Corresponding author: Elina Pradhan, Harvard T. H. Chan School of Public Health, Boston, Massachusetts, United States; ude.dravrah.liam@nahdarpe.
Introduction
This chapter analyzes the economic returns to education investments from a health perspective.1 It estimates the effects of education on under-five mortality, adult mortality, and fertility. It calculates the economic returns to education resulting from declines in under-five mortality and adult mortality, while considering the effects of education investments on income. It also develops policy-relevant recommendations to help guide education investments.
Our study adds to the evidence that education is a crucial mechanism for enhancing the health and well-being of individuals. The relationship between education and health is bidirectional, because poor health could affect educational attainment (Behrman 1996; Case, Fertig, and Paxson 2005; Currie and Hyson 1999; Ding and others 2009). Historical findings in the education and health literature have highlighted the strong association between education and health. Recent literature has exploited natural experiments to provide causal evidence of the impact of education on health. Studies show that education plays a critical role in reducing the transmission of human immunodeficiency virus/acquired immune deficiency syndrome (HIV/AIDS) in women by improving prevention and treatment. Keeping adolescent girls in secondary school significantly attenuates the risk of HIV/AIDS infection (Baird and others 2012; Behrman 2015; De Neve and others 2015). Early child development has a lifelong impact on the mental and physical health of individuals.2 Other studies have demonstrated that progress in education can increase positive health-seeking behaviors (such as accessing preventive care) and reduce overall dependency on the health system (Cutler and Lleras-Muney 2010; Feinstein and others 2006; Kenkel 1991; Sabates and Feinstein 2006).
Previous literature on education, health, and economic productivity suggests that the impact of education is more significant in times of rapid technological progress (Preston and Haines 1991; Schultz 1993). The morbidity and mortality differentials across levels of schooling are significant in the presence of increasing scientific knowledge about diseases and behaviors, as well as access to medicines and vaccines. Additionally, analysis by Jamison, Murphy, and Sandbu (2016) shows that most variation in under-five mortality can be explained by heterogeneities in the speed at which countries adopt low-cost health technologies to increase child survival.
Different studies that have assessed the effects of education on mortality and fertility show an association between educational attainment and reductions in both outcomes.3 This chapter goes beyond previous work by using improved and updated data, and by controlling tightly for country-specific effects in both levels and rates of change of mortality. Although several studies have examined the effects of female schooling on child mortality, we are aware of only one other cross-national study (Wang and Jamison 1998) that estimated the macro effects of schooling on adult mortality. Other studies have focused on the relationship between schooling and adult health, but they primarily do so for a single country or small set of countries.4 Some key findings from our study are highlighted in box 30.1.
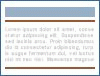
Our study comes at a critical juncture for education and health, as the global community moves forward in the context of the Sustainable Development Goals, which stress the importance of taking into account the cross-sectoral nature of global development challenges.
This chapter is organized into three broad sections:
The first section presents the results of our regression analysis, which examines the effects of increases in mean years of schooling, as well as schooling quality, on under-five mortality, adult female mortality, adult male mortality, and fertility. We also decompose the changes in mortality between 1970 and 2010, and estimate the mortality impact of education gains in the Millennium Development Goal (MDG) period. The findings from our regression inform the subsequent sections, which use the estimated effect size to determine the rates of return to and benefit-cost ratios (BCRs) of education.
The second section explores the effects of augmenting the traditional rates of return analysis for education with its mortality-related health effects. We also estimate the BCR of education from earnings-only and health-inclusive perspectives, and address the question: What would be the returns to investing US$1 in education in low-, lower-middle-, and upper-middle-income countries?
Finally, we discuss our findings, present recommendations, and consider the next steps the global education community might take to ensure that all countries make substantial progress toward global education targets.
Modeling the Effects of Educational Attainment on Health
Data and Methods
We estimated the effects of educational attainment over time, measured in mean years of schooling for ages 25 years and older. This age group was selected to ensure that the data were unlikely to contain censored observations.5 Data on mean years of schooling were obtained through the Barro and Lee (2013) dataset, which includes 92 low- and middle-income countries (LMICs), each of which included observations at five-year intervals between 1970 and 2010. Mortality rates were defined as the probability of dying between age 0 and age 5 years for under-five mortality, and the probability of dying between age 15 and age 60 years for adult mortality. The United Nations (UN) World Population Prospects (2015 revision) was used for all fertility and mortality estimates (). Annex 30A contains a full list of countries included in the analysis. Definitions of age groupings and age-specific terminology used in this volume can be found in chapter 1 (Bundy and others 2017).
Sources of Data in the Study.
Regression Models
We modeled the effects of educational attainment (female schooling, male schooling, and overall schooling) on under-five mortality, adult female mortality, and adult male mortality controlling for time and income (gross domestic product [GDP] per capita) using hierarchical linear models (HLMs) as in equation (30.1). Jamison, Murphy, and Sandbu (2016) provide a range of comparative models on under-five mortality and assess their statistical properties. They concluded that the HLM structure has the best fit to macro-level data to determine the macro-level impact of education on mortality, and we therefore develop their modeling approach here.

The under-five mortality model estimates the impact of adult education (education of those ages 25 years and older) on the mortality of those under age 5 at each time period t, while the adult mortality models estimate the impact of adult education on aggregate adult mortality or self and peer mortality, adjusting for income, any technological advancements, and secular time trends. Time is specified as a categorical variable that indicates five-year increments from 1970 to 2010, and is a proxy variable for measuring technological progress over the study period. Annex 30B contains descriptive statistics for countries included in the regression, including means and standard deviations for mortality and fertility rates, years of schooling, and test scores.
Preston (1975, 2007) shows that national income plays a critical role in improving health outcomes. He further argues that factors exogenous to income have played a crucial role in improving mortality. An influential paper by Pritchett and Summers (1996) pointed to education as well as income as being among the important factors influencing mortality decline. As highlighted by Jamison, Murphy, and Sandbu (2016), technological progress, which includes research, development and implementation advances in vaccines, sanitation, clinical care, and disease control, has played a driving role in improving health outcomes in recent years. In line with these authors, we also loosened the assumption of homogeneity of technical advancements across countries. By allowing the impact of time or technological progress to vary every five years, and by allowing for a country-specific impact of technological progress on mortality in addition to controlling for GDP, we provide conservative estimates of the impact of education on mortality and fertility. Annex 30C provides additional details on the model, and annex 30D tabulates all regression results in detail.
