NCBI Bookshelf. A service of the National Library of Medicine, National Institutes of Health.
National Academies of Sciences, Engineering, and Medicine; Division of Behavioral and Social Sciences and Education; Health and Medicine Division; Board on Behavioral, Cognitive, and Sensory Sciences; Board on Health Sciences Policy; Committee on the Health and Medical Dimensions of Social Isolation and Loneliness in Older Adults. Social Isolation and Loneliness in Older Adults: Opportunities for the Health Care System. Washington (DC): National Academies Press (US); 2020 Feb 27.
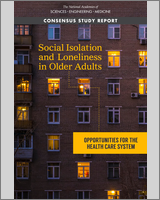
Social Isolation and Loneliness in Older Adults: Opportunities for the Health Care System.
Show detailsMany measurement tools exist to assess social isolation and loneliness (and other related concepts), but to date most of the established and widely implemented tools have been developed for research purposes. Research using these tools has focused on defining the prevalence, the risk factors, and the health impacts of social isolation and loneliness. More recently, there has been a focus on using these tools to assess the effectiveness of interventions by using measures of social isolation and loneliness as outcomes. (See Chapter 9 for more on interventions.) This chapter will examine the use of different tools related to social isolation and loneliness primarily in the research setting, and it will explore research on the use of information technology to identify individuals at risk for social isolation and loneliness. Chapter 7 will discuss the application of these tools in clinical settings. Given the complexity of the terminology used in relation to social isolation and loneliness, a reminder of key definitions is provided in Box 6-1.
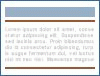
BOX 6-1
Key Definitions.
MEASUREMENT OF SOCIAL ISOLATION AND LONELINESS
The concepts of social isolation and loneliness have been defined in different ways (see Chapters 1 and 2), which has led to some variability in how these concepts are measured. When examining social isolation and loneliness in research, a number of tools capture elements of both social isolation and loneliness, which may obscure differences between these two concepts. In addition, in both research and clinical settings social isolation and loneliness may fluctuate over time. This underscores the need for serial testing to better ascertain changes over time, including the trajectories of these changes and their clinical relevance. However, measures that encompass elements of both social isolation and loneliness or, more broadly, social connection could be advantageous in clinical settings as they may probe both concepts, which would be more efficient, and they could possibly provide a stronger clinical signal. Because of the variability in existing measurement tools for social isolation and loneliness, Valtorta and colleagues (2016b) suggested that these tools can be classified along two dimensions: whether the measure looks at the structural or the functional aspects of social relationships and the degree of subjectivity required by respondents (see Figure 6-1). The researchers examined 54 measurement instruments and found that “tools explicitly designed for measuring loneliness . . . tend to be based on more subjective questions, whereas social network indices primarily use more objective measures” (p. 6).
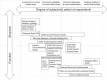
FIGURE 6-1
Multi-item questionnaires compared by structure versus function and for the degree of subjectivity. NOTE: MOS = Medical Outcomes Study; OARS = Older Americans Research and Service Center; SNI = Social Network Index; UCLA = University of California, Los (more...)
It is well accepted that the three-item UCLA Loneliness Scale (described later in this chapter) captures loneliness—a subjective self-reported measure. In contrast, the Duke Social Support Index (also described later in this chapter), while classically thought to measure social isolation, does include some subjective questions. Therefore, if a study uses the Duke Social Support Index and says it measures social isolation and not loneliness, the study may incorrectly conclude that it is only social isolation that has an effect or is being affected. The differences in measurement and how studies report outcomes as being either related to loneliness or social isolation may present challenges when comparing studies and even in meta-analyses if the studies are grouped according to how authors define social isolation and loneliness rather than according to the measurement tools used. Some of this variability in measurement likely accounts for the range of prevalence rates and inconsistencies in study conclusions. This creates a landscape in which the effects of social isolation and loneliness on health are demonstrated, but it is not always clear as to which has a greater influence. Because of this, when evaluating the literature it is imperative to examine how social isolation and loneliness are being defined and measured.
