NCBI Bookshelf. A service of the National Library of Medicine, National Institutes of Health.
July 2018
INTRODUCTION
On March 6–7, 2018, the National Academies of Sciences, Engineering, and Medicine held the second workshop of a three-part series titled Examining the Impact of Real-World Evidence on Medical Product Development. The workshops are convened under the auspices of the Forum on Drug Discovery, Development, and Translation and are sponsored by the U.S. Food and Drug Administration (FDA). The workshops are intended to advance discussions and common knowledge among key stakeholders about complex issues relating to the generation and use of real-world evidence (RWE). The second workshop focused on practical approaches for the collection of real-world data (RWD)—data generated outside of the traditional clinical trial setting—and the use of RWE.
Workshop discussions centered around three framing questions to help shift the discussion toward practical and generalizable considerations for those embarking on a study that would use RWE to inform decision making in the development and evaluation of medical products:
- 1.
When can decision makers rely on real-world data?
- 2.
When can decision makers rely on real-world treatment?
- 3.
When can decision makers learn from real-world treatment assignment?
Gregory Simon of the Kaiser Permanente (KP) Washington Health Research Institute opened the workshop by explaining that while RWE may mean something different to distinct stakeholders, the goal of the workshop was to discuss possible questions for stakeholders to refer to when considering the use of RWE in a study. The questions suggested by individual workshop participants on Day 2 of the workshop (March 7, 2018) following discussions on Day 1 (March 6, 2018) are listed in Boxes 1–3. The statements, recommendations, and opinions expressed are those of individual presenters and participants and they should not be construed as reflecting any group consensus.
WHEN CAN DECISION MAKERS RELY ON REAL-WORLD DATA?
Novel Oral Anticoagulants (NOACs) in Comparison with Warfarin
Adrian Hernandez of the Duke University School of Medicine presented on a suite of trials that investigate the use of NOACs compared to warfarin, a drug typically used in patients to prevent atrial fibrillation and reduce the risk of stroke. The following trials, which used RWD to compare treatments, served as a starting point for discussion:
- Randomized Evaluation of Long-Term Anticoagulation Therapy (RE-LY) (Connolly et al., 2009);
- Rivaroxaban Once Daily Oral Direct Factor Xa Inhibition Compared with Vitamin K Antagonism for Prevention of Stroke and Embolism Trial in Atrial Fibrillation (ROCKET AF) (Patel et al., 2011);
- Apixaban for Reduction in Stroke and Other Thromboembolic Events in Atrial Fibrillation (ARISTOTLE) (Granger et al., 2011); and
- Effective Anticoagulation with Factor Xa Next Generation in Atrial Fibrillation–Thrombolysis in Myocardial Infarction 48 (ENGAGE AF-TIMI 48) (Giugliano et al., 2013).
Hernandez explained that a 2014 meta-analysis of these trials demonstrated that NOAC treatment led to better outcomes for stroke and thromboembolic events and showed non-inferiority to warfarin. Hernandez said that all four NOAC trials favored NOACs over warfarin when it came to risk of stroke and systemic embolic events, as well as secondary outcomes, such as ischemic stroke, hemorrhagic stroke, myocardial infarction (MI), and all-cause mortality (Ruff et al., 2014). Using the ROCKET trial as an example, Hernandez emphasized the importance of assessing trial quality in order to judge the reliability of the associated outcome measurements and pointed to the frequency of enrolled patients in the therapeutic range for the various treatments as a possible surrogate for trial quality.
In conclusion, Hernandez emphasized that study cost is an important factor. Trials assessing a product early in its lifecycle include significant data collection and thus are expensive, whereas studies assessing a product later in its lifecycle—but still aiming to include an indication—can streamline study protocol and data collection, leading to lower costs. Hernandez ended by posing a question for consideration: What questions characterize the utility of an RWD source and signal reliability before a study is performed?
Discussion
During the discussion session, Hernandez and Jesse Berlin of Johnson & Johnson emphasized the importance of predetermining the population, exposure, and outcome of interest when conducting a study using RWD. Hernandez argued that careful data curation and characterization are necessary to reduce systematic bias. Simon agreed, calling for ongoing data curation and monitoring. Similarly, some panelists and participants discussed validating data as a crucial step in using RWD for research. Berlin said that coding is not always accurate, so validated algorithms and methods should be used to accurately identify patient cohorts. He also suggested that a library of validated algorithms to use during large-scale studies would be a useful research tool. Berlin and other participants also raised the point that algorithms may change over time because coding may change over time (including the introduction of new versions of coding and the implementation of new coding terminology). Robert Califf of Verily Life Sciences and Duke University said that when validating the quality of data researchers must be aware that there are multiple sources of data for one individual and it may actually be irresponsible to ignore RWD because non-traditional sources could actually capture more accurate information about a person (e.g., such as in the case of sensitive information he or she may not share with study coordinators).