Decomposition Analysis
Results from the regressions were then used to decompose the changes in under-five, adult male, and female mortality between 1970 and 2010. In this analysis, we first calculate the difference in mean covariates in the sample in 2010 compared with 1970. Then, we calculate the overall reduction in mortality when education increases by the difference in mean from 2010 to 1970, which is the impact estimate from the HLM model multiplied by the difference in the mean of that covariate. The fraction attributable to any particular covariate is then the overall reduction in mortality attributable to the changes in that particular covariate, divided by the overall change in mortality over the period. For example, equation (30.2) illustrates the estimation process for the fraction attributable to education, ΔMorted’ where
and
βed = the estimate of impact of education on mortality from the HLM model.
Estimating the Mortality Impact of Education Gains in the MDG Period
To understand the impact of education gains during the MDG period on under-five and adult mortality, we also estimate the number of excess deaths that could have occurred had educational attainment stayed at the 1990 levels. In this analysis, we model the counterfactual scenario of the number of additional deaths during 2010–15 had education stagnated at 1990 levels, where we apply the increases in education in low-income countries (LICs) and lower-middle-income countries to the coefficient from our HLM results to calculate the excess deaths. Annex table 30C.2 provides estimation details.
Results
Effects of Schooling on Adult and Under-Five Mortality and Fertility
We modeled the effects of education based on three different schooling variables: mean years of schooling for girls, boys, and both genders. The results of our analysis, which examined female and male adult mortality separately, make an important contribution to the existing evidence base. Very few studies have focused on any potential impacts that educational attainment may have on adult mortality at the macro level. To the best of our knowledge, the most recent cross-country study that specifically assessed the macro effects of schooling on adult mortality is from 1998 (Wang and Jamison 1998).
shows the results of our hierarchical models; each column represents the results for the five dependent variables—overall adult mortality, adult male mortality, adult female mortality, under-five mortality, and fertility. Panel A shows results for models in which we consider the impact of average male and female schooling on the five health outcomes. Panels B and C show the impact of female and male schooling, respectively, while controlling for the ratio of male to female years of schooling. The schooling ratio is included to control for any differential impact of male and female schooling in panels B and C, respectively.
Impact of Schooling on Health Outcomes: Results from Hierarchical Linear Models.
demonstrates that improvements in female educational attainment drove declines in mortality and fertility in LMICs between 1970 and 2010: A one-year increase in a country’s mean years of schooling (both sexes) is associated with a 2.5 percent reduction in male adult mortality and 3.1 percent reduction in female adult mortality, a 3.3 percent reduction in under-five mortality, and a 2.4 percent reduction in the total fertility rate (TFR), in LMICs (panel A of table). The effect of male schooling on adult and under-five mortality and TFR is small and often not significant. In contrast, improvements in female schooling are associated with large declines in both female and male adult mortality, accounting for much of the observed effects of education on health. A one-year increase in mean years of schooling for girls (panel B of table) is associated with reductions in female and male adult mortality of 3.7 percent and 2.2 percent, respectively; under-five mortality declines by 4.2 percent, and the TFR by 2.4 percent. The comparison of the effect of male (panel C of table) and female schooling (large effect) on adult mortality, under-five mortality, and fertility clearly shows that the education-related declines in mortality between 1970 and 2010 in LMICs are strongly linked to increases in female schooling.6
Decomposition Analysis: Reductions in Adult and Under-Five Mortality Rates from Gains in Female Schooling, 1970–2010
Based on the results of our HLM, we developed estimates of the proportion of mortality reductions between 1970 and 2010 that can be attributed to improvements in female schooling. Adult female, adult male, and under-five mortality all saw impressive reductions over this period, with particularly dramatic improvements seen in under-five mortality. Between 1970 and 2010, the global under-five mortality rate declined by 64 percent, from 139 deaths under age five years per 1,000 live births to 50 in 2010. In LICs, gains have been particularly strong since 1990: under-five mortality declined by more than 50 percent, from 186 deaths per 1,000 live births to 91, during this 20-year span. The adult mortality rate, that is, the probability that a person dies (expressed per thousand persons) between age 15 and age 60 at prevailing mortality rates, also recorded a notable decline between 1970 and 2010, falling 38 percent globally, from 247 to 153. Reductions in adult female mortality were particularly substantial, declining by 43 percent over the 40-year period.
Our decomposition analysis suggests that of the reductions in mortality seen in LICs and middle-income countries (MICs) between 1970 and 2010, 14 percent of reductions in under-five mortality, 30 percent of reductions in adult female mortality, and 31 percent of reductions in adult male mortality can be attributed to gains in female schooling (, panel A). This panel shows that technological progress, and to a much lesser extent income, affected mortality over this period, a finding in line with other studies (Jamison, Murphy, and Sandbu 2016).
Education’s Contribution to Mortality Decline.
Mortality Impact of Increases in Educational Attainment during the MDG Period
A complementary way of assessing the magnitude of education’s impact on mortality is to look at the reduction in the number of deaths resulting from a given increase in education levels. We take as an example the increase in female education in LMICs during the MDG period from 1990 to 2015. This increase was 1.5 years in LICs and 2.4 years in MICs. We ask the question: Based on the results of our model (), how many more deaths would have occurred in children under age 5 years and in adults ages 15–59 years if education levels had remained at their 1990 levels? Panel B of shows the results. We estimate that a total of 7.3 million under-five and adult deaths were averted between 2010 and 2015 because of increases in educational attainment since 1990. Total deaths averted in MICs were substantially higher than in LICs because the population exposed to mortality risk is about six times larger in MICs compared with LICs, and MICs saw a greater increase in average years of female schooling during the MDG period than did LICs.
