NCBI Bookshelf. A service of the National Library of Medicine, National Institutes of Health.
National Academies of Sciences, Engineering, and Medicine; Division on Engineering and Physical Sciences; Health and Medicine Division; Policy and Global Affairs; Division on Earth and Life Studies; Forum on Cyber Resilience; Board on Health Sciences Policy; Board on Science, Technology, and Economic Policy; Board on Agriculture and Natural Resources; Board on Life Sciences; Committee on Safeguarding the Bioeconomy: Finding Strategies for Understanding, Evaluating, and Protecting the Bioeconomy While Sustaining Innovation and Growth. Safeguarding the Bioeconomy. Washington (DC): National Academies Press (US); 2020 Jan 14.

Summary of Key Findings
- The U.S. bioeconomy relies on a complex and evolving ecosystem that extends from research and development through manufacturing, and it also encompasses related services.
- The U.S. bioeconomy draws on multiple resources and encompasses multiple applications. As a result, all regions of the United States have strengths that contribute to the bioeconomy.
- The impacts within the U.S. bioeconomy of investments that support fundamental research and the development of enabling technologies are nonlinear. These impacts cannot necessarily be predicted when initial investments are made.
- The bioeconomy is an increasingly data-driven enterprise. The development of diagnostics, drugs, synthetic biology products, and more benefits from access to information resources.
- A number of policies and practices support the U.S. bioeconomy, directed at achieving (1) a predictable and responsive regulatory environment; (2) a skilled workforce; (3) investments at multiple stages, from research to commercialization, and strategies for taking precompetitive interests of industry into account; and (4) the targeted use of incentives and market pull.
This chapter begins by reviewing innovation in the bioeconomy from research to commercial application, describing the overall U.S. system in which life sciences research is conducted and translated into innovative products and services. It then details characteristics of the surrounding ecosystems that support the U.S. bioeconomy—including regulatory and intellectual property regimes, investment sources, and workforce policies and structures. The third section of the chapter explores a number of trends and changes that are shaping and altering how the bioeconomy functions and looks ahead to the need to keep abreast of emerging trends and to undertake strategic planning. This is followed by discussion of one tool for strategic planning in support of the U.S. bioeconomy: the use of the Technology Readiness Level (TRL) scale. Throughout the chapter, selected examples of developments that are helping to power the life sciences innovation pipeline are highlighted to showcase key messages. The chapter ends with the committee’s conclusions with respect to discovery and innovation in the U.S. bioeconomy.
INNOVATION IN THE BIOECONOMY: FROM RESEARCH TO APPLICATION
As discussed in Chapter 3 (see Box 3-1) and defined by the Organisation for Economic Co-operation and Development (OECD), innovation is “a new or improved product or process (or combination thereof) that differs significantly from the unit’s previous products or processes and that has been made available to potential users (product) or brought into use by the unit (process)” (OECD/Eurostat, 2018, p. 20). A similar definition is used by the National Science Foundation (NSF) in Science and Engineering Indicators 2018 (NSB and NSF, 2018). As the pace of scientific discovery has accelerated and discoveries have evolved into practical applications for commercial products and services, the United States has realized the benefits of a national innovation ecosystem capable of transforming research discoveries into economic and societal benefits. This ecosystem is essential to the continued realization of such benefits to the United States. For the bioeconomy, the system that enables this innovation is built on fundamental advances in basic biological knowledge in concert with the continued creation and maturation of enabling platform technologies, which together are translated to meaningful application and deployed commercially (see Figure 5-1). This section reviews the role of scientific discovery and basic research, the contributions of enabling technologies, and the general process of translation and commercialization.

FIGURE 5-1
Advances in fundamental biological knowledge and in a number of enabling technologies are creating commercial opportunities with application to many sectors of the bioeconomy. An idea moves from the basic research and proof-of-concept stages (left), through (more...)
The Role of Scientific Discovery and Basic Research
For decades, the United States has led the world in investment and activity in basic life sciences research (see Chapter 4). Supported by federal funding for world-class universities, research nonprofits, and federal research laboratories, the nation’s life sciences research enterprise has helped create the foundation for discovery that is required to realize benefits across a variety of applications in health, agriculture, environment, energy, and industrial biotechnology (see Chapter 3 for detail on data and measurement strategies for capturing the scope of the U.S. bioeconomy). While it continues to be impossible to predict the nature and timing of the next significant basic research breakthrough, it is clear that the pace of knowledge accumulation is accelerating (IAC, 2014). For example, as of 2015, the amount of DNA sequence data produced was doubling every 7 months (Stephens et al., 2015).
Early-stage discovery often derives from public investment in research and in the training of scientists to seed the next-generation workforce. Although the value and importance of investment in basic scientific discovery have been known since early in the country’s history, Vannevar Bush articulated the importance of scientific research to national security and economic well-being in the letter of transmittal to President Roosevelt of his 1945 report Science, the Endless Frontier: A Report to the President: “Scientific progress is one essential key to our security as a nation, to our better health, to more jobs, to a higher standard of living, and to our cultural progress” (Bush, 1945, p. 2). One of the most exciting aspects of scientific research is that the process often begins with attempts to explore the natural world with the goal of understanding some of the principles that govern it. As scientific knowledge is gained, however, opportunities are created to use that knowledge for a variety of applications—to solve problems that previously could not have been solved; to create technologies that previously had not been imagined; and to create businesses in areas that previously had not been developed. In some cases, these benefits are reaped quickly, while in other cases, the benefits of the practical application of scientific knowledge take years or decades to be realized. Nonetheless, a signature feature of basic scientific research is that discoveries can be based on sometimes indirect, unpredictable, serendipitous events. In many such cases, the research has led to significant economic outcomes (see Box 5-1).
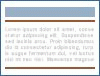
BOX 5-1
Important Outcomes Resulting from Fundamental Research.
Progress in biological discovery has also been rooted in the culture of science and reliance on fundamental principles that help advance the state of knowledge. These principles include respect for the integrity of knowledge, collegiality, honesty, objectivity, and openness (NRC, 1992), as well as recognition of the importance of adhering to rigorous scientific methods. The presentation of research results at conferences and the publication of results in the peer-reviewed literature have also been important mechanisms for the diffusion of information and methods, as well as advancement in the field. The increasing use of prepublication servers, such as BioRxiv, and rapid communication on other Internet platforms are now providing speedier access to information in an increasingly global context.
Biological research has benefited greatly from the open sharing of information, particularly in the genomics era. Such resources as GenBank, supported by the National Institutes of Health (NIH) (and coordinated with international partners such as the DNA DataBank of Japan and the European Nucleotide Archive), provide free access to hundreds of millions of DNA sequences.1 Physical repositories such as Addgene offer access to plasmids developed by researchers for dissemination to the broader life sciences community.2 Open-source software enables bioinformaticians to mix and match compatible tools in order to customize the analysis of biological data, particularly next-generation sequencing data (Carrico et al., 2019). Data scientists in all disciplines have benefited from the development and sharing of data and software for statistical analysis and machine learning; with the advent of “-omics” technologies, such as genomics and metabolomics, these tools are increasingly applied to biology. A previous report of the National Academies titled Open Science by Design notes that “openly sharing articles, code, and data in all phases of the research process is beneficial to the research community, to the broader scientific establishment, to policy makers, and to the public at large” (NASEM, 2018d, p. 107).
America’s life science research base is also amplified, and the pace of discovery is augmented, by efforts undertaken in other research-intensive countries, including China, Germany, Switzerland, and the United Kingdom, among others. The increasing extent to which scientific collaborations are global will shape progress in scientific discovery and translation in ways that are positive for the United States, as well as in ways that may pose challenges (see Chapter 7).
The Contribution of Enabling Technologies
In the life science research and innovation enterprise, basic discoveries are often accelerated by enabling technologies. Some enabling technologies (such as next-generation DNA sequencing technology or advanced genome-editing tools) are derived directly from the life sciences community, while others (such as automated liquid handling or machine learning algorithms for data analysis and inference) are derived from parallel communities and can also serve to benefit life sciences research and innovation. In an academic setting, this is manifested in the rise of core facilities that purchase, operate, and maintain specialized equipment, such as DNA sequencers, confocal microscopes, or mass spectrometers, that would otherwise be too costly for individual laboratories to purchase (Hockberger et al., 2018). High-performance computing services are also becoming available as core facilities (Courneya and Mayo, 2018). In many cases, these enabling technologies can drive the development of for-profit or not-for-profit businesses. Contract research laboratories see continued growth and can provide commodity as well as specialized services for customers (Nature Biotechnology, 2014).
Translation and Commercialization
As a given scientific community continues to mature in its understanding of basic research discoveries, opportunities arise that permit practical application of those discoveries. In the life sciences, multiple application areas including human health, agriculture, energy, industrial biotechnology, and the environment are relevant to the bioeconomy. In some cases, diverse applications can arise in the context of a particular biological discovery. For example, understanding of how cells grow can impact understanding of cancer and cancer treatments in human cells, of crop yields in plants, of assisted reproduction techniques in cattle, of remediation of environmental contaminants, or of certain types of bacteria as sustainable energy sources. It is worth noting, however, that the timescale associated with meaningful translation of basic life science discoveries into practical application can differ based on the type of organism and application area.
In the process of translation of a discovery to commercialization, a middle stage of activity occurs, often called the “valley of death,” that is considered high-risk applied research. Frequently, this research is considered too applied by funders of the basic research classically pursued by universities and is too high risk to receive attention from industry for its commercial application. Strategies for reducing this gap, including public–private partnerships and venture capital investment, can be useful in stimulating innovation. In the later stages of development pathways, as the science and technology that underlie a potential new product or service matures, the for-profit sector often drives the advances, motivated by commercial opportunities.
THE SURROUNDING ECOSYSTEM SUPPORTING THE U.S. BIOECONOMY
The U.S. bioeconomy depends on a web of federal agencies that support life sciences research. Federal and private investments catalyze and support the bioeconomy from basic science to commercialization. They include investments for intellectual property (IP) protections and regulatory frameworks that can capture returns on innovation while protecting the health and safety of people and the environment. Investments in the bioeconomy also serve to develop the necessary skilled workforce. Efforts directed to scientific and technical standards development and the use of market incentives such as government purchasing programs also contribute. This section introduces a variety of U.S. agencies, policies, and mechanisms that help in realizing the potential of scientific and technical advances and that function to support the U.S. bioeconomy.
Federal Agencies Addressing Aspects of Life Sciences Research
At least 25 agencies and departments support research and development (R&D) in areas of the life sciences (see Box 5-2, which lists the agencies and departments involved in preparations for the 2012 National Bioeconomy Blueprint). A number of additional departments have roles related to the bioeconomy and could be added to this list, including the Biomedical Advanced Research and Development Authority, U.S. Centers for Medicare & Medicaid Services, U.S. Army Research Office, U.S. Air Force Office of Scientific Research, U.S. Army Combat Capabilities Development Command, U.S. Department of Justice, and National Park Service. These agencies play key roles in supporting both basic research and discovery and translational activities within the scope of their missions, and many also support R&D in converging areas of science and technology that contribute to the bioeconomy. Given the diversity of federal stakeholders, no single agency has a clear lead in advancing U.S. bioeconomy goals. Sustaining the U.S. life sciences enterprise and advancing the U.S. bioeconomy will thus require the engagement of multiple agencies and departments across the government.
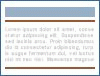
BOX 5-2
Examples of Federal Departments and Agencies That Support Biological Research.
