Authenticity, Integrity, and Security in a Digital World
Contributors
National Academies of Sciences, Engineering, and Medicine; Policy and Global Affairs; Government-University-Industry Research Roundtable; Paul Whitacre, Rapporteur.June 2019
Digital technology is incorporated into nearly every facet of American life, from commerce, community, healthcare, food systems, transportation, education, media, entertainment, and employment. Its increasingly integral nature raises critical questions moving forward, such as what emerging technologies are complicating the ability to verify authenticity and integrity in a digital world? Also, what emerging technologies enable the creation of systems of trust that enforce standards of authenticity, integrity, and security? How can partnerships between government, universities, and companies shape public policy to prioritize authenticity and integrity within systems—and who will be the stewards and custodians of such systems?
To address these and related questions, the Government-University-Industry Research Roundtable (GUIRR) held a workshop at the National Academy of Sciences on February 19-20, 2019. Through presentations from experts and robust discussions, the workshop focused on technologies, processes, and governance that affect digital privacy and security now and into the coming decades.
The meeting opened with a keynote address delivered by Kristina Johnson, chancellor of the State University of New York (SUNY). Chancellor Johnson drew on her experience in academia, business, and government offering five lessons related to the development and integration of emerging digital systems in research. Her first lesson: Data is everything, or, quoting a colleague, “they who have the most data win.” Second is to have an important problem to work on that can only be solved with the new technology, but—as a third lesson—it has to be the right problem to solve. She illustrated these lessons with her own research into optoelectronic pre-screening to automate the examination of pap smear slides.1 The automation could detect cervical cancer more accurately and at an earlier stage than manual review of slides by cytologists, potentially preventing thousands of deaths in the United States and millions worldwide.
The fourth lesson Johnson mentioned was the need for cross-disciplinary expertise on research teams. To solve the right problem with the right algorithm and on the right platform, assembling a diverse team is critical to finding solutions. Johnson's fifth and final lesson emphasized the importance of education and its impact on the workforce—to realize the potential opportunity for economic growth and other benefits of artificial intelligence (AI) (an estimated $6 trillion worldwide by 2030). To maintain competitiveness in the global economy the U.S. must have a sufficiently trained workforce with the technical skills to succeed. She called for education at all levels to expand and adapt significantly—including research at doctoral levels, hands-on applied learning, training and up-skilling for the currently employed, and certificate programs.
Johnson noted that the AI workforce shortage goes beyond the tech sector, affecting jobs in finance, healthcare, the fashion and automotive industries, and many others. The new range of workforce needs opens up the field to a new set of individuals—referring to a Colorado community college that developed a 6-week bootcamp-style course in optoelectronics. Many of the participants, including low-income individuals, went on to new employment in the field. SUNY researchers have used AI to tackle postal automation, digital humanities, and individualized medicine, among other efforts. Workforce development is a unifying focus across the 64 campuses that make up the SUNY system. She highlighted several of SUNY's relevant cross-cutting efforts, including the New York Institute for Artificial Intelligence (NY-Advance), which develops partnerships in economic development, social responsibility, and innovation.
Returning to the power of data to solve problems, Johnson described a new project at SUNY to operate the university as an open, integrated network involving multiple players inside and outside the institution. The project highlights the potential for AI to enable individualized education, in which students can be assessed and provided a course plotted through the SUNY system based on their interests and talents.
TRUST IN THE DIGITAL FUTURE
Janna Anderson, director of the Imagining the Internet Center at Elon University, and Lee Rainie, director of Internet and Technology Research at the Pew Research Center, co-presented on key findings of a 2016 study they conducted through the Pew Research Center on how technology leaders and others view the future of online trust.2 In a series of studies over the past 15 years, Pew and Elon have solicited thousands of expert opinions on hopes and fears for the future. Their most recent study asked, “Will people's trust in their online interactions, work, shopping, social connections, pursuit of knowledge and other activities be strengthened or diminished over the next 10 years?” Of the 1,200 responses received, 48 percent said trust will be strengthened, 28 percent said it will remain the same, and 24 percent said it will diminish.
