Included under terms of UK Non-commercial Government License.
NCBI Bookshelf. A service of the National Library of Medicine, National Institutes of Health.
Alfirevic Z, Keeney E, Dowswell T, et al. Which method is best for the induction of labour? A systematic review, network meta-analysis and cost-effectiveness analysis. Southampton (UK): NIHR Journals Library; 2016 Aug. (Health Technology Assessment, No. 20.65.)

Which method is best for the induction of labour? A systematic review, network meta-analysis and cost-effectiveness analysis.
Show detailsMethods for reviewing clinical effectiveness
Identification of studies
We worked with an Information Specialist to identify trials for inclusion in the NMA. We searched the CPCG’s Specialist Register [which incorporates pregnancy and postpartum searches of the Cochrane Central Register of Controlled Trials (CENTRAL), MEDLINE, EMBASE, the NHS Economic Evaluation Database (NHS EED), relevant journals and conference proceedings]. The search strategy was finalised as part of the early consultative stages of the project, and the final search on which this report is based was carried out at the end of March 2014. The search strategy is set out in Appendix 2. A full-text copy of every relevant trial report was obtained and assigned to a topic, depending on the intervention before adding to the database. We then screened all reports that were assigned to the induction of labour topic. Many of the trials identified by the search have already been included in published Cochrane reviews, but further searches identified more recent trials which, when eligible, have been included in the analysis.
Inclusion and exclusion criteria
Interventions
All randomised controlled trials (RCTs) of induction interventions as identified in Chapter 1 of this report were evaluated. Eligible trials compared any method of third-trimester cervical ripening or labour induction with an alternative intervention, placebo or no treatment. For prespecified treatments we also included trials that compared different means of administration (e.g. vaginal misoprostol vs. oral misoprostol) or different doses [e.g. low-dose misoprostol (< 50 µg) vs. high-dose (≥ 50 µg) misoprostol]. We included studies recruiting women with a viable fetus, but had no other restrictions relating to the indication for labour induction, language or date of publication.
Trials in which women were randomised to receive a combination of interventions were not eligible, except for a small number of prespecified combinations in common use (e.g. amniotomy with oxytocin). We made the decision to exclude lesser-used combinations as the network was already large, and such combinations are rarely used clinically and mainly reported in single trials.
We included all interventions for the induction of labour examined in trials even if such treatments are not used in the NHS. Treatments no longer used may not have been abandoned for evidence-based reasons, and their inclusion adds statistical power to the entire network.
We planned to include multiarm trials and cluster randomised trials with any necessary adjustments to account for cluster design effect (if triallists had not already carried out appropriate adjustment).
Participants
We included trials that recruited pregnant women for third-trimester induction of labour, carrying a viable fetus, with a range of obstetric characteristics, undergoing labour induction for varied reasons.
Outcomes
In consultation with the patient representative from the CPCG we defined seven key outcomes for the clinical evaluation of induction interventions. The first five outcomes are common to all CPCG reviews on induction of labour and have been set out in a generic protocol.42 Outcomes 6 and 7 were proposed by the consumer representative as of importance to women. Outcomes 8 and 9 were not prespecified; however, in consultation with the steering group we extracted data on neonatal intensive care unit (NICU) admission and Apgar score, as proxies for serious neonatal morbidity (as serious neonatal morbidity was poorly reported and inconsistently defined in trials) (Box 1).
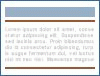
BOX 1
List of outcomes VD not achieved within 24 hours (or period specified by trial authors).
Exclusions
We excluded trials that did not report any of our key outcomes or evaluated combined interventions. The full list of references for excluded studies and the reasons for exclusion are documented in Appendices 3 and 4, Table 37.
Data extraction and risk-of-bias assessment
We obtained full-text copies of all reports identified by the search. A minimum of two investigators independently assessed all reports to determine whether or not trials used random allocation to groups, included one or more of the selected interventions and comparisons, recruited women undergoing third-trimester induction of labour, and included data on at least one of our primary outcomes. Trials meeting all of the eligibility criteria were included in the systematic review.
Data extraction was carried out by one investigator and checked by a second. Preliminary statistical analyses also highlighted some discrepancies in the extracted data, which were then doubled checked by the reviewers, and corrected if appropriate. For all included trials, we extracted data on trial and patient characteristics, and this is summarised in tables of included studies (see Appendix 5, Reference list for included studies, and Appendix 6, Table of included studies characteristics, Table 38).11,14,30,31,43–936
Study quality was assessed using the methods described in the Cochrane Handbook.937 For use in a prespecified sensitivity analysis, we assigned a judgement relating to risk of bias (low, high, unclear), based on the allocation concealment domain. We based this decision on meta-epidemiological evidence indicating the importance of this domain as a source of bias938 and on the design of obstetric trials, which often precludes blinding of participants and personnel (although not, of course, of outcome assessors).