Investments That Catalyze and Support the Bioeconomy
Government Support of Research and Development
Government R&D investments include fundamental research in biological sciences and enabling technologies, as well as investments targeted more directly in areas of biotechnology that can meet specific needs of the bioeconomy and that support specific missions of government agencies, such as the U.S. Department of Defense. Advances in the bioeconomy can also be supported by shared use of unique government R&D facilities and collaboration between government researchers and private-sector entities through the use of cooperative research and development agreements, as well as the encouragement of industrial consortia by which private firms work together to develop precompetitive technologies and supporting data. In addition, programs specifically targeted to promote small businesses’ development of technologies with the potential for commercialization, such as the Small Business Innovation Research and the Small Business Technology Transfer programs, can facilitate the transition from research to product by reducing barriers and accelerating translation (Link and Morrison, 2019; Narayanan and Weingarten, 2018).
Private Investments That Support the Bioeconomy, Including Venture Capital and Public–Private Partnerships
Support for early-stage research is essential for the discovery of new knowledge and the development of a trained talent pool, and as a catalyst for opportunities for innovation. This support, primarily from government sources and sometimes by private foundations, can establish proof of concept for new ideas and technologies. However, the endpoint of this basic research phase is typically too early in the maturation of a technology for it to move into the marketplace as a new product or service. At least two sources of investment support businesses seeking to mature technologies into commercial products and processes: the venture capital community and public–private partnerships.
The venture capital community provides critical funding to help early-stage businesses advance and develop their technologies into products. By providing cash, typically in exchange for equity and other considerations, the venture capital community can provide significant financial resources that help companies cross the valley of death, creating significant value for the investors (which often means relying on a few large payoffs to cover losses) while bringing new products and services to the market (Bristow et al., 2018; see also the assessment of metrics of U.S. leadership in Chapter 4). The world-leading entrepreneurial ecosystem in the United States contributes to economic growth (WEF, 2018) and is one of key pillars of the U.S. bioeconomy.
A large number of public–private partnerships result from efforts to bring stakeholders from the federal government together to work collaboratively and interactively with small, medium, and large companies; academia; and other nonprofits to help bring new technologies to the market. One example is the Manufacturing USA program,3 a collection of 14 manufacturing institutes, each a public–private partnership funded jointly by government, industry, and nonprofits that work to develop and advance manufacturing-related technologies. Several of these institutes, including those working on biofabrication and regenerative medicine, biopharmaceuticals, robotics, and digital technologies, connect directly to the bioeconomy.4 The program also brings together a broad cross-section of relevant government organizations, including the U.S. Departments of Agriculture, Commerce, Defense, Education, Energy, Health and Human Services, and Labor; the National Aeronautics and Space Administration (NASA); and NSF.
Another relevant class of public–private partnerships is created through the Foundation for the National Institutes of Health (FNIH). FNIH, established by Congress in 1990,5 serves to accelerate biomedical research by forging collaborations among NIH and public and private institutions. As a complement to efforts focused on developing a given technology past the valley of death, FNIH activities typically focus on large-scale programs for which broad-based expertise and engagement can create new precompetitive knowledge. For example, the Accelerating Medicines Partnership program has provided new technologies to speed up drug discovery for rheumatoid arthritis, lupus, diabetes, Alzheimer’s disease, and Parkinson’s disease (Dolgin, 2019).
Private investment has an important role as a driving force in the bioeconomy, and the value of public–private partnerships that can seed new innovation or bring stakeholders together to address the valley of death of technologies is clear. As these models expand around the world, it will be important for the United States to continue to nurture and support such efforts, as well as to identify new means and opportunities for stimulating the bioeconomy.
Support for Intellectual Property Rights
Biotechnology is one of the most research-intensive industries, and biotechnology companies must bear significant costs in bringing new products to market. Although extensive research time is needed for biotechnology companies to develop new products and processes, copying of those products and processes (by potential competitors, for example) is relatively inexpensive. For this reason, biotechnology companies often seek to protect the results of their research by securing IP rights that can provide the exclusivity needed to protect their investments.
Of the many forms of IP, patents and trade secrets are the two most commonly used by biotechnology companies to protect their innovations (Sherkow, 2016; see Box 7-2 in Chapter 7). Patents allow companies to prevent competitors from using their innovations, but offer only a limited period of exclusivity (typically 20 years from the date of filing), after which the technology described in the patent enters the public domain (Title 35 of the U.S. Code—Patents; NRC, 2004). Trade secrets, by comparison, can last indefinitely, but will not prevent a competitor from reverse engineering or independently discovering an innovation. Both mechanisms can be used strategically to help companies maintain their competitive advantage, and thereby contribute to the companies’ economic success and to the bioeconomy as a whole.
Recognizing the importance of the exclusivity provided by patents, Congress passed the Bayh–Dole Act of 1980, which vested patent rights to technologies and inventions developed with federal funding in their nongovernmental developers.6 One purpose of this legislation was to encourage universities and nonprofit research institutions to patent and license their innovations as a means of motivating private-sector companies to make further investments in commercializing innovations that would not be viable without exclusive rights. There has since been a dramatic increase in university patenting and licensing activity, although the effectiveness of the Bayh–Dole Act in encouraging technology transfer remains a matter of debate (NRC, 2011; NSB and NSF, 2018). Of note, a recent study by the National Institute of Standards and Technology (NIST) identified a number of strategies that would improve federal technology transfer policies and practices without requiring legislative changes to the Bayh–Dole Act (NIST, 2019). Although global investment in biotechnology remains strong,7,8 investment in the life sciences has been negatively affected by the increased uncertainty over the patent eligibility of biotechnology innovations. (See the discussion of the risks posed by an ineffective or inefficient IP environment in Chapter 7.) As a result, biotechnology companies are exploring mechanisms outside the U.S. patent system that could support their investments in R&D of their innovations.
As an example, at least one company has sought copyright protection for nucleotide sequences (Holman, 2017). Copyright protects the expression of ideas (or, more formally, works of authorship fixed in a tangible medium of expression)9 and can be used to protect software and data analytic tools that contribute to research and commercial translation. In the United States, however, there is currently no legislative or judicial support for copyright protection of biological sequences, and attempts to register nucleotide sequences with the U.S. Copyright Office have failed (Burk, 2018). If extended to biological sequences, copyright protection could enable open-source licensing models, but copyright would offer only shallow use protections for biological innovations while being difficult to enforce (Torrance and Kahl, 2014).
Regulatory exclusivity is another strategy enabling companies to capture benefits from innovation, offering a limited term of exclusivity in exchange for meeting regulatory requirements. Regulatory exclusivity is available for drugs, both on- and off-patent (Eisenberg, 2012), but is untested in the biotechnology sector outside of generic and orphan drugs and would likely require new legislation to create or extend to other areas of bioeconomy commercialization.
Investing in the Public Domain
Underpinning much of the U.S. bioeconomy are technologies available in the public domain. In the pre-Bayh–Dole era, most academic scientists did not seek patent protection and instead placed their innovations directly in the public domain through publications and presentations at scientific meetings in accordance with the norms of the academic research community. Like all of these unpatented technologies, many of the technologies developed by academic researchers in the nearly 40 years since the Bayh–Dole Act came into effect are in the public domain, either because researchers have pursued a public domain strategy for the dissemination of their innovations or because the period of patent protection has ended.
The patent system is often associated with exclusivity and monopoly, but in fact is one of the best mechanisms for building the public domain. The quid pro quo of the patent system is to provide a limited period of exclusivity in exchange for disclosure of the innovation to the public. Once the period of exclusivity has expired, the innovation enters the public domain. Examples of foundational biotechnologies that have entered the public domain via the patent system include the recombinant DNA technology developed by Stanley Cohen and Herbert Boyer, the polymerase chain reaction developed by Kary Mullis, and the use of green fluorescent protein for monitoring gene expression developed by Martin Chalfie. Beyond these early patented biotechnologies, it is not uncommon for researchers and others wishing to further develop or use patented technologies to simply await the expiration of the patents. As an example, researchers at the University of Arkansas developed glyphosate-tolerant varieties of soybean after Monsanto’s patent on the first generation of Roundup Ready technology expired in March 2015 (Chen et al., 2016). These varieties are available without technology fees, and farmers can save seed for planting in subsequent years. Whereas the dawn of the biotechnology revolution took place in the early 1980s, the U.S. bioeconomy has been benefiting from the era of generic biotechnology, thanks to the patent system, since the early 2000s.
Growth of biotechnology-relevant innovations in the public domain also occurs through the creation of prior art that precludes subsequent patenting. Prior art is information that has been disclosed to the public, before the earliest priority date of a patent application, that would preclude the granting of a patent for lack of novelty10 or nonobviousness.11 Despite ongoing efforts by the U.S. Patent and Trademark Office (USPTO) to improve patent quality,12 it remains challenging to get the best prior art before patent examiners during the examination process. This is particularly true for prior art published in the nonpatent literature (e.g., scientific journals, conference proceedings).13 For this reason, those wishing to contribute technology to the public domain may opt to file, and then intentionally abandon, a patent application that provides an enabling disclosure of the technology they wish to contribute. This file- and-abandon strategy replaces the pre-Leahy–Smith America Invents Act procedure known as Statutory Invention Registration14 that companies typically used to place technology in the public domain as a means of ensuring that their own use of the technology would not be jeopardized by competitor-owned patents.
In building the set of relevant innovations in the public domain, it is important to recognize that patents are not the only type of IP protection that may limit, albeit temporarily, the use of a technology. Material transfer agreements, or MTAs, are commonly used in the life sciences to govern the use of research materials such as plasmids, antibodies, cell lines, and more. Although for most research materials, MTAs need do little more than establish provenance, the high transaction costs of negotiating MTAs and the risk-aversion tendency to include unnecessarily restrictive terms have been well documented (Bubela et al., 2015; Nielsen et al., 2018; Walsh et al., 2005). In the 1990s, NIH developed the Uniform Biological Material Transfer Agreement (UBMTA) and the Simple Letter Agreement, which are now maintained as standards by the Association of University Technology Managers. While these standard MTAs have done much to streamline the MTA negotiation process, they include terms that limit the use and redistribution of materials and hence are not well suited to research materials intended for dissemination within the public domain. Recently, the OpenMTA was introduced as a standard template that would enable provenance tracking and was optimized for dissemination of unpatented materials through the public domain (Kahl et al., 2018). Based on the UBMTA template but with modifications to allow commercial use and redistribution, the OpenMTA has steadily been gaining momentum, with more than 50 signatories from academic research institutions, biotechnology companies, and community labs.15
The public domain is, in essence, a form of property that is owned by, and maintained for the benefit of, the public (Ochoa, 2002). With the continued growth of the U.S. bioeconomy, it will be important to ensure that scientists and engineers and the companies and research institutions that employ them are able to effectively leverage and build on technologies in the public domain. USPTO already provides a number of resources and training opportunities to assist inventors, entrepreneurs, and other stakeholders in better understanding and utilizing the patent system.16 In addition, nonprofit organizations such as the Public Intellectual Property Resource for Agriculture (Chi-Ham et al., 2012) and Cambia (Jefferson et al., 2018) have made available to the public a number of tools and educational materials to aid in the development of strategies that optimize the creation and use of proprietary and public-domain technologies.