The more optimistic respondents said systems will improve and people will adapt and more broadly embrace digital tools and platforms, which will be designed to strengthen trust. Those with the most hope also said researchers and others who have control over systems and tools will work to design, maintain, and evolve the tools to serve the public good. Some respondents predicted that the nature of trust will become more fluid as technology embeds itself into human and organizational relationships. While people have always had to rely on their instincts about whether to trust or not, they are now faced with many decisions to make, in many contexts, and with little information on which to base decisions.
Other experts predicted that trust will not grow, but technology usage will continue to rise as a “new normal” sets in. To benefit from digital systems, people will have to sacrifice trust or suspend their worries. Some survey respondents mentioned blockchain could help foster trust, while others expect the emerging technology to have limited value (a topic discussed in detail in a later panel). Those who said trust will diminish warned that powerful forces (e.g., corporate or government interests, or criminal elements) will threaten individuals' rights.
Rainie also described another Pew survey on the future of connectivity in the age of cyberattacks.3 Respondents were asked, given the growth of Internet of Things (IoT), “Is it likely that attacks, hacks or ransomware concerns in the next decade will cause significant numbers of people to decide to disconnect, or will the trend towards greater connectivity of objects and people continue unabated?” Only 15 percent said more people will disconnect, while 85 percent said connectivity will continue unabated. Here, six themes emerged among respondents. First, in weighing privacy and convenience, people crave connection and convenience, and a tech-linked world serves both these needs well. Second, most people said unplugging is impossible now, and will be even tougher in forthcoming decades. Third, risk is part of life, and most people do not think the worst-case scenarios will affect them. Fourth, many people expressed the hope that human ingenuity and risk mitigation strategies will make IoT safer. Fifth, notable numbers did say they will disconnect. Finally, whether or not they do, the security and privacy dangers are real. These issues will be magnified by the rapid rise of IoT.
Rainie and Anderson concluded by discussing the complex nature of trust and the challenges of monitoring societal trends related to trust by outlining several insights from their studies:
- Trust is fractured (for example, people may not trust all of government, but will trust certain agencies) and is not evenly distributed;
- Trust, or lack of it, is connected with political and social conflicts, and measures of trust often illuminate other political/ sociological issues;
- Trust is being reshaped by technology, and “distributed trust” mediated by tech platforms is rising; and
- Bots and algorithms influence information flows; deep fakes may affect trust even more.
Respondents to Pew surveys often suggest trust-building starts with community engagement. Addressing a question from the audience, Rainie challenged the popular anxiety around how digital spaces have enabled “echo chambers”—that people only consume information they already believe to be true. While confirmation bias is certainly an element of the human condition, Pew's research reflects that people engaged on particular issues are generally very familiar with the opposition's take, to effectively argue their perspective. He suggested that “empty chambers” rather than “echo chambers” create more opportunity for the spread of misinformation because of a lack of social engagement. Both Anderson and Rainie agreed on the continued need for critical thinking-oriented education as society becomes more invested in, and dependent on, digital technologies.
DIGITAL TOOLS FOR PREVENTING FAKERY, DECEPTION, AND BIAS
The next panel discussed tools to monitor, track, and prevent the misuse of digital technologies for fakery and deception, privacy invasion, and the perpetuation of bias. Aylin Caliskan, assistant professor in the department of computer science at George Washington University, spoke about algorithms as mirrors of society with a focus on identifying and quantifying bias in language models. She and colleagues have looked at how bias is embedded into semantics and ultimately into machine learning (ML) models.
To see if biases are random or embedded into systems, Caliskan needed to find a way to analyze unsupervised language models and determine how to quantify or detect these biases.4 She explained that bias patterns get embedded in semantics, which are transferred to distributional meaning (dictionaries for machines that represent the meaning of words with numbers) and ultimately systematic bias gets embedded into ML models.
Natural language processing as a service is widely available. Google, Amazon, and many others offer public ML models used billions of times every day to come up with results or outcomes. These models require a huge amount of data. They take in hundreds of billions of sentences to generate a dictionary for the internet itself, based on supervised, unsupervised, structured, and unstructured data. A neural network is trained by looking at co-occurrences of words in context.
