported adverse events with use of the device.
Factors Contributing to Accuracy
For a holistic understanding of accuracy, it is useful to borrow terminology from laboratory science and separate glucose measurement errors into three distinct categories: pre-analytical, analytical, and post-analytical errors. Pre-analytical errors are those that occur before the device makes a measurement; post-analytical errors are those happening after a measurement is made. Many tests of meter accuracy are performed under ideal laboratory conditions and thus may reflect only the analytical accuracy of the device. Most errors found in laboratory testing other than BGM have been reported to be either pre-analytical (46–68%) or post-analytical (18–47%). Indeed, only 7–13% of errors may occur during the analytical phase (Table 1) (11).
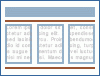
TABLE 1
Examples of Glucose Meter Measurement Errors
Pre-Analytical Factors
Early meters required users to either blot or wipe the glucose strip at a precise time interval. They also required the correct entry of a lot-specific test code. Furthermore, both underfilling and overfilling the glucose strip could have altered test results. Fortunately, these potential sources of pre-analytical error have been mostly eliminated in newer meters by either auto-correction or error detection.
However, poor surface preparation of the capillary sampling site continues to contribute to pre-analytical errors. Despite the known importance of cleansing the site, many users are unaware of or neglect this step. Both fruit handling (12) and the use of some body lotions (13) have been associated with pseudo-hyperglycemia. Additionally, glucose strips damaged by inappropriate storage conditions and the dilutional effect of extraneous fluid at the testing site have been associated with pseudo-hypoglycemia (14). The impact of these factors has increased because of the smaller sample volume required by newer meters.
Thus, although technological advances have addressed many of the pre-analytical sources of reduced accuracy, comprehensive patient education remains important to address remaining pre-analytical issues.
Analytical Factors
Glucose measuring technology has advanced in many ways to meet the demand for greater accuracy. Glucose oxidase has been the most important enzyme used to measure glucose. However, as sensors changed from optical to fully electrochemical, alternative enzymes
have been used. Although these other enzymes have generally improved performance, one—GDH-PQQ (glucose dehydrogenase pyrroloquinoline quinone)—led to harmful hypoglycemia resulting from inappropriate treatment of pseudo-hyperglycemia when maltose, galactose, and xylose were detected nonspecifically as glucose (15).
Other potential sources of error that must be assessed in glucose meters include altitude, hematocrit, ascorbic acid, and acetaminophen. All devices must include results of these tests in their labeling.
In addition to better chemistry, advances in manufacturing techniques, proper storage and distribution, and quality processes to monitor these factors remain crucial to ensuring accuracy. To date, there is no regulatory requirement that manufacturers of cleared meters proactively demonstrate the continued accuracy of their devices after initial clearance.
The Diabetes Technology Society’s comparative study (16) evaluated 18 meters and glucose strips acquired through the retail channel in 1,032 subjects with a total of 5,584 data pairs. This study expanded on previous studies (17) and evaluated all 18 meters at each of three research sites. During each of three substudies, each site evaluated six meters. In each of two subsequent studies, six additional meters were tested in round-robin format such that each site verified all meters. Only six of the 18 meters met accuracy standards (similar to, but slightly more lenient than, current regulatory standards) in all three substudies. These data suggest that real-world meter performance may frequently differ from that initially reported by manufacturers and support the need for post-marketing surveillance to ensure reliable meter accuracy.
Post-Analytical Factors
Visual inspection and comparison of a result with a color chart was commonplace with early meters, and this practice limited accuracy. Essentially all glucose meters now display data digitally to users, but despite this greater clarity, there remain several situations in which the device user may inaccurately interpret the result presented. For example, depending on locale, meters present data in either mg/dL or mmol/L. In the past, users could change the measurement units (although, occasionally, device settings unpredictably changed). Manufacturers no longer allow users to change data units, but someone using a meter that displays results in mmol/L (e.g., a meter acquired in Canada) but who is accustomed to mg/dL units (e.g., someone residing in the United States) might incorrectly interpret 10.0 mmol/L as 100 mg/dL (or vice versa). Low numeracy has also been reported to be a barrier for some users (18).
The advent of meters that store a digital record of glucose results confirmed the persistent suspicion of many clinicians regarding logbooks (19): missing, phantom (“dry-lab”), and incorrectly transcribed readings are quite common. This problem persists today in clinics where manual logbooks are still reviewed.
In addition to missing, fabricated, and incorrectly recorded data, there is short- and long-term potential to underuse or even misuse BGM data. To address this issue, structured BGM (20,21), described in more detail on pages 6-7, has been proposed as a technique to make BGM data more actionable. A further development has been meter connectivity, allowing BGM data to be transmitted via smartphone to the Cloud for enhanced remote medication management.
Innumerable mobile apps that can now accept BGM data and provide insulin dosing advice represent an additional source of post-analytical errors. Unfortunately, these apps vary considerable in quality (22). They also require configuration by programming in a patient’s insulin-to-carbohydrate (I:C) ratio (the number of grams of consumed carbohydrate that 1 unit of insulin will cover), insulin sensitivity factor (ISF; the blood glucose lowering in mg/dL to be expected per unit of insulin delivered), and duration of insulin action, and often sophisticated input from users (e.g., carbohydrate counting). These apps, and other BGM-related innovations, are discussed in more detail starting on p. 18.
Why Accuracy Matters and to Whom
Although it may seem intuitively obvious that more accurate BGM is advantageous, access to any means of BGM is such a formidable barrier in low-resource settings (23) that a broader perspective is needed. Type 1 diabetes, pregnancy, and use of a CGM device that benefits from calibration are all compelling examples of circumstances requiring high BGM accuracy. However, people with type 2 diabetes who are not taking medications likely to cause hypoglycemia may accrue benefit even from less-expensive devices with lower accuracy ratings. In recognition of this, one major manufacturer of meters introduced a semi-quantitative system using a smartphone camera (24). This approach allows a mobile app to photograph a glucose strip and provide an estimated glucose level range, rather than a specific value, and does not require users to purchase a meter. Likewise, a study of a meter that displayed color-coded glucose range data (in addition to a glucose value) demonstrated a salutary effect, particularly in people with low numeracy (25).
For PWD, meter accuracy affects not only insulin dosing decisions, but also confidence in their meter. For example, if glucose is checked multiple times within a short interval and the results differ, trust in the meter may decline. Additionally, accurate BGM is required for calibration of some CGM systems and is also needed for confirmation of CGM results or as a backup for people who use a CGM system. Table 2 summarizes groups of PWD who would benefit from greater BGM accuracy (26).
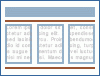
TABLE 2
PWD Requiring the Highest Possible Level of BGM Accuracy
Few prospective data directly address meter accuracy and health economics outcomes. Although it would be unethical to randomize study participants to a less accurate meter, such questions can be and have been posed through simulations. Cost savings have been modeled to occur with more accurate meters in people with type 1 or insulin-treated type 2 diabetes. These savings were primarily driven by reductions in hypoglycemia and hospitalization, with a much smaller measured contribution from reduction of long-term complications (27,28).
Accuracy alone is not enough to improve health outcomes of PWD. Accurate, actionable glucose data must be communicated to automated insulin delivery (AID) systems when used, clinicians, and the majority of PWD who do not yet use AID systems to manage glycemia. In the section starting on p. 18, we will discuss in more detail how technology (e.g., apps, expert systems, and bolus calculators) can make BGM data more actionable and reliance on such information safer and more effective.
RECOMMENDATIONS FOR BGM USE
BGM via capillary blood historically has been considered a cornerstone of diabetes self-care. Touted as a key part of patients’ daily routine, when performed regularly, it can guide insulin dosing and help patients judge whether their noninsulin medications and lifestyle changes are having favorable effects. When the desired results are not achieved, BGM values can be used by PWD and their care team to guide medication adjustments and further lifestyle modifications.
Over the past several decades, BGM technology has evolved, making routine checking more affordable and accessible, and many providers universally recommend daily BGM for all PWD. However, with the advent of newer noninsulin therapeutics that do not cause hypoglycemia and the recognition that daily BGM may be of little value in people with optimally managed type 2 diabetes has come a push to adopt a selective approach to BGM in people with diabetes. In the following sections, we discuss current recommendations for BGM in people living with diabetes (26,29), which are also summarized in Table 3.
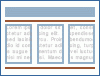
TABLE 3
BGM Frequency by Type of Diabetes and Treatment Regimen: General Recommendations
One way to determine who might benefit most from routine BGM is to consider individual patient factors such as insulin use, pregnancy, hypoglycemia history, hypoglycemia unawareness, and blood glucose levels that are difficult to normalize. Although our intent is to convey best practices supported by high-quality data, it is important to recognize that, in many cases, there is no one correct answer and that a personalized approach to BGM is recommended.
People with Type 1 Diabetes
Although the use of CGM is increasing, some people living with type 1 diabetes will choose to continue with daily BGM exclusively. Reasons some patients may decide to continue to use BGM include higher costs of CGM systems, unacceptability of being connected to a device, and overload with regard to the quantity of data CGM provides (30).
Given the day-to-day glycemic variability that people with type 1 diabetes typically experience, recommended BGM frequency per day will vary by person and anticipated daily activities. At a minimum, it is recommended that people with type 1 diabetes who are not using CGM perform BGM 4 times/day (before meals and at bedtime). Other times people with type 1 diabetes should check their blood glucose level include before exercise, during prolonged exercise, occasionally after meals, and whenever they have signs or symptoms of hypoglycemia. For people who have hypoglycemia unawareness, performing BGM before activities that require clear cognition (e.g., driving, caring for children, or operating heavy machinery) should be the norm. More frequent BGM is also needed during acute illness or times of stress. Thus, for most people with type 1 diabetes who are not using CGM, performing BGM 4–10 times/day as needed is recommended. It is essential, however, to recognize the need to personalize this recommendation for each person based on the presence of complications and variability in daily life.
Another situation in which people with type 1 diabetes must perform BGM is to calibrate certain CGM systems. Although newer systems do not require such calibration, some people still may be using older CGM models that do or the Medtronic 670G system with the Guardian sensor, which also requires BGM calibration a minimum of twice daily. Additionally, PWD who are using CGM may need to perform BGM on occasion to confirm extreme hyperglycemia or hypoglycemia measured via CGM or when they suspect CGM sensor or transmitter malfunction.
People with Type 2 Diabetes
Those Who Do Not Use Insulin
The role of BGM for people with type 2 diabetes who are not using insulin is one of the more contested issues in diabetes management. Some previous trials have shown benefit of BGM on glycemic outcomes (31–34), whereas others have shown no such benefit (35–38) in this population.
Of the studies that have shown benefit, using a structured approach and ensuring that both PWD and providers review the results has proven to be the most efficacious strategy. Examples of structured approaches include paired checking and intermittent, frequent checking over a period of several days before a clinical visit (39). These studies have been rigorous, controlled clinical trials with close participant follow-up; thus, the beneficial results may be difficult to realize in busy, real-world clinical practice settings.
Although BGM may increase patients’ awareness of glucose values, which can support heathy lifestyle choices, it is also important to consider the burden that BGM places on PWD. In large meta-analyses of BGM in people with type 2 diabetes who were not using insulin, a benefit of BGM on glycemic management was found in the short term (≤6 months), but when assessed after 1 year of routine BGM, that benefit was no longer significant (40–42). A recent large, pragmatic trial of PWD who were not using insulin and had A1Cs that reflected moderate to ideal glycemic management demonstrated that, in a real-world setting, once-daily BGM did not improve glycemic outcomes or quality of life compared to no BGM throughout an entire year (43).