The U.S. Coordinated Framework for Regulation of Biotechnology
Clear regulatory paths for bioeconomy products to enter the market in an efficient, timely, and safe manner help reduce uncertainty for new products and contribute to driving continued innovation within the bioeconomy. The U.S. government regulates many of the products, services, and production processes associated with the bioeconomy because they have the potential to impact public health, safety, welfare, or the environment. In developing the Coordinated Framework for the Regulation of Biotechnology of 1986, the U.S. government focused on characteristics of the product itself, rather than exclusively on the process by which the product was created: “The manufacture by the newer technologies [i.e., genetic engineering] of food, the development of new drugs, medical devices, biologics for humans and animals, and pesticides, will be reviewed by FDA [U.S. Food and Drug Administration], USDA [U.S. Department of Agriculture] and EPA [U.S. Environmental Protection Agency] in essentially the same manner for safety and efficacy as products obtained by other techniques” (OSTP, 1986, p. 23304). U.S. regulations are triggered by the nature of the potential risks to be mitigated—such as those posed by dangerous medical products and devices, impure or adulterated food, or environmental contamination—and not solely because the production process may have employed genetic engineering techniques. Moreover, these regulations do not aspire to eliminate all risk. As stated in the 2017 Update to the Coordinated Framework, which reaffirmed language in a 1992 update addressing the introduction of biotechnology products into the environment, “oversight is to be applied only where the risk posed by the introduction is unreasonable” (EOP, 2017, p. 4). A 2011 Executive Order further clarifies the interests of the U.S. government in efficient, effective, and innovation-conducive regulation (EOP, 2011).
According to the U.S. government, the current regulatory approach to biotechnology products effectively protects public health and the environment (EOP, 2017). However, the U.S. government also acknowledges that science and technology are moving rapidly, and that it can be difficult to determine which regulatory process is appropriate to which type of product. As a consequence, regulatory agency decision making can under some circumstances be delayed, leading to a perception that the U.S. regulatory system is not agile. For example, from 1988 to 1997, the mean approval time for genetically engineered crops was determined to be 1,321 days, and from 1998 to 2015, the mean approval time was 2,467 days (Smart et al., 2016).
The 2017 Update to the Coordinated Framework clarifies which agencies have responsibility for which types of biotech products (EOP, 2017, p. 1). Three federal agencies, acting under 11 statutes, have primary responsibility for regulating biotechnology products:
- The U.S. Food and Drug Administration (FDA) is responsible for the safety and proper labeling of human and animal foods and of cosmetics, and for the safety and efficacy of human and animal drugs and human medical devices. FDA also considers that its animal drug authorities govern the genetic engineering of animals, even apart from its effect on human foods.
- The U.S. Environmental Protection Agency is responsible for substances (including products of biotechnology) that have insecticidal, fungicidal, rodenticidal, or other toxic properties. In particular, it exercises broad authority over new chemicals in commerce, which it defines as including certain forms of genetically engineered organisms. This regulatory space has the potential for broad impact on the bioeconomy.17
- The U.S. Department of Agriculture (USDA) is responsible for plant pest and disease risks, noxious weed risks, and the safety and appropriate labeling of certain foods (i.e., meat, poultry, and egg products). USDA regulates plants genetically engineered through the use of bacteria the agency considers to be plant pests, but it does not currently assert the authority to regulate plant biotechnologies, such as genome editing, that do not use such bacteria. However, other authorities, which USDA has not used in the past to regulate biotechnology, might apply.
Table 5-1 summarizes a number of these agencies’ statutes and protection goals.
TABLE 5-1
Statutes and Protection Goals Related to the U.S. Environmental Protection Agency (EPA), the U.S. Food and Drug Administration (FDA), and the U.S. Department of Agriculture (USDA) for the Regulation of Biotechnology Products.
A 2015 White House memorandum from the director of the Office of Science and Technology Policy on “Modernizing the Regulatory System for Biotechnology Products” (OSTP, 2015) calls for a study of “the future landscape of biotechnology products” to identify potential new risks and risk assessment frameworks in order to help regulatory agencies anticipate new types of products that might not be well matched to their existing regulatory processes and risk assessment capabilities. This study was conducted by the National Academies, which in 2017 released the report Preparing for Future Products of Biotechnology (Future Products) (NASEM, 2017b). The committee that developed the Future Products report concluded that the U.S. regulatory system needs to consider many competing interests, including
supporting innovation, protecting human health, preserving biodiversity, reducing negative environment effects, promoting public confidence in the regulatory process, increasing transparency and predictability in the regulatory process, reducing unnecessary costs and burdens, making use of new tools from a broad array of disciplines, and interacting with the global economy. (NASEM, 2017b, p. 10)
It also concluded that advances in biotechnology over the next 5–10 years threaten to overwhelm the U.S. regulatory system, with regulators facing difficult challenges posed by new types of biotechnology products. Notably, product regulation in the United States includes both ex ante (pre-market testing) and ex post (evaluating performance) components, and regulatory regimes aim to optimize the two, taking benefits and risks into account (Innes, 2004). In 2019, an Executive Order was released that was aimed at capitalizing on benefits from agricultural biotechnology by modernizing regulatory oversight frameworks (White House, 2019).
The Role of Standards in Supporting Scientific and Technical Progress and Commercialization
Standards-setting activities supported by the U.S. government and by professional communities, including public–private partnerships, can clarify directions of technical progress, weighing the need not to prematurely constrain innovation by setting standards too early with the need to obtain efficiencies and improve interoperability by developing standards and relevant measurement techniques in a timely manner. The U.S. government has generally encouraged the development and use of voluntary consensus standards developed by experts in a field. The National Technology Transfer and Advancement Act of 1995 (P.L. 104-113), for example, asks NIST “to coordinate the use by Federal agencies of private sector standards, emphasizing where possible the use of standards developed by private, consensus organizations,” in addition to continuing to carry out NIST’s own important standards-setting activities.
A number of different types of open and proprietary standards and reference materials exist across the life sciences and enabling technologies that contribute to the bioeconomy. These include such diverse examples as the NIST monoclonal antibody reference material standard (NIST RM8671), which supports consistent characterization of physicochemical and biological properties of monoclonal antibodies.18 As advances in such areas as synthetic biology have continued to drive a number of developments in the bioeconomy, the field has also moved to create a corresponding standards infrastructure. In 2015, the Synthetic Biology Standards Consortium was established as a forum for academic, industry, nonprofit, and public entities to identify metrology needs and technical standards for the community.19 The development of standards can be particularly challenging in fast-moving fields such as synthetic biology. A report from RAND Europe, commissioned by the British Standards Institute and based on stakeholder interviews, illustrates many perceived benefits of standards in support of innovation and commercialization, while highlighting such challenges as high biological complexity that make effective standardization difficult (Parks et al., 2017).
Targeted Use of Government Purchasing Power and Incentive Programs for Biobased Products
The U.S. government is also a customer of bioeconomy goods and services, using procurement programs and other incentives to stimulate demand and encourage further private investment. One example—USDA’s BioPreferred program—is described in Box 5-3. The use of mandates has similarly been credited with providing incentives for industry to develop infrastructure that has advanced biofuel markets in Brazil and the United States (Cicogna et al., 2017).
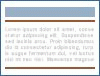
BOX 5-3
The U.S. Department of Agriculture’s (USDA’s) BioPreferred Program.
Procurement by government or public-sector entities accounts for a significant fraction of the demand for goods and services and is increasingly seen as an important factor in achieving innovation policy objectives (Uyarra and Flanagan, 2010). Public procurement is the mechanism by which governments acquire goods and services needed to fulfill their functions. Purchases occur in a number of sectors, including construction, health, custodial, food services, and transportation, as well as in security and defense. Two types of procurement with impact on innovation are “public technology procurement,” where a product does not yet exist and there is anticipated demand, and “regular public procurement,” where existing products that require no additional R&D are purchased on the basis of available information about price, quantity, and performance. A market “pull,” procurement policies can catalyze the creation of new markets and provide certainty for producers.
The Value of a Skilled Bioeconomy Workforce
As demographics change and the bioeconomy continues to grow in the United States and around the world, a diverse workforce with the skills and training to take advantage of these opportunities will be needed. The United States has a long history of public and private investment in science and technology education and training in areas that will be relevant to future economic growth in the bioeconomy. “Education in science, technology, engineering, and mathematics—STEM—develops, preserves, and disseminates knowledge and skills that convey personal, economic, and social benefits” (NSB and NSF, 2018, p. 12). Higher education, including that offered by community colleges, “provides the advanced work skills needed in an increasingly knowledge-intensive, globally integrated, and innovation-based landscape” (NSB and NSF, 2018, p. 12). In 2018, the federal government released a strategy for STEM education with three aspirational goals: build strong foundations for STEM literacy; increase diversity, equity, and inclusion in STEM; and prepare the STEM workforce for the future (White House, 2018). Similarly, recent reports from the National Academies have looked to the future of graduate and undergraduate education, including in minority-serving institutions, with recommendations to maintain the ability of U.S. educational systems to fully meet the anticipated needs of the 21st century workforce (NASEM, 2018b,c).
Such trends as the convergence of disciplines—biology, chemistry, computing, engineering, and others—to support bioeconomy R&D have led to the creation of new programs to develop the next-generation workforce. Formal training programs in engineering biology and synthetic biology at the undergraduate and graduate levels in the United States continue to evolve rapidly and are multidisciplinary, encompassing elements focused on entrepreneurship, computer training (e.g., Python boot camps), and training in the use of robotics and automation.20 The longstanding International Genetically Engineered Machine (iGEM) competition has played a valuable role in spurring further interest in synthetic biology, an area driving progress in the bioeconomy. Now 15 years old, the program has engaged more than 30,000 high school and undergraduate students and instructors, with 353 teams from around the world taking part in the 2019 competition. In addition to building scientific skills and interest in engineering biology, iGEM emphasizes responsible conduct of the scientific experiments undertaken by teams and promotes such norms.21 IGEM awards are given annually for a large range of topics, including best therapeutic, best diagnostic, best energy, best software, best information processing, and best food and nutrition project, to name just a few. (See Chapter 7 for additional detail on the iGEM competition.)
Also expanding are university biobased product engineering, processing, and product development programs to meet the needs of future industrial biotechnology companies.22 As developments in the life sciences have become more data-driven, there have also been calls for greater systematic preparation at the undergraduate level to expand data-science talent (NASEM, 2019). Finally, outside of formal academic settings, such efforts as virtual reality exercises have also been proposed to spur interest in bioeconomy-related careers (Hakovirta and Lucia, 2019).
U.S. training and workforce development most closely tied to the bioeconomy have thus far taken place predominantly in synthetic biology and biotechnology, with a few programs focused on bioprocessing.23 In contrast, a number of European programs are focused specifically on “bioeconomy” training at the master’s and Ph.D. levels (Motola et al., 2018), with some recognizing the need for training a specific cadre of economists skilled in the study of primary production, biobased value chains, and societal and economic impacts of bioeconomic developments (Lask et al., 2018). See Chapter 4 for a fuller discussion of a number of metrics in such areas as graduate enrollment and degrees awarded relevant to the U.S. and international bioeconomy workforce.
TRENDS AND CHANGES IN THE BIOECONOMY
Moving forward, the life sciences communities will likely continue to experience change in the form of growing transdisciplinary and team-based science; an increasing shift toward applying engineering approaches to biology; a global environment for science that is driven by sharing, accessing, and analyzing large amounts of data; and changing stakeholders, workforce, and supply chains. These trends will also help shape the future of the U.S. bioeconomy.
Transdisciplinary Integration
Basic research activities have historically been founded around scientific disciplines—areas of knowledge and expertise that have formed the basis for research, as well as for the training and education of the next generation of scientists. As the amount of fundamental knowledge about the world has increased in these disciplines, the life science research and educational enterprise has increasingly been focused on convergent or transdisciplinary questions24 and problems that may require expertise from teams of scientists to make key breakthroughs (see Box 5-4). The disciplines feeding into the bioeconomy also continue to evolve—some are combining in new ways, while some prior fields are coming back because of new developments.
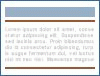
BOX 5-4
Convergence of Expertise in Tackling Mosquito-Borne Diseases.
