All Assay Guidance Manual content, except where otherwise noted, is licensed under a Creative Commons Attribution-NonCommercial-ShareAlike 3.0 Unported license (CC BY-NC-SA 3.0), which permits copying, distribution, transmission, and adaptation of the work, provided the original work is properly cited and not used for commercial purposes. Any altered, transformed, or adapted form of the work may only be distributed under the same or similar license to this one.
NCBI Bookshelf. A service of the National Library of Medicine, National Institutes of Health.
Markossian S, Grossman A, Arkin M, et al., editors. Assay Guidance Manual [Internet]. Bethesda (MD): Eli Lilly & Company and the National Center for Advancing Translational Sciences; 2004-.
Abstract
Reporting experimental and assay results can occur in many different settings, including informal laboratory meetings, technical reports, collaborative interactions, updates to management groups and presentations at professional conferences. In order to convey the intended message and make a lasting impact, the data presented must be clear to the observer or reader. Understanding key concepts and methods for reporting data is also critical to preserve scientific findings. This chapter describes some basic guidelines for reporting non-clinical data with an emphasis on standard elements of graphs and tables and the use of these tools to describe data most appropriately. Several fundamental statistical and numerical descriptions such as significant digits, replicates, error and correlations are also included, as they constitute an integral part of communicating results. These guidelines form the foundation for non-clinical data reporting mechanisms, such as laboratory notebooks and reports. While these guidelines are general in nature and may not be inclusive of the requirements for publication within specific journals, they should provide a solid basis for reporting non-clinical data, independent of the presentation venue.
Abbreviations
AGM | Assay Guidance Manual |
AUC | area under the curve |
CRC | concentration-response curve |
CV | Coefficient of Variation |
EC50 | half-maximal effective concentration (relative or absolute, see AGM chapters on Assay Operations and Glossary for further definitions) (1-3) |
HTS | high-throughput screening |
LLOQ | lower limit of quantitation |
Log | Log base 10 or Log10 |
LsA | limits of agreement |
MR | mean ratio |
n | number of replicates |
pEC50 | negative log EC50 |
PK | pharmacokinetics |
PMCC | product moment correlation coefficient |
r | Pearson’s correlation coefficient (equivalent to linear correlation coefficient) |
ρ | correlation coefficient |
SD | standard deviation |
SE | standard error |
SEM | standard error of the mean |
ULOQ | upper limit of quantitation |
Overview
Representation of data in graphs and tables is a key part of any scientific experiment. Creating appropriate figures requires familiarity with constructing them as well as knowledge of the data. The ability to convey results with clarity and accuracy does not require special skills but following some common guidelines can be very helpful in describing the data. This chapter outlines steps to make figures an effective communication tool for the scientist with a focus on assay development and high-throughput screening (HTS) applications. Certain figure types, such as flow charts and process diagrams, are not discussed in this chapter.
When considering graphs or tables for publications, grants, regulatory reports, etc. consult with the appropriate journals or technical documentation available for any specific requirements.
The main types of graphs for summarizing scientific data that are used for assay development or the HTS field include bar graphs, line graphs, scatter plots, frequency distributions, scatter box plots and heat maps. Other graph types such as spider or radar plots, pie charts, Pareto diagrams, and area charts are used less frequently and are not specifically discussed in this chapter.
Allocating most of the “graph ink” to data and minimizing extraneous ”chart junk” should be the goal of any effective graph (4). With that in mind, the main elements of many graphs include a title, axis scale with tick marks, axes labels, data, and a symbol key. When the graph is to be used in a printed format, a caption or legend and footnotes may be added. These graph components, and how to make better graphs, have been described in the literature (5-9). In addition, several books are often cited when describing graphing methods (4,10). At least one paper offers a five principal tutorial on how to visualize data (11). This chapter highlights some of the key graphing basics and considers graphs that may be used during everyday informal presentations of non-clinical data. In addition, tables are also briefly discussed.
Proper statistical treatment of the data is essential and consulting with a statistician familiar in assay development and HTS applications is highly recommended. Otherwise it is the author’s responsibility to ensure that the statistical methods support the types of conclusions being drawn and that the statistical methods are appropriate to the data based upon data type, distribution (e.g. normal vs. log-normal), and study design. More details are available in the statistics chapters of the Assay Guidance Manual (12).
Before creating graphs and tables, one should understand the numerical and statistical concepts described below. These concepts have a pivotal role in conveying data efficiently and effectively.
Rounding to Decimal Places
During the collection and manipulation of primary data, data should not be rounded until the end, when one wishes to summarize and report the findings in a table, etc. When rounding to decimal places, numbers are rounded by removing digits from the rightmost, farthest side, or the value which falsely suggests a high degree of precision. When the digit to be removed (immediately to the right of the rounding digit) is 0, 1, 2, 3 or 4, the rounding digit is “rounded down”. Similarly, when the digit to be removed is 5, 6, 7, 8 or 9, the preceding rounding digit is “rounded up”. When eliminating multiple digits from the right of a rounded digit, round from the rightmost digit to the left, ensuring to capture any influence rounding carryover from preceding digits. See Table 1 for rounding examples which include these carryover conventions.
Table 1.
Rounding examples from thousands to three decimal places
Rounding the Whole Number to: | Rounding the Decimal Number to: | ||||||
---|---|---|---|---|---|---|---|
Result | Thousand | Hundreds | Tens | Ones | Tenths | Hundredths | Thousandth |
1234.1234 | 1000 | 1200 | 1230 | 1234 | 1234.1 | 1234.12 | 1234.123 |
2345.2345 | 2000 | 2300 | 2340 | 2345 | 2345.2 | 2345.24 | 2345.235 |
3456.3456 | 3000 | 3500 | 3460 | 3456 | 3456.4 | 3456.35 | 3456.346 |
4567.4567 | 5000 | 4600 | 4570 | 4567 | 4567.5 | 4567.46 | 4567.457 |
5678.5678 | 6000 | 5700 | 5780 | 5679 | 5678.6 | 5678.57 | 5678.568 |
Although the authors subscribe to the common rounding rule which “rounds down” for digits less than 5 and “rounds up” for digits greater than 5, there are alternative “odd-even” rounding strategies for summarized values that have been published (13).
Rounding to Significant Digits
The number of significant digits or figures that are used to display a value is distinct from the number of decimal places that are used when expressing numbers. Demonstrating numerical expression consistency in tables, figures, legends, etc. is important when describing results and aids in the reporting and understanding of variability.
The following basic rules are used for rounding with significant digits:
- 1.
Non-zero digits are always significant
- 2.
A zero or zeros between two significant digits are significant
- 3.
Final or trailing zeros in a number with no decimal are not significant (e.g. 1020)
- 4.
Leading zeros in the decimal portion of a number are not significant; whereas final trailing zeros in the decimal portion of a number are significant
Expressing numbers using scientific notation can provide a useful method to demonstrate the number of significant digits associated with a value. As shown in Table 2, the defined digits which are located before the exponent part of the scientific notation expression are significant:
Table 2.
Three significant digits for numbers viewed with scientific notation
Number | Scientific Notation | # Significant Digits | Significant Digits |
---|---|---|---|
1020 | 1.02 x 103 | 3 | 1,0,2 |
102 | 1.02 x 102 | 3 | 1,0,2 |
10.2 | 1.02 x 101 | 3 | 1,0,2 |
1.02 | 1.02 x 100 | 3 | 1,0,2 |
0.102 | 1.02 x 10-1 | 3 | 1,0,2 |
0.0102 | 1.02 x 10-2 | 3 | 1,0,2 |
0.00102 | 1.02 x 10-3 | 3 | 1,0,2 |
As shown in Table 3, the number of significant digits for result values of different magnitudes (from 1 to 4), is depicted.
Table 3.
The number of significant digits from one to four for several examples.
Result with indicated # of Significant Digits | |||||
---|---|---|---|---|---|
Result | Scientific Notation | 1 | 2 | 3 | 4 |
0.1234 | 1.234 x 10-1 | 0.1 | 0.12 | 0.123 | 0.1234 |
1.234 | 1.234 x 100 | 1 | 1.2 | 1.23 | 1.234 |
12.34 | 1.234 x 101 | 10 | 12 | 12.3 | 12.34 |
123.4 | 1.234 x 102 | 100 | 120 | 123 | 123.4 |
1234 | 1.234 x 103 | 1000 | 1200 | 1230 | 1234 |
Presenting Rounded Data in Tables
When preparing summarized data tables, all result values should be expressed with the same number of significant digits. Once the desired number of significant digits has been established for the result value, use the same number of decimal places in the variability measurement (e.g. SD, SEM) (Table 4, Data Set 3).
Consider the summary presented in Table 4 that represents typical data generated for compound testing results and the associated error. Each data set in the table is discussed below.
Data Set 1: Each Result Value and the paired statistical measurement (SEM) are expressed with the same number of decimal places, in this case three. Note that the table is cumbersome when values range several magnitudes and the number of significant digits is variable.
Data Set 2: All Result Values have the same number of decimal places (three) with the paired statistical measurement (SEM) also having the same number of decimal places (three). Once again, the number of significant digits expressed for the result value is variable with anywhere from 2-7 significant digits. Summary tables with values and error like those shown are often seen when results are obtained from a database query with a set number of decimal places for the results.
Data Set 3: All Result Values are shown with the same number of significant digits (three in this example). The paired statistical measurement (SEM) has the same number of decimal places as the result value. This is the preferred method of expression for results and error. If results are obtained from a database query, there may be some manipulation required with either the result value or the error.
Table 4.
Expression of Result Values and Error in a Data Summary Table
Data Set 1 | Data Set 2 | Data Set 3 (preferred) | ||||
---|---|---|---|---|---|---|
Each Result Value and paired SEM have the same number of decimal places | All Result Values and all paired SEM have the same number of decimal places (three) | Same number of Significant Digits for all Result Values; same number of decimal places for paired SEM | ||||
Compound | Result Value | SEM | Result Value | SEM | Result Value | SEM |
1 | 0.0410 | 0.0067 | 0.041 | 0.007 | 0.0410 | 0.0067 |
2 | 2114.4 | 101.6 | 2114.437 | 101.642 | 2110 | 102 |
3 | 2635 | 238 | 2635.146 | 238.178 | 2640 | 238 |
4 | 389.3 | 35.2 | 389.358 | 35.261 | 389 | 35 |
5 | 0.188 | 0.008 | 0.188 | 0.008 | 0.188 | 0.008 |
6 | 1430.5 | 100.2 | 1430.561 | 100.263 | 1430 | 100 |
It is common practice to express assay results in summarized tables to two or three significant digits, but this can depend on the actual or perceived error associated with the process used in generating the result values. These error statistics (SD and SEM) are discussed further in a subsequent section.
Finally, expressing results using negative log transformations (e.g. pIC50, pKi, etc.) provide consistency in keeping all result values to the same number of significant digits. The concept of using negative log-transformed result values is discussed later in this chapter.
Logarithms
The logarithm (or log) of a number is the exponent that indicates the power to which another number (the base) is raised to produce the initial number. The “common” log or base-10 log is essentially the only one used in assay and screening applications and will be discussed exclusively in this chapter. The log base-10 (Log10) of a number (n) can be described as shown in the equation below:
Equation 1. Base 10 Logarithm
where 10 is the base and a is the exponent or power to which the base is raised. For example, the base 10 log of 1000 is 3, since 10 raised to the power of 3 (or 103) is 1000. When the base is not indicated it means log base 10, by convention. Table 5 shows some log10 values of several numbers.
Table 5.
Log10 values of several numbers
Value | Log10 |
---|---|
1 | 0 |
10 | 1 |
100 | 2 |
1000 | 3 |
842 | 2.93 |
35 | 1.54 |
0.1 | -1 |
0.01 | -2 |
0.001 | -3 |
Note that the log can be positive, negative or zero. However, log 0 is undefined since no number raised to the power of another number results in zero.
The antilog is the inverse log function. For instance, the antilog of -2 is 10-2 or 0.01. Software programs such as Microsoft Excel and others easily calculate log and antilog values using built in functions.
Additional information regarding the use of log values (geomean, pEC50, etc.) can be found elsewhere within this chapter. In addition, the rounding rules described above apply to log values as well.
Statistical Descriptors/Metrics
Arithmetic Mean
The mean (referred to as arithmetic mean) is the average of all results. To calculate the mean, add up all the result values in the data set and divide the sum by the number of values (n).
Geometric Mean
Use the Geometric Mean or Geomean when averaging data that have been calculated from log-normal values. The most common result types to which this often applies are potencies, affinities or inhibition constants (e.g. EC50, IC50, Ki, etc.) that have been determined from concentration response curves. The equation to calculate the Geomean for an EC50 is shown below:
Equation 2: Geometric Mean
Median
The median is the middle value of all results in a ranked list. Half of the numbers in the data set will be above the median and half the numbers will be below the median. To calculate the median, rank order the result values in the data set (in ascending order) and determine the middle value. When there is an odd number of results, the median is the middle value. When there is an even number of results, the median is the average between the two middle values.
Examples for Arithmetic Mean, Geometric Mean and Median
The following examples serve to demonstrate the difference between and use of arithmetic and geometric means as well as median values.
The arithmetic mean and the median for three different sets of numbers are compared in Table 6:
Table 6.
Arithmetic mean and median for three sets of numbers.
Result Values | Number of Results (n) | Arithmetic Mean | Median |
---|---|---|---|
18 | 3 | 25 | 26 |
26 | |||
31 | |||
18 | 4 | 27.5 | 28.5 |
26 | |||
31 | |||
35 | |||
15 | 5 | 27 | 31 |
18 | |||
31 | |||
35 | |||
36 |
A key concept is that the mean of a data set can be influenced by outliers, whereas the median is relatively resistant to outliers and is a more robust statistic when several values exist. Consider the example shown in Table 7 where a few values cause the elevated mean.
Table 7.
Effect of an outlier on the arithmetic mean and median.
Result Values | Number of Results (n) | Arithmetic Mean | Median |
---|---|---|---|
16 | 7 | 53 | 22 |
18 | |||
20 | |||
22 | |||
45 | |||
72 | |||
180 |
Note: many of the parameters discussed in the AGM chapter on Assay Operations for SAR Support (1) refer to using the median instead of the mean.
Table 8 shows the geometric and arithmetic mean for several different sets of result values:
Table 8.
Geometric mean and arithmetic mean for different sets of result values.
Result Values | Geometric Mean | Arithmetic Mean |
---|---|---|
4, 6, 9, 10, 12 | 7.6 | 8.2 |
123, 219, 228, 198, 267 | 201 | 207 |
0.25, 0.67, 0.17, 0.46, 0.91 | 0.41 | 0.49 |
2, 2, 2, 2, 2 | 2 | 2 |
1, 10, 100 | 10 | 37 |
The geometric mean is always less than the arithmetic mean unless all the result values are the same.
Standard Deviation and Standard Error of the Mean
Two statistical quantities that are often (incorrectly) used interchangeably are SEM and SD (14,15). SD is defined as:
Equation 3: Standard Deviation
where s represents SD, X represents each data point, represents the arithmetic mean of the population, and n represents the number of data points
The SD describes the variation, or dispersion, in measurements relative to the population mean. By contrast, SEM is defined as:
Equation 4: Standard Error of the Mean
where represents SEM, s represents SD, and n represents the number of data points
The SEM describes the probability that the measured mean is different from the population mean. With increasing sample size (assuming constant deviation in measurement), the SEM will approach zero.
SD and SEM represent very different concepts. The SD describes the distribution of individual data points around a mean, while the SEM describes the precision of the mean estimate.
When presenting data, figure legends should explicitly state whether the error bars represent the SD or SEM, as well as the number and type of replicates. Since SEM is always smaller when compared to SD in replicate measurements, we find that SEM is often plotted, presumably to imply less variation in the data. SD should be plotted when trying to convey the variation in the data, while SEM should be plotted when trying to convey the differences in means. In addition, with small data sets, plotting the individual replicates tends to be the best way to demonstrate the variability in the results in a manner universally understood.
Consider the following three examples in Figure 1 for a concentration-response curve (CRC) where the same data is plotted with SD, SEM, or individual run values at each concentration:

