INTRODUCTION
Populations across the world are changing in size and structure, driving prominent social, economic, and health shifts. In some countries, working-age populations are expanding to unprecedented sizes (absolutely and relatively), creating strong potential for economic growth and development (Bloom et al., 2003) along with the threat of greater unemployment and unrest (Easterlin, 1978). In other countries, population aging is creating large older-age populations, increasing older individuals' influence in societies and families (Preston, 1984) while simultaneously stressing health and pension systems (Bongaarts, 2004). Population aging has already had widespread impact, fueling concerns that aging will substantially burden families, communities, and governments. These concerns have prompted increased research3 and policy making on aging. Indeed, a 2009 survey of 970 population scientists revealed that most experts believe population aging is the most important “population issue” to study over the coming decades (Van Dalen and Henkens, 2012).
The pattern and pace of population aging vary substantially in different settings. Many high-income countries (HICs) already have sizable older-age populations, while many low- and middle-income countries (LMICs) remain relatively young. Looking to the future, population aging is expected to boom in large LMICs such as India and China and slow in the already-aged countries of Western Europe (United Nations Department of Economic and Social Affairs, 2017). Furthermore, causes of aging differ, even among countries with similar rates of aging, with the differences driven by mortality reductions in some settings and by the aging of large cohorts (like the Baby Boomers) in others. These differences have important demographic implications and suggest very different policy responses across countries. Thus, planning for global population aging requires consideration of the historical and future rates of population aging, the forces driving this aging, and their variance across countries and regions. This paper's first major goal is to show how population aging and its causes have differed historically across regions and income groups and how they will evolve in the future.
The challenges of population aging arise because older individuals tend to make smaller economic contributions and have greater needs than younger, working-age people. For example, older individuals are more likely to have functional limitations (Lin et al., 2012), require assistance for everyday tasks (Freedman and Spillman, 2014), and fall sick and suffer from health shocks and hospitalizations (Fry et al., 2005). That older individuals have lower labor income compounds the challenge of these rising age-related needs (Lee, 1994); therefore, families and governments often shoulder the costs (both market and nonmarket) of their needs. In countries with sufficient wealth, the adverse economic consequences of aging can be prevented if health and social security systems can adequately meet the needs of older individuals. However, in LMICs, where aggregate levels of wealth are much lower and welfare systems function poorly or may not exist, the financial burden of aging is more likely to fall on families or the older individuals themselves. This potential for aging without resources to care for older individuals has fueled concerns that LMICs are “growing old before rich.” The paper's second main goal is to explore the extent to which current economic and demographic trends support this view.
Most population-aging metrics are based on a chronological conception of age. Researchers, governments, and global policy makers focus on indicators, such as the share of the population above the age of 60 or 70, with the idea that these chronological measures indicate the size of the dependent population. Within this view, 10 percent growth in the share of individuals over 60 is thought to represent 10 percent growth in the dependent population, resulting in a corresponding increase in the resources required to support them. Yet not all individuals above the age of 60 are dependent, and conversely not all individuals younger than 60 are fully independent. Thus, these conventional measures of population aging may inaccurately indicate functional aging, and policies based on these measures may inadequately meet or may exceed the needs of aging populations. A small but influential series of papers has examined the macro-level discrepancies between chronological and functional aging. Lutz et al. (2008) compared chronological measures of population aging with measures based on the population share with a remaining life expectancy of 15 years and found that the magnitude of population aging is smaller when also considering changing patterns of longevity. Similarly, Sanderson and Scherbov (2010) compared chronological measures of aging (the old-age dependency ratio) with similar ratios defined by the population share with a disability in 10 HICs. They concluded that chronological measures greatly overstate the growth in the share of the dependent population (i.e., the share with a disability). The results from these papers suggest that focusing on chronological age may misrepresent actual changes in the share of the population with functional limitations. In the spirit of this work, the final section of this paper will draw on emerging rich microdata from several Health and Retirement Study (HRS) sister studies across the globe to estimate and compare chronological and functional age in three HICs and five LMICs. This section will also explore changes in the relationship between chronological and functional age over time in Mexico and Indonesia.
Our paper proceeds as follows. In the second section, we document sources of chronological population aging for recent historical and future periods by applying decomposition methods to macro-level data from the United Nations World Population Prospects, The 2017 Revision (hereafter referred to as UN WPP; United Nations Department of Economic and Social Affairs, 2017). In the third section, we discuss the issue of LMIC populations “growing old before rich” and conduct a simple empirical exercise to determine how much support we find for that view. We then shift our perspective away from macro-level chronological measures of aging in the fourth section by using microdata from the Health and Retirement Study sister studies to examine differences in functional aging across five LMICs and three HICs. Together, these distinct analyses provide a robust description of the differences in population aging occurring across income groups and regional settings. In the concluding section, we discuss the implications of our findings for future research and policy on the global demography of aging.
CHRONOLOGICAL AGING
Countries across the globe are aging; however, the rate of population aging varies tremendously across regions and countries at different levels of development. For example, the share of older individuals grew substantially over the past 50 years in HICs and in many countries in Asia. In contrast, countries in sub-Saharan Africa experienced virtually no aging over this same period (United Nations Department of Economic and Social Affairs, 2017). While these trends and patterns of population aging are well established, less is known about the historical and future sources of population aging across countries. To our knowledge, only one paper has examined this issue: Preston and Stokes (2012) used variable-r techniques to decompose the growth rate of the elderly population between 2005 and 2010. They concluded that mortality reductions across cohorts drove aging in more-developed countries, while fertility declines played a larger role in less-developed countries. Our main goal in this section is to expand on Preston and Stokes' work by estimating the historic sources of population aging between 1970 and 2015 and looking forward to 2050 by country-income groups and regions of the world.
Data and Methods
We begin by defining population aging as the change in the population share above the age of 65. Given this definition, four distinct factors drive population aging. First, mortality reductions can result in a rising elder share by allowing a greater fraction of individuals to survive to older ages. Second, fertility reductions can increase the share of older people by reducing the percentage of younger people in the population. Third, the aging of cohorts of individuals into older ages can affect the population distribution if the cohorts entering the 65+ age group are large relative to the rest of the population. While aging cohorts result from past changes in fertility and mortality, quantifying the impact of aging cohorts is relevant for policy makers because cohort influences are thought to exert a strong effect on later-life health and well-being (Finch and Crimmins, 2004). Separate consideration of cohort effects is also useful because they can lead to population aging even if current fertility and mortality are constant. Finally, migration can negatively or positively influence population aging, depending on the age composition of migrants. In this paper we consider only the first three sources of population aging, ignoring the contribution of migration (primarily because data on age-specific migration rates are very difficult to obtain). While migration is likely an important factor in population aging for some countries, we believe that ignoring migration is unlikely to introduce large errors into our estimates when looking at higher levels of aggregation such as regions and country-income groups.
