Included under terms of UK Non-commercial Government License.
NCBI Bookshelf. A service of the National Library of Medicine, National Institutes of Health.
Tylee A, Barley EA, Walters P, et al.; on behalf of the UPBEAT-UK team. UPBEAT-UK: a programme of research into the relationship between coronary heart disease and depression in primary care patients. Southampton (UK): NIHR Journals Library; 2016 May. (Programme Grants for Applied Research, No. 4.8.)
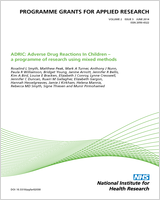
UPBEAT-UK: a programme of research into the relationship between coronary heart disease and depression in primary care patients.
Show details18 July 2013 | |
Summary: | Contains every action undertaken on the UPBEAT COHORT data set since receiving the data set |
Original data sets: | J:\Programme Grant\Rachel\UPBEAT cohort\Data |
Final data sets: | J:\Programme Grant\Rachel\UPBEAT cohort\Data\Final data sets |
Syntax: | J:\Programme Grant\Rachel\UPBEAT cohort\Data\Syntax |
Other information: | J:\Programme Grant |
Data version | Action | Syntax file |
---|---|---|
1 | Data set set up (separately for each time point) | |
Original data sets downloaded from MACRO by the Data Manager (Christopher Rowson) and emailed as a .csv file | ||
Rachel Phillips was responsible for receiving all extractions up to and including the 24-month data | ||
RP also wrote the instructions for cleaning the data sets and data checking processes | ||
Paul Williams took over for the 30-month until 48-month (final) data sets, using these instructions | ||
Original data sets as .csv files can all be found in the ‘Data’ folder under the relevant subfolders | ||
Paul Williams was also responsible for collating the data sets and merging in any additional data | ||
1.1 | Data cleaning and scale scoring | |
The same operations were undertaken for each data extraction (each time point) | ||
All data cleaning were carried out in STATA version 11.2 | ||
The instruction document (Document to outline the ordering of do files for UPBEAT data.doc) describes the cleaning process that transforms the .csv file into a cleaned STATA data set. e.g. for 30 month: | ||
30month_UPBEAT_Live20120821.csv | Extraction to STATA format | 0_insheet_30months.do |
thirty_month.dta | Full cleaning process (including the start of data cleaning) | 1_30month.do |
1.2 | Data checking | |
The instruction document (Document to outline the ordering of do files for UPBEAT data.doc) also describes the data checking process that checks for: data entry errors, correct number of participants, correct dates for the time between baseline and time point) | ||
Any data errors that were flagged when conditions were not met were noted in the document (upbeat data discrepancies v2.2.xlsx) and amendments were written into the cleaning.do file) | ||
e.g. for 30 months: | ||
30month.dta | Data errors flagged, recorded and amendments made to.do file | 1_30month.do |
A list of ID numbers was then obtained and this was checked by the researchers to see whether or not this list matches the list of participants recorded on the ACCESS database | ||
Any mis-matches flagged were recorded in the document (Access and Macro Follow ups at 30months FIXED.docx) and also (Date discrepancies CORRECTIONS.doc) | ||
Any resulting amendments were translated into STATA code and the STATA data set was changed accordingly | ||
30month.dta | Changes to be made to the participants in the data set of the time point | 30m_amendments.do |
If in the case the interview had been stored in the incorrect time point, the same.do file (30m_ammendments.do) extracts the data set, places it in the correct folder, and merges the record into the correct time point. In which case, the record would be stored as a separate data set in that folder, e.g.: | ||
30month_P14007.dta | ||
Correct dates of the interviews were checked by calculating differences between their interview date and the interview date of their baseline visit. Any participants with substantial differences (> 1 month early, > 4 months late) were investigated | ||
All reporting of date errors was recorded in the document (Date discrepancies CORRECTIONS.doc) | ||
30month.dta | Merge 30 month and baseline time point variables and create merged data set called base_30month.dta | Merging 30 months.do |
base_30month.dta | Check time difference between time points, check outliers with researchers | initial_30months.do |
30month.dta | Make amendments to data set, resulting in a cleaned final data set with the same name | 30month_correctdates.do |
A data cleaning checklist was compiled to keep track of progress for weekly meetings (Data cleaning check.xlsx) | ||
2 | data set set up (complete cohort data set) | |
The process for combining the data sets was as follows: (1) make all the data sets (of each time point) compatible; (2) create a variable to distinguish the data sets; then (3) append these data sets | ||
By appending data sets of the same observations, and mostly the same variables but different time points, we essentially create a data set in long format with many rows per participant. Matching occurs on the variables, not the unique identifiers as is normally done with merging. Appending has the advantage in this instance of creating a data set with fewer variables than what would have been produced in the wide format | ||