Effects of Different Levels of Schooling on Mortality and Fertility
In addition to analyzing the overall impact of increasing average schooling by one year in a country, we considered whether differential effects accrue at different levels of schooling (). We conducted a quadratic analysis that relaxes the assumption that each additional year of schooling has the same impact on health, hence allowing the relative change in mortality with changing years of attainment to be evaluated.7 Our analysis indicates that additional years of schooling have sustained effects on all the health outcomes we examined. The coefficient on the squared years of female schooling term is positive and significant for all health outcomes, indicating that the relative effect of education on health outcomes declines with increasing years of educational attainment. This result means that the marginal impact of schooling at the primary level is higher compared with the impact at the secondary level.
Impact of Schooling Levels on Health Outcomes.
Effects of Educational Quality
In addition to the effect of years of schooling on health, we evaluated the effects of educational quality on health outcomes. This analysis proved challenging for a variety of reasons. Most fundamentally, cross-country data on educational quality are extremely limited, particularly for LICs and lower-middle-income countries. Researchers have used results from global or regional achievement tests (such as PISA, TIMSS, SACMEQ, PASEC, and LLECE8) to standardize estimates of educational quality, based on country performance on such exams. However, significant gaps remain in both longitudinal and country coverage, and concerns have been raised about the validity of using results from a limited set of tests as a proxy for educational quality.
Because of the limited number of LMICs with longitudinal data on quality, we expanded our analysis to include high-income countries (HICs) with data on quality in the Barro and Lee (2013) dataset. Annex 30A provides a full list of countries used in the HLM regressions on quality.
To evaluate the impact of education quality on health, we ran an augmented version of the HLM in , panel B, to which we added a variable measuring schooling quality (standardized achievement test scores).
Our findings largely underscore the robustness of the impact of years of schooling on health outcomes, and further suggest that quality can have an additive and substantial impact on health outcomes (). Column (B) under each dependent variable shows the results of the HLM model with education quality proxied by the composite test scores. Comparison of the returns to mean years of schooling in column (B) as compared to column (A), where the HLM model does not control for quality, shows that the impact of returns to schooling is about the same with or without controlling for test scores. In fact, improvements in test scores are predicted to reduce mortality and fertility further, above and beyond the improvements in years of schooling.
Impact of School Quality on Health Outcomes: Results from Hierarchical Linear Models.
Given the substantial difference in a one-unit change between educational attainment (one year of schooling) and test scores (a one-point increase in scores), we also present the results of both quantity and quality by using a one standard deviation change above their mean values to enable better comparability between the two (). The results of this analysis suggest that the impact of quality is substantial. A one standard deviation change in educational quality, measured by standardized achievement scores, is associated with a 2.4 percent decline in the overall adult mortality rate, a 2.3 percent decrease in adult female mortality, and a 3.4 percent decrease in under-five mortality. In all cases, however, the impact of female educational attainment remains larger than the impact of educational quality. For the three health outcomes for which both years of schooling and test scores are significant—overall adult mortality, female mortality, and under-five mortality—the impact of female years of schooling ranges from 2 to 5.2 times the impact of quality.
Impact on Health Outcomes of a One Standard Deviation Change in Education Quantity and Quality.
Our estimates of the magnitude of the effect of education quality on under-five mortality substantially exceed those of Jamison, Jamison, and Hanushek (2007), perhaps because (1) we estimate the impact on under-five mortality rather than on infant mortality, and (2) we have more observations from LMICs than these authors. However, our sample would still benefit from additional observations for LICs, lower-middle-income countries, and upper-middle-income countries (UMICs).
Our findings show that the impact of school quality on health outcomes is considerable and merits further scrutiny. It also highlights the limitations of the data, a challenge that should be considered when interpreting these results. Of the 103 countries included in the analysis, 59 countries have fewer than four years of observations. Of those with four or more observations, 35—or 80 percent of the sample—are HICs. Further work is needed to develop robust measures of education quality that are comparable across countries and tracked over time.
Calculating Health-Inclusive Rates of Return to Education and Benefit-Cost Ratios
Previous analyses have estimated the returns to education. Using household and labor market survey data, Montenegro and Patrinos (2013, 2014) have estimated the private returns accruing from increased schooling. They note that three major findings have held across analyses:
Private returns to schooling tend to remain in the range of 10 percent per year of schooling.
Returns are, on average, higher in LMICs.
Returns to primary schooling are higher than returns to secondary schooling.
When estimating private returns to education, researchers assume that costs of schooling are absorbed by the government and that the only costs to students are the opportunity costs of forgone earnings; any gains reflect the income differential between the earnings earned by students with different levels of educational attainment. The term social rates of return refers to the rate of return to education when the full cost of schooling is incorporated. In an analysis of 15 LMICs, Psacharopoulos, Montenegro, and Patrinos (2017) further considered the full cost of schooling. They found that the social rates of return to primary education were higher than those to secondary and tertiary education for both LICs and lower-middle-income countries.9
Our analysis makes an important contribution to existing research on the rates of return to education by expanding the traditional focus on earnings returns to consider some health-related (nonmarket) externalities associated with increased educational attainment (Lochner 2011; Oreopoulos and Salvanes 2011). By capturing reductions in mortality, our analysis provides a more comprehensive evaluation of returns to schooling and strengthens the investment case for education by quantifying health returns in addition to earnings returns.
Methods
The empirical work conducted as a first step in this analysis generated coefficients for the effect of one additional year of female education on under-five mortality, adult female mortality, and adult male mortality. In this section, we use these coefficients to generate the valuation of these changes in monetary terms. Earlier research by our team, funded by the Norwegian government, reviewed available evidence on the effects of education and then estimated the economic returns resulting from the reduction in under-five mortality attributable to increases in female education (Schäferhoff and others 2015). Our analysis follows the general approach used in this previous study, but improves the methodology and expands it to incorporate the monetary value of both under-five and adult mortality reductions.10
The literature in economics of education typically reports its benefit-cost analyses as internal rates of return, namely, the value of the discount rate that makes equal the present values of the cost and benefit streams. We calculate both the rate of return and more standard benefit to cost ratios.