Caliskan and her colleagues used the implicit association test from social psychology and adapted it to machines, developing a method they term the Word Embedding Association Test. First they looked at neutral, universally accepted stereotypes not considered harmful to society, such as “flowers” as pleasant and “insects” as unpleasant, or “musical instruments” as pleasant and “weapons” as unpleasant. But more harmful race and gender stereotypes can also be replicated. These biases have harmful impacts because ML models are used to make significant decisions, such as related to insurance, legal issues, or job selection (Figure 1). For example, two people with the same qualifications, but with different names signaling a woman or an African American, can be screened differently.
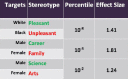
Figure 1
Word Embedding Association Test evaluating race and gender stereotypes. SOURCE: Aylin Caliskan, George Washington University, presentation to the Government-University-Industry Research Roundtable on February 20, 2019.
Societal bias in AI is built on veridical associations (such as those related to gender in different occupations); universal biases (such as the flowers-versus-insects example mentioned above); and prejudice related to culture and race. How these biases may affect AI applications such as drone surveillance and driverless cars is not yet quantified. Caliskan urged more transparency, especially when different models are combined, in order to understand how bias is introduced or amplified. She warned against building digital analogs of biases that marked previous eras. In the 1950s, for example, Robert Moses planned New York parkway bridges at a height preventing bus access to beaches and other areas on Long Island, reinforcing patterns of segregation. It is important to build responsible algorithms that can be held accountable, that are transparent, and that can be explained and interpreted to ensure a new era of fairness in machine learning.
Danny Yuxing Huang, postdoctoral research associate with the Center for Information Technology Policy at Princeton University, next discussed how to help consumers build trust in IoT devices such as security cameras, monitors, smart plugs, and other products. As IoT devices become more common and are relied upon in everyday life, questions and concerns around security and privacy will become more significant to consumers and policymakers.
According to Huang, existing trust-building tools for monitoring IoT devices are either easy to use but expensive, or are free but difficult to use. The Center for Information Technology Policy at Princeton has built a new tool called IoT Inspector that is both free to consumers and easy to use. It is an open-source, desktop application on Linux or iOS (PCs planned for the future) that passively monitors smart home networks.5 It provides a visualization of potential security and privacy issues as a one-click solution. It was also built to benefit IoT researchers because it provides a nonproprietary dataset that can measure IoT traffic in home networks.
He demonstrated how a user would download and use the IoT Inspector, and the issues it can flag. IoT Inspector uses Address Resolution Protocol (ARP) spoofing by “tricking” a device into thinking the computer on which the IoT Inspector is being run is the router. In this way, IoT Inspector operates as a middleman to intercept packets and analyze them as they go back and forth between the internet and smart home devices. The tool shows when and with whom IoT devices communicate. As one example, Huang showed how the IoT Inspector revealed a common consumer device was sending packets to an unknown ISP in China. Similarly, it detected that watching channels on Roku TV resulted in contact with third-party marketers.
After a beta period, IoT Inspector was slated for public release in late February 2019 to meet consumer demand for tools to analyze IoT devices, and to enable researchers' study of the privacy and security issues related to IoT devices and traffic on home networks.
Matt Turek, media forensics program manager at the Defense Advanced Research Projects Agency (DARPA), presented on MediFor, a DARPA program begun in 2016 to automatically assess the integrity of images and video media assets at scale. The 4-year program will involve hundreds of researcher-years of effort.
Billions of visual media assets are produced daily—on surveillance cameras, digital cameras, cell phones, or other devices—and any of those assets may need to undergo inspection for legal, intelligence, or other purposes. According to Turek, manual integrity assessments cannot keep up with the expert and automatic manipulation that can be done on these assets—compelling fake images can now be created with little to no technical skill for about $10 each. The MediFor program began with an enormous imbalance between the thousands of tools that can effectively manipulate images and the limited number of tools that can detect manipulated images. Likewise, the speed of detection technologies only allowed researchers to examine a few media assets per day, which was not adequate to meet the number of assets created daily. There was also no quantitative measure or score of integrity.
Turek used examples of manipulated images to explain three essential elements or levels of media integrity:
- 1.
Digital integrity: Are pixels, noise patterns, and digital packaging consistent or inconsistent? Are pixels blurred? Are digital fingerprints evident?
- 2.