1. Making the data sets compatible | ||
For each data set, variable names were shorted to remove the suffix containing the time point information | ||
Any variable names that were not the same in all the time points were renamed accordingly for synchronisation | ||
PYSCHLOPS variables that contained information about previous time points were dropped | ||
Data sets used were from the folder: (J:\Programme Grant\Rachel\UPBEAT cohort\Data\Final data sets) | ||
base_medic_complete.dta | ||
6month.dta | ||
12month1.dta | ||
18month.dta | ||
24month.dta | ||
30month.dta | ||
36month.dta | ||
42month.dta | ||
48month.dta | ||
2. Create a variable to distinguish time points | ||
The variable <time point> was created, containing sequential values per data set (per time point) | ||
data sets were saved locally | ||
base.dta | ||
6.dta | ||
12.dta | ||
18.dta | ||
24.dta | ||
30.dta | ||
36.dta | ||
42.dta | ||
48.dta | ||
3. Append these data sets | ||
All data sets were appended to the master data set (baseline) simultaneously. | ||
base | append all other time points into this data set, and save as upbeat_cohort.dta | upbeat_cohort_merge.do |
2.1 | Data cleaning and scale scoring | |
The process of data cleaning on the cohort data set involved (1) dropping all empty fields, (2) renaming and ordering variables appropriately, (3) ordering the data by patid and time point, and (4) inserting information into missing fields (e.g. gender was only recorded at baseline and so this information was carried across to other time points) | ||
upbeat_cohort.dta | Data cleaning (1–4) | upbeat_cohort_clean.do |
HADS anxiety missing values (> 55) to be classed as missing on variables (hads_anx anx_cat anx) | upbeat_cohort_clean.do | |
Create ROSE classifications variable (called rose_short) | upbeat_cohort_clean.do | |
Labels for GP practices | upbeat_cohort_clean.do | |
Relabeling age variables to make explicit these were age at baseline (demographics questions collected only at baseline). The creation of a new age variable to record participant age throughout the data collection | upbeat_cohort_clean.do | |
Relabeling comorbidity variables to make explicit these were age at baseline (demographics questions collected only at baseline) | upbeat_cohort_clean.do | |
upbeat_cohort.dta | Check to see whether or not the all dates and time point make sense | upbeat_cohort_inspec.do |
2.2 | Merging additional data: (1) loss information (2) cardiac investigations information (3) depression pattern information | |
Data from sources other than MACRO were entered into the cohort data set as follows | ||
2.2.1 | (1) Loss information | |
Information regarding [(1) deaths, (2) withdrawals, and (3) loss to follow ups] on the whole sample was collected by Alison Smith and Rebecca Lawson | ||
Specific categories were decided upon during weekly UPBEAT meetings with the whole team | ||
Data was entered onto an excel spreadsheet (Cohort Main Spreadsheet – Deaths,LTFU,Withdrawal.xlsx) | ||
The codebook for reasons of drop out is reported in the document (CODES FOR COHORT.doc) | ||
Data was imported into the upbeat cohort, with the following specification:
| ||
upbeat_cohort.dta | Merge in the Loss data from (Cohort Main Spreadsheet – Deaths,LTFU,Withdrawal.xlsx) | upbeat_cohort_loss.do |
Fill in demographic data into these new records | upbeat_cohort_loss.do | |
Save data set as upbeat_cohort_v2.dta | upbeat_cohort_loss.do | |
2.2.2 | (2) Cardiac investigation information | |
Information regarding cardiac investigations were collected from the GP notes for every participant in the cohort study and were inputted into an encrypted Microsoft Access database by Dr Jorge Palacios (Upbeat_Cohort_Med_Notes_2.mdb) | ||
The specific data to be inputted and the specific definitions of cardiac investigations were decided before the hard copies were examined, with exception to the ‘Rapid Access Clinic’ category*. It was decided that data on rapid access would provide important information as to the severity of chest pain problems that a participant was experiencing (by the reasoning that if they used this service, then they must have experienced a level of severity higher than ‘ROSE Exertional pain’ but lower than the severity that required an intervention or even the severity defined by a cardiac event. This was decided upon by the UPBEAT team during weekly meetings. The GP notes were re-examined to gather this information and all data were inputted into the Access database before any analysis of cardiac investigation data occurred | ||
* It was later suggested by the UPBEAT team that this ‘Rapid Access’ classification may not provide information regarding cardiac severity in the manner it was first thought to, and suggestions were made to remove it due to the following reasons: (1) it presented a very heterogeneous group of participants who had various reasons for accessing rapid access, of which the reasons were not recorded; (2) the results from the Rapid access service were not recorded so this could not be validated. Based on these two reasons, it cannot be used to determine with adequate accuracy the severity of heart problems as the other categories; and (3) participants access to the service was disparate among locations (as defined by GP practice) leading to potentially biased results dependent on where the participant lived | ||