Figure 1.
Concentration-response curve plotted with error.
A CRC (n = 3 inter-run replicates) is plotted with the error bars representing the SD (A) or SEM (B). In (C), all three independent values at each concentration are shown as data points on the graph. For A and B, the data points on the graphs are shown as the median of the independent data values from the three runs.
Confidence Interval
A confidence interval, computed from the statistics of the observed data, is an estimated range that is likely to contain the unknown parameter. For instance, the 95% confidence interval is a range of values that one can be 95% certain contains the true mean of the estimated parameter. The confidence interval for an estimated parameter is the estimate of that parameter plus or minus the quantile corresponding to the desired confidence level from the appropriate distribution (e.g., normal, t, chi-squared, etc.) times the standard error of the estimate. When the distribution is approximately normal, the confidence interval for a sample mean is given by Equation 5:
Equation 5. Confidence Interval
where is the standard error of the mean, as defined above, and is the quantile from the Student’s -distribution corresponding to the desired confidence level and sample size. For example, , for 95% confidence and a sample size of 10 (9 degrees of freedom).
Example of Methods to Plot the Same Data
An example presented in an article that was published in four separate journals shows a set of data visualized using a scatter plot, box-and-whiskers, median and quartiles, mean ± SD, mean with confidence interval (see section below) and mean with SEM (16-19). To display variability, the author suggests showing raw data, the box-and-whisker plot, median and quartiles, or mean ± SD as the most effective methods. Using these principles, Figure 2 shows all five of these plots representing data for a control compound in an assay performed over a period of time. It demonstrates the impact of each method and the resulting message about variability or error being conveyed. The scatter plot, showing all data points, is typically the preferred format, particularly with many journals.
Figure 2.
Summarized result for an assay control compound using six different methods.
The activity for an assay control compound from several different runs is plotted on the y-axis using different methods for expressing the variability. (A) Scatter plot showing all of the data values used, with the median indicated by the red line; (B) Box and whiskers plot, showing the range of data with the median indicated by the line; (C) Median and quartiles; (D) Mean with error bars (1 SD); (E) Mean with 95% confidence interval; (F) Mean with standard error of the mean. Adapted from reference (17).
Note that the box-and-whisker plot shows the distribution of a set of data by drawing a box (rectangle) that spans from the first to the third quartile, with a line at the median. The whisker on each end extends either to the most extreme data value or to a distance that is 1.5 times the interquartile range (IQR = third quartile – first quartile) from the end of the box, whichever is shorter. Data values that are more extreme than 1.5 times the IQR are plotted as individual points.
Signal-to-Noise (S/N or SNR), Signal-to-Background (S/B) and Z’-Factor
The Signal-to-Noise Ratio (SNR or S/N) can be defined as the ratio of the mean signal (s) to the standard deviation of that signal (s). Although the S/N parameter incorporates signal variability, it only evaluates one signal in the assay. Typically, assays are optimized with controls that provide biologically relevant high and low signals that together define the dynamic range. The S/N ratio evaluates only one of the two relevant signals and thus does not allow the overall assay quality to be evaluated. In Figure 3 S/N values are given for high and low assay controls in panels A-D. The S/N values are highest for the two signals in panel A due to the low variability.
Equation 6: Signal to Noise Ratio (SNR or S/N)
The S/N is the reciprocal of the coefficient of variation (CV) which is a measure of precision relative to the mean. The coefficient of variation is often expressed as percent. Percent CV values are indicated for both high and low controls in the panels of Figure 3. The high and low controls of panel A have the lowest %CV values in Figure 3 due to the low variability among data points. Alternatively, and to address the limitations noted above, S/N is sometimes defined as the ratio of the difference between high and low signals of the controls and the total variability of the signal from the controls. The “controls” here refer to whatever reflects the dynamic range of the assay signal; for example, they may be the positive control (at high concentration) and negative control (background), presence and absence of enzyme, etc.
Equation 7: Coefficient of Variation (CV)
The Signal-to-Background Ratio (S/B) is defined as the ratio of the high control mean signal (HS) to the low control mean signal (LS). While this metric is useful for describing the assay dynamic range, it does not incorporate the variability of the high (HS) or low (LS) signals. An assay with a reasonable S/B value can still be of poor quality if the variability of either signal or both are high. An example is provided in Figure 3D, which shows high variability among both high (%CV = 23) and low (%CV = 37) controls.
Equation 8: Signal to Background Ratio (S/B)
The Z’-Factor (20) is considered the best metric to describe assay quality because it incorporates the mean values of both high (HS) and low (LS) control signals as well as their variabilities (HS and LS, respectively). The Z’-factor value approaches 1 (an ideal assay) as the signal variabilities approach zero or as the dynamic range approaches ∞. An assay with a Z’-factor value above 0.5 is considered excellent. Below 0.5, the assay quality is considered progressively lower quality as the Z’-factor value approaches zero or becomes negative. Screening is essentially impossible when the Z’-factor value is less than zero. The data shown in Figure 3 panels A-D have progressively higher variability as demonstrated by the CV values. Notably, the best quality assay is shown in panel A (Z’ = 0.89), despite the modest dynamic range (S/B = 3.2). The low variability among both high and low controls is the primary driver of the excellent assay quality in this case. At the other extreme is the data in panel D, which has a Z’ value of 0, despite having an S/B value more than 2-fold higher than that of panel A.
Equation 9: Z’-Factor
Figure 3.
Commonly used metrics to describe features of assays, including S/N, CV, S/B and Z’-Factor.
Assays with varying data quality are shown with calculations of associated parameters. Solid lines represent mean signals and dashed lines represent 3 SD of the mean. (A) A high-quality assay has clear separation between high (HS) and low (LS) mean signals as well as low variability (HS and LS, respectively). Although the assay dynamic range can be considered modest (S/B = 3.2), the low variability of high and low signals (%CV = 1.4 and %CV = 3.4, respectively) results in an excellent Z’-factor value of 0.89. The low variability of the high signal results in a large S/N value of 73. (B) Despite the greater variability in the high signal (%CV = 6.5) compared to (A) that results in a lower S/N value of 15 (compared to 73), this assay has a larger dynamic range (S/B = 13), resulting in a similarly high Z’-factor value of 0.72. (C) Compared to (B), this assay has approximately 2-fold higher variability for the high signal than (%CV = 11 versus %CV = 6.5), with similar variability in the low signal. This results in lower values of S/N, S/B and Z’-factor. (D) Among the four assays, this assay has the highest variability in both high and low signals. Although the S/B value is not substantially lower than that in (C), the high variability results in low S/N and a Z’-factor near zero.
Replicates
There has been considerable focus on irreproducible research in science, identifying the issue as a crisis (21,22). Most journals have revised guidelines to address reproducibility within their respective submissions. However, there remains confusion around the definitions of basic scientific terms associated with reproducibility including replicates and repeats (23). Vaux et al. stated several fundamental principles related to statistical design with a focus on replicates and that replicates alone do not provide evidence of reproducibility (24). In addition, different disciplines (biology, chemistry, and statistics) may have different meanings for these terms, which adds to the confusion when involved in multi-functional projects or groups.
To minimize confusion and for the purposes of this chapter and the AGM, we define replicates (technical, independent and inter-run) as they apply to in vitro assay development and HTS disciplines. The definitions are followed by some specific examples. Keep in mind that these definitions may differ among various journals or funding agencies.
Technical replicates are measurements of the same sample occurring within a single run or experiment. Technical replicates can help to identify within-sample variation but are dependent replicates due to being tested under the same conditions. Technical replicates could be on the same plate or on different plates within the experiment, depending on the variability being assessed.
Independent replicates are measurements of distinct preparations of the same samples occurring within a single run or experiment. Comparison of enzyme lots or multiple batches of independently cultured and treated cell preparations are examples of independent replicates for in vitro assays. For the best estimate of between sample variations, independent replicates should be on the same plate to minimize the contribution of additional sources of error (e.g. between plate variation). If sample capacity of the plate is limited and multiple plates are required for the study, multiple independent replicates can be randomized to multiple plates with multiple technical replicates per plate.
Inter-run replicates are measurements of the same or different sample(s) across multiple runs or experiments. A compound tested in a CRC on three different days represents inter-run replicates. Each of the runs or experiments should have the same assay conditions and reagents.
Depending on the experimental design, the greater the number of measurements, the better the estimate of variation, and the better the estimate of the mean.
When presenting data, it is important to provide the number and type of replicates (24-26) and to include them in the figure legend. For written work, scientists should consider providing a statement in the experimental methods defining the nature of their technical, independent or inter-run replicates. In general, sample variation may be greater in magnitude than technical variation. The optimal type and number of replicates depends on the scientific question and the experimental methods.
In practice, the distinction between technical, independent and inter-run replicates is not always straightforward. For example, with cell-free/biochemical assays, one approach to modeling variation would be to utilize independently synthesized reagents (e.g., enzymes), though in practice this is either impractical or counter-productive (i.e., in the case of HTS where one is attempting to minimize imprecision). Steps can be taken to mitigate batch-to-batch variation in the context of large scale experiments, for example through the use of batch pooling strategies, which is described further in the AGM Chapter on Validating Identity, Mass Purity and Enzymatic Purity of Enzyme Preparations (27).
Consider the following examples describing typical assay or screening experiments and the type of replicates that would result from each format:
- Example 1. A compound is tested for inhibition of enzyme X in a 384-well microplate. Three concentration-response curves for this inhibitor are tested on the same plate, with compounds and reagents derived from the same stock solutions and tested at the same time. Variation in this experimental setup would be random, so the best description would be n = 3 technical replicates. Determine an average value at each concentration (mean or median, depending on the variability) and fit a single concentration-response curve for the entire data set. Preferably, fit all individual replicates using the curve fitting routine.
- Example 2. A compound is tested for inhibition of enzyme X in a 96-well microplate. One concentration-response curve for this inhibitor is tested on the same plate. Each well is measured ten times with a plate reader. Variation in this experimental setup would be random, so the best description would be n = 10 technical replicates. In this case, unless the detection methodology is not very robust, this would probably constitute a poor choice of replication.
- Example 3. A compound is tested for inhibition of enzyme X in a 384-well microplate. Three concentration-response curves for this inhibitor are tested on different plates, with compounds and reagents derived from the same stock solutions and tested at the same time. Variation in this experimental setup would still be random, so the best description would be n = 3 technical replicates. The most appropriate approach for data analysis is to fit each curve independently and then report the average or geomean of the potency values with variability.
- Example 4. A compound is tested for inhibition of enzyme X in a 384-well microplate. Three concentration-response curves for this inhibitor are tested, with compounds and reagents derived from the same sources, but tested on different days. These would be classified as inter-run replicates, since the same samples were tested, but in different runs. Therefore, n = 3 inter-run replicates. The most appropriate approach for data analysis is to fit each curve independently and report the mean and associated error of the results (e.g. EC50)..
- Example 5. A compound is tested for inhibition of enzyme X in a 384-well microplate. Three concentration response curves are prepared for the inhibitor. Each curve is tested with a separate lot of enzyme from Vendor Y. All three curves are run on the same day, on the same plate. This would be an example of independent replicates, n = 3 independent replicates. Including technical replicates (multiple aliquots of the same enzyme) would help to determine if between sample differences are greater than the within sample variation. Determine a single response curve for each enzyme lot.
- Example 6. A compound is tested for inhibition of enzyme X in a 384-well microplate. Three concentration response curves are prepared for the inhibitor. Each curve is prepared with a completely independent synthesis of the compound, i.e. each is a unique lot or batch. All three curves are run on the same day, on the same plate. This would be an example of independent replicates, n = 3 independent replicates. Determine a single response curve for each compound lot.
Figure 4 shows example curves that might be associated with data for technical replicates (4A), independent replicates (4B) and inter-run replicates (4C).
Figure 4.
Comparison of technical replicates, independent replicates, and inter-run replicates.
(A) Three independently-prepared concentration-response curves (from the same compound stock) were tested on the same plate within a single experiment. This represents technical replicates (n = 3). Data points are the median result values with error bars indicated as SD. (B) Enzyme progress curves for four different lots of enzyme all tested within the same experiment. This represents independent replicates (n = 4). In this example, each lot was tested only once, so there were no technical replicates associated with the data. (C) Three independent saturation-binding experiments were performed on different days. All assay conditions and reagents were identical for each of the three runs. Within each run, there were three replicates for each concentration tested. This example represents inter-run replicates (n = 3) with n = 3 technical replicates within each run. Error bars represent SD.
There are no standard guidelines for how to treat technical versus independent replicates, and in practice it will depend on the scientific question and sources of error. In a system with both technical and independent replicates, if there is significant biological error, then it may be useful to analyze independent replicates (with any associated technical replicates) separately. In such a case of significant biological error, the source should be investigated or explicitly addressed.
A related concept is the number of independent experiments or inter-run replicates required to understand the variation of a process or an assay, which has been described for assay validation studies (28). Again, the definition of a truly “independent” experiment may not always be straightforward. Independent experiments are typically performed on separate days using reagents that originate from the earliest possible source (enzyme stock, cell line stock, etc.).
There is not a consensus for the number of individual technical/independent replicates or independent experiments (inter-run replicates) required to understand the variation of a process or an assay. Several factors (time, reagent cost, etc.) may limit the number of practical replicates that can be conducted. In addition, journals may have their own guidelines on the number of replicates required for acceptable publications. Consider these factors with any study and consult a statistician to ensure an adequate level of replication.
Be cautious when terms such as “duplicate”, “triplicate” or “quadruplicate” measurements are being used and be sure that the meaning of these terms is clearly understood. It can be very confusing when reading figure legends in publications, since there are so many variations on how to write up technical replicates, independent replicates and inter-run replicates, etc. Some examples from a few issues of the same journal are shown below. These examples represent the difficulty in interpretation that can happen when there are no standards or consistency.
- Plotted is the mean of triplicate reactions
- Data points represent the mean of triplicate measurements
- Data are mean ± SEM for 16 replicates
- Data are expressed as means ± SD of a representative experiment performed in quadruplicate out of three independent experiments.
- Data points represent the mean ± SD (n = 3) of three independent experiments.
- Shown are representative figures for n = 3 independent experiments
- Data shown are the mean ± SD; n = minimum of 2 wells
- Data points represent the mean and standard deviation of three independent replicates.
These definitions and guidelines presented for technical replicates, independent replicates, and inter-run replicates may help in interpreting and understanding data. It is important to provide additional information on replicates such as whether the replicates came from the same or independent sample stock, plates, experimental runs or from multiple runs. For example, simply stating that the “data represents 16 replicates” is not useful. An example of a representative legend can be found in the Figure Legends section of this chapter. In addition, a couple of direct examples from a journal that has figure legends that fully describe the data points and error in graphs are shown below:
- Concentration-response curves, fitted according to the Hill equation, are shown for three technical replicates from the same assay plate. Error is represented by SD.
- Results are expressed as the geometric mean of 5 independent measurements all made on separate days. Error = SD.
Random and Systematic Error
This section describes random and systematic error. Understanding the type of error that may exist in an assay is an important counterpart to experiment replication and the assessment of variability in an assay.
Random errors are unpredictable and have no defined pattern. Random errors are fluctuations that are not replicated in subsequent experiments. Sources of random error may include the precision limitations of detection or liquid handing instrumentation, changing temperatures in a laboratory and fluctuations in the assay methods. An example of random error is measuring the same sample in an instrument with three different result values. As described above, technical or biological replicates can provide an estimate of variation for understanding random error within an assay. Another example of random error occurs when measuring radioactive experiments such as scintillation proximity assays or SPA as described in the AGM chapter Receptor Binding Assays for HTS and Drug Discovery (29). The variability associated with random error from radioactivity can be reduced by increasing the read time (30).
Systematic errors are persistent (unless addressed) and can be associated with instrumentation, technique or the experimental design itself. An improperly calibrated instrument can lead to constant variation. Often systematic error results in values that are proportional or scaled to the true value. Examples of systematic error in HTS can include an incorrect wavelength setting/emission filter on a detector, faulty liquid and compound dispensing instrumentation, positional effect within a detector (31), time drift in a stack of microplates being read, and edge effects associated with evaporation. Systematic errors may be difficult to identify, but a thorough knowledge of equipment and the experimental methods being used are a critical part of detecting and minimizing the effects. One group has written extensively about minimizing the impact of systematic errors in HTS data (32-35) including those associated with assay-specific and plate-specific spatial biases (36).
Some examples of plate drift and spatial effects, which are systematic errors, are shown in the HTS Assay Validation chapter of the AGM (28).
Correlation of Data
Pearson’s Correlation
Pearson’s correlation measures the strength of the linear relationship between two sets of variables and is therefore equivalent to a linear correlation. An underlying assumption is that both variables are normally distributed (bell-shaped, symmetrical distribution of data). Only extreme or obvious departures from this assumption are problematic. Other names for Pearson’s correlation include product moment correlation coefficient (PMCC) and Pearson’s r. Pearson’s correlation returns a value (the correlation coefficient, r) between -1 and 1 where:
r = -1 indicates a perfect negative linear relationship
r = 1 indicates a perfect positive linear relationship
r = 0 indicates no linear relationship
The larger the absolute value of the correlation coefficient, the stronger the linear relationship. The meaning of the correlation coefficient size varies in the scientific literature, but one suggested range (37) is shown in Table 9.
Table 9.
Meanings for Pearson’s correlation coefficient from Reference (37).
Absolute size of correlation (positive or negative) | Interpretation of correlation |
---|---|
0.90 to 1.00 | Very high correlation |
0.70 to 0.90 | High correlation |
0.50 to 0.70 | Moderate correlation |
0.30 to 0.50 | Low correlation |
0.00 to 0.30 | Negligible correlation |
The correlation coefficient number alone is not adequate for demonstration of statistical relevance. Different patterns of Y vs X relationships can have the same correlation coefficient (11,38) as shown in Figure 5. Therefore, it is always important to plot the data for any reported correlation and consult with a statistician if the resulting correlation coefficient does not match an expected visual representation of the data.