Physical integrity: Are the laws of physics violated in the image, such as shadow and lighting or multiple vanishing points?
- 3.
Semantic integrity: Does bringing in other sources, such as associated images taken at the same time, contradict the evidence in the image under review.
MediFor's integrity reasoning approach combines the digital, physical, and semantic layers into an integrated assessment; more than 50 integrity indicators have been constructed to date (Figure 2). The goal is to have a single integrity score for an asset.
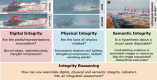
Figure 2
Elements of integrated reasoning for MediFor DARPA program. SOURCE: Matt Turek, DARPA, presentation to the Government-University-Industry Research Roundtable on February 20, 2019.
The MediFor program began with a small initial dataset in 2016. A larger-scale (although still small at internet scale) dataset of images and videos has been developed with the National Institute of Standards and Technology (NIST) for training and testing. Measuring and quantifying performance is an important part of the project. The goal is a high level of performance with minimal false positives, and performance has improved since the start of the program. Fusing the information across all the indicators results in a better job of detecting manipulation, so that entities creating automated manipulations have a greater burden to successfully deceive.
During the subsequent discussion period, an audience member observed that the democratization of digital technologies for collecting and analyzing data is likely advancing the capabilities both of nefarious actors and those trying to detect and prevent nefarious acts. The panelists also discussed the complexities of standardizing security and authentication practices across technologies; Huang mentioned that consumer awareness and advocacy can often drive the adoption of standards, and systems like the IoT Inspector empower consumers with access to information about privacy and security.
Ensuring Authenticity and Trust in Digital Systems
The third panel of the workshop moved from threats to security and privacy to a discussion of how two pioneering technologies, quantum computing and blockchain, may enable solutions to verifying and ensuring authenticity and integrity in digital systems.
Mark Jackson, scientific lead of business development for Cambridge Quantum Computing, gave an overview of the basic principles and definitions of quantum computing, stressing that quantum computing is not a faster or more advanced version of current systems, but a completely different type of computer. It is built on Quantum Bits (qubits), rather than the “1s” and “0s” of bit computing (Figure 3). Currently, more than 80 groups are building quantum computers, focusing on different strategies and technologies. While still in the early stages, Jackson predicted quantum computing will impact every area of science and technology in the next 5 to 10 years.
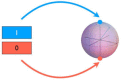
Figure 3
Difference between a bit and a quantum bit. SOURCE: Mark Jackson, Cambridge Quantum Computing, presentation to the Government-University-Industry Research Roundtable on February 20, 2019.
Jackson explained that most information sent over the internet is actually an encrypted version of that information translated through a protocol, and the receiver of the information requires a key to decrypt the information. Breaking into current encryption protocol requires a very powerful computer, running for great lengths of time (millions of years), which is not a practical reality. In the next decade or so, however, quantum computers will have this potential and could perform the decryption in minutes or seconds. While alarming, the positive news is post-quantum encryption methods are already under development, including lattice-based cryptography, code-based cryptography, and multivariate cryptography, to resist hacking. What they all have in common is they are based on random numbers, which, he said, are harder to come up with than one would assume. Cambridge Quantum Computing has built a device that uses quantum entanglement to produce certifiable random numbers as security against quantum hacking threats.
As the IoT becomes more prevalent, the need for protection against quantum hacking will grow as well. Cambridge Quantum Computing is developing a highly sophisticated form of watermarking. Practical applications include fighting photo and video piracy, enabling secure e-contracts, and protecting the integrity of healthcare data.
Mike Orcutt, associate editor, MIT Technology Review, turned to blockchain technology and how it fits into the context of digital security and privacy. As a journalist, he said he focuses on language as much as technology because how people talk about technology matters. Thus, the way people, including those in the media, talk about blockchain affects the ways they imagine its use in the future.
Bitcoin is the most prevalent use of blockchain, Orcutt said. In his opinion, understanding Bitcoin is a way through which to imagine how the technology underlying it can be used in other places. Its most important attribute is the development of cryptographic trust among a network of people. More than electronic money, Bitcoin is a network of computers distributed around the world. Shared software rules are used to collectively maintain a database that is extremely difficult to change unless the whole network agrees to change it. It is also a network of users who are willing to pay fees for the opportunity to transact in this way. The data is stored using cryptography in a way that makes it nearly impossible to tamper with. In terms of data provenance, Bitcoin keeps a public record of every transaction made in the order they were made. It has its own economy in that people invest in hardware and electricity. Most importantly, related to the workshop topic, Bitcoin is a shared data resource, a real-time truth of every bitcoin that every person holds.