Shift Toward Engineering Approaches
Research in biology has traditionally focused on small-scale, by-hand experimentation aimed at better understanding of biological phenomena. Breakthroughs in technology have enabled a shift to engineering biology for the manufacture of products. Synthetic biology is an example of this shift, with various technologies enabling engineers to “design, build, and test” biological systems (EBRC, 2019; NASEM, 2017b, 2018a). Engineering approaches continue to improve traditional bioeconomy sectors as well, with advances in data science, systems biology “-omics” methods, and automation reducing the amount of trial and error needed to improve biological processes and increasing the scale of production.
Access to and Analysis of Data
Progress in the life sciences and its translation into the bioeconomy are increasingly data-driven. The generation of large amounts of genomic data has become significantly less expensive with the development of high-throughput sequencing, and there is an increasing need to explore approaches to automated curation to assist in managing these growing data streams. However it often remains more expensive to acquire high-value data, particularly well-characterized genotype–phenotype information, than to retain collected data that may one day be of use in addressing new questions. As a result, databases that house and manage this information provide important infrastructure for discovery and innovation. Examples of how the collection, aggregation, and analysis of large genomic and personal health datasets provide new opportunities to advance human health are described below.
Population-Based Identification of New Biotherapeutic Opportunities
Approximately a decade after the turn of the millennium, there emerged a growing consensus that to meet the goals for turning the Human Genome Project into a medically relevant resource, researchers would have to obtain much larger populations than originally anticipated to identify robust genome–phenome associations of the sort that had been anticipated to accelerate medicine (Green and Guyer, 2011). To this end, it was quickly recognized that a national scientific priority would be to accurately clinically characterize these populations and also measure their genomic characteristics affordably (Kohane, 2011). In addition, human health and medicine are rapidly changing with changes in culture and environment. An example is the current obesity epidemic in many countries and the novel therapies being used to treat its consequences, such as diabetes mellitus. As a result, there is a pressing need to address questions in disease genomics at the population scale and answer them in just a few months rather than decades. Furthermore, in the face of increasing financial pressure on the scientific and health care establishments, these large and timely population studies of unprecedented size now must be performed at much lower cost per subject. Significant cost savings have already been realized in the genomic measurements themselves. “The cost of sequencing DNA dropped by seven orders of magnitude between 2002 and 2008 and has dropped by an additional order of magnitude between 2008 and 2015” (NASEM, 2017b, p. 28). Therefore, the clinical characterization linked to these genomic measurements represents residual and substantial costs.
Cost efficiency in clinical characterization (also termed phenotyping) of a population has been driven by secondary use of clinical annotations that are available in electronic health records (EHRs). Although there is considerable controversy about the clinical value of these systems, the availability of electronic codified data (e.g., diagnoses, procedures, laboratory values, demographics), electronic narrative text (e.g., clinic notes, discharge summaries, radiology summaries), and electronic images (i.e., most radiology studies and a steadily growing minority of pathology tissue histology studies) provides significant data resources. Use of these data for phenotyping populations at scale therefore rests on a multihundred billion dollar infrastructure in the United States alone to support interoperable data sharing associated with EHRs (Halamka and Tripathi, 2017), and also depends on and has accelerated the advanced development of natural language processing and image processing/classification techniques and a multitude of other machine learning methods. The ability to sift through a population with a phenotype of interest represents a substantial advantage, one for which pharmaceutical companies have paid hundreds of millions of dollars. Among these companies was Amgen, which in 2012 paid $415 million for well-characterized patient samples paired to their genetics for only tens of thousands of individuals when it acquired the deCODE project.
Although perhaps not central to the economics of these population analyses but societally just as controversial are the consent regimes under which these population data are gathered. In some instances, patients’ consent has been fully obtained for the secondary use of their data (e.g., use of the data for purposes beyond the primary reasons the data were originally collected). In other instances, however, patients’ consent has not been fully obtained or documented. Researchers of Deep Mind (a company acquired by Google), for example, were able to access the identified records of patients in the UK National Health Service (NHS) without the patients’ consent or knowledge (Powles and Hodson, 2017). Broader exploration of patient consent regimes and their implications can be found in reports from the Institute of Medicine (IOM, 2003, 2015).
Use of patient data companies The interest in access to well-characterized populations can be better understood through one of several case studies illustrating how finding the right patients for genetic studies can lead to scientific breakthroughs and new medicines that extend or significantly improve the quality of life, as well as substantial return on investment for companies and their shareholders. In 2001, toward the end of the Human Genome Project, an association was found between familial hypercholesterolemia and the gene products of PSCK9. Conversely, several individuals were found to have low levels of “cholesterol” (specifically LDL-C, a lipoprotein that carries cholesterol in blood), a specific subgroup of mutations (also termed genetic variants) in the PSCK9, and a significantly lower incidence of heart disease. It quickly became apparent that this finding provided an opportunity to engineer a “biological” (i.e., intravenously delivered monoclonal antibody) that would reproduce the effect of the genetic variants.
Several large pharmaceutical companies soon were racing to develop and have approved a biological targeting PSCK9. In 2015, Amgen received approval for evolocumab (trade name Repatha) from FDA. Annual sales of the drug are well above $100 million and continue to climb. As with all drug development, being first to market often is a significant financial advantage, and the longer a biological can be marketed while on patent, the larger is the advantage. Indeed, a judge granted Amgen an injunction against large competitors such as Sanofi that had a similar biological obtained through similar insights. Therefore, companies perceive privileged access to populations that can enable insights of this sort to be identified and then translated to a biological as a strategic asset.
Regeneron, for example, entered into a contractual agreement with Geisinger Health Systems that included, among other joint efforts, access to the phenotypic characterization of Geisinger’s patient populations (notably, but not only, through processing of Geisinger EHRs). These included specific populations of interest, such as a large group with severe obesity-related diseases, and genetically isolated populations, such as the Amish in Pennsylvania. Included in the agreement was funding for sequencing the exomes25 initially for 100,000 patients, but now with a target of at least 250,000 (Karow, 2017). The amount invested by Regeneron in this relationship with Geisinger has not been revealed but has been estimated to be in the hundreds of millions of dollars.
Recently, a flurry of discoveries of associations between specific genetic variants and clinical characteristics have been reported by Geisinger and Regeneron scientists in peer-reviewed scientific publications. Among these associations is that between mutations in the ANGPLT3 gene and decreased risk of cardiovascular disease. This finding led to the discovery of a biological designed to mimic the effect of these mutations (Dewey et al., 2017). Early access to such results is likely to account for the continued enthusiasm for this collaboration on the part of Regeneron’s leadership and expansion of the scope of the original agreement.
The UK Biobank: A national example26 The UK Biobank project provides a contrasting model of harnessing and mining patient populations to advance health care and science. The UK Biobank was established by the Wellcome Trust medical charity, the UK Medical Research Council, the UK Department of Health, and the Scottish and Welsh governments. The project depends on both the preexisting infrastructure of the UK NHS, which itself is a national asset, and in-kind contributions from the NHS. It is focused on 500,000 volunteers in the United Kingdom who, at the time of consent, ranged in age from 40 to 69. Recruitment began in 2006, and characterization and follow-up of these volunteers will continue for 30 years. Among the characterizations of these volunteers are anthropometrics (e.g., height, weight); blood and urine chemistries; clinical assessments, including those abstracted from the volunteers’ health records; and for subsets of these patients, imaging studies (e.g., cranial magnetic resonance imaging), genotyping, and whole-exome sequencing. For the latter, a consortium of companies (mostly pharmaceutical) has provided the funding.
From the start, the UK Biobank has been engineered to enable the widest array of researchers to access the data. In March 2012, applications for access were accepted from researchers worldwide, regardless of whether they were in the public or private domain. The only requirements were a research protocol and a nominal fee, along with verification by a UK Biobank committee that the research was in the public interest and related to health. Researchers using the data are encouraged to publish their findings in open-access publications or academic journals and to report all their results back to the UK Biobank. In the 6 years since datasets were opened to researchers, more than 500 studies have been initiated, and hundreds of publications have appeared in the biomedical literature.
U.S. researchers and U.S.-based companies are now using these data from the United Kingdom to identify clinically relevant results. For example, a group of investigators from Boston used the UK Biobank’s clinical and genotypic data to develop a polygenic risk score that appears to accurately identify those individuals at high risk of coronary artery disease (Khera et al., 2018). Furthermore, many of these investigators are founders of a $191 million–backed company that now seeks to “[expand] our understanding of the natural disease protection provided by genetic modifiers through an integrated approach that combines studying natural human genetic variation across the globe and conducting large-scale experiments of gene perturbations” [italics added] (MarketWatch, 2019). These results and business plans rest in large part on one of the largest open-access detailed genomic–phenomic datasets in the world—one that is open to all researchers.
From Sequence to Product: The Contribution of Bioinformatic Databases to Biotech Products
As illustrated above, the collection, aggregation, and analysis of increasingly large amounts of biosciences data has become a key feature of the bioeconomy. Open bioinformatic databases are routinely accessed by basic science researchers, as well as by industry to commercialize products. The first sequences of a human genome, simultaneously published by the for-profit company Celera Genomics and by the public international Human Genome Sequencing Consortium, depended heavily on the use of data generated by the U.S. government–led Human Genome Project (International Human Genome Sequencing Consortium, 2001; Venter et al., 2001). Molecular diagnostics and consumer-facing ancestry tools depend on the identification of single nucleotide polymorphisms (SNPs) as reported in the dbSNP database.27
New drugs can now be designed and tested in silico using protein structures derived from the Protein Data Bank.28 It has been estimated that 210 new molecular entities approved by FDA between 2010 and 2016 can be traced to 5,914 protein structures hosted in that data bank (Westbrook and Burley, 2019). Likewise, DNA synthesis technologies enable researchers to identify new gene functions through computational analysis of GenBank and other databases (Bayer et al., 2009). In addition, an important source of value in many companies rests in proprietary databases.
Both open-source and proprietary bioinformatics software tools, such as those used for genome annotation, depend on open bioinformatics data. In each case, basic research can lead to applications that were unanticipated by the researchers who deposited the original data. Databases such as GenBank also host the patented sequences that result from applied R&D as part of patent disclosure requirements.
While it may be impossible to quantify the total impact of open databases on the bioeconomy, the development of diagnostics, drugs, and synthetic biology products benefits from access to these resources. Mergers and acquisitions in the bioeconomy also provide some insight as to how companies value datasets, and these acquisitions may help identify adjacent technology sectors that have become important to the bioeconomy. For example, Indigo Ag, a company that develops microbial treatments for crops, purchased satellite imaging company TellusLabs in 2018. According to the two companies, this merger brings together datasets that can be leveraged via machine learning to better target products to individual farms.29
Contribution of Establishing Standards and Frameworks to the Utility of Life Sciences Datasets
Establishing common standards and frameworks is important to enable taking advantage of data that can advance basic science discovery and innovation. As an example, Box 5-5 describes the value of the Universal Protein Resource for aggregating and analyzing protein sequence and functional information. This example illustrates the essential need for automatic curation capabilities in modern databases, arising from the deluge of incoming data. The value of such databases is not measured by the capability to compile the data automatically, but by the user’s ability to have confidence that redundant or erroneous information has been handled.
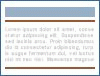
BOX 5-5
The Universal Protein Resource.