Increasingly, national organizations are encouraging providers and PWD to rethink the need for routine BGM in people with type 2 diabetes who are meeting or near their A1C targets when using medications that do not cause hypoglycemia. It is still reasonable to encourage people with type 2 diabetes who are experiencing hypoglycemia or severe hyperglycemia, using therapies within the sulfonylurea and meglitinide classes, acutely ill, using steroids, or far from meeting their glycemic targets to perform BGM, as discussed in the next section, until optimal glycemic management has been attained. Regardless of the views of specific providers and PWD on this issue, BGM is only truly valuable to PWD when they are provided feedback on the meaning of the values they obtain and guidance on how to make health behavior changes when needed.
Those Who Use Insulin
For people with type 2 diabetes who require basal insulin, BGM is recommended during basal dose titration and at any time when hypoglycemia is a concern. During dose titration, blood glucose values should be monitored in the morning while fasting and again at bedtime.
Because newer basal insulin formulations lead to less glucose variability, the frequency of BGM may be reduced when a stable dose of basal insulin has been determined. However, people using basal insulin should always have a meter and glucose strips available given the potential for hypoglycemia. If a person’s fasting glucose is within target range but the A1C value is still above goal, BGM before and after meals should be considered to check for postprandial hyperglycemia.
For most people with type 2 diabetes using a basal-bolus regimen, BGM should occur at least 4 times/day, similar to people with type 1 diabetes.
People with Prediabetes
In general, routine BGM is not recommended for people who have prediabetes. Although the postulated benefits of performing paired checking to assess the impact of certain foods on glycemic excursions are intriguing, well-designed clinical trials are lacking.
Women with Gestational Diabetes
BGM has proven efficacy in women with gestational diabetes mellitus (GDM). Most approaches include asking pregnant women at the time of GDM diagnosis to perform BGM several times daily to establish patterns of hyperglycemia. Such women are often asked to perform BGM initially at least 4 times/day, including in the morning while fasting and 1–2 hours after meals. From these data, providers can identify women who require pharmacologic therapy.
BGM values paired with food diaries can also guide dietary change recommendations, which are foundational to the treatment of GDM. Once glycemia is optimally managed, it is reasonable to decrease the frequency of BGM. This recommendation is based on trial data showing that women who performed BGM 4 times/day had babies with similar birth weights and a similar incidence of macrosomia as women who carried out BGM once every other day.
In summary, although CGM is gaining in popularity among all people with diabetes, BGM will remain an important component of diabetes self-care for many people. The engagement of both PWD and their diabetes care team in interpreting and acting on blood glucose data is crucial in all cases for BGM to be of optimal value.
USING BGM DATA TO ACHIEVE TREATMENT GOALS
For BGM to make a meaningful difference in diabetes self-management, both PWD and their diabetes care team must have the skills, understanding, and ability to change health behaviors and adjust pharmacologic therapies in response to glucose data (29,44,45). Focusing on BGM data is particularly important when glucose goals are not being met; for people who are using insulin therapy or starting or titrating glucose-lowering medications; for tracking adverse events; when changes are being made to the care regimen (including eating pattern and physical activity); when a new condition, change in condition, or instability develops; or during times of illness or stress (46,47). Table 4 identifies specific opportunities for learning from BGM data collected at different times of day (29,47,48). Additionally, PWD can be taught that BGM is a great tool for making insulin dose decisions, determining their potential risk for or the presence of hypoglycemia, getting feedback with regard to the[tbl4]glycemic consequences of their eating and physical activity choices, or whenever they might feel “off.”
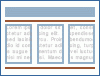
TABLE 4
Information PWD and Their Diabetes Care Providers Can Learn from BGM Performed at Different Times of Day (29)
As previously noted, a structured approach to BGM has been shown to improve outcomes (39,49). This approach, also called “structured testing” or “structured checking,” can be individualized by implementing different checking “profiles.” Examples of these profiles are described in Table 5 (50). The design of the profile can be determined based on the desire to collect specific mealtime data (e.g., checking before and after the largest meal of the day) or in an exploratory nature to understand overall glycemic patterns. The structured data then create an opportunity for informed decision-making.
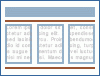
TABLE 5
Examples of BGM Structured Checking Profiles
Pattern Management
Understanding pattern management is the key to effective BGM (47). Searching for patterns in blood glucose data is like being a detective trying to finding clues that reveal trends and thereby identify opportunities to improve glycemic management. There are three factors to consider when engaging in pattern management: 1) recognition that BGM will identify the effects of food choices, physical activity, stress, and medications on daily glucose values; 2) PWD and their diabetes care providers both need education on how to interpret individual glucose values and patterns to[tbl5][tbl6]inform adjustments to therapies or lifestyle behaviors; and 3) tools such as paper logbooks or digital apps are needed to collect, analyze, and interpret data (47). Many glucose meters come with their own apps that synchronize directly with the device, allowing users to log events (e.g., carbohydrate intake, physical activity, and insulin doses) along with BGM data.
Living with diabetes 24/7, year in and year out, is challenging. Understanding the value of pattern management can help PWD take charge of their self-management and play an active role in decision-making regarding their diabetes treatment. Understanding their own BGM data can motivate them to make healthier choices and build their self-confidence. Working with a certified diabetes care and education specialist to learn about BGM and pattern management is important at the time of diagnosis and annually thereafter, as well as whenever there is a change in medication or their condition or when glycemic goals are not being met (51,52).
Early clues from random BGM values orconversations with PWD can help to identify a glucose profile that will best reveal trends in various situations. Searching for patterns requires BGM values collected at the same time of day for a minimum of 3–4 days. One framework for interpreting BGM data (47) sets out a simple three-step process: 1) obtain at least 3–4 days of data, 2) identify patterns, and 3) collaborate with the PWD to individualize diabetes care and education based on the data collected (Table 6).
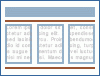
TABLE 6
Three-Step Process for Evaluating BGM Data and Sample Questions to Help Identify Opportunities for Improvement
When reviewing BGM data, certain patterns should be given higher priority than others. First, focus on episodes of hypoglycemia. After hypoglycemia has resolved, review glucose values that are above the target range. When post-meal glucose values are rising above target range, consider whether changes to both medication and health behaviors may be needed. When focusing on health behaviors, review food portions, carbohydrate counting, and the quality of food consumed.
The following case studies describe different structured checking profiles and how BGM data can be reviewed to make health and treatment decisions.
CASE 1: M.V.
M.V. is a 50-year-old woman with type 2 diabetes for 11 years, who is taking metformin 1,000 mg twice daily and glipizide 10 mg before breakfast and dinner. Her BMI is 25 kg/m2, her most recent A1C was 8.5%, and she notes feeling tired most of the time. She has not been checking her glucose.
After discussion, she agrees to perform a 7-point profile for 3 days before her next clinic visit so you can get a better idea of her glucose levels throughout the day. The data she shares at her next appointment are displayed in Table 7.
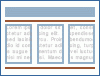
TABLE 7
Case 1: 3-Day, 7-Point Glucose Profile (mg/dL)
What questions do you ask?
Start by asking M.V. what she notices and what information she can share with you. Solicit her opinion regarding what is working and what she believes may need to change. Ask her about her food choices and portion sizes and about her physical activity.
M.V. states that she is taking her medication as directed. She says she is unable to walk after breakfast or lunch because of work and that she does not feel the need to change her eating habits.
What patterns do you see?
M.V.’s pre-breakfast values are all within her target range. However, her post-meal values are above range, as are those at bedtime. The only times during the day that she is in the target range are before breakfast and before dinner.
You learn that M.V. takes a walk every day 3 hours after lunch, which lowers her pre-dinner glucose level into the target range.
What do you suggest?
These BGM data suggest that M.V. needs more medication to manage her post-meal glucose excursions. Using a shared decision-making approach, you can discuss the pros and cons of appropriate medication options with her and arrive at a plan.
CASE 2: K.B.
K.B. is a 67-year-old woman who has lived with type 2 diabetes for 14 years. She is taking metformin 1,000 mg/day and 24 units of insulin glargine, which she reports taking every night at her 9:00 p.m. bedtime. Her BMI is 32 kg/m2.
She has been checking her fasting glucose levels several times per week, and all of her values have been <130 mg/dL. However, her A1C continues to be above goal and is currently 8.3%. She has never checked her glucose at other times of the day.
You ask her about her meals and activity and learn that her biggest meal of the day is at dinner. Together, you decide that K.B. will take a “paired checking” structured approach to BGM, checking her glucose before and 2 hours after dinner nightly for 1 week and then return for a follow-up appointment. Her BGM data are displayed in Table 8.
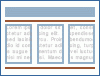
TABLE 8
Case 2: 1 Week of Paired Checking Glucose Values Before and 2 Hours After Dinner (mg/dL)
What questions do you ask?
What does K.B. notice about her glucose values? Ask her about her food choices and portion sizes at dinner.
K.B. says she has been using an online weight loss program and has been focusing on using the plate method at mealtimes. Although she does eat pasta and other carbohydrate-containing foods, she says her portion sizes are small.
What patterns do you see?
All of K.B.’s post-dinner glucose values are above her target of <180 mg/dL.
What do you suggest?
K.B. needs more medication to manage her post-meal glucose excursions. Together, you consider adding a glucagon-like peptide-1 receptor agonist to her regimen to assist with both weight loss and glycemic management. You might also suggest that K.B. use BGM to check before and after other meals to see if her glucose levels are consistently out of range at any other times.
CASE 3: P.R.
P.R. is an 80-year-old man who has been living with type 2 diabetes for 26 years. His A1C is typically in range, at <7.0%. He has been taking 36 units of insulin degludec at bedtime and 8 units of rapidacting insulin before each meal. He states that he has been “feeling low” during the day.
Sometimes he checks his glucose, but other times, he just drinks juice or eats a snack without checking. He performs BGM infrequently, but when he does, it is typically before breakfast and before bed.
He agrees to your request that he check his glucose before and after 1 meal/day for 1 week, alternating meals, as well as whenever he feels as if his glucose is low. The BGM results he brings to his follow-up visit are displayed in Table 9.
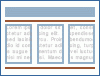
TABLE 9
Case 3: 1 Week of Staggered Profile BGM Data (mg/dL)
What questions do you ask?
What does P.R. notice about his glucose values? Was this week typical for him, including the food he ate and his physical activity?
P.R. states that he typically eats a late breakfast and often has a small lunch. He likes to garden, and he typically does that in the afternoon, often after lunch. His lunch is frequently just a handful of nuts, some berries and a diet coke. He has been on a fixed dose of prandial insulin for the past several years, but he says his appetite has changed lately.
You also ask what type of symptoms he has when his glucose values drop to <70 mg/dL and how he treats his low glucose. P.R. says that he usually feels sweaty and hungry, and then he feels shaky and lightheaded. He prefers to drink juice and keeps juice boxes in the refrigerator.
What patterns do you see?
The first pattern you address is the two episodes of hypoglycemia after lunch on Wednesday and Sunday.
What do you suggest?