Information on study setting (country and whether or not the study was carried out in an inpatient or outpatient setting), method and the type of intervention(s) (dose, mode of administration, type of preparation, e.g. slow-release pessary vs. gel, regimen and any cointerventions) was extracted. We extracted details on comparison arms (e.g. another active treatment, placebo or ‘usual care/no treatment’). Treatment arms were categorised according to the initial randomised allocation, although subsequent clinical management may have included further doses or an alternative treatment. For participants, we recorded important obstetric characteristics, including parity, previous CS, state of cervix and whether or not amniotic membranes were intact. These factors were a priori expected to be possible intervention effect modifiers. There was an additional concern that patient characteristics may be linked to the interventions that have been included in the studies. For example, if it were the case that all of the studies comparing NO with placebo predominantly included women with a previous CS, whereas the studies comparing misoprostol with placebo predominantly excluded women with a previous CS, then the indirect comparison of NO with misoprostol may not be a fair reflection of the true underlying effect in either subgroup of women. For NMA to be valid the different study populations are required to be ‘similar’ in any effect modifying covariate (see Network meta-analysis for a description of the key assumption of transitivity/consistency in NMA). It is therefore important to inspect tables of patient characteristics according to intervention comparison to assess whether or not there is an a priori reason to suspect that the transitivity/consistency assumption may not hold.
In summary, for each trial, information was extracted on:
- The interventions compared in trials (with details of dosage and regimen for pharmacological interventions).
- Number of participants in trials.
- Parity of women recruited to trials (all nulliparous, all multiparous or mixed parity).
- Whether women had ruptured or intact membranes at recruitment (all ruptured, all intact or the sample included women with both intact or ruptured membranes).
- Whether or not women had favourable or unfavourable cervical scores at recruitment (Bishop score all < 6, ≥ 6 or included women with either favourable or unfavourable scores).
- Whether or not trials included women with multiple pregnancies.
- Gestational age at recruitment (all post dates, all > 37 weeks, or the sample included women at < 37 weeks’ gestation).
- Treatment setting (women treated as inpatients or outpatients).
- Risk of bias (high, low or unclear risk of bias, based on allocation concealment).
- We also recorded whether or not the study had been funded or partly funded by pharmaceutical sponsors.
We compared the distribution of these characteristics in tabular form before we conducted the NMA (see Appendix 7, Table 39). Sensitivity analyses were planned to exclude studies that were assessed as being of unclear or high risk of bias.
Methods of evidence synthesis
Network meta-analysis
A NMA was conducted to simultaneously compare the induction interventions, placebo or no treatment for each outcome. In its simplest form, a NMA is the combination of direct and indirect estimates of relative intervention effect in a single analysis. An indirect estimate of the relative intervention effect B compared with C can be formed by comparing direct trials of A compared with C with trials of A compared with B, such that . A simple approach to combining the indirect and direct estimates of B compared with C would be to take a weighted average, for example using an inverse variance weighting.939 NMA extends the idea of an indirect comparison to simultaneously combine all evidence in a connected network of intervention comparisons.940 For random-effects (REs) models, we assume that the between studies variance is the same across all of the pairs of intervention comparisons (known as the homogeneous variance assumption). In a NMA we assume that intervention A is similar (in dose, administration, etc.) when it appears in the A versus B and A versus C studies, and also that every patient included in the network has an equal probability of being assigned to any of the interventions:940 a concept called ‘joint randomisability’.941 A first step to assess this assumption is by comparing the distribution of potential effect modifiers across the different942 comparisons,942,943 as if there is an imbalance in the presence of effect modifiers across the A versus B and A versus C comparisons, the conclusions about B compared with C may be in doubt. A second step is to use statistical measures of model fit to see if the direct estimate for a particular intervention comparison is discrepant with the NMA estimate944 (see below). When direct data were available, pairwise meta-analyses were also performed for all comparisons, and compared with the NMA treatment effect estimates to informally assess agreement.
All of the analyses were conducted within a Bayesian framework utilising OpenBUGS version 3.2.3 (www.openbugs.net; Medical Research Council Biostatistics Unit, Cambridge), using the NMA code given by Dias et al.945–948 for binomial data. We provide example code in Appendix 8. A key feature of a Bayesian analysis is that a joint distribution (called the ‘posterior’ distribution) of all model parameters (intervention effect estimates and heterogeneity) is estimated, and results are reported as summaries from this posterior distribution. For example, it is common to report the posterior median and 95% credible intervals (CrIs, which are interpreted upon there being a 95% probability that the parameter lies within this range of values, where 95% of the marginal distribution lies).