Hui Cao of Novartis noted that there are some common “rules” that could help assess uncertainty in RWD sources. For example, for certain diseases there are established algorithms that identify data for a given patient population. She pointed out that exposure can be predicted with a high level of confidence for injection or intravenous administration, but for oral drugs or inhaled products—for which exposure may be harder to determine—researchers may need to rely more on larger sample sizes. Cao pointed out that different metrics for determining outcome will vary in terms of accuracy. For example, certain events or lab measures—such as hospitalization for MI and HbAC1 measure—can be identified with higher accuracy than other events or measures such as moderate asthma exacerbations and lung function.
Khaled Sarsour of Genentech commented on the lack of alignment between randomized controlled trial (RCT) endpoints and RWE endpoints, noting that RCT endpoints are not always relevant to real-world practice. Hernandez highlighted that there should be more consideration for patient preferences and experience in medical practice. Gregory Daniel of the Duke-Margolis Center for Health Policy remarked that this misalignment only highlights the importance of RWE as a tool for a diverse group of stakeholders, including patients, payers, and providers, and the need to utilize the full suite of available evidence to evaluate a medical product.
Daniel brought to attention the use of data from mobile apps and other new sources. Hernandez said that patient-imported data require further consideration and integration in the evidence generation system. However, Daniel and Christina Stephan of the American Medical Informatics Association warned that it is hard to rely on mobile data in particular when social determinants and lifestyle factors, such as the presence of pets or the misuse of mobile devices, affect the accuracy of the data. John Burch of the Mid-America Angels Investment Group noted that existing data sources will continue to change over time and the methods used to extract and analyze data will have to change as well.
Cathy Critchlow of Amgen asked about the role of pre-specifying data analysis methodology and its necessity during the collection and analysis of RWD. Hernandez suggested that this should be done as much as possible. He emphasized that pre-specification and other efforts to create transparency around data collection make RWD much more trustworthy.
Discussion of Potential Questions Around Real-World Data
On Day 2 of the workshop, individual participants discussed potential questions for consideration when contemplating the use of RWD in a study. The starting point for the discussion was the central question from session 1: When can decision makers rely on real-world data?
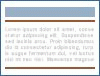
BOX 1
When Can Decision Makers Rely on Real-World Data? Questions Raised by Individual Participants on Day 2 of the Workshop
During the Day 2 discussion, several participants discussed incomplete ascertainment, due to health system issues (e.g., data fragmentation) and the likelihood of certain events occurring outside of a patient's typical health system, as a possible source of bias when collecting data. Other participants mentioned the direction of possible misclassification, given the knowledge that random misclassification biases result in different directions based on the study type (non-inferiority versus superiority), as another potential source of bias during data collection.
On the topic of expert adjudication, Hernandez mentioned that tracking minor events such as nosebleeds or bruising is a much different task than tracking significant events like major bleeding. He noted that the data collection and reporting process is often much more uncertain and variable across institutions for minor events. Joanne Waldstreicher of Johnson & Johnson pointed out there are many examples of the use of expert adjudication in the cardiovascular field. Grazyna Lieberman of Genentech commented on the importance of transparency around data sources, including the source itself, the procedures used to extract data, the completeness and accuracy of the data, and the consistency of the data collection methodology used across research.
Elise Berliner from the Agency for Healthcare Research and Quality addressed the collective need for better data and data infrastructure, recognizing that this affects not only industry and FDA but also payers. Simon agreed that even with different uses for data—clinical, quality improvement, payment, product comparison, etc.—there is shared interest and alignment across the health care system in the quality of original data. Echoing comments from Day 1, Andy Bindman of the University of California, San Francisco, said that certifying rules around the curation of data at the front end of collection could be useful. Jacqueline Corrigan-Curay of FDA commented that there should be a greater focus on prospective data collection and analysis, rather than just retrospective data.
Last, Frank Sifakis of AstraZeneca noted the importance of increased data collection for the purpose of routine business decisions, as well as supporting providers and patients in making treatment decisions. Sheila Weiss from Evidera said that while many companies already engage in such collection, the next step should be leveraging that data to identify appropriate situations when observational data can be used to make decisions about a product's efficacy or effectiveness.