P.R. should reduce his pre-meal insulin dose at lunch. Together you discuss whether he would be willing to adjust his pre-meal insulin doses based on the size of the meal he is going to eat and his anticipated physical activity. You also ask if he would be willing to check his glucose before and after lunch to help identify the best insulin dose for him at that meal.
You refer P.R. to a diabetes care and education specialist to review carbohydrate counting and portion sizes and to review BGM to develop a plan for insulin management. Using CGM could also be considered.
Solution-Focused Questioning
Assessment of blood glucose management requires frequent discussions with PWD. A questioning approach is commonly used. Often, questions can make PWD feel blamed or shamed for out-of-range glucose values, elevated A1C levels, or decisions they have made, and this perceived negativity can seem to devalue the hard work of living with a chronic condition. One alternative is to consider a more solution-focused approach (53).
In this type of approach, questions focus on what is going well for the person and are chosen to identify existing strengths. For example, instead of asking about breakfast glucose values by saying, “Why are your after-breakfast numbers so high?” consider asking, “Can you share with me an example of your typical breakfast?” From there, you can have a conversation based on the data. If the person is eating a high-carbohydrate breakfast, you might ask, “What changes would you be willing to consider to bring your after-breakfast glucose levels into the target range?”
Another strategy would be to focus on a time of the day when glucose values are in range and ask questions about those times, again to identify strengths. For example, “I see your before-dinner numbers are within your target range most of the time. How did you achieve that?” With this type of question, you are strengthening your relationship with the person by building trust and acceptance. This method also helps PWD identify their own strengths and what is working well for them. In theory, this approach will help PWD do more of the things that are working well for them and less of those that are not working well (54).
In summary, a structured approach to BGM can help PWD and their diabetes care team accurately interpret glucose data, recognize patterns, and work together to make informed treatment decisions to improve diabetes management.
OVERCOMING BARRIERS TO BGM
It is well established that BGM can serve as a basis for self-management that is effective in achieving and maintaining positive glycemic outcomes (55,56). However, it is also well established that PWD do not always perform BGM as recommended and that those who do may not take appropriate self-management actions based on their blood glucose data. In the previous section, we focused on ways to assist PWD in making optimal use of BGM to achieve their treatment goals. Here, we look at how to overcome the numerous barriers that may hinder a person’s success in that endeavor.
BGM Is Behavior
To help PWD overcome barriers to BGM, it is essential to understand that BGM is behavior. As such, it is influenced by individuals’ store of actionable BGM information, motivation to act on this information, and behavioral skills for acting on it effectively. Data collected by carrying out BGM as recommended can serve as the basis for self-management actions that result in improved diabetes health outcomes, contributing to a virtuous feedback loop (Figure 2).

FIGURE 2
An Information—Motivation—Behavioral Skills model of BGM.
The impact of BGM information and motivation will often be limited by a person’s BGM behavioral skills. Moderating factors such health literacy and ability to acquire and pay for supplies to check glucose may influence the amount of BGM data collected, a person’s motivation and ability to carry out BGM with the recommended frequency, and, ultimately, health outcomes.
Information and BGM
PWD may have information deficits that interfere with BGM (57). Some may believe that their body can “feel” when their blood glucose is within their target range; some may not be aware of glucose checking patterns that can provide meaningful information; and others may believe that their A1C results tell them everything they need to know to manage their diabetes.
Motivation and BGM
Personal and social motivation can also influence BGM (57). Personal motivation for BGM rests on a person’s perception of the overall costs and benefits of BGM. PWD who believe that BGM has important payoffs will be inclined to carry out monitoring as recommended, whereas those who believe that BGM has costs that outweigh the benefits are not as likely to consistently carry out BGM. Social motivation for
BGM rests on a person’s perception that significant others—spouses, children, diabetes care and education specialists—support their BGM efforts and endorse their interest in engaging in shared decision-making. Thus, a supportive partner, or an indifferent physician, can influence BGM performance.
Personal and social motivation to carry out BGM are often in competition with the impetus to engage in other activities that are incompatible with strictly following BGM recommendations. Many, if not most, PWD will regard BGM as having important benefits and social support, but sometimes less important benefits and less social support than other aspects of their lives (e.g., maintaining concentration at work or enjoying a meal with friends).
Behavioral Skills and BGM
Performing BGM as recommended places substantial behavioral skills demands on PWD (57). In the context of busy day-to-day life, simply remembering to perform BGM at meaningful intervals may be a challenge. Financing supplies to check glucose within complex, changing, or limited insurance coverage poses additional challenges. Maintaining access to such supplies when needed and discreetly, painlessly, and effectively lancing one’s finger or positioning a sensor, can also be challenging. Still other behavioral skills demands involve knowing how to talk to a busy HCP about problems with BGM or interpreting and using its results.
Moderating Factors and BGM
Personal characteristics of PWD and their environment can have direct and indirect impacts on BGM (57). These factors include inadequate insurance coverage, limited health literacy, burden of disease associated with diabetes (e.g., impaired eyesight, impaired dexterity, and overall medication burden), and comorbid conditions. Overall, optimal BGM will be achieved by well-informed, engaged, behaviorally skilled PWD and will be maintained when they experience positive health outcomes.
Assisting Patients in Overcoming Obstacles to BGM
Optimal BGM needs to be taught, rehearsed, refined, and reinforced by well-informed, well-motivated, and behaviorally skilled clinician educators. A diversity of interventions—including BGM device education, smartphone coaching keyed to blood glucose results, and counseling approaches linked to readiness to change—may all be effective in increasing a person’s frequency of BGM and changing patterns of checking glucose (55,58,59). In the realistic context of the busy and resource-limited clinical practice setting, means for assisting PWD in overcoming barriers to BGM must be both brief and effective and must focus on those with the greatest need.
Triage the Need for BGM Coaching
BGM counseling should target individuals who are experiencing difficulties in achieving their glycemic goals. Such difficulties can involve inadequate or uninformative testing, inability to translate results into self-management actions, or the need to change the therapeutic regimen.
Follow Principles of Effective BGM Counseling
Principles of efficient and effective BGM counseling are inspired by the procedures of and evidence base supporting Motivational Interviewing (59,60). For people identified as potentially benefiting from improved BGM practices, the following counseling approaches may prove helpful.
Acknowledge the Challenge
Many PWD are defensive and fearful of provider censure for variable performance of BGM. Legitimizing an individual’s struggle with the demands of BGM can be the first step in improving its practice. Consider asking, “Almost all of my patients find BGM to be a challenge. How is it going for you?”
View PWD as the Experts
Although clinicians are the experts in diabetes medical management, PWD are the experts in what would have to happen to improve their BGM. Change strategies that originate with PWD—as opposed to those proposed by clinicians—are often much better suited to individuals’ situations and therefore more likely to be adopted (59,60). Motivational Interviewing–inspired questions such as the following can be helpful in eliciting change strategies from PWD.
- “Can you help me understand what BGM is like for you?”
- “On a scale of 1 to 10, how important is it for you to stick to the BGM pattern we’ve discussed?” Most people will respond with a high number. Those who give a low importance rating are essentially telling the provider that they lack the information, ability, or motivation to practice BGM.
- “Why do you say 10 (or 9 or 8 or 7) and not lower?” This paradoxical question and its answer provide an opportunity for the individual to rehearse reasons for the importance of BGM.
- For those who rate importance as a 6 or lower, the most important question to ask is, “What would it take—what would have to happen—for you to rate the importance of following the BGM pattern we’ve discussed as, say, a 7 or an 8 or a 9?” Here the individual—the expert in what it would take to make BGM personally important—may tell you exactly what it would take, and this can become the core of the counseling intervention. The person—not the provider—has offered a strategy to suit the situation. This suggestion is followed by a discussion regarding how to make it happen.
- “On a scale of 1 to 10, where 1 is ‘not at all confident’ and 10 is ‘completely confident,’ how confident are you—how sure are you—that you can monitor your blood glucose in the way that we’ve discussed?” A lower confidence rating generally means that a person lacks the behavioral skills to implement the recommended pattern of testing or lacks motivation or resources to do so.
- For those who rate their self-confidence high (from 7 to 10), consider asking another paradoxical question: “Why did you say 10 (or 9 or 8 or 7) and not lower?” This question gives people an opportunity to rehearse their strengths by explaining why they feel confident about performing BGM.
- For those rating their confidence as a 6 or lower, the most important question to ask is, “What would it take—what would have to happen—for you to be, say, a 7 or an 8, or a 9 in confidence that you could follow the BGM pattern we’ve talked about?” Here the individual—the expert in what it would take to increase his or her self-confidence in BGM performance—can tell the clinician exactly what it would take to do so. This can become the core of the counseling intervention. Again, the person—not the provider—has offered a strategy to suit the situation. This suggestion is followed by a discussion regarding how to make it happen.
Set Incremental, Achievable Goals
Based on an exchange like the one described above, diabetes care providers and PWD can negotiate incremental, achievable BGM goals. Change is rarely an all-or-nothing event, and identifying and achieving an incremental goal (i.e., structured pre- and post-meal testing for 1 meal/day to assist with food choices or improved insulin dosing) can be the first step toward achievement of broader goals negotiated in future counseling exchanges.
Embracing the Spirit of Collaborative Problem-Solving
This approach to helping people with diabetes overcome obstacles to BGM is meant to suggest an overall collaborative spirit of BGM counseling that will enable diabetes care providers to triage PWD and identify those who can benefit from counseling, empathize and legitimize their struggles, elicit strategies for improvement, and negotiate achievable goals.
Attention to BGM performance and its use to inform and facilitate meaningful self-management action is part of an ongoing support process. In subsequent encounters, providers should check in concerning the attainment of negotiated goals and potentially suggest a more challenging BGM goal moving forward. For PWD who have achieved their goal, implement a relapse prevention discussion; ask how they achieved their success, and identify specific practices they can implement as needed in the future. For those who have not met the negotiated goal, process their struggle to attain the goal and elicit an alternative strategy for achieving it or modify the goal to make it more achievable. Repeat this process until BGM performance is stable, all the while continuing to empathize with the struggles, elicit solutions to emerging challenges, and reinforce successes.
The approach to diabetes self-management and BGM counseling described here is summarized in Figure 3. Clinicians interested in a more in-depth discussion of this approach are encouraged to consult references 57–60. Keep in mind that the specific steps and wording provided here are only illustrations. Many clinicians have had effective BGM support discussions by simply asking, “How’s it going for you?” and “What would have to happen to make it work better?” Most importantly, remember that PWD are your allies and experts in facilitiating their own behavior change.

FIGURE 3
A Motivational Interviewing–inspired approach to counseling PWD to address barriers to BGM.
USE OF BGM IN CGM USERS
Convenience and accuracy are characteristics of CGM that lead many to think CGM will, to a great extent, displace BGM. CGM systems are small and getting even smaller with each new generation, as well as becoming increasingly accurate. Although CGM is not as accurate as the most accurate BGM systems, it surpasses the accuracy of many glucose meters available today (16,61).
Importantly, the recent, rapid uptake of CGM is also the result, in part, of scientific data showing improved clinical outcomes of reduced hypoglycemia and improved A1C levels using CGM compared to BGM or usual care (62–67). Furthermore, an impactful recent meta-analysis of randomized controlled trials demonstrated that CGM improves glucose outcomes by increasing glycemic time in range (TIR) and decreasing time below range, time above range, and glucose variability in both type 1 and type 2 diabetes compared to usual care that included BGM (68).