Despite the value of consolidated scientific databases, the migration of The Arabidopsis Information Resource (TAIR) from a federally funded database to a not-for-profit organization providing access on a subscription basis illustrates a vulnerability of such databases (Berardini et al., 2015). TAIR curated genetic and molecular information on Arabidopsis, a model plant widely used in the global scientific community. The database was launched in 1999 and in 2014 reported 178,000 visits per month from 61,000 users worldwide. The mission of the database ecosystem was to provide gold standard functional annotation of the organism to the scientific community, but in 2014, the operators of TAIR reported that its primary mission had been “significantly curtailed” as the result of loss of its main national-level funding. The database was subsequently moved to a not-for-profit organization, and a sustainable subscription model was identified (Reiser et al., 2016). The current TAIR resource operators acknowledge that a transition to subscription-based models is not feasible for all publicly funded databases and propose a range of options to be explored. They furthermore point out that secure funding is necessary for sustainable database operation, but is not the only essential ingredient. Their first recommendation is the development of accurate computationally assisted curation, along with a more comprehensive suite of tools to reduce costs associated with creating and distributing the components of such resources within and to the scientific community. An important general consideration is which organization(s) should fund data preservation and (open) dissemination. Such investments are typically not aligned with the mission of industry; therefore, government support for such infrastructure investments may be justified when considered relative to the cost, in terms of leadership and R&D productivity, of not having such data available.
The Changing Players of Biotechnology Innovation
The formation of companies in the biotech space has changed dramatically in the past decade. While biotech investment has traditionally been focused in the pharmaceutical, agricultural, and industrial biotech sectors, a broader array of application areas and new investors has more recently emerged, including start-ups, that are focused on genetic tool development and services, high-throughput screening technology, textiles, and alternative food proteins (Schmidt, 2019). For example, in 2018 more than $3.8 billion in private capital was raised for 97 companies addressing multiple applications of synthetic biology. In comparison, the fiscal year 2018 NIH budget was more than $27 billion.30
Furthermore, many of these companies are not direct products of academic institutions but founded independently or within start-up incubators. Some of these incubators came from the traditional tech sector. An example is Y Combinator—associated with such companies as Airbnb and Dropbox—which has now funded more than 140 biotech companies, with 15 percent of its new companies funded in 2018 being involved in biotech (Rey, 2018).31 Other incubators—such as IndiBio32 or QB333 (affiliated with the University of California)—have been founded for the express purpose of launching new biotech companies. Self-funded community labs, such as BioCurious (which started on the crowdfunding platform Kickstarter), have also become de facto preincubators by offering spaces for scientists from both traditional research institutions and nontraditional backgrounds to develop concepts for companies in an open precompetitive space.
The broadening focus and background of companies in the biotech space is resulting in an ecosystem of interdependent companies, analogous to the development and maturation of the digital sector. For example, many companies focus on individual services or product categories, such as biological design and statistical software (e.g., Benchling, Synthace through its Antha software in the United Kingdom, Ryffin) or biology tool components (e.g., Synthego, Caribou). Other companies focus on increasing product yield and addressing scale-up challenges, such as by improving engineering of microbial strains in synthetic biology applications. Still other companies are forming vertically integrated “stacks” or horizontal “platforms” that bundle services together to target specific markets or consolidate work across many markets that all require specific services. An example of such a stack used to advance synthetic biology is shown in Figure 5-2. These tool and service provider companies form a life sciences supply chain that can be globally distributed.

FIGURE 5-2
The synthetic biology “stack”. This synthetic biology stack shows several layers that can contribute to a final product. Each horizontal layer represents a set of consolidated tools and services used to expedite the production of a specialized (more...)
As discussed in further detail in Chapter 3, the changing landscape of biotechnology development and the growing network of service and provider companies pose new challenges for assessing the size and value of the bioeconomy.
The Changing Bioeconomy Workforce
The rapid pace of research and reliance on enabling technologies and data sharing also pose challenges to how life sciences undergraduate and graduate students are trained, indicating that new approaches to education and training will be needed within universities. In addition, R&D activities are no longer limited to university laboratories. Technology today gives the entire community access to key resources, and science is beginning to be pursued in homes, community centers, online communities, and other nontraditional avenues. Because such simple metrics, such as counting the number of Ph.D.s issued in life sciences subfields, no longer capture all of the R&D efforts relevant to the bioeconomy, updated models for collecting bioeconomy data, including research investments and workforce numbers, will be needed.
As the biotechnology industry continues to grow, classic life sciences training provided at colleges and universities needs to evolve to help prepare students for these types of jobs (Delebecque and Philp, 2019). Students tend to lack interdisciplinary knowledge, and there tends to be a disconnect between what they are taught and what is actual industry practice (Thompson et al., 2018). Industry employees with life sciences knowledge and bachelor’s degrees are an important need for a large part of the growing bioeconomy workforce. One study showed that industrial biotechnology companies are overwhelmingly looking to hire entry-level workers with bachelor’s degrees (Delebecque and Philp, 2019). While most employers prefer applicants with a degree in the life sciences, there is greater interest in such qualities as willingness to learn. Some places are leading the way toward training the needed biotechnology workforce. California, for example, mobilized several of its community colleges to prepare a diverse array of students for future careers in the field (Monis, 2018), as illustrated by the Solano Community College Biotechnology and Science Building. This facility contains a simulation of an industrial laboratory where students can obtain hands-on experience in topics related to biomanufacturing. The course load for the college’s degree in biomanufacturing is heavily weighted toward science, in addition to courses that help the students simulate biomanufacturing procedures and production (Monis, 2018).
Regional Innovation Hubs and Geographic Distribution of the U.S. Bioeconomy
Regional ecosystems of innovation can arise near areas of major basic research investment, such as public, private, and land-grant universities and federal research laboratories (Baily and Montalbano, 2018; EUA, 2019). These innovation ecosystems, which include start-up companies, small businesses, and affiliated infrastructure, are designed to translate basic research discoveries into economic and societal impact, although the evidence that university entrepreneurship efforts can catalyze regional entrepreneurship vary (Qian and Yao, 2017).
In addition, all regions of the United States make contributions to the bioeconomy. The diversity of contributors to the bioeconomy is reflected in the geographic distribution of relevant facilities across the country. To illustrate, a set of examples focused on a comparison of the distribution of bioethanol fermentation facilities and the distribution of companies focused on biotechnology R&D is presented below. While these examples illustrate geographic distribution within the United States, many of the factors leading to these U.S. distributions can be expected to apply to global efforts to cultivate aspects of the bioeconomy.
Within the United States, fermentation capacity is predominantly for bioethanol production. Total production of bioethanol exceeded 15 billion gallons (approximately 57 billion liters) in 2017, with more than 13 billion of those gallons being produced in the Midwest (see EIA, 2017, Figure 5-334). This capacity is distributed across 200 ethanol plants, 176 of which are located in the Midwest (see EIA, 2019; Figure 5-4). The distribution of bioethanol fermentation plants is driven largely by the distribution of corn production, from which the vast majority of bioethanol is produced. In general, the transport of refined higher-value product is preferred to the transport of lower-value feedstocks, leading to fermentation capacity that is generally collocated with feedstock. The optimal locations for growing feedstocks may change as the Earth’s climate changes, calling for system designs that are resilient to changes in agricultural land use. If feedstock cultivation moves on a timescale that is faster than the replacement of fermentation capacity, new fermentation capacity may need to be built to follow the feedstocks, new feedstocks may need to be developed to supply existing facilities, or feedstocks will need to be transported.

FIGURE 5-3
U.S. ethanol production capacity by state. Significant production of ethanol for fuel is in states from PADD region 2, encompassing the Midwest. NOTE: PADD = Petroleum Administration for Defense Districts classification. SOURCE: EIA, 2017.

FIGURE 5-4
Distribution of ethanol production plants and National Institutes of Health (NIH) funding. The majority of ethanol production plants (dots) are located in the Midwest, whereas NIH funding for all purposes (shading), which in fiscal year 2018 ranged from (more...)
Corn production in the United States has grown dramatically to supply the bioethanol industry. Over the past 30 years, U.S. corn usage has more than doubled, with the vast majority of that growth going to bioethanol production, and corn usage for feed remaining effectively flat (see Figure 5-5). Currently, approximately 5 billion bushels of corn are converted to about 15 billion gallons of bioethanol. By comparison, about 315 billion gallons of oil (7.5 billion barrels35) are used in the United States each year. This relationship between corn and bioethanol output may shift dramatically with the maturation of “second-generation” biofuels that can leverage lignocellulosic biomass rather than the starch from corn as a feedstock. However, expanding fermentation capacity or redirecting some current ethanol fermenters to other bioproducts may be needed to fully exploit this possibility. A focus on higher-value products may allow dramatic growth in bioproduction without requiring massive increases in feedstock supply.

FIGURE 5-5
Corn usage in the United States since the early 2000s. Corn usage for feed, food, industrial uses, and other residual uses has remained relatively flat, while the conversion of corn into alcohol for biofuels has increased. SOURCE: https://www.ers.usda.gov/topics/crops/corn-and-other-feedgrains/feedgrains-sector-at-a-glance (more...)
In contrast to the concentration of fermentation capacity in the Midwest, biotechnology R&D is concentrated largely in coastal states. This trend is observed for NIH research funding, with nearly $10 billion of the $28 billion 2018 NIH budget being awarded to institutions in California, Massachusetts, and New York36 (see Figure 5-4). This trend is mirrored by venture capital funding, which is overwhelmingly concentrated on the coasts, regardless of sector.37 Start-ups in both such traditional sectors as biopharmaceuticals and such emerging sectors as synthetic biology have remained concentrated in coastal cities (Synbiobeta, 2018). California, Massachusetts, and New York may have a strong advantage in capturing the future growth of both biotechnology R&D and industry start-ups, as these states provide well-funded research universities, industrial research centers, and access to seed and growth capital. Regional centers with access to similar resources have been successful at growing their biotechnology workforces through focused investment and training programs (Feldman, 2019).
While bioethanol fermentation and biotech R&D represent just two facets of the bioeconomy, they illustrate the complexities of investing in the bioeconomy’s growth. For example, growth in the production of bioethanol could be encouraged through lower corn prices, breakthroughs in the utilization of cellulosic biomass, or subsidies for bioethanol-blended gasoline. Yet, those same factors might not stimulate coastal bioeconomic productivity. Similarly, investment in skilled labor to support bioethanol production would likely favor degrees in chemical engineering rather than molecular biology. To have their intended effect, then, policies intended to safeguard or grow the bioeconomy need to recognize the variability in technologies, workforces, and critical infrastructure. Global competition in the bioeconomy similarly manifests across this spectrum. For example, low-cost sugarcane in Brazil attracts U.S. companies to manufacture there, while government-supported biotech start-up incubators in the United Kingdom vie for U.S.-trained scientists. Regional variation of bioeconomy activities also suggests that different strategies may be most effective based on the region and intended impact. As discussed in this chapter, these strategies include university-driven tech transfer, as well as nonuniversity institutions such as start-up accelerators.
Interdependency and Supply Chains in the Bioeconomy
While large-scale fermentation tends to be closely associated with the regional availability of feedstocks, a resilient supply chain system will be required if growing locations for these feedstocks move in a timeframe that is faster than the replacement of fermentation capacity. In addition, many of the other critical materials of the bioeconomy, such as DNA, cells, and seeds, are mobile and are often developed across borders. A seed designed to be grown in Brazil may have been engineered in the United States using DNA synthesized in Europe with phosphoramidites and other reagents sourced from China. The complexity of these supply chains can result in unforeseen shortages of key materials. For example, more than 80 percent of the world’s supply of agar and agarose for biological research derives from the red algae of genus Gelidium that are harvested in Morocco; changes in how this harvest is managed have resulted in shortages and price increases (Santos and Melo, 2018). Complex and global supply chains can also be exploited by counterfeiting. Such products as honey are reportedly among the most commonly counterfeited foods through the addition of lower-cost sugars, use of less expensive production processes, rebranding of product origins, and other means (Zhou et al., 2018).