For our analysis, we focus on the historical period between 1970 and 2015 and the future period between 2015 and 2050. We decompose the change in the population share above the age of 65 for both periods using the line-integral decomposition method developed by Horiuchi et al. (2008). We briefly describe the approach here and refer readers to the full paper for more details. We begin by presenting an expression for the share of the population above age 65 as a function of age-specific population counts, age-specific mortality, and age-specific fertility rates. To do this, we express the age-specific population counts in any year as the age-specific counts in a previous year projected forward using the intervening mortality and fertility experiences. Specifically, we take advantage of the 5-year data intervals in UN WPP to express the share of the population above the age of 65 at any time t as a function of the population counts at time t−5, the mortality experience between times t−5 and t (estimated based on the 5-year life tables), and the age-specific fertility rates between times t−5 and t. Based on this specification, either changing initial population distributions (the measure of cohorts aging), changing intervening mortality rates, or changing fertility rates must drive any change in the share of the population above age 65 between two time points. Conceptually, any of these components' contribution can then be thought of as the difference between the observed growth in the elderly population and the growth that would result if the population were projected forward while holding the component to be evaluated constant. In practice, this approach would produce a residual interaction term because changes in any of the factors influences the level of the other factors; however, the line-integral method produces estimates without interactions by using numerical integration and assuming a proportionate change in each component over time.
Projected population aging in the future period depends on forecasted trajectories of fertility and mortality. We use several UN WPP projection variants to quantify the uncertainty in our decompositions, with the medium variant serving as a point estimate and the high and low variants as bounds. An important point is that the UN WPP variants only differ in their projections of future fertility and the resulting changes in population counts. Therefore, we do not test the sensitivity of our results to different assumptions about the magnitude of future mortality decline.
Results
Panel A of Table 11-1 presents the change in the share of adults over the age of 65 between 1970 and 2015 by World Bank country-income groups. We then decompose this change into the contributions of aging cohorts, fertility changes over the period, and mortality changes over the period. Between 1970 and 2015, the magnitude of population aging varied substantially by income level. For example, the share of adults over the age of 65 grew by 7.3 percent in HICs but only by 1.5 percent in lower-middle-income countries and remained virtually unchanged in low-income countries (LICs). For three of the four income groups, the aging of large cohorts was the largest contributor to population aging. However, a strong income gradient remains in the magnitude of this contribution: the aging of large cohorts was most important in HICs (76.6%) and decreased across income groups (75.2% in upper-middle-income countries, 59.6% in lower-middle-income countries, and 21.8% in LICs). Conversely, fertility reductions are most important for LICs (27.6%) and least important for HICs (4.6%). The role of recent mortality declines is fairly consistent across income groups, explaining around 12–19 percent of population aging for the top three income groups. Overall, these findings highlight the substantial influence that large aging cohorts have on population aging. While past histories of fertility and mortality decline determine the size of these cohorts, recent mortality and fertility changes remain important contributors to population aging, especially in LICs.
TABLE 11-1
Decomposition of Population Aging by Country-Income Group and Region, 1970–2015.
Panel B of Table 11-1 presents the same set of results, this time for regions rather than income groups. As with income groups, the magnitude of historical population aging differed substantially across regions, with the largest absolute growth in Europe (7.2%) followed by Northern America (5.1%). Africa had the least absolute aging (0.3%), which is perhaps unsurprising because most LICs are within the African continent. In contrast to income groups, the sources of population aging by region differ less strikingly across groups, potentially due to large within-region heterogeneity. Aging cohorts were responsible for 70–80 percent of population aging for all regions except Africa. Fertility declines had the largest influence in Asia and Latin America, explaining about 15 percent of population aging, while mortality improvements were most important in Northern America and Oceania (20.5% for Northern America and 23.1% for Oceania).
To understand sources of future population aging, we conduct the same decomposition exercise for projected population aging between 2015 and 2050 (Table 11-2). The expected future change in the share of individuals above age 65 depends on assumptions about the future trajectory of fertility and mortality. Therefore, each cell in Table 11-2 presents three estimates: the point estimate from the medium-variant projections and upper and lower bounds from the high and low variants. Compared with the historical changes in Table 11-1, the patterns of population aging by income group differ substantially looking forward to 2050 (Panel A). While HICs are expected to continue aging (9.8%), upper-middle-income countries are expected to undergo the largest absolute growth in the share of older people (13.4%). Additionally, lower-middle-income countries are expected to see much larger absolute growth in the share of older people (6.2%) than they did in the previous period. The sources of population aging are expected to be far more homogenous over the coming decades. For all but LICs, the continued aging of large cohorts is the dominant source of population aging, contributing more than 90 percent. Fertility reductions have a negligible expected contribution in all but LICs, and mortality reductions are only expected to contribute 5–9 percent.
TABLE 11-2
Decomposition of Population Aging by Country-Income Group and Region, 2015–2050.
In Panel B of Table 11-2, we decompose expected future aging by region. The first striking finding is that every region is expected to see greater absolute growth in the share of older individuals between 2015 and 2050, compared with the historical change between 1970 and 2015. Latin America is expected to have the largest absolute growth in older individuals (11.6%) followed closely by Europe and Asia (10.3% and 10.1% respectively). Importantly, even Africa, which underwent virtually no aging between 1970 and 2015, is expected to see 2.5 percent growth by 2050. The aging of large cohorts is, once again, by far the largest contributor to future population aging, accounting for more than 90 percent of aging in every region except Africa, where it still accounts for 83 percent. Mortality reductions are expected to have a much smaller influence, explaining only 4–10 percent of growth in the share of older individuals across regions. Finally, fertility reductions have an essentially negligible effect in every region except Africa (8.9%). While these decompositions are based on assumptions of future fertility change, looking at the high and low variants barely changes our conclusions.
Conclusions
The levels of, changes in, and sources of population aging vary across countries at various income levels and in different global regions. In 1970–2015, richer countries experienced more population aging. The aging of large cohorts, followed by mortality reductions in HICs and fertility reductions in LICs, drove most aging. Looking to 2050, middle-income countries (MICs) and countries in Asia and Latin America are expected to experience the greatest absolute growth in the share of the population above age 65. The continued aging of large cohorts is expected to primarily drive this growth, with mortality declines also playing a small role. Fertility declines are expected to make virtually no contribution to population aging in most settings. Africa and LICs are the primary exceptions to this pattern, as fertility declines are still expected to contribute significantly to future population aging. These findings highlight the long reach of historical trends; for most country-income groups and regions, the aging of large cohorts dwarfs the contribution of recent changes in mortality and fertility.
GROWING OLD BEFORE RICH
Rapid expected population aging in LMICs has spurred concern among academics and policy makers that poor countries may be “growing old before rich.” This concern extends to the general public as well, with many news media sources discussing the potential consequences of growing old before rich in large countries such as India and China (Curran, 2017; Gray, 2017). The fear is that LMICs do not yet have the resources or the national systems to meet the financial needs of large, post-employment populations of older people. If this is true, population aging will result in a growing share of older individuals living with poor welfare support. This will in turn strain individuals and families who must provide for older people. This contrasts with the historical experience of HICs, where population aging is generally believed to have occurred more gradually and at higher levels of per capita income. As a result, HICs were able to create national institutions, such as social security and pension programs, to capture a portion of these higher incomes and transfer them to meet the needs of older individuals.