In its 2020 Standards of Medical Care in Diabetes—2020 (29), the American Diabetes Association (ADA) states that, “when used properly, real-time and intermittently scanned continuous glucose monitors in conjunction with insulin therapy are useful tools to lower A1C levels and/or reduce hypoglycemia in adults with type 1 diabetes who are not meeting glycemic targets, have hypoglycemia unawareness, and/or have episodes of hypoglycemia . . . and in conjunction with insulin therapy . . . to lower A1C levels and/or reduce hypoglycemia in adults with type 2 diabetes who are not meeting glycemic targets.” There is also considerable support for a broad range of intermittent use of CGM over time (using either personal devices owned by PWD or professional CGM systems owned by medical clinics) for evaluating CGM metrics and glucose patterns for PWD who use BGM but have not achieved their glucose targets.
Despite the availability of high-quality CGM systems that can facilitate improvements in glucose management, uptake of this technology in clinical practice, while growing steadily, is still only about 50–75% in pediatric endocrine practices and 35–50% in adult endocrine practices for individuals with type 1 diabetes (69) and much lower for those with type 2 diabetes treated in primary care. The main barriers to uptake are cost, reluctance of patients to wear a CGM glucose sensor, and high time demands for implementation into usual clinical workflows coupled with inadequate reimbursement for the extra time spent. Another likely barrier to use of CGM in the primary care setting is a lack of clinician awareness regarding the availability of these systems and the potential benefits they may provide. In addition, some PWD find the CGM alarms for fluctuating glucose and the increased volume of data collected via CGM to be stressful, and others may experience difficulties with skin irritation at sensor sites.
Some have asked whether the availability of newer CGM systems that do not require calibration and are cleared for nonadjunctive insulin dosing (i.e., not requiring confirmation of glucose levels via BGM) might signal “the end of fingersticks” (70). However, despite the trend of increasing indications for CGM use and guidance to use BGM only when indicated (43) to minimize unnecessary health care costs, in practice, most PWD are taught how to use a glucose meter as part of routine self-management education, even if it is to be used only intermittently (as is often the case in type 2 diabetes). Still, the ability to perform BGM remains important even for PWD who use CGM because data are not available during CGM system warmup periods, and there will be instances in which CGM systems malfunction or require calibration. PWD also need to be able to verify their glucose status when they are symptomatic and are concerned that their CGM reading is not accurate.
Interpreting BGM and CGM Data for Clinical Decision-Making
There are some principles in the use of BGM and CGM that may enhance the effective use of each type of glucose monitoring. Forty years ago, PWD insisted, often to their skeptical medical teams, that they wanted to perform their own glucose monitoring at home, even if that meant using the crude lancet devices and slow and only moderately accurate glucose meters of that era. More recently, some clinicians have worried that CGM generates a potentially overwhelming level of data that may be confusing to PWD. Once again, however, many PWD are making it clear that they find such information helpful and that they find the emerging terminology around the concept of TIR easy to understand (71).
Efforts to standardize (72,73) and organize (74) CGM data to facilitate interpretation have led to the establishment of 10 core CGM metrics for clinical care; CGM targets for times below, in, and above glycemic ranges; and a recommended single-page CGM report known as the ambulatory glucose profile (AGP) as a starting point for CGM-informed clinical care. The ADA has included these recommendations in its 2020 Standards of Care (29).
AGP Report for CGM
A 2-week AGP report is usually generated from CGM data at face-to-face or remote telehealth visits with PWD who use CGM systems. As shown in Figure 4A, the AGP is a three-panel, one-page report showing the 10 core CGM metrics and CGM targets in the first panel. A clinician or patient can review the Time in Ranges color bar to see if the recommended TIR target of glucose in the range of 70–180 mg/dL for >70% of readings is being met while also keeping the time-below-range metrics of <70 mg/dL and <54 mg/dL to the recommended <4% and <1% of readings, respectively. This graphic display shows whether there is room for improvement. The second panel of the AGP shows a 2-week glucose profile previously known as a “modal day” or “standard day” data depiction. This panel identifies at a glance where in the 24-hour period there is room for improvement. Recommendations are to mitigate any periods of hypoglycemia first and then tackle any hyperglycemia or excessive glycemic variability. The third panel depicts each of the daily glucose profiles from the 10–14 days of CGM use that make up panel 2. Reviewing these daily views helps to sort out whether there are differences in glycemic outcomes between weekends and weekdays or if any particular day of the week stands out as needing attention. Clinicians and PWD alike have generally embraced this one-page report that allows for a dialog about the collected data and facilitates shared decision-making on next steps toward improving glycemic management.

FIGURE 4
AGP reports display glucose monitoring data for easy interpretation to facilitate shared clinical decision making. Depicted are A) an AGP report derived from CGM data and B) an AGP report derived from BGM data.
AGP Report for BGM
Although BGM had a 20-year head start on CGM, there has not been a similar concerted effort until now to generate a consensus BGM report similar to the one that has been so helpful in encouraging the uptake of CGM, despite the fact that the original AGP report conceived by Mazze et al. (75) in 1987 was developed for BGM data. Figure 4B shows a sample AGP report generated by an expert panel at the International Diabetes Center, using BGM rather than CGM data. Where possible, it follows the CGM version of the AGP (Figure 4A), including three graphic panels in a one-page report. Although 14 days is the default standard for the CGM AGP that includes several thousand glucose values, the AGP for BGM compiles 90 days of data with an average 2.2 checks/day and a few hundred total glucose checks summarized. The metrics are partly BGM-oriented, with the ability to calculate and display the average pre- and post-meal glucose values if the individual manually marks these time points. The BGM targets relate to the desired fasting, pre-meal, and post-meal glucose readings. In addition, there is the familiar color bar of glucose ranges, but for the BGM report, this is not called “Time in Ranges.” Rather, they are called “BGM Values in Ranges” because since these are intermittent BGM samples and do not cover the entire day. Instead, the percentage of values that were in the standard glucose ranges are displayed, along with the number of glucose checks that actually comprise each range. The glucose profile in the middle panel shows all of the glucose values at the times they were taken, color-coded to indicate in-range, below-range, or above-range values. In addition, the median line and interquartile range are included only when there are enough data to generate these summary metrics. This approach will often lead to gaps in the AGP graphic, but this is a teaching point to indicate that, with BGM, there are times (usually overnight) during which there is uncertainty regarding glycemic status. Finally, like the CGM report, the AGP BGM report ends with 2 weeks of individual day graphs so the clinician can see whether there are patterns of days with fewer tests performed that cannot be discerned from the combined data in panel 2.
Intermittent Use of BGM and CGM
Having a standardized and systematic approach to displaying BGM and CGM data makes for a smoother transition in glucose analysis when moving from BGM to CGM use or when using BGM and CGM intermittently. Are there data supporting the intermittent use of both methods of glucose monitoring in the management of type 1 or type 2 diabetes? This topic was nicely reviewed from the perspectives of PWD, HCPs, and health systems by Ziegler et al. (76), who concluded that the question of intermittent monitoring requires much more discussion.
For most individuals with type 1 diabetes, use of CGM is recommended on a continuous basis as much as is financially and socially acceptable (77). Many individuals with type 2 diabetes who are not on a multiple daily injection (MDI) insulin therapy regimen but who have not achieved their glycemic targets might also consider intermittent use of CGM and BGM. Professional CGM systems may facilitate this strategy for such patients. In this type of intervention, clinic-owned CGM systems are provided to PWD for 10- to 14-day wear periods, either blinded or providing real-time glycemic data. The data are then also evaluated retrospectively during a clinic or telehealth visit to assess the current state of management and look for glucose patterns. In addition, PWD can use a real-time CGM system intermittently, such as for 10–14 days once every 2–3 months, to get the value of real-time reinforcement of good food and exercise choices.
Logical Cases and Positive Anecdotal Experience
The most logical cases in which to use BGM and CGM intermittently are those in which PWD seem to value the data from CGM but do not want or cannot afford to wear a sensor continuously. In such cases, PWD can get the CGM-derived AGP and daily views during intermittent CGM use to help identify the times of day when there is room for the most improvement (i.e., very low or very high glucose levels) and then target those time periods with intermittent BGM. For example, if the person whose data is represented in Figure 4A could only afford or only wanted intermittent CGM use, that person could focus BGM between 3:00 and 7:00 a.m. and again between 7:00 and 8:00 p.m. to minimize potentially dangerous hypoglycemia and hyperglycemia, respectively, until another intermittent CGM AGP could be generated. Having the PWD direct BGM according to the previous CGM AGP can provide motivation for making lifestyle changes. It can also help to overcome clinical inertia, leading to shared decision-making regarding any necessary therapeutic changes.
One clinical scenario in which intermittent use of CGM seems particularly beneficial anecdotally is to provide a detailed glucose profile when considering adding a glucagon-like peptide 1 receptor agonist or basal insulin to a regimen of metformin and a sulfonylurea (usually reducing or stopping the sulfonylurea in the process). A second scenario in which intermittent CGM may be beneficial is to facilitate intensification from a basal insulin regimen. Often in such cases, there is a classic stair-step pattern of rising glucose after each meal until a person is very hyperglycemic at night, requiring large doses of basal insulin to try to normalize glucose levels by morning. In such instances the picture afforded by the CGM-derived AGP is so clear that PWD may agree to add mealtime insulin and adjust their basal insulin doses downward to smooth out the glucose profile. We refer to these scenarios as logical cases and anecdotal experiences because we currently lack randomized trials or even cohort-matched studies in real-world settings to confirm for larger numbers of PWD that intermittent CGM and BGM is an effective strategy (78).
More data are also needed on the appropriate use of BGM, CGM, and intermittent use of either or both in the real-world primary care setting. In 2018, an Italian team studied BGM in >13,000 individuals with type 2 diabetes from 21 clinical centers and concluded that, regardless of PWD’s therapeutic regimen, BGM was poorly utilized (defined as mostly fasting BGM, no structured BGM checking that might reveal glucose patterns, and overall very little BGM checking that would be helpful in guiding glycemic management) (79). One recent review of both BGM and CGM concluded that we will probably continue to see a mix of BGM and CGM use and, in many cases, a combined use of both monitoring methods (80). Both CGM and structured BGM can generate data to assist with pattern recognition and inform lifestyle changes and medication adjustments.
INNOVATIONS IN BGM
The use of BGM has been found to assist many PWD in achieving their glycemic targets (50,81,82). Traditional glucose meters display and store glucose measurements within the device. These data need to be retrieved before they can be analyzed by users or clinicians to guide treatment. Innovative technologies such as digital health apps that display and summarize individual blood glucose measurements and incorporate additional relevant data such as insulin doses, meals/snacks, and physical activity, can further support self-management while decreasing disease burden and benefitting overall diabetes care (83). Integration of BGM with insulin calculators, automated insulin titration software, and remote coaching are further developments that will be reviewed in this section.
Diabetes Apps and Data Management
Regulations and Recommendations
More than 1,500 mobile apps support diabetes management, many of which incorporate BGM. Digital health apps used for diabetes management include options that focus on nutrition, physical activity, glucose monitoring, insulin titration, and insulin delivery. These apps are generally intended to improve health outcomes by coaching PWD, supporting healthy eating and weight management, encouraging BGM, and helping with interpretation of glucose data and identification of glycemic patterns (22).