Figure 5.
Example plots of 8 different data sets with the same Pearson correlation coefficient value (r=0.7).
All the graphs shown have Pearson correlation coefficients equal to 0.7. The example in graph 6 is the one most typically perceived when the correlation coefficient is 0.7. (Reprinted from (38) with permission.)
Spearman’s Correlation
Spearman’s correlation is a nonparametric approach to correlation, which means the variables are not assumed to have a normal distribution. It measures the strength of the rank-ordered relationship between two variables. Computationally, Spearman’s correlation is the linear correlation of the ranks of each variable. That is, the values for each variable are separately assigned ranks 1 to n, and Spearman’s correlation is calculated on the ranks. The result is a value (the correlation coefficient, ρ) between -1 and 1 and can be interpreted in a similar fashion to Pearson’s correlation shown in Table 9.
Correlation Example
This subsection describes a common analysis made in the drug discovery process using the correlation concepts described in the previous sections.
Consider the EC50 results in Table 10 for two cell-based assays associated with different species, Assay 1 and Assay 2. The goal of the analysis is to determine whether there is a correlation between the performance of the two assays, such that one assay could be used for predictive purposes over the other assay, if needed.
Table 10.
EC50 values for two cell-based assays used for correlation analysis.
EC50, nM | ||
---|---|---|
Compound | Assay 1 | Assay 2 |
1 | 0.33 | 0.29 |
2 | 0.41 | 0.74 |
3 | 0.52 | 0.24 |
4 | 0.83 | 0.42 |
5 | 1.1 | 1.2 |
6 | 1.2 | 0.68 |
7 | 1.6 | 1.9 |
8 | 2.2 | 1.2 |
9 | 2.5 | 2 |
10 | 3 | 4 |
11 | 6 | 2 |
12 | 7 | 6 |
13 | 11 | 12 |
14 | 16 | 7 |
15 | 19 | 22 |
16 | 25 | 8 |
17 | 26 | 18 |
18 | 30 | 27 |
19 | 35 | 12 |
20 | 40 | 1 |
Performing a linear regression using GraphPad Prism software with the data yields the graph in Figure 6 and the Pearson’s correlation coefficient (r = 0.62). The x-axis is a linear scale.
Figure 6.
Linear correlation using EC50 values.
EC50 values in nM (from Table 10) determined from two different CRC assays were plotted on a linear x-axis (Assay 1) and a linear y-axis (Assay 2). Shown is the regression line (solid line) determined from the analysis along with the line of identity (dashed line, defined as unity or x=y). The Pearson’s correlation coefficient is r=0.62.
Note that the data points are tightly clustered at the lower end of the regression line. Pearson’s correlation for such highly clustered data would give disproportionately higher weight to a few data points. Since the potency data was originally derived from nonlinear regression of log-transformed concentrations (x-axis), using log transformed potency values would be the correct method for determining correlations. The use of a log transformation on potency values provides a symmetric (approximately normal) distribution, and thus all points would more equally contribute to the calculated value of Pearson’s correlation. It is recommended to perform the log transformations of the potency values using the molar scale to avoid issues with negative and positive log values in the same scale. Furthermore, some scientists prefer to express the data in terms of the negative log10 EC50 using molar units, which is referred to as pEC50 in the literature. The same correlation coefficient will be obtained whether using log-transformed or pEC50 values. The data in Table 10 are now updated in Table 11 to include the results in terms of log10 EC50 (Molar) and pEC50.
Table 11.
Results for Assay 1 and Assay 2 in Table 10 converted to Log EC50 and pEC50 values.
EC50, nM | EC50, Molar | Log EC50 Molar | -Log Molar = pEC50 | |||||
---|---|---|---|---|---|---|---|---|
# | Assay 1 | Assay 2 | Assay 1 | Assay 2 | Assay 1 | Assay 2 | Assay 1 | Assay 2 |
1 | 0.33 | 0.29 | 3.3E-10 | 2.9E-10 | -9.48 | -9.54 | 9.48 | 9.53 |
2 | 0.41 | 0.74 | 4.1E-10 | 7.4E-10 | -9.39 | -9.13 | 9.38 | 9.13 |
3 | 0.52 | 0.24 | 5.2E-10 | 2.4E-10 | -9.28 | -9.62 | 9.28 | 9.62 |
4 | 0.83 | 0.42 | 8.3E-10 | 4.2E-10 | -9.08 | -9.38 | 9.08 | 9.37 |
5 | 1.1 | 1.2 | 1.1E-09 | 1.2E-09 | -8.96 | -8.92 | 8.95 | 8.92 |
6 | 1.2 | 0.68 | 1.2E-09 | 6.8E-10 | -8.92 | -9.17 | 8.92 | 9.16 |
7 | 1.6 | 1.9 | 1.6E-09 | 1.9E-09 | -8.80 | -8.72 | 8.79 | 8.72 |
8 | 2.2 | 1.2 | 2.2E-09 | 1.2E-09 | -8.66 | -8.92 | 8.65 | 8.92 |
9 | 2.5 | 2 | 2.5E-09 | 2.0E-09 | -8.60 | -8.70 | 8.60 | 8.69 |
10 | 3 | 4 | 3.0E-09 | 4.0E-09 | -8.52 | -8.40 | 8.52 | 8.39 |
11 | 6 | 2 | 6.0E-09 | 2.0E-09 | -8.22 | -8.70 | 8.22 | 8.69 |
12 | 7 | 6 | 7.0E-09 | 6.0E-09 | -8.15 | -8.22 | 8.15 | 8.22 |
13 | 11 | 12 | 1.1E-08 | 1.2E-08 | -7.96 | -7.92 | 7.95 | 7.92 |
14 | 16 | 7 | 1.6E-08 | 7.0E-09 | -7.80 | -8.15 | 7.79 | 8.15 |
15 | 19 | 22 | 1.9E-08 | 2.2E-08 | -7.72 | -7.66 | 7.72 | 7.65 |
16 | 25 | 8 | 2.5E-08 | 8.0E-09 | -7.60 | -8.10 | 7.60 | 8.09 |
17 | 26 | 18 | 2.6E-08 | 1.8E-08 | -7.59 | -7.74 | 7.58 | 7.74 |
18 | 30 | 27 | 3.0E-08 | 2.7E-08 | -7.52 | -7.57 | 7.52 | 7.56 |
19 | 35 | 12 | 3.5E-08 | 1.2E-08 | -7.46 | -7.92 | 7.45 | 7.92 |
20 | 40 | 1 | 4.0E-08 | 1.0E-09 | -7.40 | -9.00 | 7.39 | 9.00 |
The linear regressions using the Log10-transformed EC50 values (Molar) or the pEC50 values from Table 11 are shown in Figures 7A and 7B.
Figure 7.
Linear correlation using Log10 transformed EC50 and pEC50 values.
(A) EC50 values (Molar) determined from two different CRC assays (Table 11) were Log10-transformed and plotted on a log-transformed linear x-axis (Assay 1) and a log-transformed linear y-axis (Assay 2). (B) A similar plot was created using pEC50 values (-Log Molar) for Assay 1 and Assay 2. Shown in both plots are the linear regression line (solid line) and the line of identity agreement, x=y (dashed line). Note that the correlations are mirror opposites, since the pEC50 (Plot B) is the -Log Molar EC50 (Plot A). The Pearson’s correlation coefficient is 0.83 in both plots.
The Pearson’s correlation coefficient for this example is now 0.83, and adequately displays the linear relationship between the two assays, since the data points are more evenly dispersed across the data range on the log-transformed linear scale.
To determine Spearman’s correlation coefficient for this example, the compound result values (either EC50 or log transformed) are ranked for each assay from one-to-n. The analysis can be performed in GraphPad Prism, JMP, or another suitable statistics software. Figure 8 shows a plot of the ranks for each assay. Spearman’s ρ is 0.80 for this data set.
Concordance Correlation Coefficient
Another parameter that will be useful to evaluate and report for comparing variables that are expected to yield identical results is the Concordance Correlation coefficient (39). This correlation measures the degree of agreement between the values of two variables in relation to the 45-degree line (line of agreement). Mathematically, it is approximately equivalent to first evaluating the Pearson’s correlation, which measures the degree of closeness of the values to the best straight line (least-squares regression line), and then penalizing this correlation value based on the degree of the departure of this best straight line from the 45-degree line. Therefore, the Concordance Correlation value can never be greater than the Pearson’s correlation value. This calculation is also available in the Replicate-Experiment analysis Excel template referenced below.
Agreement Between Two Variables
If the interest is more in assessing the agreement between two variables, then the Bland-Altman method (40) demonstrated in the Replicate-Experiment template available in the Replicate-Experiment Study section of the AGM chapter on HTS Assay Validation (28) could be used, followed by correlation assessments. Examples of such scenarios include comparison of two assays that are expected to give similar results, comparison of results from the same assay from different laboratories or assay reagents. From this analysis, additional information such as the limits of agreement (LsA), mean ratio (MR), minimum significant ratio (MSR), and other statistical parameters are estimated.
The Replicate-Experiment analysis for the data in Table 10 is shown in Figure 9.
P-values
In 2016, the American Statistical Association published an official statement on p-values (41). A list of the key principles from that statement is shown below:
- P-values can indicate how incompatible the data are with a specified statistical model.
- P-values do not measure the probability that the studied hypothesis is true, or the probability that the data were produced by random chance alone.
- Scientific conclusions and business or policy decisions should not be based only on whether a p-value passes a specific threshold.
- Proper inference requires full reporting and transparency.
- A p-value, or statistical significance, does not measure the size of an effect or the importance of a result.
- By itself, a p-value does not provide a good measure of evidence regarding a model or hypothesis.
We refer the reader to the main article and a discussion article for further information (41,42).
A key topic related to p-values is multiple comparisons or multiple testing. When multiple groups from the same experiment are compared pairwise or in relation to a control group, the unadjusted p-values can overstate the overall significance. Appropriate adjustments should be made using methods such as Bonferroni, Dunnett, or Tukey depending on what is being compared (i.e., comparisons vs. a control group or all possible pairwise comparisons between groups) (42). In situations with a very large number of comparisons, such as with ‘omics data, the False Discovery Rate (FDR) method is often recommended (43).
Calculation of Upper and Lower Quantitation Limits
Assays where the analyte concentration levels are calibrated from a reference standard curve entail special statistical considerations and evaluation of additional parameters during the development, optimization and validation, as explained in the Immunoassay Methods chapter in this manual (44). This includes accuracy, intermediate precision, sensitivity, dynamic range with the lower and upper quantitation limits, dilution linearity, parallelism, stability, etc. The lower limit of quantitation (LLOQ) and upper limit of quantitation (ULOQ) are defined as the lowest and highest concentrations of the analyte that can be reliably measured by the assay based on pre-specified criteria around accuracy, imprecision and sometimes total error (see Table 8 in the Immunoassay Methods chapter of the AGM (44)). Accuracy is typically defined in terms of percent relative bias of the measured analyte concentration from the calibration curve relative to its spiked nominal level. Imprecision (also referred to simply as precision) is defined by the percent coefficient of variation. Total error is defined as the sum of the absolute value of relative bias plus the imprecision (%CV). The criteria for total error, accuracy, and imprecision vary on a case by case basis. For example, accuracy and imprecision are typically expected to be < 15% for PK assays of small molecules, < 20% for PK assays of large molecules, < 25 to 30% for biomarker assays. For biomarker assays, a white paper from the cross-industry working group (45) proposed the criteria for LLOQ and ULOQ to be based on 30% total error, 25% accuracy, and 25% imprecision.
As mentioned above, a Microsoft Excel-based template for the analysis of pre-study validation data for these types of assays associated with the calculation of assay performance characteristics such as accuracy, precision, sensitivity, etc., is available in the Replicate-Experiment Study section of the AGM chapter on HTS Assay Validation. An example output from this Excel-based tool is provided in Figure 10. This is a graph of the Bias, Imprecision (%CV) and Total Error for each validation sample. If the LLOQ and ULOQ are defined as the lowest and highest concentrations where the bias and imprecision are less than 25% and the total error is less than 30%, then in this example dataset, the LLOQ is 5.9 pg/mL and the ULOQ is 550 pg/mL.
Figure 10.
Profile for Total error, precision and bias.
Profile of the % relative error (bias), % imprecision (coefficient of variation; CV) and % total error for the samples used in a validation experiment. Additional assay parameters such as the sensitivity and dynamic range are derived from this analysis. For practical purposes the useful calibration range is below the 25-30% CV line.
Area under the Curve
The area under a concentration response curve or kinetic time course can be quantified and used as a metric to compare data, which is referred to as the area under the curve (AUC). This metric provides a basis for comparing data when it is not possible to determine the values of EC50 and Emax. However, even when these parameters are determined, the AUC can be preferable because it incorporates both potency and efficacy within a single metric (46). Figure 11 illustrates the concept of AUC.
Graph Elements
Title
A graph in written format typically has a figure number and a legend title (see Figure Legends section below) whereas a graph used in a presentation or discussion may have only a title. This title can provide the speaker with a visual cue, but it should be succinct so that it does not distract the listener or reader from the message being delivered, often during a short time period. Examples of succinct titles for a standard CRC like those shown previously in Figure 1 might be:
Concentration-Response Curve for Compound X
CRC for Compound X
or simply, Compound X
Much of the context for a graph in a discussion/presentation format can be provided during the presentation.
Figure Legends
Figure legends (also referred to as captions) are crucial components of scientific figures in the literature. Remember, figures and their accompanying legends should function as stand-alone material. In other words, readers should be able to interpret the overall message of the figure without having to consult the primary text. High-quality figure legends should contain the following: title, description of techniques used, summary of results, and definitions. Note that depending on the publication source, certain components may be emphasized or de-emphasized. Figure legends typically comprise 100 to 300 words in total.
Titles should be brief and either descriptive (“Inhibition of enzyme X by compound Y”) or declarative (“Compound Y is a nanomolar inhibitor of enzyme X”). The choice of descriptive versus declarative titles may depend on journal formats or author preference. For multi-panel figures, the title should encompass a common message for all of the panels.
The techniques used should be briefly described (“Inhibition of enzyme X was determined by radiolabeled substrate incorporation”) and should be minimized to only what is necessary to understand the figure. This should include the number and type of replicates as well as independent experiments, whether the results are pooled or representative and any statistical tests utilized (e.g., SD or SEM).
Depending on the nature of the data and figure, a brief statement about the key results should be included in the figure legend (“Compound Y is 5-fold more potent than the previously reported Compound Z”).
Lastly, figure legends should define any abbreviations, symbols, coloring, or scaling. Uncommon features, such as broken axes, may need to be explicitly noted (see below).
Other important miscellaneous notes:
- For multi-panel figures, it may not be possible to describe each panel in detail. In such cases, it may be most effective (and efficient) to summarize several related panels in one statement.
- Utilize consistent verb tense. Past tense is used most often for describing completed experiments (“Inhibition of enzyme X was determined by radiolabeled substrate incorporation”), while present tense can be used for declarative statements (“Compound Y is a nanomolar inhibitor of enzyme X”).
To illustrate these concepts, consider the graph in Figure 12 and several examples of a figure legend:

Figure 12.
CRC plot with three example figure legends shown below.
Lower-quality example figure legend:
Figure 12. Effect of compound X versus enzyme Y.
Better-quality example figure legend:
Figure 12. Inhibition of enzyme Y by compound X. Enzymatic activity was determined using a radiolabeled substrate assay in triplicate. Compound X inhibits enzyme Y with an IC50 value of 10 μM.
High-quality example figure legend:
Figure 12. Inhibition of enzyme Y by compound X. Enzymatic activity of enzyme Y was determined using radiolabeled substrate assay. Compound X inhibits enzyme Y with an IC50 value of 10 ± 2 μM. Data are mean ± SD from three technical replicates.
Axis Scale
Most graphs will have a single horizontal axis (x-axis) which corresponds to the independent variable and a single vertical axis (y-axis) which corresponds to a dependent variable. The axis scale can vary depending on the type of data being displayed and the same data on different scales may not convey the same message. The main types of scales used in assay and screening applications include linear (or arithmetic) or logarithmic (or log). In addition, when data is converted to log values, the data points can be plotted on a linear scale.
- Linear scale – a linear scale will show equal spacing between the scale units or tick marks. With a linear axis, the baseline often begins with zero. Log-transformed data can be plotted on a linear scale as well.
- Log scale – a log scale will have unequal spacing between scale units or tick marks. Major tick marks will have a consistent ratio between them, such as ten. By definition, logarithmic axes do not contain negative numbers. In addition, zero cannot be plotted on a logarithmic axis. To use a logarithmic scale, the actual data values are plotted.
Consider the data values from the curves in Figure 2, shown in Table 12 with the concentration (x-axis) listed in the native format (Concentration, [nM]) and in the log-transformed format (Log M).
Table 12.
CRC data with x-axis values in native and log-transformed formats.
Concentration, [nM] | Concentration, [Molar] | Log M | Replicate 1 | Replicate 2 | Replicate 3 |
---|---|---|---|---|---|
100 | 1.00E-07 | -7.00 | 97.3 | 90.5 | 96.4 |
33.3 | 3.33E-08 | -7.48 | 94.3 | 97.9 | 100.4 |
11.1 | 1.11E-08 | -7.95 | 93.3 | 94.3 | 96.6 |
3.70 | 3.70E-09 | -8.43 | 86.6 | 86.9 | 89.2 |
1.23 | 1.23E-09 | -8.91 | 72.7 | 70.3 | 81.0 |
0.412 | 4.12E-10 | -9.39 | 53.1 | 47.3 | 55.7 |
0.137 | 1.37E-10 | -9.86 | 27.0 | 31.4 | 30.0 |
0.0457 | 4.57E-11 | -10.34 | 31.4 | -3.0 | 17.2 |
0.0152 | 1.52E-11 | -10.82 | 14.1 | -4.2 | 0.5 |
0.00508 | 5.08E-12 | -11.29 | 13.7 | -1.5 | 5.8 |
The data in Table 12 can be plotted on a log scale (Figure 13A), linear scale (Figure 13B) and using log-transformed data on a linear scale (Figure 13C).