Access data was exported to a STATA data set file (data_cardiac_investigations.dta) | ||
A codebook for the cardiac investigations was saved as a STATA data set file (tbl_cardiac intervention type.dta) | ||
Data was imported into the upbeat cohort before any analysis, with the following specification: | ||
| ||
0 no chest pain | ||
1 chest pain | ||
2 exertional chest pain | ||
3 rapid access | ||
4 Bypass graft or angioplasty | ||
5 MI | ||
6 Cardiovascular death | ||
The classification of a participant within a time point depended on their most severe data for that time point. Cardiovascular deaths (cardiac/stroke/vascular) were chosen over cardiac only deaths due to low numbers in order to increase statistical power. This ordinal variable was duplicated for the two definitions of cardiac investigation (1) first (2) severe. *After discussions regarding the appropriateness of the ‘rapid access’ classification, the two versions (first and severe) classifications were split further to include/exclude this rapid access | ||
data_cardiac_investigations.dta | Clean data and save data set as cardiac_all_wide.dta | upbeat_cohort_cardiac outcomes |
upbeat_cohort_v2.dta | Keep only variables: (id, time point and visit date). Reshape wide. Merge in the cardiac investigations data (cardiac_all_wide.dta) | upbeat_cohort_cardiac outcomes |
Visit dates for time points 0 (baseline) – 8 (48 months) were available in wide format. An additional variable was created to mark the date exactly 6 months prior to their baseline visit. All cardiac investigations were assigned to their appropriate time point based on the window (Tn-1 to Tn) | upbeat_cohort_cardiac outcomes | |
These cardiac investigations were then used to create the appropriate variables (1) first investigation per person per time point was coded into a variable, and (2) the most severe investigation per person per time point was coded into another variable. Dates of such investigations were retained alongside each of these. All other information was dropped. This data set was saved as cardiac_long.dta | upbeat_cohort_cardiac outcomes | |
Merge in the cardiac investigations data (cardiac_long.dta) | upbeat_cohort_cardiac outcomes | |
Outcome variables created for the ordinal cardiac problems (from no chest pain – chest pain – cardiac investigations – cardiovascular death). The two versions utilise the two definitions of cardiac investigation (1) first in time point (2) most severe in time point | upbeat_cohort_cardiac outcomes | |
Cleaning of variables as new records per person were added | upbeat_cohort_cardiac outcomes | |
Save data set as upbeat_cohort_v3.dta | ||
2.2.3 | (3) Depression pattern information | |
Depression episodes as defined by the HADS depression scale (cut off 8 or more = positive) were extracted from the upbeat cohort into an Excel spreadsheet for the purpose of descriptively reporting the patterns of depression throughout the cohort up to 36 months | ||
It was decided by the team that the combination of different patterns (start, end, fluctuating) and missingness should be collapsed as succinctly as possible | ||
These coding for this was performed in Microsoft Excel in the file (depression table with analysis 2.0.xls) | ||
A version of this that contained only the information on patterns and missingness was saved as a comma delimited file to be imported into STATA (dep_pat.csv) | ||
The 3 variables chosen to describe a participant’s pattern of depression episodes were | ||
| ||
upbeat_cohort_v3.dta | Merge in the patterns data from (dep_pat.csv) | upbeat_cohort_dep_patterns |
Data cleaning | upbeat_cohort_dep_patterns | |
Save data set as upbeat_cohort_v4.dta | upbeat_cohort_dep_patterns | |
3 | Creating a wide version of the data set | |
To create a wide data set, first the long data set needed to be reduced as it contained 940 variables. A wide version of which would contain (8 × 940 variables) and it was decided appropriate to reduce this for simplicity | ||
The method for creating a wide version of the data set consisted of (1) retaining only key variables, (2) add suffix ‘_’ to the end of variable names, and (3) reshape wide | ||
A list of key variables of immediate interest was selected to be retained in the wide version of the data set (to save space). These variables along with others as requested were retained | ||
upbeat_cohort_v4.dta |
| upbeat_cohort_wide.do |
| upbeat_cohort_wide.do | |
| upbeat_cohort_wide.do | |
Save data set as upbeat_cohort_v4_wide.dta | upbeat_cohort_wide.do |
- The UPBEAT-UK study cohort audit trail - UPBEAT-UK: a programme of research into...The UPBEAT-UK study cohort audit trail - UPBEAT-UK: a programme of research into the relationship between coronary heart disease and depression in primary care patients
Your browsing activity is empty.
Activity recording is turned off.
See more...