Although the field of digital health apps has been developing rapidly, regulations and guidelines have lagged behind. Mobile apps are still largely unregulated unless they meet the definition of a medical device for therapeutic or diagnostic purposes. Recently, however, regulatory agencies in the United States and abroad have recognized the rapid growth of this market and have released guidelines and policies (22). Many European countries assess whether a product meets the standards set by the E.U. Medical Device Directive (84). In the United States, the FDA in 2019 released an updated version of its Policy for Device Software Functions and Mobile Medical Applications (85).
The FDA requires evidence of safety, performance, and clinical effectiveness, using a risk-based approach (22,85,86). Mobile apps that provide insulin dose calculations are considered medical devices; those that track, record, or make behavioral suggestions about fitness, health, or wellness (e.g., dietary logs, calorie counters, and activity monitors) are not considered medical devices (85). FDA-cleared glucose meter–connected apps are described in Table 10.
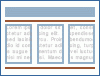
TABLE 10
FDA-Cleared Glucose Meter–Connected Apps
Although digital health apps have great potential to benefit PWD, they can generate challenges for clinicians. HCPs need to understand not only the potential benefits, but also the potential risks with regard to data confidentiality, security, accuracy, and reliability (22). Readers are referred to a recently published consensus report from the Diabetes Technology Working Group of the ADA and the European Association for the Study of Diabetes for a detailed review of this topic and recommendations for the use of this technology in clinical practice (22).
Cloud-Based Solutions
Busy practitioners are often presented with multiple BGM communications from PWD, who use many different meters and may send in handwritten data logs or use meter-specific proprietary downloading software and nonstandardized data reports. In addition, the lack of integration of these reports with HCPs’ electronic medical record (EMR) systems makes the documentation of glucose levels cumbersome. These problems have led to the development of Cloud-based, device-agnostic diabetes data management systems, the largest of which are Glooko (known in Europe as Diasend-Glooko) and Tidepool. Both provide users with standardized reports that can assist in BGM pattern recognition and facilitate shared decision-making.
The Glooko platform (https://glooko.com, Glooko) is available to practices by subscription (87). It allows rapid in-clinic or remote uploading of data from >70 different glucose meters and numerous insulin pumps and CGM systems (Figure 5) and potential integration into EMR systems. The Tidepool platform (https://tidepool.org, Tidepool Project), run by a nonprofit organization, provides free software for PWD and clinicians to upload, share, and review data from >25 meters, as well as most insulin pumps and CGM devices (Figure 6). Tidepool has a “copy as text” feature, enabling copying and pasting of BGM data into clinic notes (88).
Although diabetes apps have tremendous potential (89), barriers exist for many individuals. These include low literacy and numeracy levels, inability or challenges with regard to using smartphone or other smart devices (e.g., older adult or those with impaired vision or hearing); costs, low digital (i.e., computer or Internet) literacy, limited Internet access, and insufficient broadband Internet service or “dead zones” in urban and rural settings (22,83,90).
Insulin Dose Calculators
Successful diabetes self-management enables PWD to achieve their glycemic goals and is especially important for those on insulin therapy (45). Intensive insulin therapy requires the completion of multiple complex daily tasks. These include monitoring glucose levels before meals and at bedtime; counting carbohydrates and calculating insulin doses for each meal or using fixed insulin doses with carbohydrate-consistent meals; adjusting insulin doses to correct hypoglycemia or hyperglycemia; and calculating correction doses based on an individualized ISF or a predetermined insulin algorithm. All these actions must be performed every day to achieve and maintain euglycemia and avoid short- and long-term complications. These tasks can be especially challenging for PWD with low literacy or numeracy levels (91).
Insulin dose calculators are incorporated into insulin pumps, but many PWD who use an MDI insulin regimen instead of a pump can also benefit from such a tool to improve their dosing accuracy. Dose calculators are preprogrammed with a user’s ISF, individualized I:C ratio, and target glucose range. When users enter a blood glucose reading and the amount of carbohydrate they intend to eat, the calculator will provide a recommended insulin dose.
Numerous insulin calculator apps are available, but the accuracy and reliability of some are poor. An evaluation of 46 such apps found that two-thirds posed a risk for inappropriate dosing (92,93). Therefore, clinicians must proceed with great caution when recommending an insulin dose calculator to limit exposure of PWD to incorrect calculations that could result in erroneous insulin dosing recommendations with potentially catastrophic results.
Eiland et al. (94) identified 20 diabetes self-management apps that were cleared by the FDA or bore a CE (European Conformity) mark and had evidence of efficacy, safety, or feasibility reported in peer-reviewed literature. Of these 20 apps, fewer than half contained insulin dose calculators. Since 2019, insulin dose calculator apps have been considered medical devices and are regulated by the FDA (86,94). Those available in the United States are described in Table 11.
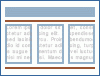
TABLE 11
Insulin Dose Calculators Available in the United States
Connected Care
The term “connected diabetes care” (95) refers to diabetes management systems that use smartphone apps, connected devices, and remote human and automated coaching. The goal of such systems is to provide PWD with remote education, coaching, and communication to augment their usual care and improve self-management and outcomes.
Most such systems use connected BGM. Some programs use a business-to-business-to-consumer model, providing the program to an employer or insurer, which in turn offers it to PWD, and some offer peer support and an interactive curriculum. All connected diabetes care companies with remote human coaches employ teams of diabetes care and education specialists, nurses, dietitians, and exercise scientists. Presently, Virta Health (https://virtahealth.com) and Onduo Health (https://onduo.com) consider themselves to be virtual medical clinics and employ physicians who can prescribe and titrate medications (96,97). The most salient features of selected connected care companies and their key published data are summarized in Table 12 (98–113).
Artificial Intelligence
Artificial intelligence (AI) decision-support systems are becoming commercially available and have the potential to improve diabetes management by providing tools to automate insulin titration and dose adjustment recommendations for PWD on insulin therapy. One such system is the DreaMed Advisor Pro (https://dreamed-diabetes.com, DreaMed), which received FDA clearance in 2018.
With this system, data from CGM or BGM and insulin pumps are uploaded to a data management system such as Tidepool or Glooko. After uploading and analyzing the data, the Advisor searches for hyperglycemic or hypoglycemic patterns and produces an insulin titration recommendation for optimization of insulin pump settings. Future versions are expected to incorporate smart insulin pens for patients using an MDI insulin regimen. Clinicians can review the automated recommendations, edit them, and share them with PWD either during a visit or electronically. In the future, availability of additional AI systems is anticipated.
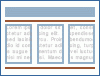
TABLE 12
Major Connected Diabetes Care Companies and Published Evidence of Their Impact
To summarize, diabetes self-management is often limited by the absence of real-time BGM data needed to make informed decisions to help PWD achieve and maintain their glycemic targets. The advent of mobile health apps allows PWD to use their glucose meters in a partner-based model, through which app-based BGM becomes a “health companion,” providing reminders for performing BGM and taking medications and offering immediate feedback and graphic depictions of current glycemic status. Device-agnostic software platforms can enable clinicians’ easy review and assessment of BGM data as displayed in standardized reports, facilitating treatment optimization. For PWD using MDI insulin therapy, insulin dose calculators, as stand-alone tools or integrated into insulin pens, can ease the burden of complex dose calculations.
However, challenges remain. Regulatory agencies must ensure that available apps are safe (i.e., accurate), and HCPs need improved access to FDA-cleared apps and the knowledge and confidence to use them in clinical practice. Apps should be affordable and available in multiple languages, and access to smartphones and Internet services must be expanded.
CONCLUSION
Throughout the past several decades, BGM has been fundamental for optimal diabetes self-management, and it will remain an important tool for PWD for years to come. BGM devices have become smaller and more accurate and now require less blood and include more features such as Cloud-based data management systems and connectivity to other devices. The accuracy of BGM is crucial because its results are used to direct therapy.
Accuracy can vary among devices. Errors can be caused by use of expired, improperly stored, or damaged strips; poor technique, including inadequate site cleansing; conditions such as hypoxemia and anemia; and the use of high-dose vitamin C and acetaminophen. Over the years, the standards required to gain FDA clearance have become more stringent (9), and more accurate glucose meters are now available in the marketplace. However, there is no formal re-evaluation of meters after clearance to ensure continued device accuracy.
BGM is only helpful if PWD know how to view, interpret, and act on their results. Education is essential in this regard. PWD need to learn proper technique, overcome barriers to use, and receive training in understanding and using their results to optimize glycemic management.
The recommended frequency of BGM should be individualized. People with type 1 diabetes will generally need to perform BGM 4–10 times/day, whereas those with type 2 diabetes who are at or near their glycemic targets and not taking medications that can cause hypoglycemia may use BGM less frequently or not at all (Table 3).
Pattern recognition, including identifying glycemic excursions related to eating, physical activity, stress, and illness, is important and should be used to help direct treatment. The use of structured BGM profiles such checking before and after meals, before and after exercise, and at bedtime and fasting, can assist in adjusting food, physical activity, treatment regimens, and medication dosing. BGM also is needed when hypoglycemia is suspected and, for PWD using insulin, before driving or performing other crucial tasks (29). Adopting a counseling strategy that focuses on the information, motivation, and behavioral skills PWD need to succeed and incorporates the use of negotiated, incremental, achievable goals that are elicited, if possible, from PWD rather than HCPs, will facilitate efficient and effective use of BGM.
Differences abound in the ability of various glucose meters to communicate with other glycemic management systems. Integration of meters with Cloud-based data management systems facilitates remote monitoring. The use of additional innovations such as insulin dosing calculators, smartphone apps, remote coaching, and artificial intelligence systems is on the rise, and their availability and accessibility are expected to expand.
Glucose monitoring can now be accomplished via CGM as well as BGM. The ADA recommends that PWD who use CGM have the ability to perform BGM to verify readings when symptomatic, if they are concerned that their CGM transmitter or sensor is not working properly, and to calibrate certain CGM devices per manufacturers’ instructions (29). In general, the use of CGM is costlier than BGM and is not necessary for people with type 2 diabetes who are not treated with insulin and are maintaining their glycemic goals. For those who have not achieved their glycemic goals, directed BGM based on glucose patterns observed through the intermittent use of CGM can help PWD make needed lifestyle and medication adjustments.
As countries worldwide struggle with health care costs, it is important for all HCPs to recommend glucose monitoring approaches that are cost-effective. BGM is less expensive than CGM and sufficient for many people with type 2 diabetes. BGM also needs to be available for people using CGM. The ability to download, examine, interpret, and transmit stored glucose data facilitates communication with HCPs and empowers PWD to make health decisions to manage their condition. When used as part of a diabetes management plan and with appropriate support, BGM can help PWD better reach their glycemic goals.
Acknowledgments
Editorial and project management services were provided by Debbie Kendall of Kendall Editorial in Richmond, VA. The authors acknowledge the important contributions of Pat Stenger, RN, CDCES, and Holly Schachner, MD, to the section titled “Overcoming Barriers to BGM” and of Gregg D. Simonson, PhD, to the subsections on the AGP reports for CGM and BGM in the section titled “Use of BGM in CGM Users.”