WHEN CAN DECISION MAKERS RELY ON REAL-WORLD TREATMENT?
Lithium for Suicidal Behavior in Mood Disorders: A Classic RCT Addressing a Real-World Question
Ira Katz of the Corporal Michael J. Crescenz Veterans Affairs Medical Center presented on the U.S. Department of Veterans Affairs (VA) study investigating the use of lithium for the prevention of suicide among VA patients with major depression.1 Katz explained that the use of lithium to prevent suicide has long been hypothesized, but it has been difficult to carry out an RCT due to safety and treatment control concerns. Previous RCTs were subject to a negative indication bias due to the sensitivity of lithium dosing. Katz said at least two prior studies were terminated because of issues with recruitment, but previous research indicated support for the hypothesis that lithium can prevent suicide among patients with bipolar disorder and depression.
Katz explained that the VA is the ideal setting for a trial on this topic because of its large population of study-qualified patients and numerous study centers dispersed nationwide. While depression is required in the inclusion criteria for the study, patients with comorbidities—including histories of substance abuse and posttraumatic stress disorder—are allowed as well. Doing so, Katz said, is an admission that most patients at risk for suicide experience co-morbidities and investigators should consider how previous RCTs in this field have filtered out patients who are at risk for suicide primarily because of comorbidities.
To determine the effect size needed for the study, Katz said that study investigators surveyed health care providers about the necessary effect size to modify practice, which indicated that a fairly low effect size would still be an improvement. Even with this threshold, monitoring patients can be complex. For example, Katz cited a patient who was recruited for the trial and who received shipments of the medication despite not submitting monthly blood samples. In this case, the study care team followed good clinical management for the patient rather than the requirements of the RCT. Katz concluded by emphasizing a key monitoring question for studies being conducted in real-world settings: How can investigators reconcile the need to remain flexible while caring for patients with complex needs and the need to adhere to strict protocol in an RCT setting?
Discussion
During the subsequent discussion, panelists and participants considered the lithium use case presented by Katz as well as the NOACs example presented earlier by Hernandez, which also illuminated considerations for real-world treatment, such as choosing an appropriate standard of care to compare to an investigational treatment, in addition to the data reliability issues discussed previously.
Workshop participants discussed desired standards of care in real-world treatment studies. Simon said that generally, care used in research should be at least as good as the typical standard of care in the real world. However, Simon, Hernandez, and Michael Horberg of the KP Mid-Atlantic Permanente Medical Group pointed out that “standard of care” varies significantly across different health systems and regions in the United States as well as abroad. Ultimately, Simon noted, it is the responsibility of researchers to identify and implement a reasonable standard of treatment for RWE studies. Simon and Jonathan Watanabe of the University of California, San Diego, also emphasized that the control treatment should be “better than average” in the real world. Watanabe noted that the real world is not static and therefore the comparator treatment should include a “floor” that is up to par with an ever-changing treatment environment, especially considering the variability in standards across clinical environments.
Sarsour addressed the topic of safety monitoring in real-world trials and noted that the value of safety monitoring is dependent on the lifecycle phase of the drug of interest. Lithium, he noted, has been used for decades and it is unlikely any major new safety information will come out of the VA's study. Although Califf pointed out that collecting data on non-serious adverse events for treatments already used widely can be costly, Katz said that collecting that information—including events not related to the study outcomes—can still be useful because it may serve as an internal control indicative of the level of care in a particular study.
Participants grappled with concerns about patient behavior and adherence to treatment framed by Katz's presentation. Expounding on the topic of complex patients, Simon and Horberg discussed non-adherent behavior in trials. Simon said that while human behavior, behavioral error, and comorbidities make science harder, they are also components of the real world that can make results more generalizable and relevant.
Horberg pointed to an example from a recent trial for pre-exposure prophylaxis (PrEP) in HIV prevention used by sero-discordant couples, during which couples were consistently advised to use condoms, but more than 100 pregnancies still occurred. The researchers took advantage of these situations to measure the couples for HIV transmission and assess the real-world effectiveness of the treatment. He said that this type of human behavior should generally be expected in real-world trials and suggested that real-world patient behavior should be incorporated into RWE studies and assessed as an endpoint to judge the success of a particular treatment.