Estimating both internal rates of return and BCRs involved the following four broad steps:
First, we used the effects of education on under-five mortality, adult male mortality, and adult female mortality from our cross-country regressions as the basis for our health-inclusive rate-of-return (RoR) and BCR analysis. From the regressions, we obtained the level of mortality reductions resulting from one more year of female schooling for each income group. For example, the average years of schooling in lower-middle-income countries is six years; our RoR and BCR calculations for these countries then estimated the rate of return to increasing female schooling from six years, on average, to seven years.
Second, applying methods similar to Global Health 2035 (Jamison and others 2013a, 2013b) and our Norwegian Agency for Development Cooperation (Norad) study, we placed dollar values on these mortality reductions. We calculated the expected health value at age a, expressed in dollars, associated with the assumed one-year increase in education level using the information on dollar value of mortality reductions combined with status quo mortality rates and fertility rates. The value-of-a-life-year (VLY) methodology used here underestimates the VLY in LICs compared with UMICs. While there is some evidence in the literature to support this assumption because the economic component of the VLY is dependent on the economic productivity of a country, there is limited reason to assume that the social VLY would differ by a country’s economic productivity (Stenberg and others 2016). We applied a conservative value of a statistical life (VSL) estimate in our study, and provide upper and lower bounds of RoR estimates and BCRs in annex 30D to illustrate the uncertainty around life year valuations.
Third, we calculated the earnings value for an increment in education. We received smoothed age-earnings profiles for LICs, lower-middle-income countries, and UMICs from Psacharopoulos, Montenegro, and Patrinos (2017) for different levels of schooling. We then estimated the marginal increase in earnings at each age across each schooling level (as in our example, where we estimated the expected level of mortality reductions resulting from one additional year of schooling for individuals with a starting level of six years). The earnings value of this increment in education for a person of age a is simply the difference between the age-earnings profiles of a secondary school graduate and a primary school graduate divided by the number of years of secondary schooling.
Fourth, we drew on cost data from the International Commission on Financing Global Education Opportunity, which provides estimates of the direct cost (c1) for schooling at the respective grade levels in each income group (). The direct cost is the cost of teacher time, implicit rent on facilities, and consumables such as textbooks. We assumed that if children are in school, they forgo earnings, so the earning value of a person of age a will be negative at the age of entry for the additional year of schooling (A). The direct cost of schooling at ages greater than A is assumed to be zero. Similarly, the opportunity cost (c2) of attending one more year of school was calculated as the earnings forgone by attending one more year of school. Similar to direct costs, the opportunity costs of schooling at ages greater than A is also zero. Annex 30Eß∑ discusses our approach in estimating the direct and opportunity costs of schooling in detail, and it tabulates the costs used in our analysis.
Direct per-pupil annual costs of schooling (unweighted), in 2012 US$.
Estimating Internal Rate of Return
Equation (30.3) expresses the net present value of costs and benefits (ePVNR[rs]), in a standard RoR (rs) analysis:
The standard RoR (rs) is simply the value of rs such that the net present value of earnings (ePVNR[rs]) is zero. Standard RoRs calculated are then compared with the health-inclusive RoRs, which we label hRoRs. Equation (30.4) gives the present value of net benefits when the benefit stream is augmented by the value of education’s health effect:
The health-inclusive RoR (rh), hRoR, is simply that value of rh such that the health-inclusive net present value (hPVNR(rh)) is zero.
Estimating Benefit-Cost Ratios
To calculate the health-inclusive BCRs, we simply apply the annual discount rate of 3 percent to all costs and benefits. The health-inclusive BCR at discount rate (r), hBCR(r), is listed below in equation (30.5), and the earnings-only BCR, eBCR(r) in equation (30.6).
Annex 30D provides the detailed methods used for RoR and BCR calculations, and an example of how benefits to reductions in under-five and adult male and female mortalities are valued.
Results
Health-Inclusive Rate of Return from Investments in Education
The standard social rate of return or earnings return is the rate of return to schooling considering direct costs, opportunity costs, and earnings benefits from schooling. Our initial calculations suggest that the earnings return of investing in an additional year of schooling in LICs is 11 percent (). These standard social rates of return, however, do not consider other social benefits of schooling. Here, we consider the added benefit of schooling on potential reductions in under-five mortality, adult male mortality, and adult female mortality.
Rate of Return of One Additional Year of Schooling in LICs, Lower-Middle-Income Countries, and UMICs. percent
Including the health benefits due to an additional year of schooling, the rate of return to investing in an additional year of schooling in LICs increases to 16 percent (14 percent to 18 percent).11 This means that the rate of return to education increases significantly when the returns to education resulting from reductions in adult mortality and under-five mortality are added to the standard rate of return.
demonstrates that the health benefits accruing from education are comparable to and at certain ages even exceed earnings benefits in LICs. This is particularly true during early adulthood (ages 20–40 years), when the benefits of reduced adult and under-five mortality are 20 percent larger than the earnings benefits. The protective benefit of education for reducing under-five mortality is particularly impressive in these settings, where under-five mortality rates remain high.
Benefit Stream for LICs from One Additional Year of Schooling.
The health-inclusive social rate of return calculations that consider health benefits show that the returns resulting from lower mortality are high in lower-middle-income countries, where the updated social returns with health, at 9.3 percent (8.4 percent to 10 percent) are 34 percent (21 percent to 46 percent) of the standard social rate of return (see and ).
Benefit Stream for Lower-Middle-Income Countries from One Additional Year of Schooling.
In addition to calculating rates of return for LICs and lower-middle-income countries, we estimate that the standard social rate of return of increasing schooling by a year in UMICs is 3.0 percent ( and ). The health-inclusive RoR is 4.7 percent (4.1 percent to 5.3 percent), which is approximately 55 percent (36 percent to 74 percent) of the returns from earnings.
Benefit Stream for UMICs from One Additional Year of Schooling.
The results tabulated in the chapter consider the VSL to be 130 times GDP per capita, which is a conservative estimate compared with the Global Health 2035 series and our previous Norad report. The estimated health-inclusive rates of return are sensitive to the VSL assigned to mortality reductions. In annex figure 30F.1, we also present the estimated internal rates of return at VSLs of 80 to 180 times GDP per capita. At 14 percent and 8.5 percent rates of return, the health-inclusive returns to education are high in LICs and lower-middle-income countries, respectively, even with the lowest VSL multiplier used.