Although this belief is widely held, whether LMICs are indeed growing old before rich has not been empirically examined, as far as we are aware. In this section, we conduct a simple exercise to determine how much support exists for this view. Using historical and projected information on population aging, gross domestic product (GDP) per capita, and national health expenditure per capita, we assess whether aging in LMICs will actually occur at lower overall levels of per capita income and health expenditure relative to the historical experience of HICs.
Data and Methods
For this exercise, we use a chronological measure of population aging, the share of the population above the age of 65 (drawn from the UN WPP). We focus on two measures of national income, both drawn from the World Bank's World Development Indicators Database (WB WDI; see World Bank, 2017). The first indicator is GDP per capita, normalized across countries and time using purchasing power parity adjustments such that all values are expressed in 2017 U.S. dollars (USD). While GDP per capita is an appealing measure of overall national income, it is a rather imprecise indicator of national investments in health. In addition to social security spending, health expenditures are a key input for maintaining the welfare of older individuals. Therefore, we also use data on health expenditures per capita, normalized to 2011 USD (data were not available in 2017 values).
To determine whether LMICs are growing old before rich, we first group countries into three income categories (high-, middle-, and low-income countries) and then plot the relationship between the share of the population above the age of 65 and the wealth indicators for each year from 1990 to 2015. If LMICs are indeed growing old before rich, at any given share of older individuals, we should observe lower levels of wealth in LMICs compared with HICs. However, because LMICs have yet to reach levels of aging comparable to the recent historical experience of HICs, we cannot directly compare across income groups. We must therefore infer what GDP per capita will be in LMICs when they reach levels of population aging comparable to HICs. To do this, we project GDP per capita for LMICs into the future using the prior 10-year average growth rate. In alternative analyses, we projected GDP using growth rates over other time horizons and found no qualitative change to our conclusions.
Results
Figure 11-1 plots GDP per capita (in 2017 USD) against the share of the population above 65 years of age for LICs, MICs, and HICs for the period 1990 to 2040. The solid points represent observed data points, while the crosses represent projected data points based on historic GDP per capita growth rates and expected future shares of older individuals. Because LICs are not expected to reach levels of population aging comparable to MICs and HICs by 2040, the main comparison in the figure is between MICs and HICs. Our findings do not support the view that MICs are growing old before rich when compared with HICs' historical experience. To the contrary, when MICs reach shares of older individuals observed historically in HICs, they are expected to be at higher levels of GDP per capita than observed in HICs. In fact, a 2.9 percent annual growth rate—less than half the annual average growth rate for MICs in recent years—would be sufficient to match the historical income-to-aging ratio of HICs by the time the share of older adults in MICs reaches the HIC 1990 level. These results are robust to using health expenditure per capita, rather than GDP, in conducting the analyses.

FIGURE 11-1
Share of population above age 65 and GDP per capita in 2017 USD (purchasing power parity adjusted), 1990–2040. NOTES: Projected points are calculated based on the average GDP per capita growth rate over the past 10 years of observed data and the (more...)
Conclusions
Despite the widespread concern that LMICs are growing old before rich, we do not find evidence to support this view. Our results suggest that MICs will actually have higher levels of both GDP per capita and health expenditures per capita when they reach shares of older individuals comparable to the recent experiences of HICs. However, this conclusion is only valid under the assumption that MICs will continue to experience GDP and health expenditure growth at levels suggested by the 10-year historical growth rate. If the growth rate deviates from its historical average, MICs may well reach greater shares of older individuals at lower levels of wealth.
Beyond having sufficient levels of national wealth, MICs' ability to meet the needs of a growing older-age population also depends on having institutions to transfer this wealth to older individuals efficiently. While most MICs nominally have social security and old-age protection schemes (Social Security Administration, 2016, 2017a, 2017b), more research is needed to determine if these programs are sufficiently funded, operate efficiently, and provide coverage to the complete population of older individuals.
FUNCTIONAL AGE AND AGING
The previous analyses in this paper treated age as a purely chronological phenomenon, classifying individuals as old when they reach age 65 and defining population aging as the share of individuals who meet this threshold. Based on these conventional measures, HICs currently have the highest share of older individuals, but MICs are expected to age the most rapidly over the coming decades. The importance of chronological age stems partly from the fact that ability to function independently in society is strongly tied to a person's age (Katz, 1983; Covinsky et al., 2003). However, at any given chronological age, functional ability likely varies significantly both within and across populations. Therefore, chronological measures of aging may mask important heterogeneity in actual functional ability at both individual and population levels.
At the individual level, age is often used as one of the most important proxies for a person's needs. Age is used to determine eligibility for pensions and government benefits such as social security and Medicare (Social Security Administration, 2016, 2017a, 2017b) and to evaluate health and mortality risks (Lloyd-Jones et al., 2004). Both uses of chronological age are not based on some inherent characteristic of age but rather on tight correlations between age and health, ability to work and be independent, and general functional ability. However, if functional ability varies across and within populations at any given chronological age, then these approaches may mischaracterize individual needs and risks. Two individuals at similar ages may have different functional abilities and consequently require different resources. This issue of comparability may be further heightened when comparing individuals of similar ages across countries.
At the population level, chronological measures of age may also understate or overstate the consequences of population aging. This is reflected in popular statements such as “age is just a number” and “60 is the new 50,” which seem to imply that functional abilities may be improving over generations. If so, conventional aging measures may overstate the consequences of population aging because more-recent generations of older individuals are expected to have better health and require fewer resources in adulthood (Lutz et al. [2008] and Sanderson and Scherbov [2010] reached this conclusion for HICs). Conversely, if functional abilities are worsening across generations, conventional measures of aging would understate the likely consequences of population aging. This discrepancy between chronological age and functional ability can also affect cross-sectional interpretations of age composition across countries. For example, if two countries have similar shares of the population above age 65 but highly disparate levels of disability, chronological measures would equate the two populations when in reality one has a much larger dependent subpopulation.
The potential discrepancies between functional and chronological age have led to several studies that have sought to reconceptualize and develop alternative measures of aging. For example, Sanderson and Scherbov (2005) developed an alternative measure of aging based on remaining life expectancy. The key insight behind this measure is that an individual's health needs and functional status tend to be closely related to their remaining life expectancy, regardless of their age or their population group's total life expectancy (Riffe et al., 2015). Using this type of indicator, Gietel-Basten et al. (2016) compared traditional old-age dependency ratios to ratios based on remaining life expectancy for several world regions. They ultimately concluded that chronological-based measures substantially overstate the future magnitude of population aging over the coming decades when compared with measures based on remaining life expectancy. While remaining life expectancy–based measures are instructive, they are limited in value because they do not use direct measurements of functional aging such as disability or morbidity status. As an alternative, Sanderson and Scherbov (2017) proposed a new measure, known as “alpha-age,” that expresses an individual's age based on the value for that individual of an aging-related indicator relative to a standard age pattern. Because alpha-ages can be estimated for different indicators of aging, this approach allows for a more comprehensive comparison, in an interpretable metric, of aging across multiple dimensions.
In this section, we adapt Sanderson and Scherbov's alpha-age methodology using microdata (the HRS sister studies) from five LMICs and three HICs to compare functional and chronological aging across and within countries over time. We seek to address two broad questions: (1) for any given chronological age, how much variation exists in functional age across countries; and (2) for a given chronological age, is functional age within countries improving over time (e.g., is 60 really the new 50, or is it the new 70)?