STRATEGIC PLANNING IN SUPPORT OF THE U.S. BIOECONOMY
The pace of advances in the life sciences and converging scientific and technical fields continues to grow through the efforts of diverse stakeholders in public and private organizations in the United States and around the world and supported by multiple funding sources, as well as a growing system of supply and service provider companies. This complexity makes strategic planning in support of the U.S. bioeconomy highly challenging. Nevertheless, some strategies that may be able to help identify and anticipate trends can be explored. This section illustrates how mapping against TRLs can contribute to further planning.
The TRL scale provides one lens through which the complexity of funding from invention to commercialization has been examined. This scale represents the stages of maturity of a technology, from basic research through the establishment of proof of concept (TRLs 1–3), through additional laboratory testing and prototype validation (TRLs 4–6), to integration in a pilot system and demonstration of readiness for full commercial deployment (TRLs 7–9).
Although it originated in engineering disciplines, the concept of the TRL scale has been adopted, with requisite criterion adaptation, to give funders and policy makers a tool for managing bioscience investments, as illustrated by cases in Europe and the United Kingdom. The European Association of Research and Technology Organisations (EARTO) traced the history of the TRL scale from its origins in NASA and the U.S. Department of Defense, where it was devised to “enable assessment of the maturity of a particular technology and the consistent comparison of maturity between different types of technologies” (EARTO, 2014). EARTO’s purpose was to establish TRLs as a policy tool in national funding for the European Union’s Horizon 2020 program. As a result, the European Union is now capable of assessing aspects of its bioeconomy efforts in part through a TRL lens (Spatial Foresight et al., 2017). In the European Union, the TRL concept is now being applied to Responsible Research and Innovation via the European Research Area Network Cofund for Biotechnologies (ERA CoBioTech), where evaluation criteria include funding applicants presenting their project outputs in the area of technological and economic development “by describing an envisioned plan to achieve a higher TRL of the processes and technologies.”38,39
In another example, the UK Engineering and Physical Sciences Research Council has also applied TRLs to its health care investment framework.40 The concept of TRLs figures explicitly in the definition of the UK National Industrial Biotechnology Strategy for 2030. “The vision for UK IB is one that transcends politics, where finance is available for business growth and innovation across Technology Readiness Levels.”41
Within the United States, TRLs are used in national investment strategies. A recent report by the National Academies arrayed broad U.S. programs along a TRL axis (NASEM, 2017a). NSF used TRLs in assessing funding flow into synthetic biology, and the National Institute of Food and Agriculture at USDA has created a TRL for crop research readiness.42 The interplay of government, industry, and venture funding sources can be illustrated by bioenergy refinery development within the U.S. Department of Energy. Male (2019) documents the investment needed to move from bench-scale biomass conversion (grams per day, investment $1–$5 million) to a full production plant (>250 tonnes per day biomass, investment $250–$500 million).
TRLs serve as a convenient x-axis for examining the so-called “valley of death” for movement from invention to commercialization. The Global Federation of Competitiveness Councils uses this format to illustrate the gap between earlier technology developers in the public sector and later commercial producers in the private sector (see Figure 5-6). The gap is due to the inability to fund derisking activities, including prototype development and the collection of data necessary for manufacturing scale-up.

FIGURE 5-6
Mapping research generally undertaken or supported by the federal government and universities along a TRL axis. These entities fund primarily TRLs 1–4, basic research to proof of feasibility. Efforts supported or undertaken by the private sector (more...)
The NSF Engineering Research Centers program has elaborated aspects of a policy funding strategy for Engineering Research Centers to address this gap (Jackson, 2011). Jackson likens the innovation ecosystem to biological ecosystems observed in nature. One concept relevant to the design–build–test cycle is the role of rapid-prototyping infrastructure. Jackson argues that a “bridge” across the valley of death can be created by infrastructure investments that enable rapid prototyping. Such investments lower costs to start-ups for engaging in innovation and raise the success rate of innovation toward a commercially relevant target.
CONCLUSIONS
This chapter described the system for translating research into innovation in the U.S. bioeconomy; trends in the pace, nature, and scope of developments that support life sciences innovation; and a number of areas in which federal and private-sector policies and practices support and sustain U.S. leadership in the bioeconomy. Based on the findings documented in this discussion, the committee arrived at several conclusions related to discovery and innovation in the bioeconomy.
Conclusion 5-1: Maintaining U.S. bioeconomy leadership will require sustaining a vibrant science and technology base in relevant areas, an ecosystem that encompasses start-up companies as well as large-scale manufacturing, skilled human resources, an agile and effective regulatory system, and other policies that support innovation and commercialization of the research and entrepreneurial enterprise.
A number of trends are driving discovery and economic impact in the bioeconomy, including increasingly convergent/transdisciplinary science; a shift toward applying engineering approaches to biological problems; access to large biological datasets and the tools needed to analyze such data; and new opportunities to translate research to innovation in start-up incubators, community labs, and other venues that complement traditional university- and national laboratory-based research.
Conclusion 5-2: The continued discovery of new and exciting biology and the continued creation of enabling platform technologies and shifts in how researchers and developers approach problems that require transdisciplinary integration are needed to sustain the creation of new application areas in the bioeconomy and to accelerate the timelines for commercial translation.
Conclusion 5-3: Strategies that enhance access to data repositories and to software and other tools for data analysis, along with creation of data standards frameworks, would increase the ability of U.S. researchers and developers to create bioeconomy opportunities. The impact of expanded access to these resources is challenging to quantify, but a sense of their potential value can be extrapolated from investments in data being made by private-sector companies.
As the United States continues to grow and sustain its bioeconomy ecosystem, it is important to recognize that all stakeholders are involved in these efforts that it will be important to integrate their input. To assist policy makers and stakeholders in the bioeconomy, the committee notes the following.
Conclusion 5-4: No one entity within the U.S. government or among nonfederal stakeholders is responsible for the bioeconomy. This reality creates a gap in the ability of policy makers to anticipate trends and develop coherent policies to support continued U.S. growth and leadership in the bioeconomy. However, the expanded use of such planning tools as Technology Readiness Levels, biobased procurement programs, and other strategies would provide opportunities to support and grow the bioeconomy across all regions of the United States, enabling bioeconomy development to contribute to both urban and rural prosperity.
This chapter of the report has explored how best to sustain the ecosystem of stakeholders within the bioeconomy. The next chapter shifts the discussion to additional strategies for looking to the future to anticipate trends and changes through horizon scanning processes that can help support improved strategic planning.
REFERENCES
- Apweiler R, Bairoch A, Wu CH, Barker WC, Boeckmann B, Ferro S, Gasteiger E, Huang H, Lopez R, Magrane M, Martin MJ, Natale DA, O’Donovan C, Redaschi N, Yeh LSL. UniProt: The Universal Protein Knowledgebase. Nucleic Acids Research. 2004;32:D115–D119. [PMC free article: PMC308865] [PubMed: 14681372]
- Baily MN, Montalbano N. Clusters and innovation districts: Lessons from the United States Experience. Washington, DC: Brookings Institution; 2018. [September 3, 2019]. https://www
.brookings .edu/wp-content/uploads /2018/01/es_20180116 _bailyclustersandinnovation.pdf. - Bayer TS, Widmaier DM, Temme K, Mirsky EA, Santi DV, Voigt CA. Synthesis of methyl halides from biomass using engineered microbes. Journal of the American Chemical Society. 2009;131(18):6508–6515. doi: 10.1021/ja809461u. [PubMed: 19378995]
- Berardini TZ, Reiser L, Li D, Mezheritsky Y, Muller R, Strait E, Huala E. The Arabidopsis Information Resource: Making and mining the “gold standard” annotated reference plant genome. Genesis. 2015;53:474–485. [September 3, 2019]; https://www
.arabidopsis .org/about/Berardini _et_al-2015-genesis.pdf. [PMC free article: PMC4545719] [PubMed: 26201819] - Bristow MR, Leinwand LA, Olson EN. Entrepreneurialism in the translational biologic sciences: Why, how, and however. JACC: Basic to Translational Science. 2018;3(1):1–8. [PMC free article: PMC6058957] [PubMed: 30062188]
- Bubela T, Guebert J, Mishra A. Use and misuse of material transfer agreements: Lessons in proportionality from research, repositories, and litigation. PLoS Biology. 2015;13:e1002060. [PMC free article: PMC4315468] [PubMed: 25646804]
- Burk DL. DNA copyright in the administrative state. UC Davis Law Review. 2018;51:1297–1349.
- Bush V. Science, the endless frontier: A report to the President. 1945. [September 3, 2019]. https://www
.nsf.gov/od /lpa/nsf50/vbush1945.htm. - Carrico JA, Rossi M, Moran-Gillad J, Van Domselaar G, Ramirez M. A primer on microbial bioinformatics for nonbioinformaticians. Clinical Microbiology and Infection. 2019;24(4):342–349. [PubMed: 29309933]
- Chen P, Bacon R, Hart T, Orazaly M, Florez-Palacios L, Manjarrez-Sandoval P, Wu C, Rogers D, Bathke G, Ahrent-Wisdom D, Sherman R, Clark S. Purification and production of breeder seed and foundation seed of University of Arkansas System Division of Agriculture soybean lines. In: Ross J, editor. Arkansas Soybean Research Studies. Arkansas Agricultural Experiment Station. 2016. [October 14, 2019]. https:
//arkansas-ag-news .uark.edu/pdf/648 _Arkansas_Soybean_Research _Studies_2016.pdf. - Chi-Ham CL, Boettiger S, Figueroa Balderas R, Bird S, Geoola JN, Zamora P, Alandete-Saez M, Bennett AB. An intellectual property sharing initiative in agricultural biotechnology: Development of broadly accessible technologies for plant transformation. Plant Biotechnology Journal. 2012;10(5):501–510. [PubMed: 22221977]
- Cicogna MPV, Khanna M, Zilberman D. Handbook of bioenergy economics and policy. II. New York: Springer International Publishing; 2017. Prospects for biofuel production in Brazil: Role of market and policy uncertainties; pp. 89–117.
- Courneya JP, Mayo A. High-performance computing service for bioinformatics and data science. Journal of the Medical Library Association. 2018;106(4):494–495. [PMC free article: PMC6148605] [PubMed: 30271293]
- Cumbers J. Presentation to the Committee on Safeguarding the Bioeconomy. Jan 28, 2019. [October 30, 2019]. Defining the bioeconomy. http://nas-sites
.org /dels/studies/bioeconomy/meeting-1. - Delebecque CJ, Philp J. Education and training for industrial biotechnology and engineering biology. Engineering Biology. 2019;3(1) doi: 10.1049/enb.2018.0001.