While discussing NOACs, Robert Temple of FDA said that simpler care—as long as it does not come at the expense of quality—can help diminish problems around adherence. He highlighted long-acting injectable anti-psychotic drugs in contrast to oral anti-psychotic medication as an example. Sebastian Schneeweiss of Brigham and Women's Hospital and Harvard Medical School asked how much discretion regulators have to regulate clinical strategies around certain products that could be observed with RWD versus a specific molecule in a narrow sense. Ultimately, Temple and Horberg said the health care system is responsible for problems around patient adherence and compliance, a reality that is not acknowledged often enough. Sarsour suggested that adherence and compliance should be prioritized as the research community moves toward a more patient-centered approach.
Building off of the theme of compliance, Simon mentioned the concept of a “randomized nudge” trial as a possible solution for poor patient adherence. The trial framework would allow investigators to “nudge” prospective patients and providers toward a certain treatment, notifying them that a successful treatment is available for use without necessarily assigning them to a specific treatment group. Simon referenced the IMPACT-AFib trial2 (Implementation of an RCT to Improve Treatment with Oral Anticoagulants in Patients with Atrial Fibrillation), which leverages this concept. Simon and Temple also debated the use of secondary randomization, in which a randomized group of “nudged” patients is compared to a group that is not “nudged,” and whether these types of randomized trials could help untangle confusion around confounding by indication versus compliance.
Several panelists and participants discussed the importance of blinding during different stages of the study cycle, including patient and/or provider blinding during treatment assignment, blinding of treatment group during analysis, and the blinding of assessors during outcome assessment. Simon argued that blinding of outcome assessment is critical, because the potential introduction of bias during outcome assessment is detrimental to trial results. Blinding during analysis is also important and could be done better, he said. Blinding patients and providers, however, may not be necessary and could introduce distortion of both the patient population in the trial and the true nature of the treatment. Katz commented that partially randomized patient preference designs would alleviate those distortions, offering an opportunity for those who declined participation in a blinded study the ability to choose a treatment, after which investigators could compare the outcome of the randomized group and the non-randomized group.
Critchlow asked how blinding affects clinical equipoise and whether the perception of equipoise could shift over the course of a study. She pointed out that accumulating information and potentially attributing it to one treatment could be particularly biased because the perceived effects may or may not be real. Simon related this question to the concept of unbiased outcome ascertainment, stating that if beliefs about a treatment would influence the reporting or ascertainment of the outcome, blinding becomes absolutely necessary. Overall, Simon and Temple both stressed the importance of blinding during outcome assessment but questioned its value for patients and providers during treatment, especially if it alters behavior. Watanabe pushed back against the value of the limited use of blinding. He said that if investigators make the effort to blind analysts and raters during outcome assessment to reduce bias, it seems like minimal investment to blind prescribers as well, because one could imagine a prescriber preferring a NOAC to warfarin because NOACs are less labor intensive to administer, thus biasing the results.
Individual workshop participants discussed some parameters of studies or treatments that could suggest when blinding would be helpful. Bill Potter of the National Institute of Mental Health commented that the need for blinding may change based on the subjectivity of the outcome being measured; for example, amyloid deposition would not change regardless of blinding. Hernandez observed that while blinding can ensure consistent behavior by patients and providers, it can also create a more homogeneous study population because of the burden of treatment and the possibility of receiving a placebo. Jennifer Graff of the National Pharmaceutical Council, discussing systemic bias in real-world treatment, mentioned that health care systems can encourage patients to behave a certain way or select certain treatments for non-clinical purposes. For example, reimbursement rules could nudge patients to choose one treatment option over another. Temple suggested there may be different considerations around blinding in high-risk versus low-risk populations.
Discussion of Potential Questions Around Real-World Treatment
On Day 2 of the workshop, individual participants discussed potential questions for consideration when contemplating the role of real-world treatment in research. The starting point for the discussion was the central question from session 2: When can decision makers rely on real-world treatment?
During the discussion on real-world treatment during Day 2 of the workshop, several participants discussed possible lessons and considerations about study conditions in which patient self-monitoring is acceptable and safe. Some mentioned the potential effect of research location and control group selection (including the average quality of care for the control group) on results. Others mentioned the importance of distinguishing between bad outcomes and bad treatment and the likely role of simpler care protocols making trial results easier to interpret. Some participants discussed the ascertainment of treatment effects from different populations and commented that ascertainment is often easier from low-risk populations despite the possible ethical necessity of including high-risk populations in research. Last, several speakers said that from a research or regulatory perspective, collecting data on non-serious adverse events is not useful late in a product's lifecycle, but it can serve as a built-in checkpoint that patients are being cared for appropriately.