Data and Methods
To address our main questions, we must first generate comparable estimates of functional ability across countries. To do this, we take advantage of the HRS sister studies: a group of global aging studies with harmonized data collection procedures and comparable survey questions.4 Specifically, we use data from the following countries (and datasets): China (China Health and Retirement Longitudinal Survey), Costa Rica (Costa Rican Longevity and Healthy Aging Study), Indonesia (Indonesian Family Life Survey [IFLS]), Mexico (Mexican Health and Aging Study), South Africa (Health and Aging in Africa: A Longitudinal Study of an INDEPTH Community in South Africa), Netherlands (Survey of Health, Ageing and Retirement in Europe [SHARE]), Spain (SHARE), and the United States (HRS). For our main analyses, we use data from recent waves of each survey, focusing specifically on adults over the age of 60. Table 11-3 lists more details on the survey waves and sample sizes.
TABLE 11-3
Data Sources, Waves, and Sample Sizes for HRS Sister Studies.
Our primary measure of functional limitation is whether individuals report an activity of daily living (ADL) limitation. ADLs are an extremely common metric of physical functioning (Katz, 1983), measuring individuals' ability to perform essential self-care tasks such as bathing, dressing, and eating independently. ADL limitations are appealing as a measure of functional age because they capture individuals' ability to function independently. In each survey, individuals were asked questions about their ability to perform several different ADLs without difficulty, with difficulty, or not at all (slight variations occurred in the way the question was asked across datasets, but all followed this same structure). To ensure comparability across surveys, we use data on the subset of ADL questions included in every survey (dressing, eating, bathing, getting out of bed, and walking across a room). Individuals are classified as ADL-limited if they reported difficulty or inability to perform any of the five ADLs.
We estimated functional age in each country by comparing the age- and sex-specific prevalence of ADL disability in the country to the age at which that specific prevalence is reached on a “frontier” disability age pattern. For this exercise, the frontier population represents the currently best attainable age pattern of ADL limitations. To construct the frontier age pattern (separately by sex), we first pooled data for 15 SHARE countries and estimated the lowest age- and sex-specific prevalence of disability at every age. We then constructed the frontier age pattern by combining the lowest observed age-specific prevalence of ADL disability across age groups. Because the frontier is made by combining information from multiple countries, the resulting age pattern may be irregular across age. To account for this possibility, we smoothed the observed age pattern with a logistic regression to ensure that prevalence of disability increases monotonically with age.
We then estimated a functional age for each chronological age (in 5-year age groups between 60 and 85) as the age in the frontier population with the same prevalence of disability. For example, if the prevalence of disability reaches 40 percent at age 65 in Indonesia but only does so at age 75 in the frontier population, we would assign Indonesians with a chronological age of 65 a functional age of 75. Finally, to answer our first main question of how much variation exists in functional age across countries, we plot the relationship between chronological and functional age for each country separately by sex.
Our second main question is whether functional age, at any given chronological age, is getting better or worse over time. To answer this question, we would ideally compare functional age across several birth cohorts. Unfortunately, the HRS sister studies are relatively recent, and few have collected data long enough to permit comparisons across multiple birth cohorts. However, Mexico and Indonesia have data separated by roughly a decade (between 2001 and 2012 in Mexico and between 2000 and 2014 in Indonesia). For these two countries, we attempted to identify the degree to which morbidity is expanding or compressing across generations by comparing the difference in functional ability for two periods roughly a decade apart.
While this approach is instructive for comparing cross-country differences in functional ability, it does not account for differential old-age mortality across countries. Because the salience of disability is often thought to arise from how long individuals spend disabled, comparisons of pure age patterns may overstate or understate differences in the average time that older individuals live with a disability. One approach to compare expected years lived with disability across countries is to combine the age- and sex-specific prevalence of disability across countries with the UN WPP life tables using the Sullivan method. This approach estimates disabled and disability-free life expectancy under the strong assumption that the prevalence of disability is stable over time. Despite the strength of this assumption, the Sullivan method provides a useful back-of-the-envelope approach to compare the duration of time spent disabled across countries. Using the Sullivan method, we compared cross-country differences in two measures of disability duration: the expected number of years an individual will spend disabled at age 60 and the fraction of remaining life expectancy at age 60 spent disabled.
A major limitation of ADL measures is that they are self-reported. If individuals perceive and answer questions about limitations in a systematically different way, observed differences within and across countries may not represent objective differences in disability status. Anchoring vignettes are commonly used to correct for reporting heterogeneity (King and Wand, 2006). However, vignettes are often only requested for general health status and not for specific domains of health such as functional limitations. ADL-specific vignettes (e.g., asking respondents if they consider an individual who needs to pause three times while walking 100 meters as having trouble with that activity) could greatly help ensure consistent measurement and comparability across responses. In the absence of these data, ADLs can be compared with other measurements of health to better understand whether observed differences in ADLs represent true health differences. If, for example, South African men report very low levels of ADL limitations compared with other countries but are also more obese than individuals in other countries, we might believe that South African men are underreporting ADL limitations. This approach does not solve the issue of reporting heterogeneity, but rather provides a heuristic to qualitatively assess how much confidence one should place in the observed patterns. We adopted this approach and compared the cross-country correlation between measured age-specific body mass index (BMI), the prevalence of poor self-rated health, and the prevalence of ADL limitations. A high correlation between the prevalence of disability and other health indicators may provide some indication that the disability measure is partially capturing an objective measure of health status. A low correlation between the two measures may indicate that reporting differences are driving differences across ages and countries or that the processes that cause disability in these countries are not necessarily related to BMI or poor self-rated health.
Results
Figure 11-2 shows the cross-sectional age-specific prevalence of any ADL disability by country and separately by sex. For all countries, we observe an increasing prevalence of ADL disability with age, with higher levels among women than among men. Beyond these two broad patterns, the initial levels and age gradients of disability vary substantially across countries and by sex. For both men and women, Mexican adults have the highest age-specific prevalence of ADL disability at nearly every age, with a disability prevalence at age 85 of more than 40 percent for men and more than 50 percent for women. Chinese adults follow closely, with similarly high levels of disability, especially at older ages. In contrast, Dutch adults report some of the lowest levels of ADL disability at every age. The differences between these extremes are striking: at age 85, the prevalence of disability in the Netherlands is around 20 percent for men and 35 percent for women—nearly 20 percentage points lower than same-aged Mexican adults. Surprisingly, we do not observe a clear country-income gradient in the prevalence of ADL disability. At any given age, substantial heterogeneity exists across countries, with richer countries such as Spain and the United States often underperforming poorer countries such as Indonesia and South Africa.
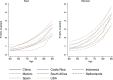
FIGURE 11-2
Age-specific prevalence of any ADL disability across countries separately by sex. NOTES: Solid lines represent low- and middle-income countries and dashed lines represent high-income countries. SOURCE: Data are from the HRS sister studies.