- Dewey FE, Gusarova V, Dunbar RL, O’Dushlaine C, Schurmann C, Gottesman O. Genetic and pharmacologic inactivation of ANGPTL3 and cardiovascular disease. New England Journal of Medicine. 2017;377:211–221. doi: 10.1056/NEJMoa1612790. [PMC free article: PMC5800308] [PubMed: 28538136]
- Dolgin E. Nature Reviews Drug Discovery. Feb 27, 2019. [September 3, 2019]. Massive NIH–industry project opens portals to target validation. https://www
.nature.com /articles/d41573-019-00033-8. [PubMed: 30936505] - EARTO (European Association of Research and Technology Organisations). The TRL scale as a research & innovation policy tool, EARTO recommendations. 2014. [October 3, 2019]. https://www
.earto.eu /wp-content/uploads/The _TRL_Scale_as_a_R _I_Policy_Tool_-_EARTO _Recommendations_-_Final.pdf. - EBRC (Engineering Biology Research Consortium). Engineering biology: A research roadmap for the next-generation bioeconomy. 2019. [September 3, 2019]. https://roadmap
.ebrc.org. - EIA (U.S. Energy Information Administration). U.S. ethanol production capacity continues to increase. 2017. [September 3, 2019]. https://www
.eia.gov/todayinenergy /detail.php?id=31832. - EIA. U.S. fuel ethanol plant production capacity. 2019. [September 3, 2019]. https://www
.eia.gov/petroleum /ethanolcapacity/index.php. - Eisenberg RS. Patents and regulatory exclusivity. In: Danzon P, Nicholson S, editors. The Oxford Handbook of the Economics of the Biopharmaceutical Industry. Oxford, UK: Oxford University Press; 2012. [October 14, 2019]. pp. 167–198. https://repository
.law .umich.edu/book_chapters/126. - EOP (Executive Office of the President). Improving regulation and regulatory review. 2011. [January 18, 2011]. [October 1, 2019]. (Executive Order 13563). https:
//obamawhitehouse .archives.gov/thepress-office /2011/01 /18/executive-order-13563-improving-regulation-and-regulatoryreview. - EOP. Modernizing the regulatory system for biotechnology products: Final version of the 2017 update to the coordinated framework for the regulation of biotechnology. 2017. [October 3, 2019]. https:
//obamawhitehouse .archives.gov/sites /default/files/microsites /ostp/2017_coordinated _framework_update.pdf. - EOP. Efficient federal operations. Executive Order 13834. Federal Register. 2018;83:23771–23774. [October 18, 2019]; https://www
.federalregister .gov/documents /2018/05/22/2018-11101 /efficient-federal-operations. - EUA (European University Association). The role of universities in regional innovation ecosystems. 2019. [September 3, 2019]. https://eua
.eu/downloads /publications/eua %20innovation%20ecosystem %20report%202019v1 .1_final_digital.pdf. - Feldman M. Presentation to the Committee on Safeguarding the Bioeconomy. May 1, 2019. [October 14. 2019]. The economics of innovation and the commercialization of research. http://nas-sites
.org /dels/studies/bioeconomy/meeting-3. - Gilbert JA, Melton L. Verily project releases millions of factory-reared mosquitoes. Nature Biotechnology. 2018;36:781–782. [PubMed: 30188519]
- Golden JS, Handfield RB, Daystar J, McConnell TE. U.S. Department of Agriculture. 2016. An economic impact analysis of the U.S. biobased products industry. https://www
.biopreferred .gov/BPResources /file/BiobasedProductsEconomicAnalysis2016.pdf. - Green ED, Guyer MS. National Human Genome Research Institute. Charting a course for genomic medicine from base pairs to bedside. Nature. 2011;470:204–213. doi: 10.1038/nature09764. [PubMed: 21307933]
- Hakovirta M, Lucia L. Informal STEM education will accelerate the bioeconomy. Nature Biotechnology. 2019;37:103–104. [September 3, 2019]; https://www
.nature.com/articles/nbt.4331. [PubMed: 30605146] - Halamka JD, Tripathi M. The HITECH era in retrospect. New England Journal of Medicine. 2017;377:907–909. doi: 10.1056/NEJMp1709851. [PubMed: 28877012]
- Hockberger P, Weiss J, Rosen A, Ott A. Building a sustainable portfolio of core facilities: A case study. Journal of Biomolecular Techniques. 2018;29(3):79–92. [PMC free article: PMC6078059] [PubMed: 30140172]
- Holman CM. Charting the contours of a copyright regime optimized for engineered genetic code. Oklahoma Law Review. 2017;69(3) [October 14, 2019]; http:
//digitalcommons .law.ou.edu/olr/vol69/iss3/2. - IAC (InterAcademy Council). Inventing a better future: A strategy for building worldwide capacities in science and technology. Amsterdam, The Netherlands: InterAcademy Council; 2014. [October 3, 2019]. http://www
.interacademies .org/33347.aspx?id=33347. - Innes R. Enforcement costs, optimal sanctions, and the choice between ex-post liability and ex-ante regulation. International Review of Law and Economics. 2004;24:29–48.
- International Human Genome Sequencing Consortium. Initial sequencing and analysis of the human genome. Nature. 2001;409(6822):860–921. [PubMed: 11237011]
- IOM (Institute of Medicine). Responsible research: A systems approach to protecting research participants. Washington, DC: The National Academies Press; 2003. https://doi
.org/10.17226/10508. [PubMed: 20669487] - IOM. Informed consent and health literacy: Workshop summary. Washington, DC: The National Academies Press; 2015. https://doi
.org/10.17226/19019. - Jackson D. National Science Foundation. 2011. What is an innovation ecosystem. http://erc-assoc
.org /sites/default/files /topics/policy_studies /DJackson_Innovation %20Ecosystem_03-15-11.pdf. - Jefferson OA, Jaffe A, Ashton D, Warren B, Koellhofer D, Dulleck U, Ballagh A, Moe J, DiCuccio M, Ward K, Bilder G, Dolby K, Jefferson RA. Mapping the global influence of published research on industry and innovation. Nature Biotechnology. 2018;36:31–39. [PubMed: 29319684]
- Kahl L, Molloy J, Patron N, Matthewman C, Haseloff J, Grewal D, Johnson R, Endy D. Opening options for material transfer. Nature Biotechnology. 2018;36(10):923–927. [PMC free article: PMC6871013] [PubMed: 30307930]
- Karow J. GenomeWeb. Nov 16, 2017. [September 3, 2019]. Geisinger experience implementing precision medicine spurs new effort to help others do the same. https://www
.genomeweb .com/molecular-diagnostics /geisinger-experience-implementing-precision-medicine-spursnew-effort-help. - Khera AV, Chaffin M, Aragam KG, Haas ME, Roselli C, Choi SH, Natarajan P, Lander ES, Lubitz SA, Ellinor PT, Kathiresan S. Genome-wide polygenic scores for common diseases identify individuals with risk equivalent to monogenic mutations. Nature Genetics. 2018;50:1219–1224. doi: 10.1038/s41588-018-0183-z. [PMC free article: PMC6128408] [PubMed: 30104762]
- Kohane IS. Using electronic health records to drive discovery in disease genomics. Nature Reviews Genetics. 2011;12(6):417–428. doi: 10.1038/nrg2999. [PubMed: 21587298]
- Lask J, Maier J, Tchouga B, Vargas-Carpintero R. The bioeconomist. In: Lewandowski I, editor. Bioeconomy: Shaping the transition to a sustainable, biobased economy. Cham, Switzerland: Springer; 2018. pp. 343–356. doi: 10.1007/978-3-319-68152-8.
- Link AN, Morrison LTR. Innovative activity in minority-owned and women-owned business: Evidence from the U.S. Small Business Innovation Research Program. Cham, Switzerland: Springer; 2019. The U.S. small business innovation research program; pp. 13–27. doi: 10.1007/978-3-030-21534-7.
- Male J. Project Peer Review. Mar 4, 2019. [October 14, 2019]. BETO overview, slide 18 2019. https://www
.energy.gov /sites/prod/files/2019 /03/f60/Day%201_Plenary _Male_BETO_Overview.pdf. - MarketWatch. Maze Therapeutics launches with $191 million to focus on translating genetic insights into new medicines. 2019. [September 3, 2019]. https://www
.marketwatch .com/press-release /maze-therapeutics-launches-with-191-million-to-focus-on-translating-genetic-insights-into-new-medicines-2019-02-28. - Molino A, Larocca V, Chianese S, Musmarra D. Biofuels production by biomass gasification: A review. Energies. 2018;11(4):811. doi: 10.3390/en11040811.
- Monis I. Designing for STEM: California community colleges are helping shape the STEM workforce of the future. Planning for Higher Education Journal. 2018;47(1):32–38.
- Motola V, De Bari I, Pierro N, Giocoli A., ENEA. Bioeconomy and biorefining strategies in the EU member states and beyond. 2018. [October 30, 2019]. (IEA bioenergy task 42). https://www
.ieabioenergy .com/wp-content/uploads /2018/12/Bioeconomy-and-Biorefining-Strategies _Final-Report_DEC2018.pdf. - Narayanan D, Weingarten M. Chapter 9—An introduction to the National Institutes of Health SBIR/STTR programs. In: Behrns KE, Gingles B, Sarr MG, editors. Medical innovation: Concept to commercialization. Cambridge, MA: Academic Press; 2018. pp. 87–100.
- NASEM (National Academies of Sciences, Engineering, and Medicine). A new vision for center-based engineering research. Washington, DC: The National Academies Press; 2017a. https://doi
.org/10.17226/24767. - NASEM. Preparing for future products of biotechnology. Washington, DC: The National Academies Press; 2017b. https://doi
.org/10.17226/24605. [PubMed: 28737846] - NASEM. Biodefense in the age of synthetic biology. Washington, DC: The National Academies Press; 2018a. https://doi
.org/10.17226/24890. [PubMed: 30629396] - NASEM. Data science for undergraduates: Opportunities and options. Washington, DC: The National Academies Press; 2018b. https://doi
.org/10.17226/25104. [PubMed: 30407778] - NASEM. Graduate STEM education for the 21st century. Washington, DC: The National Academies Press; 2018c. https://doi
.org/10.17226/25038. - NASEM. Open science by design: Realizing a vision for 21st century research. Washington, DC: The National Academies Press; 2018d. https://doi
.org/10.17226/25116. [PubMed: 30212065] - NASEM. Minority serving institutions: America’s underutilized resource for strengthening the STEM workforce. Washington, DC: The National Academies Press; 2019. https://doi
.org/10.17226/25257. - Nature Biotechnology [Editorial] The service-based bioeconomy. Vol. 32. 2014. [October 14, 2019]. p. 597. https://www
.nature.com/articles/nbt.2961. - Nielsen J, Bubela T, Chalmers DRC, Johns A, Kahl L, Kamens J, Lawson C, Liddicoat J, McWhirter R, Monotti A, Scheibner J, Whitton T, Nicol D. Provenance and risk in transfer of biological materials. PLoS Biology. 2018;16(8):e2006031. [PMC free article: PMC6107254] [PubMed: 30102688]
- NIST (National Institute of Standards and Technology). Return on investment initiative for unleashing American innovation. 2019. [October 14, 2019]. (NIST Special Publication 1234). https://nvlpubs
.nist .gov/nistpubs/SpecialPublications/NIST .SP.1234.pdf. - NRC (National Research Council). Responsible science, Volume I: Ensuring the integrity of the research process. Washington, DC: National Academy Press; 1992.
- NRC. A patent system for the 21st century. Washington, DC: The National Academies Press; 2004. https://doi
.org/10.17226/10976. - NRC. Managing university intellectual property in the public interest. Washington, DC: The National Academies Press; 2011. https://doi
.org/10.17226/13001. - NRC. Convergence: Facilitating transdisciplinary integration of life sciences, physical sciences, engineering, and beyond. Washington, DC: The National Academies Press; 2014. https://doi
.org/10.17226/18722. [PubMed: 24830066] - NSB and NSF (National Science Board and National Science Foundation). 2018 science & engineering indicators—Digest. 2018. [October 8, 2019]. https://www
.nsf.gov/statistics /2018/nsb20181 /assets/1407/digest.pdf. - Ochoa TT. Origins and meanings of the public domain. University of Dayton Law Review. 2002;28:215. [October 14, 2019]; https:
//digitalcommons .law.scu.edu/facpubs/80. - OECD (Organisation for Economic Co-operation and Development)/Eurostat. Oslo manual 2018: Guidelines for collecting, reporting and using data on innovation. 4th ed. Paris, France: OECD Publishing; 2018. doi: 10.1787/9789264304604-en.