Figure 13.
CRC data plotted with three different x-axis scales.
Data from Table 12 was plotted using a log x-axis scale (A), linear x-axis scale (B) or log-transformed data on a linear x-axis scale (C). Data points for all graphs are the mean of three technical replicates. Error bars represent SD. The same nonlinear equation (4-parameter Hill equation (47)) was used for each curve fit.
Comments about each sub-figure are shown below:
Figure 13A. This figure uses the actual concentration values (Molar) with an x-axis log scale. The resulting curve fit from GraphPad Prism is ambiguous (compare the curve fit of 13A and 13C, which both use the same nonlinear curve fitting routine, but difference x-axis scales). This type of scale is not typically used with CRC data.
Figure 13B. This figure uses a linear scale for the actual concentration values. As a result, the data points on the graph will be clustered at one end of the scale and the curve fit is also ambiguous. This type of scale is seldom used with CRC data that has a concentration range over several log scales.
Figure 13C. This figure uses log-transformed concentration values on a linear scale and is the most common method for plotting CRC data. The common sigmoidal curve resulting from the nonlinear regression analysis is shown.
In a different example, consider the data in Table 13 from a standard radioligand binding saturation analysis (29). The goal of this type of experiment is to determine a plateau of binding activity that results from varying the concentration of radioligand (x-axis) and measuring the binding response (pmol/mg).
Table 13.
Data from a saturation binding analysis.
nM | Log nM | pmol/mg |
---|---|---|
0.30 | -0.516 | 0.385 |
0.53 | -0.274 | 0.587 |
0.98 | -0.008 | 0.908 |
1.7 | 0.238 | 1.38 |
2.7 | 0.432 | 1.93 |
4.4 | 0.646 | 3.13 |
6.9 | 0.842 | 3.70 |
11 | 1.05 | 4.57 |
18 | 1.26 | 5.00 |
29 | 1.47 | 5.35 |
47 | 1.67 | 5.68 |
75 | 1.87 | 5.77 |
Like Figure 13, the data in Table 13 was plotted using the concentration vales on the x-axis with a log scale (Figure 14A), a linear scale (Figure 14B) and a linear scale using log-transformed data (Figure 14C).

Figure 14.
Saturation binding data plotted with three different x-axis scales.
Data from Table 13 was plotted using a log x-axis scale (A), linear x-axis scale (B) or log-transformed data on a linear x-axis scale (C). Data points are from a single measurement at each concentration level and were fit in GraphPad Prism with nonlinear regression routines as follows: (A) and (B) using a one-site hyperbolic function; (C) using a four-parameter log inhibitor versus response function.
Figure 14A. Using the actual concentration values with an x-axis logarithmic scale can yield information about the level of saturation achieved.
Figure 14B. A linear scale is used with the actual concentration values. The binding activity does not appear to change after ~30 nM concentration on the x-axis.
Figure 14C. Log-transformed concentration values on a linear scale are possible in software programs, however, this format is seldom used.
The graph in Figure 14B is most commonly used when evaluating saturation binding experiments. However, the graph in Figure 14A may be a better example of demonstrating that the binding activity reaches a significant asymptote.
Therefore, the type of scale chosen for each graph should be carefully evaluated to ensure that information is conveyed as desired.
Axis Scale Range
In most cases, the vertical (y) axis should begin at zero. Not having the origin begin at zero can distort the relative magnitudes between data values. This concept is shown in Figure 15A (scale begins at 1.6) and Figure 15B (scale begins at zero) for the same data values.

Figure 15.
Example bar graphs with non-zero and zero y-axis origin.
(A) Y-axis scale begins at a value of 1.6 and skews the relative difference between the three samples. (B) Y-axis scale begins at zero and the relative differences between the three samples are properly depicted.
Starting the y-axis scale at zero may be preferred, but consider the example shown in Figure 16 where the same data is plotted with a y-axis scale that begins at zero (Figure 16A) and a y-axis scale that does not begin at zero (Figure 16B).

Figure 16.
Example line graphs with non-zero and zero y-axis origin.
(A) Y-axis scale begins at a value of zero; the data appears to be uniform. (B) Y-axis scale begins at a value of 3800 and a repetitive pattern is observed. This is an example of systematic error.
While this data has been exaggerated to demonstrate a point, these types of patterns can exist with assays that involve automated liquid handlers, plate transfers, and detection equipment and usually suggest a causal relationship that can be investigated. If identified, these effects can be corrected and quality control chart monitored to reduce the overall noise and variation in the assay (1). Viewing data by rows or columns with a non-zero y-axis scale may be necessary to identify potential issues or patterns.
An example where a reduced scale may be required is demonstrated in Figure 17. Here a single obvious extreme outlier (data point in red) enlarges the scale, which masks the effect of the remaining data including the variability of n=3 technical replicates. Changing the scale so that the obvious outlier is not shown may yield the desired curve. An explanation for excluding the data point should be described in a figure legend, text, orally, etc.

Figure 17.
Effect of including all data when an extreme outlier exists.
(A) One extreme outlier (in red) creates a large y-axis scale that obscures the remaining data points. (B) The extreme outlier is omitted and a standard sigmoidal CRC curve is observed compared to the previously flattened CRC shown in A (n=3 technical replicates).
Alternatively, the use of a broken axis (see Figure 19) may be an acceptable alternative to show both the expected CRC and the outlier.
Finally, two different samples that are tested in the same experiment should have the same scale range so that relative differences are obvious to the observer. Figure 18 demonstrates how conclusions could be misinterpreted when the y-axis scales for like-treated samples are not kept the same. In this example, Sample 1 and Sample 2 are two different preparations of the same protein tested in the same assay with the same conditions and reagents. Figure 18 panels A and C may give the appearance that Sample 1 and Sample 2 have similar activities, since y-axis scales, relative to each maximum, were used in the two graphs. Figure 18 panels B and D demonstrate the difference in relative activities for the two samples, when the same y-axis scale is used in each graph.

Figure 18.
The effect of using different y-axis scales on similarly treated samples.
Sample 1 and Sample 2 are two different protein preparations that were treated in the same experiment with the same reagents and conditions. Nonspecific binding (solid squares) and total binding in the absence of competitor (open circles) were measured. In A and C, the y-axis scale range is determined by the maximum observed response for each sample. In B and D, the same y-axis scale range is used for both samples. Panels B and D give an accurate representation of their relative activities compared to each other (n=1).
Therefore, the scale range should be carefully evaluated to ensure that information is conveyed appropriately. In some cases, the activity of Sample 1 should be depicted as shown in Figure 18A to indicate that there is potentially a useable signal with that sample, depending on the situation.
Generally, it is acceptable to extend the scale range a few percent on either side of the axis to avoid data points on the plot frame (if using one) or on the axis extremes.
Broken Axes
If a broken axis is used to emphasize a specific point regarding the data being plotted, a note to indicate the broken axis in the figure legend, text, discussion, etc. should be included. This alerts the observer to this non-standard technique and can prevent misinterpretation of the data. Figure 19 utilizes a broken y-axis to capture all data points of a CRC experiment (same data presented in Figure 17). It demonstrates the value of a broken axis so that key CRC information is retained while including all data points.

Figure 19.
Using a broken axis to include all data points in a CRC.
A broken y-axis is used to include an outlier while still maintaining an appropriate concentration response curve for analysis. See the graphs in Figure 17 to compare other possible ways to present this data (n=3 technical replicates).
Tick Marks
The most common placement of tick marks in a graph are on the outside of the axis; however, tick marks inside the axis are acceptable in many cases.
The number of tick marks being used on a graph axis should be chosen to represent the scale range adequately, without clutter, including extraneous tick marks that distract the reader. Placing tick marks at round integer numbers to correspond with the range is most frequently practiced. Minor tick marks and unlabeled tick marks should be avoided whenever possible.
The examples shown in Figure 20 demonstrate three different axis scales for the same data range. In Figure 20A, the tick marks are integers and evenly spaced across the scale range, which represents a desirable presentation of the axis. In Figure 20B, there are unnecessary minor tick marks included. In Figure 20C, there are too many numbered major tick marks and the labels are uneven integers.
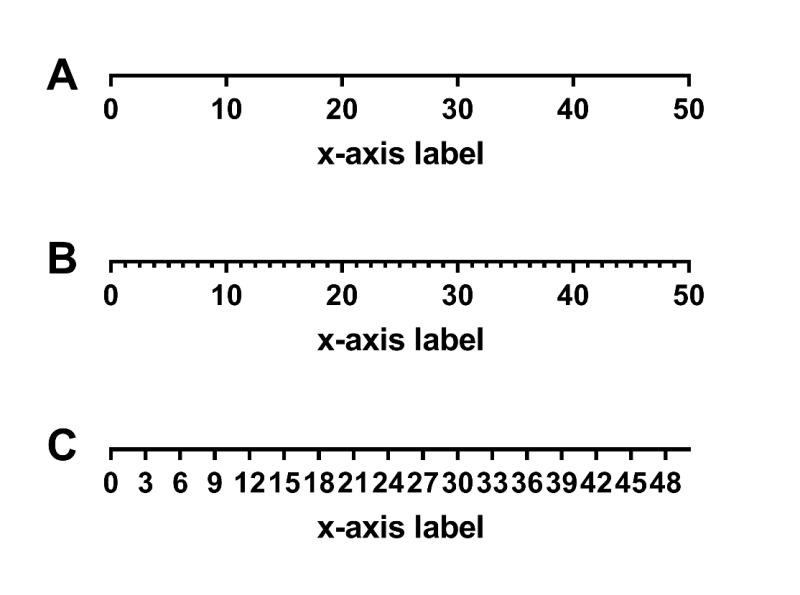
Figure 20.
The effect of the number of tick mark labels on a graph axis.
(A) A standard, visually acceptable axis with even integers spaced across the entire length of the axis. (B) This axis has unmarked minor tick marks which do not add information necessary to understand a graph. (C) This axis has too many tick mark labels and the labels are non-standard integers.
When the data values being plotted are large, resulting in axis labels with several zeros (Figure 21A), divide the numbers by a constant factor and indicate the manipulation in the axis label as shown in Figure 21B. The message and interpretation of the data is the same with the y-axis scale depicted in Figure 21B being clearer.