Though Figure 11-2 reveals differences in disability across age, sex, and country, interpreting the magnitude of these differences is not straightforward. One way to conceptualize the magnitude of these differences is by converting age-specific levels of disability to a functional age by benchmarking each country against a “best-attainable” frontier population. Figure 11-3 compares chronological and functional age for each country separately by sex. These results reveal large differences between chronological and functional age for many countries. The consistent ADL disadvantage we observe for Mexican adults in Figure 11-2 now translates into about a 15-year difference between functional and chronological age for men and a startling 15- to 20-year difference for women. These results imply that older Mexican adults have the functional capacity of much older individuals in the frontier population. We observe similarly large discrepancies for Chinese adults. At the other end of the spectrum, we find much smaller differences between chronological and functional age for individuals in the Netherlands. Overall, these results reveal pronounced and consistent variation in functional age at any given chronological age, with differences between the best- and worst-performing countries ranging from 10 to 20 years across chronological ages.

FIGURE 11-3
Functional and chronological age across countries separately by sex. NOTES: Functional age is estimated by benchmarking disability levels to a frontier population. Solid lines represent low- and middle-income countries and dashed lines represent high-income (more...)
Our results so far have focused on the cross-sectional differences in functional age across populations, yet the degree to which these findings can translate to conclusions about functional aging at the population level depends on how functional ability is changing over time. Figures 11-4 and 11-5 compare functional ability in Mexico and Indonesia for two time periods roughly a decade apart. We observe a striking increase in the prevalence of disability at nearly every age between 2001 and 2012 in Mexico (Figure 11-4), with a larger absolute increase for men than for women. Figure 11-5 plots a similar comparison of disability prevalence over time in Indonesia. Because the 2000 wave of the IFLS did not ask the full set of ADL questions, we constructed an alternative measure of physical disability based on the set of common indicators between 2000 and 2014. These results for Indonesia show a pattern similar to those for Mexico: at many ages, levels of disability are higher in 2014 than in 2000. These findings bring into question the stability of disability over time in Mexico and Indonesia and suggest a substantial expansion of morbidity, even as life expectancy continues to increase in both countries (United Nations Department of Economic and Social Affairs, 2017).

FIGURE 11-4
Change over time in ADL disability separately by sex, Mexico, 2001–2012. NOTES: Shaded regions represent 95 percent confidence intervals. SOURCE: Data are from the Mexican Health and Aging Study.
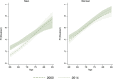
FIGURE 11-5
Change over time in disability separately by sex, Indonesia, 2000–2014. NOTES: Disability measure used is based on a set of comparable physical disability indicators from the two waves of data. Shaded regions represent 95 percent confidence intervals. (more...)
Finally, we investigated differences in the duration of time spent disabled across countries. Tables 11-4 and 11-5 present the Sullivan method estimates of total, disability-free, and disabled life expectancy for men and women. After accounting for differences in old-age mortality, our conclusions about the relative levels of functional ability across countries remain mostly unchanged. For example, Mexican adults have the highest levels of disability duration, both in absolute numbers of years lived with disability and as a share of overall life expectancy. Our conclusions about the best-performing countries are also consistent across the two methods: we find low levels of years lived with disability and shares of total life expectancy spent disabled for adults in the Netherlands, South Africa, and Indonesia. Beyond the differences across countries, these findings highlight the large sex differences in disability. In nearly every country, women live longer with disability and spend more of their lives disabled relative to men.
One interesting finding that emerges from this analysis is that countries with the highest absolute levels of years lived with disability also tend to have the largest shares of overall life expectancy spent disabled. If we assume that countries with lower life expectancies will ultimately resemble the higher life-expectancy countries, our results suggest an almost systematic expansion of morbidity as life expectancy increases. An important caveat is that these analyses are based on cross-sectional estimates of disability; in the presence of morbidity expansion and increasing life expectancy, all the estimates presented here should serve as lower bounds of the true years lived with disability.
TABLE 11-4
Years of Total, Disabled, and Disability-Free Life Expectancy for Men at Age 60 (e60) across Countries.
TABLE 11-5
Years of Total, Disabled, and Disability-Free Life Expectancy for Women at Age 60 (e60) across Countries.
Estimates based on self-reported measures of disability may not represent real differences in health across countries if individuals in some areas systematically interpret and answer disability questions differently. To determine whether our measures of self-reported ADL disability are accurately capturing variations in health, we plotted the relationship between the age-specific prevalence of ADL disability and two measures of health (BMI and poor self-rated health) separately for men and women. We find mixed results for BMI, with virtually no relationship for men and a potentially negative correlation between age-specific mean BMI and age-specific disability prevalence for women. By contrast, the correlations between self-reported ADL disability and poor self-rated health are more consistent, with a weak but positive relationship for both men and women. These results do not have a straightforward interpretation but suggest that either individual perceptions of health status do not accurately reflect objective health or the processes that result in poor self-rated health and ADL disabilities are not closely related to those that determine BMI. Testing the correlation of ADL disabilities to other objective biomarker measurements such as blood pressure, cholesterol, and spirometry values could further clarify this relationship.
Conclusions
The evidence suggests that focusing on chronological age masks substantial heterogeneity in physical functioning across countries and over time. Differences in the age-specific prevalence of disability at any given chronological age are stark and do not attenuate at older ages. In particular, Mexican and Chinese adults have much higher levels of disability compared with adults in other countries, a difference that translates to a 10- to 20-year gap in functional age relative to the best-performing countries. These differences remain salient even after accounting for differential old-age mortality across countries. We also do not observe a country-income gradient for functional age, with richer countries such as the United States and Spain performing much worse than LMICs such as South Africa and Indonesia.
Although long-term data were only available for two of the LMICs we considered (Mexico and Indonesia), evidence from both countries suggests that old-age morbidity is getting worse over time. For example, at nearly every age, the prevalence of disability is higher among Mexicans in 2012 than in 2001 (other studies have found similar findings for Mexico; see, e.g., Díaz-Venegas et al., 2015). Understanding why disability is expanding in countries like Mexico and Indonesia should be a first-order priority for future research and policy on aging.
CONCLUSIONS
We find four major stylized facts on the demography of aging in LMICs. First, MICs and countries in Asia and Latin America are expected to experience the largest absolute levels of population aging over the coming decades, driven primarily by the aging of large cohorts and secondarily by continued mortality reductions. The large magnitude of expected population aging in these countries has generated a concern that MICs and countries in Asia and Latin America are growing old before rich. However, we do not find any support for this belief; indeed, our second major finding is that as MICs undergo population aging, they may actually have higher levels of national wealth compared with the historical experience of HICs. Third, large cross-national differences exist in functional ability, with countries such as Mexico and China having a functional age that is consistently 10 to 20 years higher than best-performing countries such as the Netherlands at any given chronological age. Fourth, some evidence suggests that morbidity may be expanding over time in LMICs, with poorer physical functioning in old age among more recent cohorts. These last two findings suggest that chronological measures of aging may mask heterogeneity in functional ability both across countries and within countries over time.
Our findings raise several important future questions for the global demography of aging. In the following section, we conclude by discussing three major areas of future research and some potential short- and long-run policy solutions to promote healthy aging globally.