Dualities of Interest
R.S.W. has received research support through her institution from Boehringer Ingelheim, Diasome, Eli Lilly, Insulet, Kowa, Medtronic, and Tolerion and receives royalty payments from Wolters Kluwer Health (Up-to-Date). She has also received honoraria for presentations from the American Diabetes Association.
G.A. has served as a consultant for Dexcom and Insulet, and her institution has received research grant support from AstraZeneca, Dexcom, Eli Lilly, Insulet, and Novo Nordisk.
T.S.B. has received research support from Abbott, Capillary Biomedical, Dexcom, Diasome, El Lilly, Kowa, Lexicon, Medtronic, Medtrum, Novo Nordisk, REMD, Sanofi, Senseonics, Viacyte, vTv Therapetics, and Zealand Pharmaceuticals; honoraria for consulting from Abbott, Lifescan, Novo Nordisk, and Sanofi; and honoraria for speaking engagements from Medtronic and Sanofi.
R.M.B. has received research support from, consulted for, or been on a scientific advisory board of Abbott Diabetes Care, Ascensia, DexCom, Hygieia, Johnson & Johnson, Lilly, Medtronic, Novo Nordisk, Onduo, Roche, Sanofi, and United Healthcare. His technology research is funded in part by the National Institutes of Health/ National Institute of Diabetes and Digestive and Kidney Diseases and the Leona M. and Harry B. Helmsley Charitable Trust. R.M.B.’s employer, the nonprofit HealthPartners Institute, contracts for his services, and no personal income goes to him for these services.
W.A.F. has received research support and has been a consultant to and speaker for Ascensia and Roche.
D.A.G. is a consultant for Lifescan and Lifescan Diabetes Institute; serves on an advisory board for Novo Nordisk; in addition to her consulting work and prior to the publication of this compendium, she was hired as an employee of Dexcom.
L.A.Y. has received research support from the American Diabetes Association, Bayer, Boehringer Ingelheim, Dexcom, Johnson & Johnson, Lexicon, Novo Nordisk, Sanofi, and vTv Therapeutics.
No other potential conflicts of interest relevant to this work were reported.
Author Contributions
Lead author R.S.W. reviewed all content and wrote the introduction and conclusion. G.A. wrote the section titled “Innovations in BGM.” T.S.B. wrote the section titled “BGM Accuracy.” R.M.B. wrote section titled “Use of BGM in CGM Users.” W.A.F. wrote the section titled “Overcoming Barriers to BGM.” D.A.G. wrote the section titled “Using BGM Data to Achieve Treatment Goals.” L.A.Y. wrote the section titled “Recommendations for BGM Use.” All authors reviewed and edited the manuscript and approved the final version for publication. R.S.W. is the guarantor of this work.
References
- 1.
- Howe-Davies S, Holman RR, Phillips M, Turner RC. Home blood sampling for plasma glucose assay in control of diabetes. BMJ 1978;2:596–598 [PMC free article: PMC1607495] [PubMed: 568013]
- 2.
- Skyler JS, Lasky IA, Skyler DL, Robertson EG, Mintz DH. Home blood glucose monitoring as an aid in diabetes management. Diabetes Care 1978;1:150–157 [PubMed: 729439]
- 3.
- Hirsch IB. History of glucose monitoring. In Hirsch IB, Battelino T, Peters AL, Chamberlain JJ, Aleppo G, Bergenstal RM. Role of Continuous Glucose Monitoring in Diabetes Treatment. Arlington, Va., American Diabetes Association, 2018
- 4.
- Diabetes Control and Complications Trial Research Group; Nathan DM, Genuth S, Lachin J, et al. The effect of intensive treatment of diabetes on the development and progression of long-term complications in insulin-dependent diabetes mellitus. N Engl J Med 1993;329:977–986 [PubMed: 8366922]
- 5.
- American Diabetes Association. Consensus statement on self-monitoring of blood glucose. Diabetes Care 1987;10:95–99 [PubMed: 3552518]
- 6.
- Joint Committee for Guides in Metrology. International Vocabulary of Metrology – Basic and General Concepts and Associated Terms (VIM). 3rd ed. 2008 version with minor corrections. Available from https://www
.bipm.org /utils/common/documents /jcgm/JCGM_200_2012.pdf. Accessed 26 July 2020 - 7.
- Clarke WL, Cox D, Gonder-Frederick LA, Carter W, Pohl SL. Evaluating clinical accuracy of systems for self-monitoring of blood glucose. Diabetes Care 1987;10:622–628 [PubMed: 3677983]
- 8.
- Parkes JL, Slatin SL, Pardo S, Ginsberg BH. A new consensus error grid to evaluate the clinical significance of inaccuracies in the measurement of blood glucose. Diabetes Care 2000;23:1143–1148 [PubMed: 10937512]
- 9.
- U.S. Food and Drug Administration. Self-monitoring blood glucose test systems for over-the-counter use: guidance for Industry and Food and Drug Administration staff. Available from https://www
.fda.gov/regulatory-information /search-fda-guidance-documents /self-monitoring-blood-glucose-test-systems-over-counter-use-0. Accessed 26 July 2020 - 10.
- U.S. Food and Drug Administration. Blood glucose monitoring test systems for prescription point-of-care use: guidance for industry and Food and Drug Administration staff. Available from https://www
.fda.gov/regulatory-information /search-fda-guidance-documents /blood-glucose-monitoring-test-systems-prescription-point-care-use. Accessed 26 July 2020 - 11.
- Cornes MP, Atherton J, Pourmahram G, et al. Monitoring and reporting of preanalytical errors in laboratory medicine: the UK situation. Ann Clin Biochem 2016;53:279–284 [PubMed: 26195485]
- 12.
- Hirose T, Mita T, Fujitani Y, Kawamori R, Watada H. Glucose monitoring after fruit peeling: pseudohyperglycemia when neglecting hand washing before fingertip blood sampling: wash your hands with tap water before you check blood glucose level. Diabetes Care 2011;34:596–597 [PMC free article: PMC3041187] [PubMed: 21282342]
- 13.
- Omengue AM, Sobngwi E, Dehayem M, et al. Effect of body lotions on capillary blood glucose measurement: interference of hydroquinone-containing body lotion with capillary glucose measurement. Eur Endocrinol 2018;14:44–46 [PMC free article: PMC5954594] [PubMed: 29922351]
- 14.
- Ginsberg BH. Factors affecting blood glucose monitoring: sources of errors in measurement. J Diabetes Sci Technol 2009;3:903–913 [PMC free article: PMC2769960] [PubMed: 20144340]
- 15.
- Frias JP, Lim CG, Ellison JM, Montandon CM. Review of adverse events associated with false glucose readings measured by GDH-PQQ–based glucose test strips in the presence of interfering sugars. Diabetes Care 2010;33:728–729 [PMC free article: PMC2845013] [PubMed: 20351227]
- 16.
- Klonoff DC, Parkes JL, Kovatchev BP, et al. Investigation of the accuracy of 18 marketed blood glucose monitors. Diabetes Care 2018;41:1681–1688 [PubMed: 29898901]
- 17.
- Freckmann G, Schmid C, Baumstark A, Pleus S, Link M, Haug C. System accuracy evaluation of 43 blood glucose monitoring systems for self-monitoring of blood glucose according to DIN EN ISO 15197. J Diabetes Sci Technol 2012;6:1060–1075 [PMC free article: PMC3570840] [PubMed: 23063032]
- 18.
- Kerr D. Poor numeracy: the elephant in the diabetes technology room. J Diabetes Sci Technol 2010;4:1284–1287 [PMC free article: PMC3005037] [PubMed: 21129322]
- 19.
- Given JE, O’Kane MJ, Bunting BP, Coates VE. Comparing patient-generated blood glucose diary records with meter memory in diabetes: a systematic review. Diabet Med 2013;30:901–913 [PubMed: 23324062]
- 20.
- Polonsky WH, Fisher L, Schikman CH, et al. Structured self-monitoring of blood glucose significantly reduces A1C levels in poorly controlled, noninsulin-treated type 2 diabetes: results from the Structured Testing Program study. Diabetes Care 2011;34:262–267 [PMC free article: PMC3024331] [PubMed: 21270183]
- 21.
- Bosi E, Scavini M, Ceriello A, et al; PRISMA Study Group. Intensive structured self-monitoring of blood glucose and glycemic control in noninsulin-treated type 2 diabetes: the PRISMA randomized trial. Diabetes Care 2013;36:2887–2894 [PMC free article: PMC3781531] [PubMed: 23735724]
- 22.
- Fleming GA, Petrie JR, Bergenstal RM, Holl RW, Peters AL, Heinemann L. Diabetes digital app technology: benefits, challenges, and recommendations: a consensus report by the European Association for the Study of Diabetes (EASD) and the American Diabetes Association (ADA) Diabetes Technology Working Group. Diabetes Care 2020;43:250–260 [PubMed: 31806649]
- 23.
- Klatman EL, Jenkins AJ, Ahmedani MY, Ogle GD. Blood glucose meters and test strips: global market and challenges to access in low-resource settings. Lancet Diabetes Endocrinol 2019;7:150–160 [PubMed: 30072234]
- 24.
- Roche. Roche receives CE Mark for its Accu-Chek SugarView app. Available from https://www
.roche.com /media/releases/med-cor-2019-12-10.htm. Accessed 26 July 2020 - 25.
- Grady M, Katz LB, Strunk CS, Cameron H, Levy BL. Examining the impact of a novel blood glucose monitor with color range indicator on decision-making in patients with type 1 and type 2 diabetes and its association with patient numeracy level. JMIR Diabetes 2017;2:e24 [PMC free article: PMC6238893] [PubMed: 30291065]
- 26.
- Bailey TS, Grunberger G, Bode BW, et al; American Association of Clinical Endocrinologists (AACE); American College of Endocrinology (ACE). American Association of Clinical Endocrinologists and American College of Endocrinology. 2016 outpatient glucose monitoring consensus statement. Endocr Pract 2016;22:231–261 [PubMed: 26848630]
- 27.
- McQueen RB, Breton MD, Ott M, Koa H, Beamer B, Campbell JD. Economic value of improved accuracy for self-monitoring of blood glucose devices for type 1 diabetes in Canada. J Diabetes Sci Technol 2015;10:366–377 [PMC free article: PMC4773951] [PubMed: 26275642]
- 28.
- McQueen RB, Breton MD, Craig J, et al. Economic value of improved accuracy for self-monitoring of blood glucose devices for type 1 and type 2 diabetes in England. J Diabetes Sci Technol 2018;12:992–1001 [PMC free article: PMC6134622] [PubMed: 29681171]
- 29.
- American Diabetes Association. 7. Diabetes technology: Standards of Medical Care in Diabetes—2020. Diabetes Care 2020;43(Suppl. 1):S77–S88 [PubMed: 31862750]
- 30.
- Engler R, Routh TL, Lucisano JY. Adoption barriers for continuous glucose monitoring and their potential reduction with a fully implanted system: results from patient preference surveys. Clin Diabetes 2018;36:50–58 [PMC free article: PMC5774999] [PubMed: 29382979]
- 31.
- Barnett AH, Krentz AJ, Strojek K, et al. The efficacy of self-monitoring of blood glucose in the management of patients with type 2 diabetes treated with a gliclazide modified release–based regimen: a multicentre, randomized, parallel-group, 6-month evaluation (DINAMIC 1 study). Diabetes Obes Metab 2008;10:1239–1247 [PubMed: 18494813]
- 32.