Figure 21.
Y-axis scale with large numbers.
Plotted are raw data values using exponential notation on the y-axis scale (A) and a transformed scale on the y-axis using a multiplier expression indicated in the x-axis label (B). In this example, data points are connected with straight lines. The connected segments imply an appropriate continuous trend between the data points.
Axes Labels
Accurate, unambiguous axes labels are important to avoid confusion regarding the data being plotted in a graph. As an example, all of the following labels were intended to represent log-transformed concentrations plotted on the x-axis. They were found within published journal articles for CRCs. Some comments are listed after the label:
Log [Compound]: Doesn’t specify the concentration units
Log [Compound] (M): Acceptable
Log [Compound], M: Acceptable
Log Compound Concentration (M): Acceptable (less standard)
Log [Compound]/M: Unclear, without further information on whether the x-axis values are a ratio
[Compound] in Log (M): Acceptable (less standard)
Log10 (Concentration (M)): Acceptable (less standard)
[Compound], M: Doesn’t specify that that the concentrations are log-transformed
Compound [Log M]: Non-standard
Log Compound, M: Suggests log of a compound name rather than a compound concentration
[Compound], Log M: Possibly suggests that the units are Log M
Compound, Log [M]: Acceptable (less standard)
This example illustrates a couple of important principles:
- 1.
Be consistent with the labels throughout a set of graphs
- 2.
Make sure that the label describes accurately what is being plotted
Y-axis labels should follow the same principles as those listed above. An important example is a scale from zero to 100 that could be a percent scale or actual data values. The label should always indicate percent if that is the intention of the data being plotted.
Types of Graphs
Many graph and figure types exist so that your data can be tailored to a specific purpose or audience, regardless of whether it is in written or spoken format. A table for choosing when to use common data presentation techniques has been previously published (48). One online source even describes 44 types of graphs that can be chosen to present information (49). This section focuses on bar graphs, line graphs, scatterplots, frequency distributions, and heat maps.
Bar Graph
Bar graphs contain horizontal or vertical bars with lengths proportional to the value of the data. A bar graph is usually used to show the relative differences between categories of data. See Figure 15 for an example.
If a bar graph has too many bars, it becomes cumbersome and can be difficult to interpret (Figure 22A). A scatterplot or heat map may be better choices for this level of data. Too few bars (Figure 22B) and the data in the plot could be displayed in a table or described within text and still be effective.

Figure 22.
Bar graphs with too many and too few values.
A representation of a bar graph with too many data points or bars (A) or too few bars (B).
With respect to bar graphs, the following is also recommended:
- Use colorblind-accessible color combinations whenever possible. Red/green pairings are most problematic but refer to ColorBrewer, an online diagnostic tool for evaluating the robustness of individual color schemes, for more information.
- Ideally, keep text on the baseline axis in the horizontal direction, without overlap.
Line Graph
A line graph is a series of data points connected by a line or line segments. The lines may be connected data points (Figure 1B), a linear regression fit (Figure 6 or Figure 7) or a nonlinear regression fit (Figure 14). For the purposes of definition, linear regression uses a linear model to determine the relationship between a dependent variable and one or more independent variables. By contrast, non-linear regression is a form of analysis modeled by a non-linear function that utilizes model parameters and one or more independent variables.
Like a bar graph, if there are too many lines the reader is distracted from the message of the data in a line graph. This is especially true if the curves being shown have similar activities (Figure 23). Figures with too many curves often force the preparer to include a legend within the graph, which can be distracting and, in many cases, is considered chart junk.
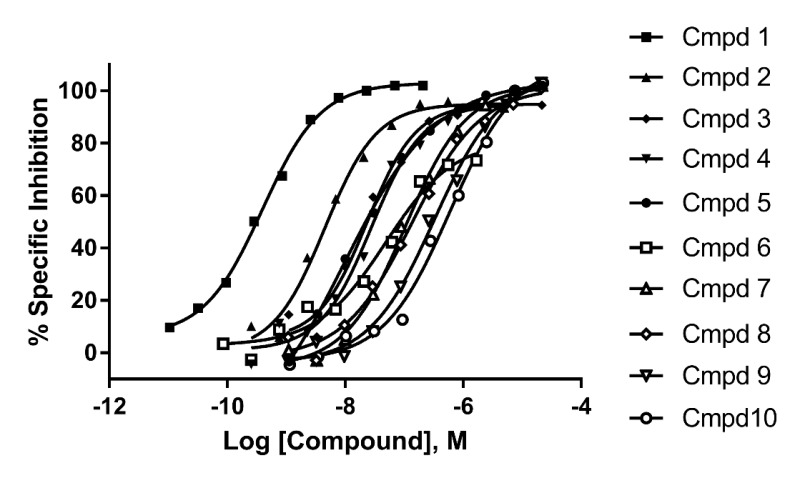
Figure 23.
Multiple compound CRC graph.
In this graph, the CRC for several compounds are shown with nonlinear regression curve fits. Each line has a unique symbol and the symbol legend appears at the right. There are too many compounds being shown on this graph to decipher the individual information.
Considerations include:
- Use colorblind-accessible color combinations whenever possible. (See above for more info.)
- Use colors only when necessary – avoid excessive use.
- The thickness of lines should be such that they are clearly visible but do not obscure individual data points.
- The method used to fit a set of data points to a line should always be conveyed with the graph (via legend, text, discussion, etc.).
In place of Figure 23, showing a representative curve for one or two compounds along with a table of calculated results (e.g. pIC50) for the other compounds tested may provide an improved approach for presenting this type of data. An example of this concept is shown in Figure 24 where the two compounds with the largest difference in activity are displayed in the graph (A) and the activity (pIC50) of all the compounds tested is displayed in the table (B).
Figure 24 represents an additional reason for using negative log-transformed activity values (pIC50) when comparing compounds. The larger the pIC50 value, the more potent the compound. Other advantages include the following: the nonlinear curve fit routine solves for the log IC50, the error associated with the pIC50 is symmetric and normally-distributed and expressed with the same level of significance (see significant digits section) as well as making the determination of the geometric means easier.

Figure 24.
CRC and table for several test compounds.
(A) Concentration response curves for two compounds in a study (n = 1). The curves represent the most potent compound (Compound 1, closed squares) and least potent compound (Compound 10, open circles) in an experiment that tested ten compounds. (B) Table showing the pIC50 values for all ten compounds tested in the experiment.
Scatter Plot
A scatter plot (also referred to as a scatter gram) is a graphic visualization of two-dimensional data using dots to represent data values. Scatter plots have x and y-axes and each data point is a coordinate on the plot. Scatterplots are often used to demonstrate the activity of the wells on a microplate, such as plate uniformity studies (28) and large data sets. In addition, they are the type of graphs used in the correlation plots discussed earlier (Figure 6 and 7).
Color coding data points can provide additional information within a scatter plot as shown in Figure 25 for plate data with the color-coded max and min plate controls. However, color-coding individual data points can be tedious and the data may be better represented with a heat map (Figure 28 and 29).

Figure 25.
Scatterplot example.
Individual well data from a 384-well plate that includes positive controls (red) and negative controls (blue). Wells are aligned by columns on the plate.
With respect to scatter graphs, the following is also recommended:
- Use colorblind-accessible color combinations whenever possible. (See above for more info.)
- Data points that are too large obscure the detail of the pattern or trend being depicted in the scatterplot.
Frequency Distribution
A frequency distribution typically uses bars or rectangles and can include a further analysis for normality, such as a Gaussian curve, embedded in the plot. The x-axis represents a range or group of ranges (“bins”) and the y-axis represents the frequency at each range or bin. An example of a frequency distribution is shown in Figure 26. In this example, the three panels demonstrate frequency distributions using an appropriate number of bins (A), too many bins (B) and too few bins (C).

Figure 26.
Frequency distribution.
Activity is divided into bins of percent specific inhibition (x-axis). The number of compounds in each bin (y-axis) are represented by the bars. To test for normality, a Gaussian distribution (red line) was fit to the frequency data. (A) Represents an appropriate number of separation bins (at 10% inhibition intervals), while (B) has too many separation bins (at 5% inhibition intervals) and (C) has too few separation bins (at 20% inhibition intervals).
With respect to frequency distributions, the following is also recommended:
- The number of bins ultimately depends on the number of data points, and determining the number of bins (as demonstrated above), may be a trial and error process to achieve a desired graphical result.
Alternative Plots
While widely used, bar graphs have significant limitations. Since bar graphs only display summary statistics (generally mean and SD or SE) it is possible to generate identical bar graphs from different data sets due to outliers, bimodal distributions, differences in sample sizes, confounding variables or other reasons (50). Alternative graphical methods which display all of the data and the distribution information, such as Univariate Scatterplots, Box plots (which graphically overlay the summary statistics) or Violin plots are preferable. Since most preclinical studies use relatively small sample groups (n < 15) this is very feasible and Weissgerber et al (51) have provided an online tool which allows scientists to easily generate and download these plots using their own data. (Figure 27).
Figure 27.
Many different distributions can lead to the same bar graph.
The full data may suggest different conclusions from the summary statistics. The means and SE values for the four example datasets shown in B-E are all within 0.5 units of the means and SE values shown in the bar graph A. ρ values were calculated in R statistical software (version 3.0.3) using an unpaired t-test, an unpaired t-test with Welch’s correction for unequal variances, or a Wilcoxon rank sum test. In B, the distribution in both groups appears symmetric. Although the data suggest a small difference between groups, there is substantial overlap between groups. In C, the apparent difference between groups is driven by an outlier. D suggests a possible bimodal distribution. Additional data are needed to confirm that the distribution is bimodal and to determine whether this effect is explained by a covariate. In E, the smaller range of values for group 2 may simply be due to the fact that there are only three observations. Additional data for group 2 would be needed to determine whether the groups are actually different. var, variance. (Adapted from Weissgerber et al. (50) under a creative commons license. Figure and figure legend used with permission.)
Heat Maps
A heat map is a representation of data values using a color scale or grayscale. The resulting graph can be in a matrix format, which makes them popular for data generated using microplates. Figure 28 shows a heat map for the same data that was previously graphed in a scatter plot (Figure 25).