The health-inclusive rates of return are relatively larger in lower-middle-income countries, compared with UMICs, because of higher mortality in lower-middle-income countries. In particular, the returns to reductions in under-five mortality are higher in lower-middle-income countries than in UMICs, where under-five mortality rates are less than half those in lower-middle-income countries. As shown in , the earnings benefits of schooling are consistently higher than the health benefits across all ages in UMICs. In addition, compared with lower-middle-income countries, the absolute value of health benefits and earnings benefits are higher in UMICs because of differences in GDP and VSL valuations across these two income groups.
Benefit-Cost Analysis
In addition to the internal rate of return, the returns to education can alternatively be conceptualized in the form of a benefit-cost analysis. Our results suggest that there is an enormous payoff to investing in education when investments are assessed from a health perspective. Every dollar invested in female schooling in LICs and lower-middle-income countries would return US$10 and US$3.8, respectively, in earnings and reductions in under-five and adult mortality.
For our analysis, we assumed a discount rate of 3.0 percent, which is consistent with the discount rate used in other benefit-cost calculations in public health, including the 2013 Lancet Commission on Investing in Health. Although benefits exceed costs for all income groups even when taking into account only the earnings effects of education, the additional benefits from health are significant, particularly in LICs and lower-middle-income countries.
As with RoR estimates, the BCRs are also estimated with some uncertainty. We present sensitivity analyses of the ratios in annex figure 30F.1 where we estimate BCRs for a VSL ranging from 80 to 180 times GDP per capita. In annex figure 30F.2 we present the range of BCR estimates for discount rates from 1.0 percent to 5.0 percent. Similar to internal rate of return results, we find that the health-inclusive benefit of an additional year of schooling is substantial for LICs and lower-middle-income countries even at the lowest VSL multiplier used, with returns of US$8.3 and US$3.3, respectively, for every dollar spent.
In LICs, the health benefits of education represent an impressive 92 percent increase over the earnings-only BCR; in lower-middle-income countries, health augments the traditional BCR by 44 percent. Put in other terms, 48 percent (US$4.7) of returns would come from the effect of schooling on mortality in LICs, while 31 percent (US$1.1) of the returns to education in lower-middle-income countries result from the effect on adult and under-five mortality. Even in UMICs, where lower mortality rates and higher educational attainment might suggest smaller gains, the BCR increases by 47 percent when health is taken into account, with health gains representing 32 percent (US$0.47) of the health-inclusive BCR ().
Benefit-Cost Ratios of One Additional Year of Schooling in LICs, Lower-Middle-Income Countries, and UMICs.
Discussion
Our results on under-five mortality are broadly consistent with previous robust analyses of the effect of schooling on under-five mortality, including that of Jamison, Murphy, and Sandbu (2016), who found that a one-year increase in female education was associated with a 3.6 percent decline in under-five mortality among 95 LMICs between 1970 and 2004. Our study, and other tightly controlled studies like Jamison, Murphy, and Sandbu (2016), yield estimates of education’s effects on under-five mortality that fall well below what is often reported in the literature.
Our previous analyses have also established a clear link between schooling and improved under-five health. A meta-analysis, conducted as part of our previous study for the Oslo Summit on Education, found that one additional year of female schooling was associated with a decrease in under-five mortality of between 3.6 percent and 9.9 percent (Schäferhoff and others 2015). This finding shows that our estimate on under-five mortality, while still substantial, is at the bottom end of the range of previous studies. Even this lower estimate of effect size yields a quantitatively important effect on mortality and, as we have shown, is a significant addition to the estimated economic rate of return to education. Additionally, our results show that educational quality affects health above and beyond years of schooling, but better data and further research are needed to better understand the relationship, particularly in LMICs.
The strong impact that education has on female mortality is striking and contributes further evidence on the beneficial impacts of education to women’s well-being. Schools are frequently used as channels for health information, notably, education on sexual and reproductive health. More-educated people have better access to and understanding of healthy behavior and practices. Moreover, the impact of education on women’s empowerment and decision-making power is well documented (International Center for Research on Women 2005; World Bank 2014). Hence, educated women not only have increased access to health services and information, but they are better able to make healthier choices because of their increased bargaining and decision-making power within their households.
Gains in female educational attainment have been impressive over the past 40 years. The mean years of schooling attained by girls in low- and middle-income countries have increased from about 2 in 1970 to more than 6 in 2010; the ratio of male-to-female educational attainment has increased from 67 percent to 86 percent. As our analysis shows, these gains in female schooling were pivotal in reducing under-five mortality and adult mortality. However, women’s educational attainment continues to lag behind men’s. In the LICs included in our analysis, mean educational attainment for women remained only 2.8 years in 2010, suggesting that many girls either do not attend or at least fail to complete primary school. Further reductions in mortality can be achieved with health-focused policies, as well as education policies that address out-of-school children, especially out-of-school girls.
Our analysis is limited by the paucity of data. The VLY estimates used in the health-inclusive rate of return and BCR analysis are based on evidence mostly from developed economies. Given the range of literature from LMICs, UMICs, and HICs and the uncertainty around VLY, the results presented in this chapter are based on a conservative estimate. Further sensitivity analysis using a range of VLY estimates is presented in annex 30F. Additionally, the rate of return analyses use modeled costs of schooling given the lack of comprehensive country data on private and public expenditures on schooling. While estimating the impact of schooling on health outcomes, we recognize that the bidirectionality of the relationship between education and health could bias our estimates. Our models on adult mortality estimate the relationship between education and self and peer mortality—poor health especially during school years could limit schooling, which in turn could affect health in adult years. It would be important to continue empirical research to precisely quantify this relationship. Investments in data are also needed to understand education quality—our analyses on education quality were severely restricted by the lack of data on education quality for LICs and lower-middle-income countries.