The following sections provide a list of and brief explanations for some of the most widely used measurement tools for social isolation and loneliness. The committee emphasizes that this is not a comprehensive list of all available tools and does not represent an endorsement of this committee but rather serves to demonstrate the range of tools being used. Ultimately, one size does not fit all. For any given intervention, the tool picked should be tailored to assess what change in social isolation or loneliness is expected to be affected, over what time period, and whether the effect is sustained. Unless there is compelling evidence to suggest a new measurement tool, researchers and program evaluators should try to use existing and validated tools (see Recommendation 7-1 in Chapter 7). However, questions remain as to how existing tools can be used in clinical settings (for more on this, see Chapter 7).
Berkman–Syme Social Network Index
The Berkman–Syme Social Network Index (see Box 6-2) focuses on the general adult population and measures social integration versus isolation by looking at marital status, frequency of contact with other people, participation in religious activities, and participation in other club or organization activities (Berkman and Syme, 1979). This measure was recommended for inclusion in electronic health records (EHRs) as a measure of social isolation by a prior Institute of Medicine committee (IOM, 2014). (See Chapter 7 for more on EHRs.)
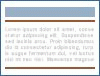
BOX 6-2
Berkman–Syme Social Network Index.
The Revised UCLA Loneliness Scale
The Revised UCLA (R-UCLA) Loneliness Scale is a 20-item, self-administered questionnaire that has become a standard measurement of subjective loneliness (Russell, 1996). The three-item UCLA Loneliness Scale was developed for use in telephone surveys in which questions are posed to the person being assessed (Hughes et al., 2004; see Box 6-3). The three-item scale is being used widely in both research and clinical settings in the United States as a brief assessment of loneliness.
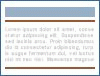
BOX 6-3
Three-Item UCLA Loneliness Scale.
Steptoe Social Isolation Index
In a study by Steptoe and colleagues (2013), the authors created an index of social isolation. The index involves a five-point scale, with one point being assigned for each of the following factors:
- Unmarried/not cohabiting
- Less than monthly contact (including face-to-face, by telephone, or in writing/email) with children
- Less than monthly contact (including face-to-face, by telephone, or in writing/email) with other family
- Less than monthly contact (including face-to-face, by telephone, or in writing/email) with friends
- No participation in social clubs, resident groups, religious groups, or committees
People with a score of 2 or more were defined as being socially isolated.
Duke Social Support Index
The Duke Social Support Index is a 35-item measure of various dimensions of social support, including social network (e.g., proximity of family), social interaction (e.g., frequency of interactions), subjective support (e.g., perceptions of relationships), and instrumental support (e.g., availability of help) (Koenig et al., 1993). However, the interview required for this measure is lengthy. As a result, two abbreviated versions of the tool have been developed: an 11-item scale that includes subscales for social interaction and subjective support and a 23-item scale that adds a subscale for instrumental support (Koenig et al., 1993).
Lubben Social Network Scale
The Lubben Social Network Scale is an adaptation of the Berkman–Syme Social Network Index developed to focus specifically on older adults (Lubben, 1988). At that time, Lubben found that marital status and participation in religious activities vary less in the older adult population. Therefore, this 10-item measure focuses more heavily on the quality and frequency of an individual's relationships with family and friends. Lubben and colleagues have published revised versions of the Lubben Social Network Scale including an abbreviated six-item version (Lubben and Gironda, 2003; Lubben et al., 2006).
de Jong Gierveld Loneliness Scale
The de Jong Gierveld Loneliness Scale is an 11-item questionnaire designed to assess both overall loneliness and two specific types of loneliness (de Jong Gierveld and Kamphuis, 1985; see Table 6-1). This scale includes two subscales: a three-item emotional loneliness subscale (aimed at loneliness due to the lack of a close, intimate relationship) and a three-item social loneliness subscale (aimed at loneliness due to the lack of a broader social network) (de Jong Gierveld and Van Tilburg, 2006). As with the R-UCLA Loneliness Scale, the length of this instrument can be challenging to use in large surveys. As a result, a shortened 6-item scale comprising two of the 3-item subscales within the original 11-item questionnaire has been used (de Jong Gierveld and Van Tilburg, 2006; Weiss, 1974; see Table 6-1).
Cornwell Perceived Isolation Scale
Cornwell and Waite (2009) measured perceived isolation by creating a nine-item scale that combines indicators of perceived lack of social support and of loneliness. The first six items came from asking individuals the following questions: “How often can you open up to your family if you need to talk about your worries?” and “How often can you rely on them for help if you have a problem?” (Cornwell and Waite, 2009). These same questions were then asked again in relation to their friends and then in relation to their spouse or partner. The last three items come from the three-item UCLA Loneliness Scale (described above). For this scale, the authors standardized each item and averaged scores, with a higher score indicating greater perceived isolation.