The Role of Public Policy
Establishing the cost-effectiveness and return on investment of interventions to promote functional independence among older individuals will be essential for crafting public policy over the coming decades. However, in the short run, simple environmental and technological changes do have the potential to provide large welfare improvements for older individuals. These types of changes provide more immediate benefits than interventions to prevent the underlying causes of disability in old age, which may take decades to realize. For example, studies have found that hearing loss is associated with poorer quality of life across multiple measures of well-being (Dalton et al., 2003). Therefore, interventions as simple as providing hearing aids may greatly improve the welfare of older individuals (Chisolm et al., 2007). Similarly, redesigning bedrooms and bathrooms to ease everyday self-care might drastically improve older individuals' ability to be independent (Petersson et al., 2008; Fänge and Iwarsson, 2005). Identifying other simple changes that enable greater independence among older individuals and reduce the burden of caregiving on families will be an important area of future work. Research must also examine the extent to which public policy solutions drawn from HICs will work in LMICs. For example, solutions such as nursing homes or assisted living facilities may see poor usage in countries in which older individuals traditionally live with their children.
Improving the quality of medical care may also provide a cost-effective way of improving functional ability among individuals with noncommunicable diseases (NCDs) such as hypertension or diabetes. Studies from multiple LMICs have found a large burden of disability and mortality due to cardiovascular events such as stroke and diabetes (Kalkonde et al., 2015; Andrade, 2009; Maredza et al., 2015; Tyrovolas et al., 2015; Ferri et al., 2011). Medical and health systems improvements targeting post-stroke care or managing diabetes complications may be effective at reducing these NCDs' disabling consequences. While such potential improvements are promising, many challenges remain in improving the quality of NCD care in LMICs. Future research will have to bring together experts from several fields to address barriers at the health system, physician, and patient levels.
Biology and Epidemiology of Aging: Preventing Functional Dependency
In the long run, preventing physical and economic dependency among future generations in MICs may be more cost-effective than the solutions discussed in the previous section. Addressing this goal will require improving our understanding of the causes of premature morbidity and mortality and identifying whether they are fundamentally different in HICs and LMICs. Related analyses will require a deeper integration of studies of the basic biology of aging with public health, demography, and economics research. Within the field of biology, a large and active community of researchers is already working to identify the biological mechanisms that cause old-age morbidity and mortality. The goal of this work is to promote longevity with the absence of morbidity, or what biologists refer to as “health span.” Using animal models and experimental methods, biologists have identified several potential physiological mechanisms that regulate the pace of aging (López-Otín et al., 2013). Drawing from these insights, we believe an exciting area of future research for population scientists will be to determine which biological mechanisms are important for explaining variation in functional ability at the population level.
Research integrating biological insights into population science has become more feasible due to efforts to collect biomarkers in large-scale population surveys, such as the HRS sister studies (Rehkopf et al., 2016). However, because biomarker data have only recently started to be collected in population surveys, current research is limited to short follow-up windows between biomarker measurement and future health outcomes. Comprehension of the most salient causes of premature morbidity and mortality in older age will be strengthened by studying the role of biological conditions earlier in life and dynamic changes over the life course. This later goal will require not only richer measurements and longer follow-up periods but also the adoption and development of new methods to properly deal with dynamic treatment effects, treatment confounder feedback, and other issues that arise with dynamic measurements. Although a rich literature exists in epidemiology and biostatistics to specifically address these methodological challenges, these methods have been slow to move into the social sciences (Robins, 1986; Hernan and Robins, 2018). Better collaborations among researchers across fields will be essential for pushing research on aging and the life course forward.
Economic Consequences of Healthy Aging
Estimating the consequences of healthy aging on various dimensions of economic well-being is important for setting public policy agendas and valuing the full benefits of health interventions. Current valuations are based primarily on data from HICs, yet substantial reasons exist to expect that the value and consequences of health in old age differ in LMICs. Based on time-use data, older individuals in LMICs are on average more engaged in productive activities compared with their counterparts in HICs, spending more time working, contributing to housework, and caring for children (Charmes, 2015). Thus, interventions that promote healthy aging may have greater value in LMICs than patterns of time use in HICs would suggest.
Second, healthy aging may also have indirect intergenerational effects on other household members. Because older individuals in LMICs are far more likely to reside with their children (Bongaarts and Zimmer, 2002), the burden of caring for older individuals may fall on other members of the household, particularly women. Therefore, promoting healthy aging may reduce the burden of care on other household members, enabling them to engage in other productivity activities. Understanding how improvements to older-adult health affect the dynamics of time transfers and labor force participation within households in LMICs remains an important open question.
Both the direct and indirect intergenerational economic effects of healthy aging may aggregate into economic growth at the macro level. However, other household and institutional considerations will determine whether healthy aging leads to growth or is offset by greater expenditure on older individuals. At the household level, postponing morbidity and increasing life expectancy could lead to greater household income through the direct effect of older individuals being more productive and the indirect effects on other members of the household. Yet if older individuals are not engaged in productive activities, their consumption will have to be funded by a combination of household and government transfers. Therefore, healthy aging may not improve growth if the magnitude of spending needed to meet the consumption needs of a longer-lived population outweighs the increase in income being generated. Research is needed to understand how to convert improved functional capacity into productive activity and capture this wealth for future generations of older individuals. Institutional reforms that encourage savings and investment while also strengthening national pension and social security programs may be an important first step to achieving these goals (Mason and Lee, 2006; Gribble and Bremner, 2012).
Data Sources for the Future Study of Aging in LMICs
With the continued emergence of HRS sister studies across the world, the data sources for the study of aging globally are increasing, each with specific benefits and drawbacks. In this section, we briefly summarize the virtues and limitations of the most common global aging datasets. This list is not meant to be exhaustive but rather to highlight three of the most promising sources for the future study of aging globally.
HRS Sister Studies
The HRS sister studies have several important benefits, including a longitudinal study design; rich social, health, and economic information on individuals and households; and harmonization that facilitates crosscountry comparisons. The surveys' panel design is extremely well suited for studying aging, allowing researchers to track dynamic changes in aging and use individual-level variation to identify the determinants and consequences of functional changes with age. These data can be used to make cross-sectional comparisons, both within and across countries, and to identify trends and changes over time. The HRS sister surveys also provide one of the few sources of biomarker measurements in LMICs, allowing for objective measurements of health status.
Despite their many benefits, the HRS sister studies have a few important drawbacks. At present, the HRS studies in most LMICs have not been running long enough to study aging over time or across cohorts. Until more waves of data become available, current research using these data will have to rely heavily on cross sections or limited changes observable in short panels. Second, although these studies collect mortality follow-up, whether the surveys can be used to generate accurate mortality estimates remains unclear. Finally, for many of the sister studies, the sample sizes are often not large enough to power subnational or subpopulation analyses of aging.
The inclusion of several additional variables could also enhance the research value of these surveys. Collecting a broader set of biomarker measurements would allow for more robust assessment of health and functional status. For example, incorporating measurements of vitamin D might be useful for evaluating NCD risk, insofar as vitamin D deficiency is particularly prevalent among older adults and is associated with several NCDs (including cardiovascular illness, respiratory problems, cancer, and bone disease) as well as functional decline and increased likelihood of harmful falls among the elderly (Sahota, 2014). Collecting more information on factors that influence cognitive test scores, such as sleep quality and hearing and vision ability, would be useful for improving measurements of cognitive capability and changes over time. Data on indoor and outdoor particulate matter would also be useful for examining the impact of pollution on functional capacity in old age.