This study shows that the existing estimates of the rate of return to education are quantitatively important underestimates. This finding results from the systematic inclusion of the dollar value of education’s favorable effect on health. Although investments in education are not undertaken specifically to improve health, they produce substantial health returns. In fact, returns to education investments on health are likely to be larger than reported in this study. To the best of our knowledge, our study is the most comprehensive assessment of the monetized health benefits resulting from education, but it underestimates the full effects of education on health. This is the case because it is focused on the impact of education on adult mortality and under-five mortality. Other health outcomes—most important, the effects of education on morbidity—are not considered in our study.
Nevertheless, a BCR that takes into account the health impact of increases in education provides a forceful rationale for a much stronger cross-sectoral collaboration between the education and health sectors.
Conclusions
This study shows that although investments in education are not undertaken specifically to improve health, they produce substantial health returns. Returns are particularly high in LICs and lower-middle-income countries. Our evidence also exemplifies the important determinants of health that lie outside the health sector. Addressing these determinants requires cross-sectoral collaboration and links between education and health. Other research has shown that improved health is also linked to better education.
The need for cross-sectoral work is captured in the Sustainable Development Goals, and certain funders have already begun to strengthen the links between the two sectors. The Global Fund to Fight AIDS, Tuberculosis and Malaria has begun to finance education by supporting conditional cash transfers to keep girls in school in four Sub-Saharan African countries with high HIV/AIDS prevalence and incidence, with the objective of reducing HIV transmission. The government of Norway has strengthened cross-sectoral links through its global health and education Vision 2030 initiative. Other donors could rethink their strategies, which in many cases still reflect separate approaches to education and health.
Based on our results, we conclude the following:
Returns to education are substantially higher than generally understood, and it is important for donors and countries to reflect this in their investment decisions.
The results strongly indicate that female education matters more than male education in achieving health outcomes. Investments targeted to girls’ education yield a substantial return on health. Increased efforts are needed to close remaining gender gaps.
It is important to get children into school because of the substantial health effects resulting from school attendance, even while awaiting further improvements in quality, which our analysis also show to be important.
The highly positive BCR that takes into account the health impact of education provides a compelling rationale for much stronger cross-sectoral collaboration between the education and health sectors.
Despite the recent shift in the global dialogue on quality of education in LMICs, substantial gaps remain in the availability of data on the quality of education and learning, among other data and knowledge gaps. These gaps are largely the result of limited donor investments in global public goods for education. Increased donor support would facilitate better research and progress measurement.
Annexes
The online annexes to this chapter are as follows. They are available at http://www.dcp-3.org/CAHD.
Annex 30A. Countries Included in the Regression Analysis
Annex 30B. Descriptive Statistics
Annex 30C. Technical Annex: Hierarchical Linear Model
Annex 30D. Incorporating Education’s Effect on Mortality into Internal Rates of Return
Annex 30E. Cost of Education, by Level
Annex 30F. Sensitivity Analysis of Benefit-Cost Ratios and Internal Rate of Return
Disclaimer
This paper was initially prepared for the International Commission on Financing Global Education Opportunity as a background paper for the report, “The Learning Generation: Investing in Education for a Changing World.” The views and opinions in this background paper are those of the author(s) and are not endorsed by the Education Commission or its members. For more information about the Commission’s report, please visit http://report.educationcommission.org.
Acknowledgments
We would like to thank George Psacharopoulos and Susan Horton for their helpful feedback, and Austen Peter Davis, Desmond Bermingham, and Tore Godal for directions on an earlier analysis. We are grateful to Harry Patrinos, Claudio Montenegro, and George Psacharopoulos for sharing data on age-earnings profiles aggregated by income groups.
We thank the International Commission on Financing Global Education Opportunity, which funded this study. We also thank Norad for supporting an initial study of the economic returns of education resulting from reductions in under-five mortality, which was prepared for the Oslo Summit on Education for Development (July 2015).
Notes
World Bank Income Classifications as of July 2015 are as follows, based on estimates of gross national income (GNI) per capita for 2014:
Low-income countries (LICs) = US$1,045 or less
Middle-income countries (MICs) are subdivided:
none a. | lower-middle-income = US$1,046 to US$4,125 |
none b. | upper-middle-income (UMICs) = US$4,126 to US$12,735 |
High-income countries (HICs) = US$12,736 or more.
Since the chapter was written, the income classifications of some countries have changed. As of July 2016, Cambodia is a lower-middle-income country; Senegal is a low-income country; Tonga is a lower-middle-income country, and República Bolivariana de Venezuela is an upper-middle-income country.
- 1
See Schäferhoff and others (2015) for an initial study of the economic results of education from reductions in under-five mortality commissioned by Norad.
- 2
- 3
For a systematic meta-analysis, see Schäferhoff and others (2015). See also, for example, Caldwell (1980); Wagstaff (1993); Filmer and Pritchett (1999); Grossman (2006); Gakidou and others (2010); Gupta and Mahy (2003); Kuruvilla and others (2014); Jamison and others (2013); Jamison, Murphy, and Sandbu (2016); Wang and others (2014).
- 4
Matsumura and Gubhaju (2001) on Nepal; Shkolnikov and others (1998) on the Russian Federation; Hurt, Ronsmans, and Saha (2004) on Bangladesh; Yamano and Jayne (2005) on Kenya; de Walque and others (2005) on Uganda; Lleras-Muney (2005) on the United States; Rowe and others (2005) on Nepal.
- 5
For example, years of schooling for students age 15 years would underestimate their full educational attainment because they are still in school.
- 6
- 7
Conducting a categorical levels analysis would have required data on the length of each level of schooling for each country in each time period (year). For example, one country may define primary school as having a five-year duration, while another may define it as seven years; furthermore, country definitions of levels of schooling change over time. Because we lacked accurate data on levels over time, it was not possible to run such an analysis.
- 8
Program for International Student Assessment (PISA) (OECD 2012); Trends in International Mathematics and Science Study (TIMSS) (Mullis and Martin 2013); Southern and Eastern Africa Consortium for Monitoring Educational Quality (SACMEQ) (Hungi 2011); Program for the Analysis of CONFEMEN Education Systems (PASEC) (PASEC 2015); Latin American Laboratory for Assessment of the Quality of Education: Regional Comparative and Explanatory Study (LLECE) (UNESCO 2015).