TABLE 6-1Items of the 11-Item (original) and 6-Item (short) de Jong Gierveld Loneliness Scales
Statement | Original Emotional Subscale | Original Social Subscale | Short Emotional Subscale | Short Social Subscale |
---|---|---|---|---|
| X | |||
| X | |||
| X | X | ||
| X | X | ||
| X | |||
| X | |||
| X | X | ||
| X | X | ||
| X | X | ||
| X | X | ||
| X |
- a
Item should be reversed before scoring.
Campaign to End Loneliness Measurement Tool
The Campaign to End Loneliness (see more on the campaign in Chapters 8 and 9) developed a three-item tool to measure loneliness (see Box 6-4). The guidance for using the tool notes that “the main purpose of this tool is to measure the change that happens as a result of an intervention to address loneliness” (Campaign to End Loneliness, 2019a, p. 12). The tool was developed “in partnership with over 50 older people, service providers, commissioners and housing associations” (Campaign to End Loneliness, 2019a, p. 13).
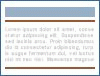
BOX 6-4
Campaign to End Loneliness Measurement Tool.
MEASURING IMPACT FOR SOCIAL ISOLATION AND LONELINESS
The committee's review of the literature on social isolation and loneliness revealed a number of measurement and interpretation challenges pertaining to prevalence rates and outcomes that need to be addressed. These challenges are summarized in the following sections.
Measuring the Prevalence of Social Isolation and Loneliness
The committee emphasizes the importance of using validated tools in the assessment of social isolation and loneliness (rather than using only parts of existing tools or creating new, unvalidated tools). Validation means that the tool has been shown to accurately reflect the level of social isolation or loneliness (and is not biased). When studying the prevalence of loneliness or isolation, it is most important to choose a measurement tool that matches the research question. For example, if one is looking to establish the prevalence of loneliness in a given population, it is necessary to pick a measurement tool that is validated to measure loneliness rather than other aspects of social connection. (Chapter 7 further discusses the importance of using validated tools in clinical settings.)
Given the confusion surrounding the definitions of social isolation and loneliness, using a validated tool for the specific construct being evaluated is of the utmost importance. Employing an unvalidated tool or, as mentioned previously, a tool that was designed to assess social isolation for a study that is actually examining loneliness, may yield inaccurate results. To address this issue, Chapter 7 (and Recommendation 7-1 in particular) further discusses the use of validated tools in clinical settings.
Measuring Social Isolation and Loneliness as Outcomes of an Intervention
When seeking to understand whether a particular intervention has an effect on social isolation or loneliness, it is important to choose a measurement tool that can be used both before and after the implementation of an intervention in order to detect and quantify any difference in the score. Although numerous measurement tools are available, in those cases when a researcher seeks to compare the impacts of different interventions, it is important to use the same measurement tool for the different interventions being compared.
In observational or longitudinal studies, it is critical to use a common, standardized assessment at all time-points during the study duration. The length of follow-up and frequency of measurement are particularly important for a few reasons. For example, because social isolation and loneliness may be episodic for some, it is important to have several time periods of measurement in order to determine if observed differences are true reflections of changes in outcomes or if they represent measurement variability or natural fluctuations over time. There is no set standard for the number of time-points or numbers of assessments per unit of time used to determine social isolation and loneliness. As a general principle, having more than two data points (the beginning and the end of the study) can help to reliably measure trajectories of social isolation and loneliness. Because of the large health effects of social isolation and loneliness (see Chapters 2 and 3), studies with longer follow-up periods that can demonstrate sustained effects during an intervention (and even months after an intervention) will be more useful. (See Chapter 9 for more on interventions.)
Overall Measure Quality
As is discussed in more detail in Chapter 7, concerns exist regarding the quality and relevance of current tools, and particularly as to whether the tools developed decades ago can fully capture the expectations and values of older adults today. This is likely to be especially relevant for measures of social isolation as modes of interaction have changed significantly in recent years and decades. For example, questions about living alone or participation in religious activities may not fully reflect preferences in today's society. Also, alternative modes of communication to the “telephone” (e.g., social media, instant messaging, video conferencing) may not be fully captured in these measures. On the other hand, measures of loneliness may not be influenced by social changes because responses are subjective to the current context. While current tools may have limitations, the committee asserts that the use of existing validated tools is important to advance the role of the health care system in addressing social isolation and loneliness (see Chapter 7). Furthermore, researchers need to strive toward a goal of measure development and evaluation in this space to ensure that the available tools for social isolation and loneliness can fully capture the experience of today's older adults.