- Durán A, Martín P, Runkle I, et al. Benefits of self-monitoring blood glucose in the management of new-onset type 2 diabetes mellitus: the St Carlos Study, a prospective randomized clinic-based interventional study with parallel groups. J Diabetes 2010;2:203–211 [PubMed: 20923485]
- 33.
- Guerci B, Drouin P, Grangé V, et al; ASIA Group. Self-monitoring of blood glucose significantly improves metabolic control in patients with type 2 diabetes mellitus: the Auto-Surveillance Intervention Active (ASIA) study. Diabetes Metab 2003;29:587–594 [PubMed: 14707887]
- 34.
- Schwedes U, Siebolds M, Mertes G; SMBG Study Group. Meal-related structured self-monitoring of blood glucose: effect on diabetes control in non-insulin–treated type 2 diabetic patients. Diabetes Care 2002;25:1928–1932 [PubMed: 12401734]
- 35.
- Davidson MB, Castellanos M, Kain D, Duran P. The effect of self monitoring of blood glucose concentrations on glycated hemoglobin levels in diabetic patients not taking insulin: a blinded, randomized trial. Am J Med 2005;118:422–425 [PubMed: 15808142]
- 36.
- Farmer AJ, Wade AN, French DP, et al. Blood glucose self-monitoring in type 2 diabetes: a randomised controlled trial. Health Technol Assess 2009;13:iii–iv, ix–xi, 1–50 [PubMed: 19254484]
- 37.
- Kleefstra N, Hortensius J, Logtenberg SJJ, et al. Self-monitoring of blood glucose in tablet-treated type 2 diabetic patients (ZODIAC). Neth J Med 2010;68:311–316 [PubMed: 20739728]
- 38.
- Muchmore DB, Springer J, Miller M. Self-monitoring of blood glucose in overweight type 2 diabetic patients. Acta Diabetol 1994;31:215–219 [PubMed: 7888692]
- 39.
- Polonsky WH, Fisher L, Schikman CH, et al. A structured self-monitoring of blood glucose approach in type 2 diabetes encourages more frequent, intensive, and effective physician interventions: results from the STeP study. Diabetes Technol Ther 2011;13:797–802 [PubMed: 21568751]
- 40.
- Malanda UL, Welschen LMC, Riphagen II, Dekker JM, Nijpels G, Bot SDM. Self-monitoring of blood glucose in patients with type 2 diabetes mellitus who are not using insulin. Cochrane Database Syst Rev 2012;1:CD005060 [PubMed: 22258959]
- 41.
- Farmer AJ, Perera R, Ward A, et al. Meta-analysis of individual patient data in randomised trials of self monitoring of blood glucose in people with non-insulin treated type 2 diabetes. BMJ 2012;344:e486 [PubMed: 22371867]
- 42.
- Machry RV, Rados DV, de Gregório GR, Rodrigues TC. Self-monitoring blood glucose improves glycemic control in type 2 diabetes without intensive treatment: a systematic review and meta-analysis. Diabetes Res Clin Pract 2018;142:173–187 [PubMed: 29857093]
- 43.
- Young LA, Buse JB, Weaver MA, et al; Monitor Trial Group. Glucose self-monitoring in non-Insulin–treated patients with type 2 diabetes in primary care settings: a randomized trial. JAMA Intern Med 2017;177:920–929 [PMC free article: PMC5818811] [PubMed: 28600913]
- 44.
- International Diabetes Federation. Self-monitoring of blood glucose in non-insulin treated type 2 diabetes. Available from https://www
.idf.org/e-library /guidelines /85-self-monitoring-of-blood-glucose-in-non-insulin-treated-type-2-diabetes.html. Accessed 29 July 2020 - 45.
- American Diabetes Association. 6. Glycemic targets: Standards of Medical Care in Diabetes—2020. Diabetes Care 2020;43(Suppl. 1):S66–S76 [PubMed: 31862749]
- 46.
- American Association of Diabetes Educators. An effective model of diabetes care and education: revising the AADE7 Self-Care Behaviors. Diabetes Educ 2020;46:139–160 [PubMed: 31928334]
- 47.
- Association of Diabetes Care and Education Specialists. The Art and Science of Diabetes Care and Education. 5th ed. Chicago, IL, Association of Diabetes Care and Education Specialists, 2020
- 48.
- American Association of Diabetes Care and Education Specialists. AADE7 self-care behaviors. Available from https://www
.diabeteseducator .org/living-with-diabetes /aade7-self-care-behaviors. Accessed 29 July 2020 - 49.
- Bonomo K, De Salve A, Fiora E, et al. Evaluation of a simple policy for pre- and post-prandial blood glucose self-monitoring in people with type 2 diabetes not on insulin. Diabetes Res Clin Pract 2010;87:246–251 [PubMed: 19954855]
- 50.
- Schnell O, Alawi H, Battelino T, et al. Addressing schemes of self-monitoring of blood glucose in type 2 diabetes: a European perspective and expert recommendation. Diabetes Technol Ther 2011;13:959–965 [PubMed: 21714682]
- 51.
- Beck J, Greenwood DA, Blanton L, et al; 2017 Standards Revision Task Force. 2017 National standards for diabetes self-management education and support. Diabetes Educ 2017;43:449–464 [PubMed: 28753378]
- 52.
- Powers MA, Bardsley JK, Cypress M, et al. Diabetes self-management education and support in adults with type 2 diabetes: a consensus report of the American Diabetes Association, the Association of Diabetes Care & Education Specialists, the Academy of Nutrition and Dietetics, the American Academy of Family Physicians, the American Academy of PAs, the American Association of Nurse Practitioners, and the American Pharmacists Association. Diabetes Care 2020;43:1636–1649 [PubMed: 32513817]
- 53.
- Ross T. Greenwood D. A paradigm shift: taking a solution-focused approach to practice. ADCES in Practice 2020;8:40–45
- 54.
- Lutz AB. Learning Solution-Focused Therapy: An Illustrated Guide. Arlington. VA, American Psychiatric Publishing, 2013
- 55.
- Centers for Disease Control and Prevention. Diabetes self-management education and support (DSMES) toolkit. Available from https://www
.cdc.gov/diabetes /dsmes-toolkit /background/benefits.html. Accessed 30 July 2020 - 56.
- Berard LD, Siemens R, Woo V. 2018 clinical practice guidelines: monitoring glycemic control. Can J Diabetes 2018;42(Suppl. 1):S47–S53 [PubMed: 29650111]
- 57.
- Fisher WA, Kohut T, Stegner P, Schachner H. Understanding self-monitoring of blood glucose among individuals with type 1 and type 2 diabetes: an Information–Motivation–Behavioral Skills analysis. Diabetes Educ 2011;37:85–94 [PubMed: 21292622]
- 58.
- American Diabetes Association. 5. Facilitating behavior change and well-being to improve health outcomes: Standards of Medical Care in Diabetes—2020. Diabetes Care 2020;43(Suppl. 1):S48–S65 [PubMed: 31862748]
- 59.
- Fisher WA, Cornman DH, Kohut T, Schachner H, Stenger P. What primary care providers can do to address barriers to self-monitoring of blood glucose. Clin Diabetes 2013;31:34–42
- 60.
- Rollnick S, Miller WR, Butler CC. Motivational Interviewing in Health Care: Helping Patients Change Behavior. New York, NY, Guilford Press, 2008
- 61.
- Ekhlaspour L, Mondesir D, Lautsch N, et al. Comparative accuracy of 17 point-of-care glucose meters. J Diabetes Sci Technol 2017;11:558–566 [PMC free article: PMC5505415] [PubMed: 27697848]
- 62.
- Edelman SV, Argento NB, Pettus J, Hirsch IB. Clinical implications of real-time and intermittently scanned continuous glucose monitoring. Diabetes Care 2018;41:2265–2274 [PubMed: 30348844]
- 63.
- Petrie JR, Peters AL, Bergenstal RM, Holl RW, Fleming GA, Heinemann L. Improving the clinical value and utility of CGM systems: issues and recommendations: a joint statement of the European Association for the Study of Diabetes and the American Diabetes Association Diabetes Technology Working Group. Diabetes Care 2017;40:1614–1621 [PubMed: 29070577]
- 64.
- Oskarsson P, Antuna R, Geelhoed-Duijvestijn P, Kröger J, Weitgasser R, Bolinder J. Impact of flash glucose monitoring on hypoglycaemia in adults with type 1 diabetes managed with multiple daily injection therapy: a pre-specified subgroup analysis of the IMPACT randomised controlled trial. Diabetologia 2018;61:539–550 [PMC free article: PMC6448969] [PubMed: 29273897]
- 65.
- Haak T, Hanaire H, Ajjan R, Hermanns N, Riveline J-P, Rayman G. Flash glucose-sensing technology as a replacement for blood glucose monitoring for the management of insulin-treated type 2 diabetes: a multicenter, open-label randomized controlled trial. Diabetes Ther 2017;8:55–73 [PMC free article: PMC5306122] [PubMed: 28000140]
- 66.
- Heinemann L, Freckmann G, Ehrmann D, et al. Real-time continuous glucose monitoring in adults with type 1 diabetes and impaired hypoglycaemia awareness or severe hypoglycaemia treated with multiple daily insulin injections (HypoDE): a multicentre, randomised controlled trial. Lancet 2018;391:1367–1377 [PubMed: 29459019]
- 67.
- Janapala RN, Jayaraj JS, Fathima N, et al. Continuous glucose monitoring versus self-monitoring of blood glucose in type 2 diabetes mellitus: a systematic review with meta-analysis. Cureus 2019;11:e5634 [PMC free article: PMC6822918] [PubMed: 31700737]
- 68.
- Maiorino MI, Signoriello S, Maio A, et al. Effects of continuous glucose monitoring on metrics of glycemic control in diabetes: a systematic review with meta-analysis of randomized controlled trials. Diabetes Care 2020;43:1146–1156 [PubMed: 32312858]
- 69.
- Foster NC, Beck RW, Miller KM, et al. State of type 1 diabetes management and outcomes from the T1D Exchange in 2016–2018. Diabetes Technol Ther 2019;21:66–72 [PMC free article: PMC7061293] [PubMed: 30657336]
- 70.
- Beck RW, Brown SA, Lum JW, Kovatchev BP. Nonadjunctive use of continuous glucose monitoring: the end of fingersticks? Diabetes Technol Ther 2020 Feb;22:67–68 [PubMed: 31657622]
- 71.
- Mullen DM, Bergenstal RM, Johnson ML. Patients’ interpretation of ambulatory glucose profile (AGP) CGM and pump reports [Abstract]. Diabetes 2020;69 (Suppl. 1):1204-P
- 72.
- Danne T, Nimri R, Battelino T, et al. International consensus on use of continuous glucose monitoring. Diabetes Care 2017;40:1631–1640 [PMC free article: PMC6467165] [PubMed: 29162583]
- 73.
- Battelino T, Danne T, Bergenstal RM, et al. Clinical targets for continuous glucose monitoring data interpretation: recommendations from the International Consensus on Time in Range. Diabetes Care 2019;42:1593–1603 [PMC free article: PMC6973648] [PubMed: 31177185]
- 74.