Bitcoin shows that a working blockchain system is not just one thing, but rather many things with the whole greater than the sum of its parts. To him, glossing over Bitcoin ignores crucial questions: Who are the network participants? What is the data resource they need to share? Most importantly, why do they need cryptographic trust?
To provide an example of another potential application, Orcutt summarized an article he wrote for MIT Technology Review6 about recent trials run by the Centers for Disease Control and Prevention (CDC) using blockchain for public health surveillance. One of the software engineers interviewed said public health has many existing networks that could benefit from blockchain—for example, to share data about a disease outbreak or drug shortage in real time a cryptographic ledger would show the data was correct and not tampered with. An incentive to contribute to the network would be needed, perhaps in some form of cryptocurrency. Blockchain could mediate the transactions.
Without advocating one way or the other, Orcutt said these are the kinds of ideas to wrestle with for those interested in blockchain. Fundamental technical hurdles exist, including that it uses a lot of electricity, is slow in processing transactions, and poses novel security challenges. Significant questions remain about how exactly to govern decentralized networks, and it is still difficult for regular people to use. The technology has been compared to a creation of a fundamental internet protocol—if that analogy is correct, he said, a great deal of technical work is required to see if blockchain has important uses beyond cryptocurrency.
DATA ETHICS, GOVERNANCE, AND PARTNERSHIPS
In introducing the final panel of the workshop, GUIRR co-chair Al Grasso noted the morning panels focused on technology tools and processes. The afternoon session turned to ethics, governance, and partnerships undergirding the technology, including international implications.
Anjanette Raymond, associate professor of business law and ethics at the Kelley School of Business at Indiana University, spoke about sharing data in a “knowledge commons” approach to solve problems. She argued that the solution to many large problems, such as climate change, exists within a commons approach in which multiple stakeholders share data—and at the heart of this approach is a new way of looking at what data is or should be shared, and what data should be open, free, and accessible to all.
According to Raymond, historic laws are now being coded into algorithms, which has created larger problematic impacts and responses. As an example, when NASA livestreamed its Rover event on YouTube, the YouTube algorithm identified the livestream as plagiarism because a newspaper had written about the event beforehand, and the livestream was shut down. The flaw in the system was that there was no overriding human factor or “eject button” to indicate the legitimacy of NASA's broadcast. This example demonstrates how difficult it is to apply laws that were written before the current era of “coding” laws into practice.
In contrast, open research ecosystems involve all stakeholders, so that, for example, scientists and policy makers can support each other. Raymond argued that human-centered design and open science or data will not solve problems independently without thinking of the complexity of an entire ecosystem with multiple paths and solutions. Open science and data have large funding and storage implications—Raymond urged tackling the questions about why data should be open, rather than deferring to cost-benefit analysis that may discourage deployment.
A cybersecurity expert, an ethicist, and Raymond—who is a lawyer—co-authored a paper on issues around automated decision-making.7 Describing this paper, Raymond suggested that arguments centered on privacy and concepts of property ownership do not address many problems concerning end users' rights—instead the focus should be on understanding the impacts on all the actors within the ecosystem of converting data into knowledge. For example, federal law requires financial institutions not to discriminate in lending, yet these institutions must use rough proxies because they cannot gather demographic information (a legacy from when people could be discriminated against by race when they applied for loans in person). She questioned why demographic information cannot be gathered and siloed while the lending decisions are made, and then combined to understand any persistent bias patterns. While Raymond recognized that privacy must be respected, as a framework for addressing complex data ethics questions, privacy protection can also stand in the way of protecting social goods.