IDENTIFICATION OF INDIVIDUALS AND POPULATIONS AT RISK
In addition to the measures described above, information technology offers the possibility of detecting or predicting patterns of social isolation and loneliness in older adults. This can be done without using specific tools or measurement scales. For instance, data mining can be used to assess or predict patterns of social isolation and loneliness (Austin et al., 2016); to this end various sources of data have been explored, including passive monitoring sensors, wearables, and programs to track social media. However, the development of algorithms to accurately predict social isolation or loneliness has proved challenging because of the lack of a specific outcome measure that is available at a large scale in EHRs or other sources of data (e.g., hospital readmission or death). One benefit may be that for both research and clinical settings this could reduce the burden on individuals, providers, and researchers in terms of the time needed to answer certain questionnaires.
Prediction
Multiple approaches exist for identifying individuals at risk for social isolation or loneliness, including self-referral, community referral, formal assessments, and predictive analytics. In health care, predictive analytics have been widely used to enable better decision making and to support preventive care (Wang et al., 2018). For example, one hospital system used predictive analytics on data from medical sensors to predict patients' movements and monitor their actions throughout their hospital stay, allowing the health system to provide services more efficiently, optimize operations, and reduce medical risk (Wang et al., 2018). The section on predictive analytics in Chapter 7 describes opportunities for health care systems to develop predictive strategies based on the vast datasets within health information systems.
Identifying Social Isolation and Loneliness at the Individual Level
In clinical research, machine learning1 technologies have been used to analyze social media data to predict depression (Eichstaedt et al., 2018), suicidality (Braithwaite et al., 2016), and posttraumatic stress disorder (Coppersmith et al., 2014). Natural language processing (NLP)2 technologies and machine learning are also being used to examine EHR data to identify individuals at risk for a variety of behavioral issues, including suicidality (Walsh et al., 2018). Given the lack of standardization for assessing social isolation and loneliness in clinical documentation, researchers have used NLP techniques to identify mentions of social isolation in clinical notes. In one study, investigators used validated scales and expert opinion to develop a lexicon representing concepts related to social isolation (Zhu et al., 2019). Among the terms in the algorithm were “lack companionship,” “isolated,” and “feel left out.” The algorithm was tested in a dataset of clinical notes from 1,057 prostate cancer patients, and the performance was evaluated using chart review as a gold standard. The algorithm correctly identified 36 cases of social isolation, and an additional 4 cases were determined to be false positives. Reviewers found one false negative in a sampling of the algorithm's negative cases. In another study, NLP was used to analyze transcripts of psychotherapy sessions to identify symptom and diagnostic information using not only language but also interactional variables such as turn-taking in the conversation between provider and patient (Gaut et al., 2017).
Some health care organizations use fields in the EHR to document “living alone” as a proxy for social isolation. Given the many life circumstances implied by living alone (e.g., being independent), these data alone are of little use in identifying patients at risk for negative outcomes related to social isolation and loneliness (LaWall et al., 2019). (See Chapter 7 for more on the use of information technology in clinical settings.)
Population-Level Identification and Targeting
In general, technologies that analyze large public and private datasets are being used both in and out of clinical settings to identify target groups of individuals according to behavioral characteristics, which has raised various ethical and social concerns (Eubanks, 2018; Zuboff, 2019). For example, in industry, video games and other Web-based mobile interventions are designed for targeted appeal and subsequent loyalty. However, there is a dearth of research on how individuals experiencing social isolation and loneliness are targeted by such industry interventions. That is, the outcome of the product design could encourage increased use of their products, leading to increased social isolation or loneliness.
Key ethical issues related to the use of large datasets to predict social isolation and loneliness include the violation of privacy and the creation of new, harmful stereotypes, though various other ethical concerns may also arise, depending on the way in which identification is conducted and used for targeting.