- Bergenstal RM, Ahmann AJ, Bailey T, et al. Recommendations for standardizing glucose reporting and analysis to optimize clinical decision making in diabetes: the ambulatory glucose profile (AGP). Diabetes Technol Ther 2013;15:198–211 [PubMed: 23448694]
- 75.
- Mazze RS, Lucido D, Langer O, Hartmann K, Rodbard D. Ambulatory glucose profile: representation of verified self-monitored blood glucose data. Diabetes Care 1987;10:111–117 [PubMed: 3552508]
- 76.
- Ziegler R, Heinemann L, Freckmann G, Schnell O, Hinzmann R, Kulzer B. Intermittent use of continuous glucose monitoring: expanding the clinical value of CGM. J Diabetes Sci Technol. Epub ahead of print on 17 February 2020 (doi: 10.1177/1932296820905577 [PMC free article: PMC8120049] [PubMed: 32064909]
- 77.
- Marks BE, Wolfsdorf JI. Monitoring of pediatric type 1 diabetes. Front Endocrinol (Lausanne). 2020;11:128 [PMC free article: PMC7089921] [PubMed: 32256447]
- 78.
- Heinemann L. Continuous glucose monitoring (CGM) or blood glucose monitoring (BGM): interactions and implications. J Diabetes Sci Technol 2018;12:873–879 [PMC free article: PMC6134292] [PubMed: 29648465]
- 79.
- Rossi MC, Lucisano G, Ceriello A, et al; AMD Annals-SMBG Study Group. Real-world use of self-monitoring of blood glucose in people with type 2 diabetes: an urgent need for improvement. Acta Diabetol 2018;55:1059–1066 [PubMed: 30062588]
- 80.
- Heinemann L, Stuhr A, Brown A, et al. Self-measurement of blood glucose and continuous glucose monitoring: is there only one future? Eur Endocrinol 2018;14:24–29 [PMC free article: PMC6182926] [PubMed: 30349591]
- 81.
- Miller KM, Beck RW, Bergenstal RM, et al; T1D Exchange Clinic Network. Evidence of a strong association between frequency of self-monitoring of blood glucose and hemoglobin A1c levels in T1D Exchange clinic registry participants. Diabetes Care 2013;36:2009–2014 [PMC free article: PMC3687326] [PubMed: 23378621]
- 82.
- Ziegler R, Heidtmann B, Hilgard D, Hofer S, Rosenbauer J, Holl R; DPV-Wiss-Initiative. Frequency of SMBG correlates with HbA1c and acute complications in children and adolescents with type 1 diabetes. Pediatr Diabetes 2011;12:11–17 [PubMed: 20337978]
- 83.
- Ristau RA, Yang J, White JR. Evaluation and evolution of diabetes mobile applications: key factors for health care professionals seeking to guide patients. Diabetes Spectr 2013;26:211–215
- 84.
- Kramer DB, Xu S, Kesselheim AS. Regulation of medical devices in the United States and European Union. N Engl J Med 2012;366:848–855 [PubMed: 22332952]
- 85.
- U.S. Food and Drug Administration. Policy for device software function and mobile medical applications: guidance for industry and Food and Drug Administration staff. Available from https://www
.fda.gov/media/80958/download. Accessed 3 June 2020 - 86.
- U.S. Food and Drug Administration. Device software functions including mobile medical applications. Available from https://www
.fda.gov/medical-devices /digital-health /device-software-functions-including-mobile-medical-applications. Accessed 3 June 2020 - 87.
- Glooko. Why we are a platform, not just an app. Available from https://www
.glooko.com /2016/05/platform-not-just-app. Accessed 6 June 2020 - 88.
- Tidepool. How to copy as text (Basics, Trends, Device Settings). Available from https://support
.tidepool .org/hc/en-us/articles /360035823492-How-to-copy-as-text-Basics-Trends-Device-Settings. Accessed 6 June 2020 - 89.
- Pew Research Center. Mobile fact sheet. Available from https://www
.pewresearch .org/internet/fact-sheet/mobile. Accessed 4 May 2020 - 90.
- Mayberry LS, Lyles CR, Oldenburg B, Osborn CY, Parks M, Peek ME. mHealth interventions for disadvantaged and vulnerable people with type 2 diabetes. Curr Diab Rep 2019;19:148 [PMC free article: PMC7232776] [PubMed: 31768662]
- 91.
- Cavanaugh K, Huizinga MM, Wallston KA, et al. Association of numeracy and diabetes control. Ann Intern Med 2008;148:737–746 [PubMed: 18490687]
- 92.
- Hirsch IB, Parkin CG. Unknown safety and efficacy of smartphone bolus calculator apps puts patients at risk for severe adverse outcomes. J Diabetes Sci Technol 2016;10:977–980 [PMC free article: PMC4928215] [PubMed: 26798082]
- 93.
- Huckvale K, Adomaviciute S, Prieto JT, Leow MK, Car J. Smartphone apps for calculating insulin dose: a systematic assessment. BMC Med 2015;13:106 [PMC free article: PMC4433091] [PubMed: 25943590]
- 94.
- Eiland L, McLarney M, Thangavelu T, Drincic A. App-based insulin calculators: current and future state. Curr Diab Rep 2018;18:123 [PubMed: 30284645]
- 95.
- Levine BJ, Close KL, Gabbay RA. Reviewing U.S. connected diabetes care: the newest member of the team. Diabetes Technol Ther 2020;22:1–9 [PubMed: 31483160]
- 96.
- Virta Health. Home page. Available from https://virtahealth
.com. Accessed 6 June 2020 - 97.
- Onduo. Home page. Available from https://onduo
.com. Accessed 6 June 2020 - 98.
- Turner RM, Ma Q, Lorig K, Greenberg J, DeVries AR. Evaluation of a diabetes self-management program: claims analysis on comorbid illnesses, health care utilization, and cost. J Med Internet Res 2018;20:e207 [PMC free article: PMC6035341] [PubMed: 29934284]
- 99.
- Cecelia Health. Improving diabetes health through scalable, personalized coaching. Available from https://www
.ceceliahealth .com/improving-diabetes-health-through-scalable-personalized-coaching. Accessed 30 July 2020 - 100.
- Gal RL, Cohen NJ, Kruger D, et al. Diabetes telehealth solutions: improving self-management through remote initiation of continuous glucose monitoring. J Endocr Soc 2020;4:bvaa076 [PMC free article: PMC7448105] [PubMed: 32864542]
- 101.
- Downing J, Bollyky J, Schneider J. Use of a connected glucose meter and certified diabetes educator coaching to decrease the likelihood of abnormal blood glucose excursions: the Livongo for Diabetes program. J Med Internet Res 2017;19:e234 [PMC free article: PMC5527250] [PubMed: 28698167]
- 102.
- Bollyky JB, Bravata D, Yang J, Williamson M, Schneider J. Remote lifestyle coaching plus a connected glucose meter with certified diabetes educator support improves glucose and weight loss for people with type 2 diabetes. J Diabetes Res 2018;2018:3961730 [PMC free article: PMC5977036] [PubMed: 29888288]
- 103.
- Bollyky JB, Melton ST, Xu T, Painter SL, Knox B. The effect of a cellular-enabled glucose meter on glucose control for patients with diabetes: prospective pre-post study. JMIR Diabetes 2019;4:e14799 [PMC free article: PMC6803884] [PubMed: 31593545]
- 104.
- Debong F, Mayer H, Kober J. Real-world assessments of mySugr mobile health app. Diabetes Technol Ther 2019;21(Suppl. 2):S235–S240 [PubMed: 31169427]
- 105.
- Dixon RF. Real-world glycemic control data from the initial launch of the Onduo Virtual Diabetes Clinic. Presentation at the American Diabetes Association research symposium on Use of Real-World Data to Improve the Prevention and Care of Diabetes-Related Outcomes, Washington, DC, 16–18 November, 2018
- 106.
- Layne JE, Zisser H, Bergenstal RM, et al. Change in A1C with and without intermittent use of CGM in adults with type 2 diabetes participating in the Onduo virtual diabetes clinic [Abstract]. Diabetes 2020;69(Suppl. 1):70-OR
- 107.
- Osborn CY, van Ginkel JR, Rodbard D, et al. One Drop | Mobile: an evaluation of hemoglobin A1c improvement linked to app engagement. JMIR Diabetes 2017;2:e21 [PMC free article: PMC6238886] [PubMed: 30291059]
- 108.
- Osborn CY, van Ginkel JR, Marrero DG, Rodbard D, Huddleston B, Dachis J. One Drop | Mobile on iPhone and Apple Watch: an evaluation of HbA1c improvement associated with tracking self-care. JMIR Mhealth Uhealth 2017;5:e179 [PMC free article: PMC5729227] [PubMed: 29187344]
- 109.
- Kumar S, Moseson H, Uppal J, Juusola JL. A diabetes mobile app with in-app coaching from a certified diabetes educator reduces A1C for individuals with type 2 diabetes. Diabetes Educ 2018;44:226–236 [PubMed: 29575982]
- 110.
- Athinarayanan SJ, Adams RN, Hallberg SJ, et al. Long-term effects of a novel continuous remote care intervention including nutritional ketosis for the management of type 2 diabetes: a 2-year non-randomized clinical trial. Front Endocrinol (Lausanne) 2019;10:348 [PMC free article: PMC6561315] [PubMed: 31231311]
- 111.
- Quinn CC, Clough SS, Minor JM, Lender D, Okafor MC, Gruber-Baldini A. WellDoc mobile diabetes management randomized controlled trial: change in clinical and behavioral outcomes and patient and physician satisfaction. Diabetes Technol Ther 2008;10:160–168 [PubMed: 18473689]
- 112.
- Quinn CC, Shardell MD, Terrin ML, Barr EA, Ballew SH, Gruber-Baldini AL. Cluster-randomized trial of a mobile phone personalized behavioral intervention for blood glucose control. Diabetes Care 2011;34:1934–1942 [PMC free article: PMC3161305] [PubMed: 21788632]
- 113.
- Agarwal P, Mukerji G, Desveaux L, et al. Mobile app for improved self-management of type 2 diabetes: multicenter pragmatic randomized controlled trial. JMIR Mhealth Uhealth 2019;7:e10321 [PMC free article: PMC6329896] [PubMed: 30632972]
The opinions expressed are those of the authors and do not necessarily reflect those of Ascensia, Lifescan, or the American Diabetes Association. The content was developed by the authors and does not represent the policy or position of the American Diabetes Association, any of its boards or committees, or any of its journals or their editors or editorial boards.
To request permission to reuse or reproduce any portion of this publication, please contact gro.setebaid@snoissimrep.
- [PREFACE]
- [INTRODUCTION]
- BGM ACCURACY
- RECOMMENDATIONS FOR BGM USE
- USING BGM DATA TO ACHIEVE TREATMENT GOALS
- OVERCOMING BARRIERS TO BGM
- Assisting Patients in Overcoming Obstacles to BGM
- Embracing the Spirit of Collaborative Problem-Solving
- USE OF BGM IN CGM USERS
- INNOVATIONS IN BGM
- CONCLUSION
- Acknowledgments
- References
- NLM CatalogRelated NLM Catalog Entries
- PMCPubMed Central citations
- PubMedLinks to PubMed
- The Role of Blood Glucose Monitoring in Diabetes ManagementThe Role of Blood Glucose Monitoring in Diabetes Management
Your browsing activity is empty.
Activity recording is turned off.
See more...