National Sample Surveys
Many countries have national administrative and sample survey data sources that are often used for planning and surveillance. Examples of these types of data are the National Sample Survey system in India, the National Household Sample Survey in Brazil, and the Encuesta Nacional de Salud y Nutrición (National Survey of Nutrition and Health) in Mexico. These surveys often have very large sample sizes because they are used to generate national and subnational estimates. The surveys also tend to specialize on specific domains of well-being, focusing, for example, exclusively on health or labor market conditions. Therefore, any single survey often has more detail than the comparable module in the HRS sister surveys. This combination of large sample sizes and detailed information is useful for studies of aging that examine within-country variation across subpopulations or geographic areas, potentially allowing for the identification of health systems and policy effects.
However, these surveys usually do not allow for tracking individuals over time. Furthermore, while specialized surveys have rich information on specific domains of aging, linking information across different domains for individuals is often not possible. Because the data are also not harmonized, data quality can be difficult to evaluate. Finally, most of these surveys do not record incidence of mortality, and as a result they cannot be used to study longevity. Were future surveys to gather the information necessary to link observations across domains and waves and to link mortality incidence, this would greatly expand the analyses that could be conducted with the collected data. Still, noting that these surveys are designed primarily for administrative, rather than research, purposes is important. Thus, analyses that take advantage of both the large sample sizes of the National Sample Surveys and the richer detail of the HRS sister surveys will likely yield more compelling findings.
Demographic and Health Surveys (DHS)
The DHS is one of the longest-running survey programs in LMICs and has several important benefits. The DHS is conducted in many countries with a standardized survey protocol, allowing changes to be examined over time and across countries. The DHS has also started to introduce biomarker measurements in many countries. However, the biggest limitation of the DHS for the study of aging is the age range of participants. Historically, the DHS has focused on children and reproductive-age women, with little to no data on older individuals. Mortality also has to be indirectly estimated and often cannot be linked to individual-level characteristics. Collecting information on sexual behavior, availability of contraception, fertility decisions, and household economic characteristics, all of which are largely absent from these surveys, would be helpful in elucidating the underlying drivers of contextual demographic changes. Finally, the sample sizes in many countries are quite small and individuals are not tracked over time.
Despite the substantial and increasing amount of data sources available for studying aging in LMICs, significant geographic gaps remain in our knowledge of aging. For example, many countries in South America, Africa, and Central Asia do not have HRS-type sister studies. Building and developing new surveys for these regions will be critical for developing a more comprehensive global aging knowledge base. Even in countries with rich data, much potential remains untapped to use and combine existing data sources better. Together, efforts to address these limitations could greatly assist in providing a more complete picture of aging and developing policy suitable for promoting healthy aging and general well-being.
Footnotes
- 1
David E. Bell Postdoctoral Fellow, Harvard Center for Population and Development Studies, Harvard T.H. Chan School of Public Health, Harvard University, nsudharsanan@hsph.harvard.edu.
- 2
Clarence James Gamble Professor of Economics and Demography, Department of Global Health and Population, Harvard T.H. Chan School of Public Health, Harvard University.
We would like to thank Mark Hayward, David Weir, and Rebecca Wong for their valuable feedback. We would also like to thank Malay Majmundar for his guidance, the participants of the National Academies of Sciences Workshop on the Future Directions for the Demography of Aging for their insightful comments, Daniel Cadarette and Alex Khoury for their helpful research assistance, and the Carnegie Corporation of New York for its financial support.
- 3
This statement is based on Web of Science citation reports for the terms “population aging” and “population ageing”.
- 4
See Gateway to Global Aging Data at https://g2aging
.org [February 2018].
REFERENCES
- Andrade FCD. Measuring the impact of diabetes on life expectancy and disability-free life expectancy among older adults in Mexico. Journals of Gerontology Series B: Psychological Sciences and Social Sciences. 2009;65(3):381–389. [PMC free article: PMC2853602] [PubMed: 20028950]
- Bloom D, Canning D, Sevilla J. The Demographic Dividend: A New Perspective on the Economic Consequences of Population Change. Santa Monica, CA: Rand Corporation; 2003.
- Bongaarts J. Population aging and the rising cost of public pensions. Population and Development Review. 2004;30(1):1–23.
- Bongaarts J, Zimmer Z. Living arrangements of older adults in the developing world: An analysis of Demographic and Health Survey household surveys. Journals of Gerontology Series B: Psychological Sciences and Social Sciences. 2002;57(3):S145–S157. [PubMed: 11983741]
- Charmes J. Time Use Across the World: Findings of a World Compilation of Time-Use Surveys. New York: UNDP Human Development Report Office; 2015. [April 2018]. no. 97, September. http://hdr
.undp.org/sites /default/files/charmes _hdr_2015_final.pdf. - Chisolm TH, Johnson CE, Danhauer JL, Portz LJP, Abrams HB, Lesner S, McCarthy PA, Newman CW. A systematic review of health-related quality of life and hearing aids: Final report of the American Academy of Audiology Task Force on the Health-Related Quality of Life Benefits of Amplification in Adults. Journal of the American Academy of Audiology. 2007;18(2):151–183. [PubMed: 17402301]
- Covinsky KE, Palmer RM, Fortinsky RH, Counsell SR, Stewart AL, Kresevic D, Burant CJ, Landefeld CS. Loss of independence in activities of daily living in older adults hospitalized with medical illnesses: Increased vulnerability with age. Journal of the American Geriatrics Society. 2003;51(4):451–458. [PubMed: 12657063]
- Curran E. Bloomberg News, May 5, 2017. 2017. [April 2018]. Parts of Asia will grow old before getting rich, IMF warns. https://www
.bloomberg .com/news/articles/2017-05-09 /parts-of-asia-will-grow-old-before-getting-rich-imf-warns. - Dalton DS, Cruickshanks KJ, Klein BEK, Klein R, Wiley TL, Nondahl DM. The impact of hearing loss on quality of life in older adults. Gerontologist. 2003;43(5):661–668. [PubMed: 14570962]
- Díaz-Venegas C, De La Vega S, Wong R. Transitions in activities of daily living in Mexico, 2001-2012. Salud Pública de México. 2015;57:s54–s61. [PMC free article: PMC4688039] [PubMed: 26172235]
- Easterlin RA. What will 1984 be like? Socioeconomic implications of recent twists in age structure. Demography. 1978;15(4):397–432. [PubMed: 738471]
- Fänge A, Iwarsson S. Changes in ADL dependence and aspects of usability following housing adaptation—A longitudinal perspective. American Journal of Occupational Therapy. 2005;59(3):296–304. [PubMed: 15969277]
- Ferri CP, Schoenborn C, Kalra L, Acosta D, Guerra M, Huang Y, Jacob KS, Rodriguez JJ, Salas A, Sosa AL. Prevalence of stroke and related burden among older people living in Latin America, India and China. Journal of Neurology, Neurosurgery and Psychiatry. 2011;82(10):1074–1082. [PMC free article: PMC3171978] [PubMed: 21402745] [CrossRef]
- Finch CE, Crimmins EM. Inflammatory exposure and historical changes in human life-spans. Science. 2004;305(5691):1736–1739. [PubMed: 15375259]
- Freedman VA, Spillman BC. Disability and care needs among older Americans. Milbank Quarterly. 2014;92(3):509–541. [PMC free article: PMC4221755] [PubMed: 25199898]
- Fry AM, Shay DK, Holman RC, Curns AT, Anderson LJ. Trends in hospitalizations for pneumonia among persons aged 65 years or older in the United States, 1988-2002. Journal of the American Medical Association. 2005;294(21):2712–2719. [PubMed: 16333006]
- Gietel-Basten S, Scherbov S, Sanderson W. Towards a reconceptualising of population ageing in emerging markets. Vienna Yearbook of Population Research. 2016;14:41–65. [CrossRef]
- Gray A. World Economic Forum, October 6, 2017. 2017. [April 2018]. China will grow old before it gets rich. https://www
.weforum.org /agenda/2017/10/china-will-grow-old-before-it-gets-rich. - Gribble JN, Bremner J. Achieving a demographic dividend. Population Bulletin. 2012;67(2):16.