Implications and Ethical Concerns
While the use of data to identify, predict, and potentially mitigate social isolation and loneliness is considered promising by many, some concerns exist about the ways in which data might be used and the ways in which assessment may be ethically problematic. This section will consider various ethical issues concerning the measurement and monitoring of social isolation and loneliness. The ethical considerations described here pertain not only to research studies examining social isolation and loneliness but also to clinical practice and the implementation of interventions or strategies by health care organizations. This list is not comprehensive, and serious thought should be given to other potential ethical concerns that may arise due to the nature of a specific assessment tool or monitoring program.
Informed Consent
Informed consent is a requisite for all clinical care, beginning with the assessment of the problem and continuing through any provided treatment or intervention (whether social or medical in nature). Adequate informed consent entails ensuring that a patient understands the facts of the medical issue at hand, the implications of choosing to treat or forgo treatment, and the potential risks and benefits of the assessment or treatment. Informed consent enables older adults to weigh the pros and cons of an intervention. Informed consent is ongoing and provides an opportunity for older adults to have a dialogue with their practitioners about the course of their treatment (Price et al., 2012; Reid et al., 2018). Individuals are considered capable of providing consent only if they have adequate reasoning faculties and are in possession of all the relevant facts. It is possible that older adults with cognitive limitations such as dementia or memory loss may not be able to give informed consent, and in this case individuals with proxy status or legally authorized representatives (such as family members or spouses) may be asked to provide consent. In situations when a person is not fully able to make a decision but still not quite at the surrogate decision-making stage, a joint decision-making model can be invoked in which trusted family members or legally authorized representatives or both assist the older adult with the decision-making process, taking into consideration the person's past and present wishes (Galambos et al., 2018; Nuffield Council on Bioethics, 2009). It is important to note, however, that for assessments in health care settings (e.g., questions about smoking and other health care risks), while patients have the option to not answer questions, clinicians would rarely provide informed consent for each question asked.
Autonomy
In addition to issues of informed consent, a variety of bioethical principles relevant to the use of data for the assessment of social isolation or loneliness also need to be considered. Broadly speaking, the concept of autonomy stipulates that individuals have the right to make their own decisions about what entails “living a good life.” In medicine specifically, patients are understood to have the right to make decisions regarding what medical care or procedures they accept and the circumstances surrounding that care. Importantly, autonomous decision making is free of outside coercion. In order for a patient to make an autonomous decision, he or she must understand the potential risks and benefits of a given procedure and the likelihood of the procedure's success. Notably, it is possible for individuals to lose the ability to make autonomous decisions if they are suffering from mental or cognitive deficits and are unable to fully grasp the implications of a medical procedure or treatment.
Regarding social isolation and loneliness, as social relationships typically fall outside of the realm of medical care, some individuals may object to the health care system assessing—or “judging”—their relative levels of social isolation or loneliness. For example, respecting an older adult's autonomy may mean respecting a person's choice to live alone or to be alone. In these situations, periodic assessment and monitoring of older adults' social isolation and loneliness may be the approach that is required to respect their living preferences. (See more in Chapter 7.)
Privacy
With the increasing digitalization of information and health records, concerns about medical privacy have become more widespread. Respecting individual privacy entails ensuring that health information is captured and stored in a protected way and that protected health information is not shared without an individual's specific approval. The Privacy Rule, part of the U.S. federal law known as the Health Insurance Portability and Accountability Act (HIPAA), gives individuals rights over their own protected health information and sets rules and limits on who can access and share or receive that information when it is documented in electronic format. Health care staff are required to comply with such regulations when sharing any information in the health record, including any assessment information on social isolation and loneliness. It is also important that older adults be given the opportunity to designate how and with whom their health information should be shared. Once an understanding is reached about information sharing, the necessary permissions and releases should be documented. A periodic review of these sharing preferences should occur and any changes be noted formally.
Individuals experiencing isolation or loneliness may feel embarrassed or uncomfortable acknowledging their isolation to others, and it is possible that individuals might experience stigma after being labeled as “lonely” by a health care provider. Because of this potential harm from stigma, it is extremely important that information about isolation captured in the health record be treated as protected health information. An older adult should be provided the opportunity to determine how and with whom assessment information on social isolation and loneliness is shared. Initial and periodic clarification as to whom older adults select to have access to this information will help ensure that information is shared within the parameters set by the older adult.
Additionally, the way in which patients' data are used in research also falls under HIPAA and other related human-subjects research protections.