- 9
The authors noted that this characterization of rates of return overlooks many of the important returns that might also be associated with improved educational attainment. Furthermore, the social rates of return were highest for tertiary education in UMICs. The authors note that given almost universal primary completion rates in UMICs, there is an unsatisfactory control group of noncompleters to compare with, likely understating returns at the primary level (Psacharopoulos, Montenegro, and Patrinos 2017).
- 10
Our methods build on those used by The Lancet Commission on Investing in Health, which used existing literature to propose a standardized approach to placing dollar values on mortality change. See Cropper, Hammitt, and Robinson (2011); Jamison and others (2013a, 2013b); Viscusi (2015).
- 11
All figures were calculated using a VSL of 130 times GDP per capita. We conducted additional analyses using a VSL of 80 times GDP per capita (lower bound) and 180 times GDP per capita (upper bound). The figures in parentheses refer to these lower- and upper-bound estimates.
References
Angrist N, Patrinos H. A, Schlotter M. 2013. “An Expansion of a Global Data Set on Educational Quality: A Focus on Achievement in Developing Countries.”
Policy Research Working Paper 6356, World Bank, Washington, DC.
Baird S J, Garfein R S, McIntosh C T, Özler B. 2012. “Effect of a Cash Transfer Programme for Schooling on Prevalence of HIV and Herpes Simplex Type 2 in Malawi: A Cluster Randomised Trial.”
The Lancet
379 (9823): 1320–29. [
PubMed: 22341825]
Barro R, Lee J W.
2013. “A New Data Set of Educational Attainment in the World, 1950–2010.”
Journal of Development Economics
104 (September):184–98.
Behrman J A.
2015. “The Effect of Increased Primary Schooling on Adult Women’s HIV Status in Malawi and Uganda: Universal Primary Education as a Natural Experiment.”
Social Science and Medicine Journal
127
108–15. [
PubMed: 24985789]
Behrman J R.
1996. “The Impact of Health and Nutrition on Education.”
World Bank Research Observer
11 (1): 23–37.
Bongaarts J.
2010. “The Causes of Educational Differences in Fertility in Sub-Saharan Africa.”
Vienna Yearbook of Population Research
8
31–50.
Bundy D A P, de Silva N, Horton S, Patton G C, Schultz L, Jamison D T. 2017. “Child and Adolescent Health and Development: Realizing Neglected Potential.” In Disease Control Priorities, Child and Adolescent Health and Development, (third edition): Volume 8 edited by Bundy D A P, de Silva N, Horton S, Jamison D T, Patton G C, editors.
Washington, DC: World Bank.
Caldwell J C.
1980. “Mass Education as a Determinant of the Timing of Fertility Decline.”
Population and Development Review
6 (2): 225–55.
Case A, Fertig A, Paxson C. 2005. “The Lasting Impact of Childhood Health and Circumstance.”
Journal of Health Economics
24 (2): 365–89. [
PubMed: 15721050]
Cropper M, Hammitt J. K, Robinson L A.
2011. “Valuing Mortality Risk Reductions: Progress and Challenges.”
Annual Review of Resource Economics, Annual Reviews
3 (1): 313–36.
Currie J, Hyson R. 1999. “Is the Impact of Health Shocks Cushioned by Socioeconomic Status? The Case of Low Birthweight.”
American Economic Review
89 (2): 245–50.
De Neve J W, Fink G, Subramanian S V, Mayo S, Bar J. 2015. “Length of Secondary Schooling and Risk of HIV Infection in Botswana: Evidence from a Natural Experiment.”
The Lancet Global Health
3 (8): e470–77. [
PMC free article: PMC4676715] [
PubMed: 26134875]
de Walque D, Nakiyingi-Miiro J S, Busingye J, Whitworth J A.
2005. “Changing Association between Schooling Levels and HIV-1 Infection over 11 Years in a Rural Population Cohort in South-West Uganda.”
Tropical Medicine and International Health
10 (10): 993–1001. [
PubMed: 16185233]
Ding W, Lehrer S F, Rosenquist J N, Audrain-McGovern J. 2009. “The Impact of Poor Health on Academic Performance: New Evidence Using Genetic Markers.”
Journal of Health Economics
28 (3): 578–97. [
PubMed: 19217678]
Feenstra R C, Inklaar R, Timmer M P.
2015. “The Next Generation of the Penn World Table.”
American Economic Review
105 (10): 3150–82.
Feinstein L, Sabates R, Anderson T M, Sorhaindo A, Hammond C. 2006. “What Are the Effects of Education on Health?” In Measuring the Effects of Education on Health and Civic Engagement: Proceedings of the Copenhagen Symposium,171–354. Paris: Organisation for Economic Co-operation and Development.
http://www.oecd.org/edu/innovation-education/37425753.pdf.
Filmer D, Pritchett L. 1999. “Child Mortality and Public Spending on Health: How Does Money Matter?”
Social Science and Medicine
49 (10): 1309–23. [
PubMed: 10509822]
Gakidou E, Cowling K, Lozano R, Murray C J.
2010. “Increased Educational Attainment and Its Effect on Child Mortality in 175 Countries between 1970 and 2009: A Systematic Analysis.”
The Lancet
376 (9745): 959–74. [
PubMed: 20851260]
Grossman M.
2006. “Education and Nonmarket Outcomes.”
Handbook of the Economics of Education,577–633. Amsterdam: Elsevier.
Gupta N, Mahy M. 2003. “Adolescent Childbearing in Sub-Saharan Africa: Can Increased Schooling Alone Raise Ages at First Birth?”
Demographic Research
8 (4): 93–106.
Hurt L S, Ronsmans C, Saha S. 2004. “Effects of Education and Other Socioeconomic Factors on Middle Age Mortality in Rural Bangladesh.”
Journal of Epidemiology and Community Health
58 (4): 315–20. [
PMC free article: PMC1732720] [
PubMed: 15026446]
Jamison D T, Jha P, Malhotra V, Verguet S. 2013. “Human Health: The Twentieth-Century Transformation of Human Health: Its Magnitude and Value.” In How Much Have Global Health Problems Cost the World? edited by Lomborg B, editor.