- Hernan MA, Robins JM. Causal Inference. Boca Raton, FL: Chapman & Hall/CRC; 2018.
- Horiuchi S, Wilmoth JR, Pletcher SD. A decomposition method based on a model of continuous change. Demography. 2008;45(4):785–801. [PMC free article: PMC2832329] [PubMed: 19110897]
- Kalkonde YV, Deshmukh MD, Sahane V, Puthran J, Kakarmath S, Agavane V, Bang A. Stroke is the leading cause of death in rural Gadchiroli, India. Stroke. 2015;46(7):1764–1768. [PubMed: 25999388]
- Katz S. Assessing self-maintenance: Activities of daily living, mobility, and instrumental activities of daily living. Journal of the American Geriatrics Society. 1983;31(12):721–727. [PubMed: 6418786]
- King G, Wand J. Comparing incomparable survey responses: Evaluating and selecting anchoring vignettes. Political Analysis. 2006;15(1):46–66.
- Lee RD. Demography of Aging. Washington, DC: National Academy Press; 1994. The formal demography of population aging, transfers, and the economic life cycle. In National Research Council; pp. 8–49.
- Lin S-F, Beck AN, Finch BK, Hummer RA, Master RK. Trends in U.S. older adult disability: Exploring age, period, and cohort effects. American Journal of Public Health. 2012;102(11):2157–2163. [PMC free article: PMC3471673] [PubMed: 22994192]
- Lloyd-Jones DM, Wilson PWF, Larson MG, Beiser A, Leip EP, D'Agostino RB, Levy D. Framingham risk score and prediction of lifetime risk for coronary heart disease. American Journal of Cardiology. 2004;94(1):20–24. [PubMed: 15219502]
- López-Otín C, Blasco MA, Partridge L, Serrano M, Kroemer G. The hallmarks of aging. Cell. 2013;153(6):1194–1217. [PMC free article: PMC3836174] [PubMed: 23746838] [CrossRef]
- Lutz W, Sanderson W, Scherbov S. The coming acceleration of global population ageing. Nature. 2008;451(7179):716–719. [PubMed: 18204438] [CrossRef]
- Maredza M, Bertramm MY, Tollman SM. Disease burden of stroke in rural South Africa: An estimate of incidence, mortality and disability adjusted life years. BMC Neurology. 2015;15(1):54. [PMC free article: PMC4396076] [PubMed: 25880843] [CrossRef]
- Mason A, Lee R. Reform and support systems for the elderly in developing countries: Capturing the second demographic dividend. Genus. 2006;62(2):11–35.
- Petersson I, Liljam M, Hammel J, Kottorp A. Impact of home modification services on ability in everyday life for people ageing with disabilities. Journal of Rehabilitation Medicine. 2008;40(4):253–260. [PubMed: 18382820]
- Preston SH. Children and the elderly: Divergent paths for America's dependents. Demography. 1984;21(4):435–457. [PubMed: 6394374]
- Preston SH, Stokes A. Sources of population aging in more and less developed countries. Population and Development Review. 2012;38(2):221–236. [PMC free article: PMC3957219] [PubMed: 24653544]
- Rehkopf DH, Rosero-Bixby L, Dow WH. A cross-national comparison of 12 biomarkers finds no universal biomarkers of aging among individuals aged 60 and older. Vienna Yearbook of Population Research. 2016;14:255–277. [CrossRef]
- Riffe T, Chung PH, Spijker J, MacInnes J. Time-to-death patterns in markers of age and dependency. Vienna Yearbook of Population Research. 2015;14:229–254.
- Robins J. A new approach to causal inference in mortality studies with a sustained exposure period—Application to control of the healthy worker survivor effect. Mathematical Modelling. 1986;7(9-12):1393–1512.
- Sahota O. Understanding vitamin D deficiency. Age and Ageing. 2014;43(5):589. [PMC free article: PMC4143492] [PubMed: 25074537]
- Sanderson WC, Scherbov S. Average remaining lifetimes can increase as human populations age. Nature. 2005;435(7043):811–813. [PubMed: 15944703]
- Sanderson WC, Scherbov S. Remeasuring aging. Science. 2010;329(5997):1287–1288. [PubMed: 20829469]
- Sanderson WC, Scherbov S. A unifying framework for the study of population aging. Vienna Yearbook of Population Research. 2017;14:7–40. [CrossRef]
- Social Security Administration. Social Security Programs throughout the World: The Americas, 2015. Washington, DC: U.S. Government Printing Office; 2016.
- Social Security Administration. Social Security Programs throughout the World: Asia and the Pacific, 2016. Washington, DC: U.S. Government Printing Office; 2017a.
- Social Security Administration. Social Security Programs Throughout the World: Africa, 2017. Washington, DC: U.S. Government Printing Office; 2017b.
- Tyrovolas S, Koyanagi A, Garin N, Olaya B, Ayuso-Mateos JL, Miret M, Chatterji S, Tobiasz-Adamczyk B, Koskinen S, Leonardi M. Diabetes mellitus and its association with central obesity and disability among older adults: A global perspective. Experimental Gerontology. 2015;64:70–77. [PubMed: 25688991]
- United Nations Department of Economic and Social Affairs. World Population Prospects: The 2017 Revision. New York: United Nations; 2017.
- Van Dalen HP, Henkens K. What is on a demographer's mind? A worldwide survey. Demographic Research. 2012;26:363–408.
- World Bank. World Development Indicators, 2017. Washington, DC: World Bank; 2017.
Publication Details
Author Information and Affiliations
Authors
Nikkil Sudharsanan1 and David E. Bloom2.Copyright
Publisher
National Academies Press (US), Washington (DC)
NLM Citation
Sudharsanan N, Bloom DE. The Demography of Aging in Low- and Middle-Income Countries: Chronological versus Functional Perspectives. In: National Academies of Sciences, Engineering, and Medicine; Division of Behavioral and Social Sciences and Education; Committee on Population; Majmundar MK, Hayward MD, editors. Future Directions for the Demography of Aging: Proceedings of a Workshop. Washington (DC): National Academies Press (US); 2018 Jun 26. 11.