Wendy Belluomini, director of IBM Research Ireland, spoke about the worldwide data protection/privacy landscape. A wide array of laws, regulations, and other mechanisms are in place in different states, countries and regions. She noted the public in Europe is generally more aware of privacy issues than in the United States, and European Union (EU) regulations reflect that higher concern. The EU's General Data Protection Regulation (GDPR) carries large penalties for companies found in violation. An individual owns his or her data, and consent is much more limited. This consent is based on the idea that “I have the right to be forgotten,” and applies to anyone residing in the EU (including her, a U.S. citizen residing in Ireland, she noted). Companies also must communicate about a data breach within 72 hours. The rest of the world, she suggested, will eventually follow the EU example.
Belluomini described factors changing the landscape in data privacy fall within six categories—in some cases the factors are fighting against each other:
- 1.
Political: Governments are moving to digitization of data, but complex regulations and practices make that difficult;
- 2.
Technological: Enormous developments in AI are pushing the use of data in IoT and edge computing and increasing the value of data;
- 3.
Economic: Stable economic growth in major markets, rapid growth of development markets, and the rise of the sharing economy all have data implications;
- 4.
Societal: Rising use of customer service in evaluating product and business quality; increasing use of online services; data-sharing transparency;
- 5.
Ecological/environmental: Technological integration in various industries and markets;
- 6.
Legal: Data breach and privacy regulations.
She said that IBM and other companies are developing tools to protect the privacy of data while still using it, including federated AI and next-gen cryptography, and quantitated risk assessments to manage risk.
Belluomini closed by highlighting three challenges for governments in protecting privacy. First, there is a need for regulatory consistency. The current landscape makes it difficult for large corporations and especially smaller entities that want to participate across jurisdictions. Second, work is needed to update thinking about privacy and regulation, and to incorporate technology risk and solutions into regulatory frameworks. Third, she agreed with other presenters about the value of providing examples of using data for public good, while preserving privacy, so the public understands the benefits and not just the risks.
Footnotes
- 1
For more information on the research, see: Ramkumar Narayanswamy and Kristina M. Johnson (1998), Optoelectronic pre-screening to automate the examination of pap smear slides, Applied Optics 37(25).
- 2
Pew Research Center, August 2017, “The Fate of Online Trust in the Next Decade.” Available at: http://www
.pewinternet .org/2017/08/10/the-fate-of-online-trust-in-the-next-decade/. - 3
Pew Research Center, June 2017, “The Internet of Things Connectivity Binge: What Are the Implications?” Available at: http://www
.pewinternet .org/2017/06/06/the-internet-of-things-connectivity-binge-what-are-the-implications/. - 4
Aylin Caliskan, Joanna Bryson, and Arvind Narayanan, 2017, Semantics derived automatically from language corpora contain human-like biases. Science 359(6334), pp. 183–186.
- 5
For more details, see: https:
//iot-inspector.princeton.edu. - 6
Mike Orcutt, “Why the CDC wants in on blockchain,” MIT Technology Review, October 2, 2017.
- 7
Anjanette H. Raymond, Emma Arrington Stone Young, and Scott J. Shackelford, 2018, Building a better HAL 9000: Algorithms, the market, and the need to prevent the engraining of bias, Northwestern Journal of Technology and International Property, 15(7).
This Proceedings of a Workshop—in Brief has been prepared by Paula Whitacre as a factual summary of what occurred at the meeting. The committee's role was limited to planning the meeting. The statements made are those of the author or individual meeting participants and do not necessarily represent the views of all meeting participants, the planning committee, or the National Academies of Sciences, Engineering, and Medicine.
Grace Wang, State University of New York; Timothy Persons, Government Accountability Office.
Susan Sauer Sloan, Director, GUIRR; Megan Nicholson, Program Officer; Lillian Andrews, Senior Program Assistant; Clara Savage, Financial Officer; Cyril Lee, Financial Assistant.
To ensure that it meets institutional standards for quality and objectivity, this Proceedings of a Workshop—in Brief was reviewed by Terry Benzel, University of Southern California and Jonathan Pettus, Dynetics. Marilyn Baker, National Academies of Sciences, Engineering, and Medicine, served as the review coordinator.
For additional information regarding the workshop, visit http://www.nas.edu/guirr.
The National Academies of SCIENCES • ENGINEERING • MEDICINE
The nation turns to the National Academies of Sciences, Engineering, and Medicine for independent, objective advice on issues that affect people's lives worldwide.