- OMB (Office of Management and Budget). Report to Congress on Implementation of Section 6002 of the Resource Conservation and Recovery Act (RCRA); Section 9002 of the Farm Security and Rural Investment Act of 2002; and Section 9002 of the Agricultural Act of 2014. 2017. [October 14, 2019]. https://www
.whitehouse .gov/sites/whitehouse .gov/files/omb/procurement /reports/2017_rcra_report.pdf. - OSTP (Office of Science and Technology Policy). Coordinated framework for regulation of biotechnology; announcement of policy and notice for public comment. Federal Register. 1986;51(123):23302–23309. https://ww
.govinfo.gov /content/pkg/FR-1986-06-26 /pdf/FR-1986-06-26.pdf. [PubMed: 11655807] - OSTP. Modernizing the regulatory system for biotechnology products. Memorandum for Heads of Food and Drug Administration, Environmental Protection Agency, and Department of Agriculture; Jul 2, 2015. [October 30, 2019]. https://www
.epa.gov/sites /production/files /2016-12/documents /modernizing_the_reg _system_for_biotech_products_memo_final .pdf. - Parks S, Ghiga I, Lepetit L, Parris S, Chataway J, Morgan Jones M. Developing standards to support the synthetic biology value chain. Santa Monica, CA: RAND Corporation; 2017. doi: 10.7249/RR1527.
- Powles J, Hodson H. Google DeepMind and healthcare in an age of algorithms. Health and Technology. 2017;7(4):351–367. doi: 10.1007/s12553-017-0179-1. [PMC free article: PMC5741783] [PubMed: 29308344]
- Qian H, Yao X. The role of research universities in U.S. college-town entrepreneurial ecosystems. 2017. doi: 10.13140/RG.2.2.12370.15043.
- Reiser L, Berardini TZ, Li D, Muller R, Strait EM, Li Q, Mezheritsky Y, Vetushko A, Huala E. Sustainable funding for biocuration: The Arabidopsis Information Resource (TAIR) as a case study of a subscription-based funding model. Database: The Journal of Biological Databases and Curation. 2016:baw018. doi: 10.1093/database/baw018. [PMC free article: PMC4795935] [PubMed: 26989150]
- Rey D. Y Combinator. Sep 11, 2018. [September 3, 2019]. There are now 141 bio companies funded by YC. https://blog
.ycombinator .com/there-are-now-141-bio-companies-funded-by-yc. - Santos R, Melo RA. Global shortage of technical agars: Back to basics (resource management). Journal of Applied Phycology. 2018;30(4):2463–2473. [September 3, 2019]; https://link
.springer .com/article/10.1007/s10811-018-1425-2. [PMC free article: PMC6096785] [PubMed: 30147238] - Schmidt C. Synbiobeta. Apr 10, 2019. [September 3, 2019]. Meet the 25 synthetic biology companies that raised $652M this quarter. https://synbiobeta
.com /meet-the-25-synthetic-biology-companies-that-raised-652m-this-quarter. - Sherkow JS. Protecting products versus platforms. Nature Biotechnology. 2016;34:462–465. [PubMed: 27153268]
- Smart RD, Blum M, Wessler J. Trends in approval times for genetically engineered crops in the United States and the European Union. Journal of Agricultural Economics. 2016;68:182–198. [October 18, 2019]; https:
//onlinelibrary .wiley.com/doi/abs/10 .1111/1477-9552.12171. - Spatial Foresight, SWECO, ÖIR, t33, Nordregio, Berman Group, and Infyde. Mapping of EU member states’/regions’ research and innovation plans & strategies for smart specialisation (RIS3) on bioeconomy for 2014–2020. DG Research & Innovation, European Commission; Brussels: 2017. [October 3, 2019]. Bioeconomy development in EU regions. https://ec
.europa.eu /research/bioeconomy /pdf/publications/bioeconomy _development_in_eu_regions.pdf. - Stephens ZD, Lee SY, Faghri F, Campbell RH, Zhai C, Efron MJ, Iyer R, Schatz MC, Sinha S, Robinson GE. Big data: Astronomical or genomical. PLoS Biology. 2015;13(7):e1002195. [PMC free article: PMC4494865] [PubMed: 26151137]
- Synbiobeta. Synthetic biology annual investment report. 2018. [September 3, 2019]. https://synbiobeta
.com /reports/investment-report-2018. - Thompson C, Sanchez J, Smith M, Costello J, Madabushi A, Schuh-Nuhfer N, Miranda R, Gaines B, Kennedy K, Tangrea M, Rivers D. Improving undergraduate life science education for the biosciences workforce: Overcoming the disconnect between educators and industry. CBE—Life Sciences Education. 2018;17(3) [October 18, 2019]; https://www
.lifescied .org/doi/full/10.1187/cbe .18-03-0047?url_ver=Z39 .88-2003&rfr_id=ori %3Arid%3Acrossref .org&rfr_dat =cr_pub%3Dpubmed&. [PMC free article: PMC6234802] [PubMed: 30142049] - Torrance AW, Kahl L. Bringing standards to life: Synthetic biology standards and intellectual property. Santa Clara High Technology Law Journal. 2014;30(2) [October 14, 2019]; https://ssrn
.com/abstract=2426235. - UniProt Consortium. UniProt: A hub for protein information. Nucleic Acids Research. 2015;43:D204–D212. [PMC free article: PMC4384041] [PubMed: 25348405]
- UniProt Consortium. UniProt: The Universal Protein Knowledgebase. Nucleic Acids Research. 2017;45:D158–D169. [PMC free article: PMC5210571] [PubMed: 27899622]
- UniProt Consortium. UniProt: A worldwide hub of protein knowledge. Nucleic Acids Research. 2019;47:D506–D515. [PMC free article: PMC6323992] [PubMed: 30395287]
- Uyarra E, Flanagan K. Understanding the innovation impacts of public procurement. European Planning Studies. 2010;18(Issue 1) doi: 10.1080/09654310903343567.
- Venter JC, Adams MD, Myers EW, Li PW, Mural1 RJ, Sutton GG, Smith HO, Yandell M, Evans CA, Holt R. The sequence of the human genome. Science. 2001;291(5507):1304–1351. [PubMed: 11181995]
- Walsh JP, Cho C, Cohen WM. View from the bench: Patents and material transfers. Science. 2005;309(5743):2002–2003. [PubMed: 16179461]
- WEF (World Economic Forum). The Global Competitiveness Report 2018. 2018. [October 18, 2019]. http://reports
.weforum .org/global-competitiveness-report-2018. - Westbrook JD, Burley SK. How structural biologists and the protein data bank contributed to recent FDA new drug approvals. Perspective. 2019;27(2):211–217. doi: 10.1016/j.str.2018.11.007. [PMC free article: PMC7325526] [PubMed: 30595456]
- White House. The National Bioeconomy Blueprint. 2012. [September 3, 2019]. https:
//obamawhitehouse .archives.gov/sites /default/files/microsites /ostp/national _bioeconomy_blueprint_april_2012.pdf. - White House. The U.S. STEM strategy. 2018. [September 3, 2019]. https://www
.whitehouse .gov/wp-content/uploads /2018/12/STEM-Education-Strategic-Plan-2018.pdf. - White House. Executive order on modernizing the regulatory framework for agricultural biotechnology products. 2019. [September 3, 2019]. https://www
.whitehouse .gov/presidential-actions /executive-order-modernizing-regulatory-framework-agricultural-biotechnology-products. - Wince-Smith D. Medium. Feb 14, 2017. [September 3, 2019]. Universities are wellsprings of innovation, drivers of regional economies. https://blog
.thegfcc .org/universities-are-wellsprings-of-innovation-drivers-of-regional-economies-8a3c097e6cc. - Zhou X, Taylor MP, Salouros H, Prasad S. Authenticity and geographic origin of global honeys determined using carbon isotope ratios and trace elements. Scientific Reports. 2018;8 [August 1, 2019]; https://www
.nature.com /articles/s41598-018-32764-w. [PMC free article: PMC6168535] [PubMed: 30279546]
Footnotes
- 1
- 2
- 3
- 4
Examples include the Advanced Regenerative Manufacturing Institute (BioFabUSA), the National Institute for Innovation in Manufacturing Biopharmaceuticals, the Advanced Robotics for Manufacturing Institute, and Manufacturing Times Digital.
- 5
See www
.fnih.org. - 6
Bayh–Dole Act—Patent and Trademark Law Amendments Act (P.L. 96-517, December 12, 1980).
- 7
The Q3 2019 Global Venture Capital Report, 07 October 2019, https://news
.crunchbase .com/news/the-q3-2019-global-venture-capital-report-seed-stage-deals-increase-while-broaderfunding-environment-shows-signs-of-erosion. - 8
Synthetic Biology Investment Report 2019 Q2, 17 July 2019, https://synbiobeta
.com /wpcontent/uploads /2019/07/Synthetic-Biology-Investment-Report-2019Q2-SynBioBeta.pdf. - 9
Title 17 of the U.S. Code—Copyrights.
- 10
35 U.S.C. § 102.
- 11
35 U.S.C. § 103.
- 12
- 13
Colleen V. Chien, Comparative Patent Quality and the Prior Art Gap, guest post 01 October 2019, https://patentlyo
.com /patent/2019/10/comparative-patent-quality.html. - 14
35 U.S.C. § 157 (pre-Leahy–Smith America Invents Act) Statutory invention registration.
- 15
- 16
- 17
According to the 2017 Update to the Coordinated Framework: “Examples of TSCA [Toxic Substances Control Act] applications include intergeneric microbial biotechnology products for biomass conversion for chemical production; microbial fuel cells; mining and resource extraction; building materials; waste remediation and pollution control; non-pesticidal agriculture applications such as bio-fertilizers; weather and climate modification; various consumer products and all other applications of intergeneric microbial biotechnology products not otherwise excluded under TSCA” (EOP, 2017, p. 13).
- 18
- 19
- 20
- 21
See www
.igem.org. - 22
- 23
- 24
Convergence has been defined as “an approach to problem solving that cuts across disciplinary boundaries. It integrates knowledge, tools, and ways of thinking from life and health sciences, physical, mathematical, and computational sciences, engineering disciplines, and beyond to form a comprehensive synthetic framework for tackling scientific and societal challenges that exist at the interfaces of multiple fields. By merging these diverse areas of expertise in a network of partnerships, convergence stimulates innovation from basic science discovery to translational application” (NRC, 2014, p. 1).
- 25
The exome encompasses those parts of a genome that contain the regions (or exons) of genes that encode proteins.
- 26
- 27
- 28
- 29
- 30
- 31
Ginkgo Bioworks was the first biotechnology company within the Y Combinator incubator. By valuation, it is currently among the top 20 Y Combinator companies (https://www
.ycombinator.com/topcompanies), which helps to illustrate the current climate of investor interest in biotechnology. - 32
See https://indiebio
.co. - 33
See https://qb3
.org. - 34
Midwest as defined by Petroleum Administration for Defense Districts.
- 35
- 36
- 37
- 38
- 39
Molino and colleagues (2018) have produced a comprehensive analysis of global second-generation biofuel production plant technologies arranged according to TRL.
- 40
- 41
- 42
- THE ECOSYSTEM OF THE U.S. BIOECONOMY - Safeguarding the BioeconomyTHE ECOSYSTEM OF THE U.S. BIOECONOMY - Safeguarding the Bioeconomy
- PPP1R16B [Emydura macquarii macquarii]PPP1R16B [Emydura macquarii macquarii]Gene ID:137157172Gene
Your browsing activity is empty.
Activity recording is turned off.
See more...