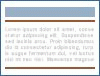
BOX 2
When Can Decision Makers Rely on Real-World Treatment? Questions Raised by Individual Participants on Day 2 of the Workshop
WHEN CAN DECISION MAKERS LEARN FROM REAL-WORLD TREATMENT ASSIGNMENT
Health Care Database Analyses of Medical Products for Regulatory Decision Making
Schneeweiss presented on non-interventional health care database analyses and their potential for the development of RWE. Schneeweiss described that within the world of biomedical data, there are broad categories, including RCT-derived data and non-interventional data. Non-interventional data can be broken down further into research data and transactional data (e.g., data from a claims database). Schneeweiss explained that transactional data is crucial to understand the benefits and risks of medical products outside of controlled research investigations in routine care settings.
Schneeweiss explained that given the expanding role of RWE in regulatory decision making—supporting secondary indications, adaptive pathways, safety, and other areas—the use of RWD analyses is expanding; in a project among Aetion, Inc.; Brigham and Women's Hospital; and FDA, 30 RCTs that were designed for drug approvals are in the process of being identified for reproduction by database studies to understand how well the results can be predicted with RWD. Schneeweiss stated that when conducting RWD studies, discovering the unintended—including harmful—effects of a product can usually be executed with higher confidence than analyzing intended product effects because there is less patient selection regarding the unknown unintended effect, which reduces confounding.
Schneeweiss acknowledged that investigators prefer RCTs because of the amount of control and transparency they offer: RCTs include random treatment assignment, a controlled outcome measurement, and often clear and simple study implementation. However, he emphasized that there are certain situations that have a higher likelihood of being able to be studied with database analyses. These situations include
- An active comparator (which reduces confounding by design);
- Measurable exposures and outcomes; and
- Measurable confounders.
Schneeweiss cited the ONTARGET trial (which compared telmisartan to ramipril for the reduction of cardiovascular risk) as an ideal example to meet these conditions for RWD replication (Fralick et al., 2018; Yusuf et al., 2008), as demonstrated in an article he co-authored in 2018 describing the near replication of results from the ONTARGET trial using a health care database analysis. He also noted that transparent and structured reporting of the complex methodologies used in RWD studies is a way to achieve confidence when comparing non-randomized studies to RCTs. Emerging analytic tools are now built for transparency and reproducibility; meanwhile, line programming is considered error prone, is not able to be validated at scale, lacks transparency, and is hard to reproduce by other researchers. Ultimately, Schneeweiss posed several critical questions to workshop participants:
- Which questions are answerable using RWD analyses versus only RCTs?
- Are non-interventional RWD study protocols precise enough to translate intention into action?
- What are the factors that must be present to make database analyses reproducible?
To conclude, Schneeweiss presented a list of questions to consider—which he framed as a pathway—for embarking on an RWD analysis:
- Is the setting adequate for an RWD analysis?
- Is data quality fit for purpose?
- Is the data analysis plan based on epidemiologic study design principles?
- Was balance in confounding factors between treatment groups achieved?
Discussion
Panelists discussed the role of randomization in clinical research. Califf said that while many single-armed studies already exist, the medical research community should embrace the use of randomization more than in the current environment, contrasting the lack of randomization in medical research to its widespread use among Silicon Valley technology companies and other business environments. However, he noted that randomization is not widely used in oncology research or accelerated treatment approvals and there may be applicable lessons to learn from that work. David Madigan of Columbia University acknowledged that while randomization is a key tool in clinical trial study design, there is a desperate need for more evidence to answer questions when RCTs simply are not a realistic solution, whether due to cost or feasibility. He offered assessing the long-term effects of bisphosphonates, a class of drugs used to prevent the loss of bone density, as an example.
Additionally, Madigan noted that randomization solves the central issue of unmeasured confounding, but it cannot overcome data source–derived problems such as missing data or systemic bias. Madigan said that in observational studies, propensity score matching—as first described in work by Paul Rosenbaum and Donald Rubin (Rosenbaum and Rubin, 1983)—can be used to mimic the effect of randomization on the dataset. Propensity scoring, he said, attempts to estimate the probability of being in a certain treatment group based on a variety of covariates, such as age, sex, pre-existing conditions, or comorbidities. Madigan also noted that a propensity score analysis would ideally be paired with a gamma analysis, a secondary analysis developed by Rosenbaum that quantifies the necessary size of an unmeasured confounder to invalidate study results. This type of analysis would make it easier to interpret propensity score matching and would also help both evidence generators and evidence users think more comprehensively about a study, Madigan said. Sarsour agreed that confounding by indication is often the key barrier to successful observational studies and said that better characterization and accounting for confounders would make observational studies more feasible. Martin stated that analyses like this would enable a thoughtful and quantitative consideration of both observational study results, as well as clinical phenomena that could be relevant and important enough to change the findings of a study.