Figure 28.
Heat map for the 384-well plate data shown in Figure 21.
The scale at the right of the heat map shows the colors associated with the signal range. Note that in this example, the positive controls are in wells A1-H2 and I23-P24. The negative controls are in wells I1-P2 and A23-H24.
Heat maps can be used to view multiple plates at a time and to assist with efficient identification of data patterns, position effects and trends (52).
With respect to heat maps, the following is also recommended:
- Individual data points can be framed with a thin border. Frames are typically avoided when there is a large amount of data points such that the frames would obscure the interpretation of the data.
- Heat maps can be displayed in color or grayscale. The choice of color versus grayscale often depends on the data being presented. For data with large dynamic ranges, two colors may be appropriate, whereas for data with small dynamic ranges, grayscale may be sufficient (Figure 29). As stated previously, the use of red and green coloring schemes should be avoided. (This is perhaps a relic of certain microarrays that utilized red and green fluorescent proteins to assay gene expression.)
- As with other plot formats, the scaling of the color can be adjusted to better illustrate key scientific points, such as plate positional effects where relatively minor systematic errors may be significant (Figure 29).
- Outliers can be displayed on heat maps as a separate color and then noted in the legend.
- Consider providing a supplemental file listing the data in numerical format. Data points can also be printed within each matrix point, though this can add considerable visual artifacts that detract from interpreting the figure (Figure 29E).
- The use of multiple colors (“rainbow schemes”) can make the perception of gradients difficult (Figure 29F) and are generally more appropriate for categorical or grouped data.
- Another approach to emphasizing positional effects is to express data as deviation from the mean or median values (Figure 29G). This is also amenable to analyzing data for up- or down-regulation, such as gene expression or protein abundance.
Figure 29.
Effect of color schemes on heat maps.
Data are normalized fluorescence intensity measurements from a uniformity plate (i.e., all wells should have equal, 100% max signal) to assess for microplate positional effects. Clearly, rows I, J, M, and N show decreased signal, which is due to a clogged liquid dispensing nozzle. Panels A and B demonstrate the effect of varying scales; a coloring scheme starting at the minimum value (panel B) better highlights the systematic errors in this particular data. The same data can be plotted in color (panels C and D), depending on the desired aesthetics. Individual values can be printed within each matrix point (panel E), but this generally adds noise and should be done only when necessary. The same data can be plotted in a rainbow coloring scheme (panel F), though the meaning of individual colors is not necessarily intuitive. Finally, the same data can be plotted as a function of deviation from the mean or median (panel G). (Unpublished data courtesy of JL Dahlin.)
Three-Dimensional Graphs
In most cases, 3-dimensional (3D) graphs are distracting to the reader and can be difficult to interpret due to the added complexity or distortion that can occur. They are often used in business publications and newspapers but should be avoided in scientific data presentations. A simple, classic example is shown in Figure 30 using a standard bar graph and a 3-D bar graph generated with Microsoft Excel. In the example, both samples 2 and 4 have median values above 100% of the control in the standard bar graph. However, in the 3-D bar graph, it appears that samples 2 and 4 do not reach the 100% of control level based on the axis scale lines for the graph. While this is a matter of perspective, it can lead to incorrect analysis and conclusions.
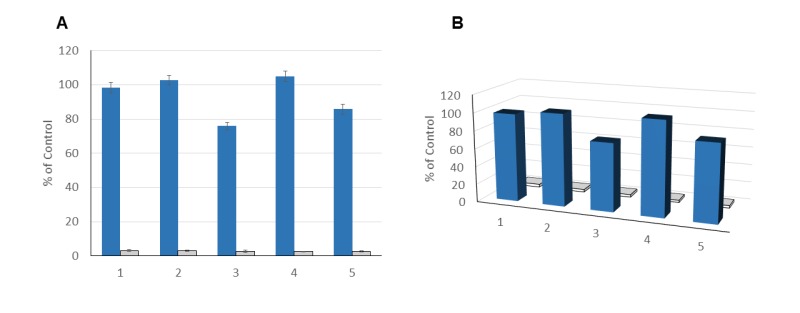
Figure 30.
Standard and 3D bar graphs.
(A) (Typical bar graph) and (B) (3-D bar graph) are from the same data. Five different samples were tested for activity against a control. Blue bars are total activity and grey bars are nonspecific activity. Bars are the median of 8 technical replicates from the same run.
Graphing/Statistical Software Programs
This chapter asserts no preference in software used for creating graphs or statistically analyzing data. Specific programs cited are at the discretion of the authors, based on experience. Some notable software programs for graphing or statistical analysis include, but are not limited to, the list below.
GraphPad Prism
JMP
KaleidaGraph
MATLAB
Microsoft Excel
Minitab
Origin
R Statistical Package
SAS
ScreenAble
SigmaPlot
SPSS
Stata
Tables
It is important to note that graphs and tables may lead to completely different interpretations, even with the same data set. Each has a purpose, depending on how the data will be used and who the consumers of the data will be. Large tables do not work well in a presentation setting and graphs may not provide the level of detail required for a further calculation or show exact values and differences when analyzing data.
Tables should not contain so much data that they are hard to follow. Likewise, tables should not have print that is so small that it is difficult to read. Conversely, simple tables with only a few values may be less effective.
Considerations for tables include:
- Include a legend/caption where needed to describe or define any abbreviations, etc.
- Include units with the table (e.g. as part of the header title for the column)
- Use a minimum number of significant digits that are consistent throughout the table
- Shading rows or columns can help improve readability
- Use lines to separate sections; do not overuse lines within the table
- Include only essential data
- Be clear, concise and legible
- There should be adequate space within the table for clarity
- Use caution with word wrapping, especially when it only occurs in a couple of table cells
Some examples of tables appear earlier in this chapter.
Interactive Data Visualization
Visual inspection of graphic displays can identify natural groupings of data points suggestive of correlations in the underlying data. Modern computer graphics interfaces often have the capability to use a computer’s mouse or other pointing device to select one or more data points in a graphic for further investigation. Some of the more commonly encountered types of these tools are discussed below.
Data Hints
The data or chart “hint” is often used to describe the action that occurs when the mouse hovers over, or is clicked on, single points (e.g. scatterplot) or specific sub-plot regions of a multi-plot display. Typically, this is used to display an informational child window near the selected point/region. An example of a chart hint is shown in Figure 31. If a data grid is visible in the application, then a useful side effect would be to select (highlight) the row of interest.