Studies with 0% or 100% events in all arms were excluded from the analysis because these studies provide no evidence on relative effects.946 For studies with 0% or 100% events in one arm only, we planned to analyse the data without continuity corrections when computationally possible. Where this was not possible, we used a continuity correction where we added 0.5 to both the number of events and the number of non-events, which has shown to perform well when there is an approximate 1 : 1 randomisation ratio across intervention arms.949 In Chapter 3, we report any adjustments made.
Both fixed-effects and REs (when sufficient data were available) models were considered on the basis of model fit. Goodness of fit was measured using the posterior mean of the residual deviance, which is a measure of the magnitude of the difference between the observed data and the model predictions for those data.950 Smaller values are preferred, and in a well-fitting model the posterior mean residual deviance should be close to the number of data points.950 Of course, improvements in model fit can always be achieved by making the model more and more complex, but at the risk of losing generalisability and interpretability. To account for this we report the deviance information criterion (DIC), which penalises model fit with model complexity.950 Finally, we report the between-studies standard deviation (SD) (heterogeneity parameter) to assess the degree of statistical heterogeneity. Model selection was based on all of these statistics: posterior mean residual deviance, posterior median between-study heterogeneity, and DIC. In comparing models, differences of ≥ 5 points for posterior mean residual deviance and DIC were considered meaningful,950 with lower values being favoured. Heterogeneity was reported as the posterior median between trial SD (τ) with its 95% CrI.
We planned to conduct sensitivity analyses excluding studies at high risk of bias for allocation concealment, for all analyses. Consistency between the different sources of indirect and direct evidence was explored statistically by comparing the fit of a model assuming consistency with a model that allowed for inconsistency (also known as an unrelated treatment-effect model). If the inconsistency model had the smallest posterior mean residual deviance, heterogeneity, or DIC value then this indicates potential inconsistency in the data. When model fit was suggestive of inconsistency our first step was to restrict trials to those at low risk of bias. If model fit was not improved, we planned further subgroup analyses using the potential treatment effect modifiers identified above (see Data extraction and risk-of-bias assessment).
A Bayesian analysis requires prior distributions to be specified on all model parameters that are being estimated. A prior distribution reflects our belief about the values that a parameter can take in advance of observing the data. Vague (flat) prior distributions were specified for treatment effect and heterogeneity parameters, so that our results are driven by the observed data (see Appendix 9 for full details of the prior distributions assumed). Convergence was assessed using the Brooks–Gelman–Rubin diagnostic951 and was satisfactory by 68,000 simulations for all outcomes.952 A further simulation sample of at least 58,000 iterations post convergence was obtained, on which all reported results were based.
Relative intervention effects are reported as posterior median odds ratios (ORs) and 95% CrI. All reported outcomes are negative events and so an OR < 1 is interpreted as the active intervention reducing the odds of the event. We calculated the probability of each treatment being first, second, third, etc. most effective for each outcome and report the results using ‘rankograms’. Peaks in the rankogram graph indicate the most likely rank for each intervention type. Flat lines indicate a high degree of uncertainty for the ranking of that intervention type. As this metric can be unstable and difficult to interpret (e.g. when there is a high probability of being both ‘best’ and ‘worst’ on an outcome), we also report posterior mean rank of each treatment (and 95% CrI), with the convention that the lower the rank the better the treatment. We also report the absolute probability of an event for each intervention. To estimate the absolute probability, we selected vaginal PGE2 (tablet) as the baseline intervention and conducted a fixed-effects meta-analysis on vaginal PGE2 arms to produce only an ‘average’ intervention effect to which the relative treatment effects (as estimated from the NMA) were added. Note that this is modelled externally to the NMA. We note that this may not generalise to any one setting, as it is based on all of the trials in the NMA, and refer the reader to Chapter 4, Assessment of cost-effectiveness for UK-specific absolute estimates.
Pairwise meta-analyses
For completeness, and to informally assess the consistency assumption of NMA, we conducted pairwise meta-analyses for all intervention comparisons for which direct head-to-head evidence was available. The method of estimation was identical to that described above for the NMA, except that we did not apply the consistency assumption, so that we obtained separate intervention effect estimates for each pairwise comparison. For the REs models, we assumed that the heterogeneity parameter was common across intervention comparisons, to reflect the assumption made in the NMA and allow a fair comparison of the intervention effect estimates.
- Methods for assessment of clinical effectiveness - Which method is best for the ...Methods for assessment of clinical effectiveness - Which method is best for the induction of labour? A systematic review, network meta-analysis and cost-effectiveness analysis
Your browsing activity is empty.
Activity recording is turned off.
See more...