Madigan and Schneeweiss agreed that the research community must overcome the barrier of unmeasured confounding by setting standards around data use. Schneeweiss noted that FDA's Sentinel Initiative includes tools for expedited bias sensitivity analyses. Session moderator Richard Platt of Harvard Medical School reiterated this point, highlighting that the Sentinel Initiative articulates well-established principles of data use in advance of a study, requires transparent study designs, and incorporates data interpretation measures that take into account plausible alternative explanations for findings.
Madigan, Schneeweiss, and Platt mentioned a lack of reproducibility of some study results as an important factor reducing confidence in non-interventional RWD analyses. Referring to a commentary he wrote in the Journal of the American Medical Association (Califf, 2018), Califf warned that the interested community will need to avoid allowing researchers to conduct observational analyses repeatedly and in isolation until they find answers that match with RCT evidence. He said that with this concern looming, it is still difficult to trust observational data analyses as independently valuable compared to RCTs. Temple wondered how one could control for this problem and David Martin of FDA said that transparency in study approach is one important way to address it. Platt also noted that pre-specifying—as he described when discussing Sentinel—is one way to mitigate this concern. Simon suggested an “all by all” approach: Rather than limiting the number of analyses, the community could include and compare all analyses conducted. Simon said this approach would act as “simulating the null distribution.”
Cao commented on the perceived value of prospective observational studies. Cao said there is value in the studies if they leverage existing platforms and do not add to the burden of data collection already in place, but otherwise questioned the value of prospective observational work. Martin responded that he views prospective observational studies as one option in a broader menu of potential study designs. He also noted that a potential strength of prospective observational studies might be that they are better able to incorporate patient preferences through the realignment of incentives.
Graff asked how the business ecosystem will be able to use and trust health data in a machine-learning environment. Madigan noted that there is a large body of work on large-scale predictive modeling in health care and there has been substantial progress in recent years. Schneeweiss mentioned an example, referring to a case in which a health plan considered switching to a more expensive anti-diabetic drug that would have reduced total cost of care based on robust RWD analyses. He argued that it is critical for health plans and other business entities to utilize health care data appropriately, because they can positively affect both a company's bottom line and clinical outcomes. In the case of this particular anti-diabetic drug, senior decision makers relied on RWE produced with their own data using an advanced RWD analytic platform. This switch ultimately resulted in better health outcomes and reduced the total cost of care for the health plan.
Watanabe asked how pharmacy benefit managers and other stakeholders could make the outcomes of RWD studies more central to the needs of real-world populations, given that populations analyzed in studies are often not reflective of the real world. Platt said there is more variation in the data available to researchers today than there was even a decade ago. Schneeweiss argued that the heterogeneity in analytic findings from health care databases today stems mostly from the provision of health care and its recording, rather than true genetic variation; he said that treatment administration as a clinical strategy, such as deciding dose adjustments or encouraging adherence, should become a larger focus.
Martin concluded Day 1 of the workshop by comparing current work around RWD analysis to a 1984 paper on large simple trials (Yusuf et al., 1984), which hinted at underlying issues in the big data environment. He said the authors wrote that the effects of treatments on major endpoints are easier to operationalize in major trials and Martin observed that there is a corollary now when considering outcomes based on claims and electronic health record data. Schneeweiss also emphasized that when conducting RWD analyses it is important to consider both the “when” and the “how” questions: There is established knowledge on how to conduct such studies, but the question of when such analyses will likely produce valid findings will depend on the study question and the available data.
Discussion of Potential Questions Around Treatment Assignment and Randomization
On Day 2 of the workshop, individual participants discussed potential questions for consideration when contemplating the use of treatment assignment. The starting point for discussion was the central question from session 3: When can decision makers learn from real-world treatment assignment?
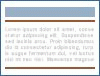
BOX 3
When Can Decision Makers Learn from Real-World Treatment Assignment? Questions Raised by Individual Participants on Day 2 of the Workshop
During the question-generating discussion on Day 2 of the workshop, several workshop participants considered the question of when there could be confidence in inference from non-randomized comparisons and discussed possible conditions, including the expectation of large effects, long study durations and the assessment of long-term outcomes, and other situations when RCTs are not practical. Other speakers pointed out additional conditions, such as when the reliability of the effect is dependent on clinical observation and the selection of historical comparators; when outcome is proximal to treatment; when there is a high degree of similarity between comparison groups; when the pathway from treatment to outcome is relatively clear (without complexity or reciprocal effects); and when treatment allocation methods are relatively transparent.