Beneficence and Non-Maleficence
The principles of beneficence and non-maleficence are related, though they are not the same. Beneficence requires that medical procedures be provided with the intent of doing good for the patient and serving in the patient's best interest, and it demands that health care providers continuously maintain their own skills and knowledge in order to provide the best treatment for their patients. Non-maleficence refers to making sure that medical procedures and treatments do not harm patients nor others in society (Beauchamp and Childress, 2013). Both are core moral principles that are incorporated into health professions' codes of conduct. When applied in the area of social isolation and loneliness, these principles require knowledge of the adverse risks and benefits associated with the intervention under consideration and with alternative courses of action as well as the risks and benefits of not intervening at all (Bantry-White, 2018; Beauchamp and Childress, 2013; Reid et al., 2018).
Additionally, concerns about the exploitation of individuals suffering from isolation or loneliness must be taken into account when considering possible assessment tools or interventions. It is possible, for instance, that individuals who are isolated are more susceptible to exploitation or abuse (physical, emotional, or financial). It is also possible that certain technologies might exploit lonely or isolated elders, such as robo-calls, phishing emails, or other financial scams. And it is possible that technologies used to identify individuals at risk of isolation or loneliness could be exploited for commercial marketing and monetary gain.
Populations at Risk
Certain populations, such as low-income, minority, and lesbian, gay, bisexual, and transgender elders, may be disproportionately affected by the social determinants of health. It is critical to have assessment tools for social isolation and loneliness that do not further exacerbate inequalities between minority or at-risk groups and the general population. One way to ensure ethical assessment is to implement assessments across entire practice populations, rather than targeting specific subgroups. Focusing on groups of a particular social status may have negative implications for trust in the health care system and could very well be unethical in certain circumstances. In addition, assessing populations more broadly allows for a focus on primary prevention.
As mentioned in the above section on privacy, assessment might involve stigmatizing the conditions of social isolation and loneliness, which is an undesirable outcome for various reasons: individuals may avoid seeking help for social isolation or loneliness if they are embarrassed or ashamed, leading to negative health consequences, and the implementation of assessment for social isolation or loneliness might itself bring about the creation of new, harmful stereotypes. For example, public health campaigns have been successful in reducing the prevalence of smoking in the American population. However, much of this success has to do with shaming individuals who smoke cigarettes. Shaming individuals for health issues that may be out of their control (like social isolation and loneliness) could be extremely harmful.
FINDINGS AND CONCLUSIONS
- The concepts of social isolation and loneliness have been defined in different ways, which has led to variability in how these concepts are measured.
- Picking assessment tools that match the research question or intervention is critical.
- The length of time for follow-up in an intervention is an important part of determining the clinical utility of an outcome.
- The use of standardized and validated measurement tools will help build a more robust evidence base in which results are comparable to other studies.
- Measures developed decades ago may not appropriately account for newer modes of interaction and communication (e.g., social media, instant messaging, video conferencing).
- More effort is needed to update existing measures as well as to develop better instruments for assessing social isolation and loneliness that can fully capture the experience of today's older adults.
- Technological advances such as machine learning, EHRs, and predictive analytics show promise as potential ways to identify social isolation and loneliness.
- A variety of ethical concerns are associated with measuring and assessing an individual's levels of social isolation and loneliness. As further work is done to identify individuals at risk of isolation or loneliness and to identify promising treatments for these conditions, serious consideration should be given to ethical issues that might arise from the use of a specific assessment tool or intervention technique.
Footnotes
- 1
Machine learning, a subset of artificial intelligence, refers to the “algorithms, tools, and techniques that give computers the ability to learn from experience and data” (CTA, 2018, p. 21).
- 2
Natural language processing is “technology that produces conversational text or speech understandable by humans” (CTA, 2018, p. 21).
- Assessment of Social Isolation and Loneliness in Research - Social Isolation and...Assessment of Social Isolation and Loneliness in Research - Social Isolation and Loneliness in Older Adults
- Collecting and Analyzing Data: Doing and Thinking - Clinical MethodsCollecting and Analyzing Data: Doing and Thinking - Clinical Methods
- MIR141 microRNA 141 [Gallus gallus]MIR141 microRNA 141 [Gallus gallus]Gene ID:777853Gene
Your browsing activity is empty.
Activity recording is turned off.
See more...