207–46. Cambridge U.K.: Cambridge University Press.
Jamison D T, Summers L H, Alleyne G, Arrow K J, Berkley S., and others. 2013a. “Global Health 2035: A World Converging within a Generation.”
The Lancet
382 (9908): 1898–955. [
PubMed: 24309475]
Jamison E A, Jamison D T, Hanushek E A.
2007. “The Effects of Education Quality on Income Growth and Mortality Decline.”
Economics of Education Review
26 (6): 772–89.
Kenkel D S.
1991. “Health Behavior, Health Knowledge, and Schooling.”
Journal of Political Economy
99 (2): 287–305.
Kuruvilla S, Schweitzer J, Bishai D, Chowdhury S, Caramani D., and others. 2014. “Success Factors for Reducing Maternal and Child Mortality.”
Bulletin of the World Health Organization
92 (7): 533–54. [
PMC free article: PMC4121875] [
PubMed: 25110379]
Lleras-Muney A.
2005. “The Relationship between Education and Adult Mortality in the United States.”
Review of Economic Studies
72
189–221.
Lochner L.
2011. “Nonproduction Benefits of Education: Crime, Health, and Good Citizenship.”
Handbook of the Economics of Education Vol. 4, 183–274. Amsterdam: Elsevier.
Martin T C, Juarez F. 1995. “The Impact of Women’s Education on Fertility in Latin America: Searching for Explanations.”
International Family Planning Perspectives
21 (2): 52–57.
Matsumura M, Gubhaju B. 2001. “Women’s Status, Household Structure and the Utilization of Maternal Health Services in Nepal.”
Asia-Pacific Population Journal
16 (1): 24–44.
Mboup G, Saha T. 1998. “Fertility Levels, Trends, and Differentials.”
DHS Comparative Studies 28, Macro International, Calverton, MD
Montenegro C E, Patrinos H A. 2013. “Returns to Schooling around the World.”
Background paper for World Development Report 2013, World Bank, Washington, DC.
Montenegro C E, Patrinos H A. 2014. “Comparable Estimates of Returns to Schooling around the World.”
Policy Research Working Paper 7020, World Bank, Washington, DC.
Muhuri P K, Blanc A K, Rutstein S O.
1994. “Socioeconomic Differentials in Fertility.”
DHS Comparative Studies 13, Macro International, Calverton, MD.
Oreopoulos P, Salvanes K. 2011. “Priceless: The Nonpecuniary Benefits of Schooling.”
Journal of Economic Perspectives
25 (2): 159–84.
Preston S H. 1975. “The Changing Relation between Mortality and Level of Economic Development.”
Population Studies
29 (2): 231–48. [
PubMed: 11630494]
Preston S H. 2007. “The Changing Relation between Mortality and Level of Economic Development.”
International Journal of Epidemiology
36 (3): 484–90. [
PubMed: 17550952]
Preston S H, Haines M R.
1991. Fatal Years: Child Mortality in Late Nineteenth-Century America. Princeton, NJ: Princeton University Press.
Pritchett L, Summers L. 1996. “Wealthier Is Healthier.”
Journal of Human Resources
31 (4): 841–68.
Psacharopoulos G, Montenegro C E, Patrinos H A.
2017. “Education Financing Priorities in Developing Countries.”
Journal of Educational Planning and Administration
31 (1): 5–16.
Rowe M, Thapa B K, Levine R, Levine S, Tuladhar S S.
2005. “How Does Schooling Influence Maternal Health Practices? Evidence from Nepal.”
Comparative Education Review
49 (4): 512–33.
Sabates R, Feinstein L. 2006. “The Role of Education in the Uptake of Preventative Health Care: The Case of Cervical Screening in Britain.”
Social Science and Medicine
62 (12): 2998–3010. [
PubMed: 16403597]
Schultz T W.
1993. Origins of Increasing Returns. Oxford U.K.: Blackwell Publishers.
Schäferhoff M, Evans D, Burnett N, Komaromi P, Kraus J., and others. 2015. “Estimating the Costs and Benefits of Education from a Health Perspective.”
Prepared for the Oslo Summit on Education for Development, July
6–7.
Shkolnikov V M, Leon D A, Adamets S, Andreev E, Deev A. 1998. “Educational Level and Adult Mortality in Russia: An Analysis of Routine Data 1979 to 1994.”
Social Science and Medicine
47 (3): 357–69. [
PubMed: 9681906]
Stenberg K, Sweeny K, Axelson H, Temmerman M, Sheehan P. 2016. “Returns on Investment in the Continuum of Care for Reproductive, Maternal, Newborn, and Child Health.” In Disease Control Priorities, Reproductive, Maternal, Newborn, and Child Health (third edition): Volume 2 edited by Black R, Laxminarayan R, Temmerman M, Walker N, editors. . Washington, DC: World Bank.
United Nations, Department of Economic and Social Affairs, Population Division. 2015. “World Population Prospects: The 2015 Revision.”
DVD edition.
Viscusi W K.
2015. “The Role of Publication Selection Bias in Estimates of the Value of a Statistical Life.”
American Journal of Health Economics
1 (1): 27–52.
Wagstaff Adam. 1993. “The Demand for Health: An Empirical Reformulation of the Grossman Model.”
Health Economics
2 (2): 189–98. [
PubMed: 8261039]
Wang H, Liddell C A, Coates M M, Mooney M D, Levitz C E., and others. 2014. “Global, Regional, and National Levels of Neonatal, Infant, and Under-5 Mortality during 1990–2013: A Systematic Analysis for the Global Burden of Disease Study 2013.”
The Lancet
384 (9947): 957–79. [
PMC free article: PMC4165626] [
PubMed: 24797572]
Wang J, Jamison D T.
1998. “Regression Residuals as Performance Measures: An Assessment of Robustness in the Context of Country-Level Data Draft”
Presented at the Annual Meeting of the American Educational Research Association, San Diego, CA, April
13–17.
Yamano T, Jayne T S.
2005. “Working-Age Adult Mortality and Primary School Attendance in Rural Kenya.”
Economic Development and Cultural Change
53 (3): 619–53.