Simon mentioned prospective treatment assignment and unmeasured confounding in the absence of randomization as high-priority concerns. He also acknowledged that randomization is difficult to implement because it is expensive, time consuming, and often unpopular among both patients and providers. Building on a topic from Day 1 of the workshop, Temple and Mark McClellan of the Duke-Margolis Center for Health Policy discussed the potential use of “randomized nudge” trials as a way to improve patient adherence during studies.
CLOSING REMARKS
McClellan concluded the workshop by thanking the participants and reminding them that this workshop, the second in a three-part series, is part of a larger activity examining the impact of RWE on medical product development. He noted that the third workshop, scheduled to take place on July 17–18, 2018, will move the conversation forward from practical approaches to applications for the collection and use of RWE.
REFERENCES
- Califf RM. Comparison of observational data and the ONTARGET results for telmisartan treatment of hypertension: Bull's-eye or painting the target around the arrow? JAMA Internal Medicine. 2018;178(1):63–65. [PubMed: 29159401]
- Connolly SJ, Ezekowitz MD, Yusuf S, Eikelboom J, Oldgren J, Parekh A, Pogue J, Reilly PA, Themeles E, Varrone J, Wang S, Alings M, Xavier D, Zhu J, Diaz R, Lewis BS, Darius H, Diener HC, Joyner CD, Wallentin L. Dabigatran versus warfarin in patients with atrial fibrillation. New England Journal of Medicine. 2009;361(12):1139–1151. [PubMed: 19717844]
- Fralick M, Kesselheim AS, Avorn J, Schneeweiss S. Use of health care databases to support supplemental indications of approved medications. JAMA Internal Medicine. 2018;178(1):55–63. [PMC free article: PMC5833514] [PubMed: 29159410]
- Giugliano RP, Ruff CT, Braunwald E, Murphy SA, Wiviott SD, Halperin JL, Waldo AL, Ezekowitz MD, Weitz JI, Špinar J, Ruzyllo W, Ruda M, Koretsune Y, Betcher J, Shi M, Grip LT, Patel SP, Patel I, Hanyok JJ, Mercuri M, Antman EM. Edoxaban versus warfarin in patients with atrial fibrillation. New England Journal of Medicine. 2013;369(22):2093–2104. [PubMed: 24251359]
- Granger CB, Alexander JH, McMurray JJV, Lopes RD, Hylek EM, Hanna M, Al-Khalidi HR, Ansell J, Atar D, Avezum A, Bahit MC, Diaz R, Easton JD, Ezekowitz JA, Flaker G, Garcia D, Geraldes M, Gersh BJ, Golitsyn S, Goto S, Hermosillo AG, Hohnloser SH, Horowitz J, Mohan P, Jansky P, Lewis BS, LopezSendon JL, Pais P, Parkhomenko A, Verheugt FWA, Zhu J, Wallentin L. Apixaban versus warfarin in patients with atrial fibrillation. New England Journal of Medicine. 2011;365(11):981–992. [PubMed: 21870978]
- Patel MR, Mahaffey KW, Garg J, Pan G, Singer DE, Hacke W, Breithardt G, Halperin JL, Hankey GJ, Piccini JP, Becker RC, Nessel CC, Paolini JF, Berkowitz SD, Fox KAA, Califf RM. Rivaroxaban versus warfarin in nonvalvular atrial fibrillation. New England Journal of Medicine. 2011;365(10):883–891. [PubMed: 21830957]
- Rosenbaum PR, Rubin DB. The central role of the propensity score in observational studies for causal effects. Biometrika. 1983;70(1):41–55.
- Ruff CT, Giugliano RP, Braunwald E, Hoffman EB, Deenadayalu N, Ezekowitz MD, Camm AJ, Weitz JI, Lewis BS, Parkhomenko A, Yamashita T, Antman EM. Comparison of the efficacy and safety of new oral anticoagulants with warfarin in patients with atrial fibrillation: A meta-analysis of randomised trials. The Lancet. 2014;383(9921):955–962. [PubMed: 24315724]
- Yusuf S, Collins R, Peto R. Why do we need some large, simple randomized trials? Statistics in Medicine. 1984;3(4):409–420. [PubMed: 6528136]
- Yusuf S, Sleight P, Anderson C, Teo K, et al. Telmisartan, ramipril, or both in patients at high risk for vascular events. New England Journal of Medicine. 2008;358(15):1547–1559. for the ONTARGET Investigators. [PubMed: 18378520]
Footnotes
- 1
More information about the study is available at https:
//ClinicalTrials .gov/ct2/show/NCT01928446 (accessed May 16, 2018). - 2
More information about the study is available at https:
//clinicaltrials .gov/ct2/show/NCT03259373 (accessed May 17, 2018).