Figure 31.
The result of a chart hint action on a multi-panel CRC plot.
In this example, hovering over the curve provides a value for each of the parameters listed as a quick reference to the underlying data row contents. The availability of hints for graphics is typically indicated by a hand with pointing finger cursor.
Brushing Data
Data brushing refers to using the pointer in a click-and-drag fashion to define a region that encompasses one or more data points of interest on a chosen graphic. The selected points/region are subsequently “highlighted” (e.g. using color or plot characters) in the selection plot device in order to focus subsequent User attention. Typically, graphic plots using other variables/responses are similarly updated and the relevant data grid rows are selected. For maximal utility, this should be a two-way process and the ability to highlight graph points from row selections of data displayed in grids following complex sorting operations should also be available.
Context Menus
Many graphics displays allow the development of menu functionality for common operations such as copying, printing and file creation to be readily available to the user. The content of these menus can be programmatically linked to the type of plot and the data under consideration. Plot scaling can be keyed to mouse behavior (e.g. mouse wheel) and, for 3-dimensional graphics, useful behaviors such as rotational direction and speed can be intuitively linked to gestural pointer actions. Figure 32 demonstrates the concept of a context menu.
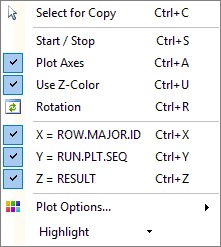
Figure 32.
Example of a Context Menu associated with a 3D graphics display.
Several options for rotational control, plotting of axes etc. can be accessed using either the pointer or keyboard shortcut combinations. Options with checkboxes are those that have an on/off toggle function.
Databases
A well designed and properly implemented Relational Database Management System (RDBMS) (53) is a valuable tool not only for the storage of information but also for promoting both reproducibility of experiments and consistent, appropriate data reporting. A database offers a number of advantages when compared to flat files such as spreadsheets:
- Data Safety and Integrity. Built in features of the database automatically back up data and track any changes made to the data. In the event of errors, changes can be rolled back
- Data Consistency. Much of the data in spreadsheets is repeated and subject to errors in data entry such as typographic errors or cut and paste errors. A structured data model eliminates this redundancy and provides tools to validate data entries. Additionally, having all the data in one place simplifies the identification of systematic shifts or random errors in the data.
- Change Management. Results can be recalculated automatically from the original primary data to explore new models or allow for changes in methodology. Explanations of any changes along with a record of when and who made the change(s) are also systematically captured.
- Data Analysis and Reporting. Structured reports ensure that data is always presented in a consistent manner with proper mathematical and statistical treatment, regardless of the sophistication of the user, while still allowing for export and exploration of the data to other tools. Best practices, such as those described in this chapter, can be incorporated into these reports. Reporting of both primary and derived data has also become more common for both publication and funding and has been demonstrated to enhance both transparency and reproducibility.
There are numerous commercial and open source implementations of RDBMS technologies. All of them feature some implementation of standardized Structured Query Language (SQL) commands for obtaining results for data reporting purposes. For maximal data integrity and control of data flow, the chosen system should also feature a procedural language for sophisticated development and implementation of the automated processes discussed above. Most commercially available Laboratory Information Management Systems (LIMS) use RDBMS technology to provide much of their functionality and represent a path to obtain the benefits of a relational database without the need to directly manage one.
References
- 1.
- Beck B, Chen YF, Dere W, Devanarayan V, Eastwood BJ, Farmen MW, et al. Assay Operations for SAR Support. In: Sittampalam GS, Gal-Edd N, Arkin M, Auld D, Austin C, Bejcek B, et al., editors. Assay Guidance Manual. Bethesda (MD)2004.
- 2.
- Devanarayan V, Sawyer BD, Montrose C, Johnson D, Greenen DP, Sittampalam GS, et al. Glossary of Quantitative Biology Terms. In: Sittampalam GS, Gal-Edd N, Arkin M, Auld D, Austin C, Bejcek B, et al., editors. Assay Guidance Manual. Bethesda (MD)2004.
- 3.
- Campbell RM, Dymshitz J, Eastwood BJ, Emkey R, Greenen DP, Heerding JM, et al. Data Standardization for Results Management. In: Sittampalam GS, Gal-Edd N, Arkin M, Auld D, Austin C, Bejcek B, et al., editors. Assay Guidance Manual. Bethesda (MD)2004.
- 4.
- Tufte ER. The Visual Display of Quantitative Information. 1st ed. Chelshire, Conneticut: Graphics Press; 1983.
- 5.
- King L. Preparing better graphs. Journal of Public Health and Emergency. 2018;2(1)
- 6.
- Boers M. Designing effective graphs to get your message across. Annals of the rheumatic diseases. 2018;77(6):833–9. [PubMed: 29748338] [CrossRef]
- 7.
- Kelleher C, Wagener T. Ten guidelines for effective data visualization in scientific publications. Environmental Modelling & Software. 2011;26(6):822–7. doi: . [CrossRef]
- 8.
- Puhan MA, ter Riet G, Eichler K, Steurer J, Bachmann LM. More medical journals should inform their contributors about three key principles of graph construction. J Clin Epidemiol. 2006;59(10):1017–22. [PubMed: 16980140] [CrossRef]
- 9.
- Rougier NP, Droettboom M, Bourne PE. Ten Simple Rules for Better Figures. PLOS Computational Biology. 2014;10(9):e1003833. [PMC free article: PMC4161295] [PubMed: 25210732] [CrossRef]
- 10.
- Cleveland WS. The Elements of Graphing Data. Summit, NJ: Hobart Press; 1985.
- 11.
- Cabanski C, Gilbert H, Mosesova S. Can Graphics Tell Lies? A Tutorial on How To Visualize Your Data. Clin Transl Sci. 2018;11(4):371-7. doi: 10.1111/cts.12554. PubMed PMID: 29603646; PubMed Central PMCID: PMCPMC6039197. [PMC free article: PMC6039197] [PubMed: 29603646] [CrossRef]
- 12.
- Haas JV, Eastwood BJ, Iversen PW, Weidner JR. Minimum Significant Ratio - A Statistic to Asses Assay Variability. In: Sittampalam GS, Gal-Edd N, Arkin M, Auld D, Austin C, Bejcek B, et al., editors. Assay Guidance Manual. Bethesda (MD)2004.
- 13.
- Blackstone EH. Rounding numbers. J Thorac Cardiovasc Surg. 2016;152(6):1481–3. [PubMed: 27726878] [CrossRef]
- 14.
- Nagele P. Misuse of standard error of the mean (SEM) when reporting variability of a sample. A critical evaluation of four anaesthesia journals. Br J Anaesth. 2003;90(4):514–6. [PubMed: 12644429]
- 15.
- Sedgwick P. Standard deviation or the standard error of the mean. Br Med J. 2015;350:h831. [PubMed: 25691433] [CrossRef]
- 16.
- Motulsky HJ. Common misconceptions about data analysis and statistics. Naunyn-Schmiedeberg's archives of pharmacology. 2014;387(11):1017-23. doi: 10.1007/s00210-014-1037-6. PubMed PMID: 25213136; PubMed Central PMCID: PMCPMC4203998. [PMC free article: PMC4203998] [PubMed: 25213136] [CrossRef]
- 17.
- Motulsky HJ. Common misconceptions about data analysis and statistics. The Journal of pharmacology and experimental therapeutics. 2014;351(1):200–5. [PubMed: 25204545] [CrossRef]
- 18.
- Motulsky HJ. Common misconceptions about data analysis and statistics. Pharmacology research & perspectives. 2015;3(1):e00093. doi: 10.1002/prp2.93. PubMed PMID: 25692012; PubMed Central PMCID: PMCPMC4317225. [PMC free article: PMC4317225] [PubMed: 25692012] [CrossRef]
- 19.
- Motulsky HJ. Common misconceptions about data analysis and statistics. Br J Pharmacol. 2015;172(8):2126-32. doi: 10.1111/bph.12884. PubMed PMID: 25134425; PubMed Central PMCID: PMCPMC4386986. [PMC free article: PMC4386986] [PubMed: 25134425] [CrossRef]
- 20.
- Zhang JH, Chung TD, Oldenburg KR. A Simple Statistical Parameter for Use in Evaluation and Validation of High Throughput Screening Assays. J Biomol Screen. 1999;4(2):67–73. [PubMed: 10838414]
- 21.
- Baker M. Is there a reproducibility crisis? Nature. 2016;533(7604):452–4. [PubMed: 27225100] [CrossRef]
- 22.
- Fanelli D. Opinion: Is science really facing a reproducibility crisis, and do we need it to? Proc Natl Acad Sci U S A. 2018;115(11):2628-31. doi: 10.1073/pnas.1708272114. PubMed PMID: 29531051; PubMed Central PMCID: PMCPMC5856498. [PMC free article: PMC5856498] [PubMed: 29531051] [CrossRef]
- 23.
- Goodman SN, Fanelli D, Ioannidis JP. What does research reproducibility mean? Sci Transl Med. 2016;8(341):341ps12. [PubMed: 27252173] [CrossRef]
- 24.
- Vaux DL, Fidler F, Cumming G. Replicates and repeats--what is the difference and is it significant? A brief discussion of statistics and experimental design. EMBO Rep. 2012;13(4):291-6. doi: 10.1038/embor.2012.36. PubMed PMID: 22421999; PubMed Central PMCID: PMCPMC3321166. [PMC free article: PMC3321166] [PubMed: 22421999] [CrossRef]
- 25.
- Bell G. Replicates and repeats. BMC Biol. 2016;14(1):28. [PMC free article: PMC4825082] [PubMed: 27055650] [CrossRef]
- 26.
- Blainey P, Krzywinski M, Altman N. Replication. Nat Methods. 2014;11:879. [PubMed: 25317452] [CrossRef]
- 27.
- Scott JE, Williams KP. Validating Identity, Mass Purity and Enzymatic Purity of Enzyme Preparations. In: Sittampalam GS, Gal-Edd N, Arkin M, Auld D, Austin C, Bejcek B, et al., editors. Assay Guidance Manual. Bethesda (MD)2004. [PubMed: 22553867]
- 28.
- Iversen PW, Beck B, Chen YF, Dere W, Devanarayan V, Eastwood BJ, et al. HTS Assay Validation. In: Sittampalam GS, Gal-Edd N, Arkin M, Auld D, Austin C, Bejcek B, et al., editors. Assay Guidance Manual. Bethesda (MD)2004.
- 29.
- Auld DS, Farmen MW, Kahl SD, Kriauciunas A, McKnight KL, Montrose C, et al. Receptor Binding Assays for HTS and Drug Discovery. In: Sittampalam GS, Coussens NP, Brimacombe K, Grossman A, Arkin M, Auld D, et al., editors. Assay Guidance Manual. Bethesda (MD)2004.
- 30.
- Kahl SD, Hubbard FR, Sittampalam GS, Zock JM. Validation of a High Throughput Scintillation Proximity Assay for 5-Hydroxytryptamine1E Receptor Binding Activity. Journal of Biomolecular Screening. 1997;2(1):33–40.
- 31.
- Brideau C, Gunter B, Pikounis B, Liaw A. Improved statistical methods for hit selection in high-throughput screening. J Biomol Screen. 2003;8(6):634–47. [PubMed: 14711389] [CrossRef]
- 32.
- Dragiev P, Nadon R, Makarenkov V. Systematic error detection in experimental high-throughput screening. BMC bioinformatics. 2011;12:25-. doi: 10.1186/1471-2105-12-25. PubMed PMID: PMC3034671. [PMC free article: PMC3034671] [PubMed: 21247425] [CrossRef]
- 33.
- Kevorkov D, Makarenkov V. Statistical Analysis of Systematic Errors in High-Throughput Screening. Journal of Biomolecular Screening. 2005;10(6):557–67. [PubMed: 16103415] [CrossRef]
- 34.
- Makarenkov V, Kevorkov D, Zentilli P, Gagarin A, Malo N, Nadon R. HTS-Corrector: software for the statistical analysis and correction of experimental high-throughput screening data. Bioinformatics. 2006;22(11):1408–9. [PubMed: 16595559] [CrossRef]
- 35.
- Makarenkov V, Zentilli P, Kevorkov D, Gagarin A, Malo N, Nadon R. An efficient method for the detection and elimination of systematic error in high-throughput screening. Bioinformatics. 2007;23(13):1648–57. [PubMed: 17463024] [CrossRef]
- 36.
- Mazoure B, Nadon R, Makarenkov V. Identification and correction of spatial bias are essential for obtaining quality data in high-throughput screening technologies. Scientific reports. 2017;7(1):11921. [PMC free article: PMC5607347] [PubMed: 28931934] [CrossRef]
- 37.
- Mukaka MM. A guide to appropriate use of Correlation coefficient in medical research. Malawi Med J. 2012;24(3):69-71. PubMed PMID: PMC3576830. [PMC free article: PMC3576830] [PubMed: 23638278]
- 38.
- Chambers JM. Graphical Methods for Data Analysis: Wadsworth International Group; Boston : Duxbury Press, Belmont, CA; 1983.
- 39.
- Lin LI. A concordance correlation coefficient to evaluate reproducibility. Biometrics. 1989;45(1):255–68. Epub 1989/03/01. [PubMed: 2720055]
- 40.
- Bland JM, Altman DG. Measuring agreement in method comparison studies. Stat Methods Med Res. 1999;8(2):135–60. [PubMed: 10501650] [CrossRef]
- 41.
- Wasserstein RL, Lazar NA. The ASA's Statement on p-Values: Context, Process, and Purpose. The American Statistician. 2016;70(2):129–33. [CrossRef]
- 42.
- Bretz F, Hothorn T, Westfall P. Multiple Comparisons Using R. New York, NY: Chapman and Hall/CRC; 2010.
- 43.
- Benjamini Y, Hochberg Y. Controlling the False Discovery Rate: A Practical and Powerful Approach to Multiple Testing. Journal of the Royal Statistical Society Series B (Methodological). 1995;57(1):289–300.
- 44.
- Cox KL, Devanarayan V, Kriauciunas A, Manetta J, Montrose C, Sittampalam S. Immunoassay Methods. In: Sittampalam GS, Coussens NP, Nelson H, Arkin M, Auld D, Austin C, et al., editors. Assay Guidance Manual. Bethesda (MD)2004.
- 45.
- Lee JW, Weiner RS, Sailstad JM, Bowsher RR, Knuth DW, O'Brien PJ, et al. Method validation and measurement of biomarkers in nonclinical and clinical samples in drug development: a conference report. Pharmaceutical research. 2005;22(4):499–511. [PubMed: 15846456] [CrossRef]
- 46.
- Fallahi-Sichani M, Honarnejad S, Heiser LM, Gray JW, Sorger PK. Metrics other than potency reveal systematic variation in responses to cancer drugs. Nature chemical biology. 2013;9(11):708. [PMC free article: PMC3947796] [PubMed: 24013279]
- 47.
- Weiss JN. The Hill equation revisited: uses and misuses. FASEB journal : official publication of the Federation of American Societies for Experimental Biology. 1997;11(11):835–41. Epub 1997/09/01. [PubMed: 9285481]
- 48.
- Franzblau LE, Chung KC. Graphs, Tables, and Figures in Scientific Publications: The Good, the Bad, and How Not to Be the Latter. The Journal of Hand Surgery. 2012;37(3):591–6. doi: . [PubMed: 22305731] [CrossRef]
- 49.
- Lile S. 44 Types of Graphs: Perfect for Every Top Industry [Accessed January 23, 2019]. Available from: https://visme
.co/blog/types-of-graphs/. - 50.
- Weissgerber TL, Milic NM, Winham SJ, Garovic VD. Beyond bar and line graphs: time for a new data presentation paradigm. PLoS Biol. 2015;13(4):e1002128. doi: 10.1371/journal.pbio.1002128. PubMed PMID: 25901488; PubMed Central PMCID: PMCPMC4406565. [PMC free article: PMC4406565] [PubMed: 25901488] [CrossRef]
- 51.
- Weissgerber TL, Savic M, Winham SJ, Stanisavljevic D, Garovic VD, Milic NM. Data visualization, bar naked: A free tool for creating interactive graphics. J Biol Chem. 2017;292(50):20592-8. doi: 10.1074/jbc.RA117.000147. PubMed PMID: 28974579; PubMed Central PMCID: PMCPMC5733595. [PMC free article: PMC5733595] [PubMed: 28974579] [CrossRef]
- 52.
- Dahlin JL, Sinville R, Solberg J, Zhou H, Han J, Francis S, et al. A cell-free fluorometric high-throughput screen for inhibitors of Rtt109-catalyzed histone acetylation. PLoS One. 2013;8(11):e78877. doi: 10.1371/journal.pone.0078877. PubMed PMID: 24260132; PubMed Central PMCID: PMCPMC3832525. [PMC free article: PMC3832525] [PubMed: 24260132] [CrossRef]
- 53.
- Relational Model. In Wikipedia, The Free Encyclopedia: Wikepedia Contributors; 2019.
- Abstract
- Abbreviations
- Overview
- Rounding to Decimal Places
- Rounding to Significant Digits
- Presenting Rounded Data in Tables
- Logarithms
- Statistical Descriptors/Metrics
- Example of Methods to Plot the Same Data
- Correlation of Data
- Agreement Between Two Variables
- P-values
- Calculation of Upper and Lower Quantitation Limits
- Area under the Curve
- Graph Elements
- Types of Graphs
- Graphing/Statistical Software Programs
- Tables
- Interactive Data Visualization
- Databases
- References
- Folic acid supplementation and malaria susceptibility and severity among people taking antifolate antimalarial drugs in endemic areas.[Cochrane Database Syst Rev. 2022]Folic acid supplementation and malaria susceptibility and severity among people taking antifolate antimalarial drugs in endemic areas.Crider K, Williams J, Qi YP, Gutman J, Yeung L, Mai C, Finkelstain J, Mehta S, Pons-Duran C, Menéndez C, et al. Cochrane Database Syst Rev. 2022 Feb 1; 2(2022). Epub 2022 Feb 1.
- The future of Cochrane Neonatal.[Early Hum Dev. 2020]The future of Cochrane Neonatal.Soll RF, Ovelman C, McGuire W. Early Hum Dev. 2020 Nov; 150:105191. Epub 2020 Sep 12.
- Review Consolidated standards of reporting trials (CONSORT) and the completeness of reporting of randomised controlled trials (RCTs) published in medical journals.[Cochrane Database Syst Rev. 2012]Review Consolidated standards of reporting trials (CONSORT) and the completeness of reporting of randomised controlled trials (RCTs) published in medical journals.Turner L, Shamseer L, Altman DG, Weeks L, Peters J, Kober T, Dias S, Schulz KF, Plint AC, Moher D. Cochrane Database Syst Rev. 2012 Nov 14; 11(11):MR000030. Epub 2012 Nov 14.
- Macromolecular crowding: chemistry and physics meet biology (Ascona, Switzerland, 10-14 June 2012).[Phys Biol. 2013]Macromolecular crowding: chemistry and physics meet biology (Ascona, Switzerland, 10-14 June 2012).Foffi G, Pastore A, Piazza F, Temussi PA. Phys Biol. 2013 Aug; 10(4):040301. Epub 2013 Aug 2.
- Standardizing definitions and reporting guidelines for the infertility core outcome set: an international consensus development study.[Fertil Steril. 2021]Standardizing definitions and reporting guidelines for the infertility core outcome set: an international consensus development study.Duffy JMN, Bhattacharya S, Bhattacharya S, Bofill M, Collura B, Curtis C, Evers JLH, Giudice LC, Farquharson RG, Franik S, et al. Fertil Steril. 2021 Jan; 115(1):201-212. Epub 2020 Nov 30.
- Basic Guidelines for Reporting Non-Clinical Data - Assay Guidance ManualBasic Guidelines for Reporting Non-Clinical Data - Assay Guidance Manual
Your browsing activity is empty.
Activity recording is turned off.
See more...