DISCLAIMER:
This Proceedings of a Workshop—in Brief was prepared by Ben Kahn, Amanda Wagner Gee, and Carolyn Shore as a factual summary of what occurred at the workshop. The statements made are those of the rapporteurs or individual workshop participants and do not necessarily represent the views of all workshop participants; the planning committee; or the National Academies of Sciences, Engineering, and Medicine.
REVIEWERS:
To ensure that it meets institutional standards for quality and objectivity, this Proceedings of a Workshop—in Brief was reviewed by Elise Berliner, Agency for Healthcare Research and Quality; and Hui Cao, Novartis. Lauren Shern, National Academies of Sciences, Engineering, and Medicine, served as the review coordinator.
SPONSOR:
This workshop was partially supported by the U.S. Food and Drug Administration.
For additional information regarding the workshop, visit http://nationalacademies.org/hmd/Activities/Research/DrugForum/2018-MAR-06.aspx.
Suggested citation: National Academies of Sciences, Engineering, and Medicine. 2018. Examining the impact of real-world evidence on medical product development: II. Practical approaches: Proceedings of a workshop—in brief. Washington, DC: The National Academies Press. doi: https://doi.org/10.17226/25176.
Health and Medicine Division
The National Academies of SCIENCES • ENGINEERING • MEDICINE
The nation turns to the National Academies of Sciences, Engineering, and Medicine for independent, objective advice on issues that affect people's lives worldwide.
Rapporteurs: Carolyn Shore, Amanda Wagner Gee, and Ben Kahn.
- NLM CatalogRelated NLM Catalog Entries
- PMCPubMed Central citations
- PubMedLinks to PubMed
- Review Examining the Impact of Real-World Evidence on Medical Product Development: I. Incentives: Proceedings of a Workshop—in Brief[ 2018]Review Examining the Impact of Real-World Evidence on Medical Product Development: I. Incentives: Proceedings of a Workshop—in BriefNational Academies of Sciences, Engineering, and Medicine, Health and Medicine Division, Board on Health Sciences Policy, Forum on Drug Discovery, Development, and Translation. 2018 Feb 12
- Review Examining the Impact of Real-World Evidence on Medical Product Development: Proceedings of a Workshop Series[ 2019]Review Examining the Impact of Real-World Evidence on Medical Product Development: Proceedings of a Workshop SeriesNational Academies of Sciences, Engineering, and Medicine, Health and Medicine Division, Board on Health Sciences Policy, Forum on Drug Discovery, Development, and Translation, Shore C, Gee AW, Kahn B, Forstag EH. 2019 Feb 6
- Review Real-World Evidence Generation and Evaluation of Therapeutics: Proceedings of a Workshop[ 2017]Review Real-World Evidence Generation and Evaluation of Therapeutics: Proceedings of a WorkshopNational Academies of Sciences, Engineering, and Medicine, Health and Medicine Division, Board on Health Sciences Policy, Forum on Drug Discovery, Development, and Translation. 2017 Feb 15
- Review Advancing the Science of Patient Input in Medical Product R&D: Towards a Research Agenda: Proceedings of a Workshop—in Brief[ 2018]Review Advancing the Science of Patient Input in Medical Product R&D: Towards a Research Agenda: Proceedings of a Workshop—in BriefNational Academies of Sciences, Engineering, and Medicine, Health and Medicine Division, Board on Health Sciences Policy, Forum on Drug Discovery, Development, and Translation. 2018 Dec 13
- Review Impact of Real-World Data on Market Authorization, Reimbursement Decision & Price Negotiation.[Ther Innov Regul Sci. 2021]Review Impact of Real-World Data on Market Authorization, Reimbursement Decision & Price Negotiation.Pulini AA, Caetano GM, Clautiaux H, Vergeron L, Pitts PJ, Katz G. Ther Innov Regul Sci. 2021 Jan; 55(1):228-238. Epub 2020 Aug 28.
- Examining the Impact of Real-World Evidence on Medical Product DevelopmentExamining the Impact of Real-World Evidence on Medical Product Development
Your browsing activity is empty.
Activity recording is turned off.
See more...