Abstract
Complex interventions and large-scale transformations of services are necessary to meet the health-care challenges of the 21st century. However, the evaluation of these types of interventions is challenging and requires methodological development.
Innovations such as cluster randomised controlled trials, stepped-wedge designs, and non-randomised evaluations provide options to meet the needs of decision-makers. Adoption of theory and logic models can help clarify causal assumptions, and process evaluation can assist in understanding delivery in context. Issues of implementation must also be considered throughout intervention design and evaluation to ensure that results can be scaled for population benefit. Relevance requires evaluations conducted under real-world conditions, which in turn requires a pragmatic attitude to design. The increasing complexity of interventions and evaluations threatens the ability of researchers to meet the needs of decision-makers for rapid results. Improvements in efficiency are thus crucial, with electronic health records offering significant potential.
Scientific summary
Complex interventions and large-scale transformations of services are necessary to meet the health and social care challenges of the 21st century. The evaluation of complex interventions and large-scale transformations of services is challenging and continues to demand methodological innovation
Innovations in design (cluster randomised controlled trials, stepped-wedge designs and non-randomised evaluations) provide decision-makers with a variety of options to meet their evaluation needs. The complexity of modern interventions requires effective intervention development. The use of theory and logic models can make explicit how the intervention is expected to impact on process and outcome. The use of detailed process evaluation to understand delivery in context is also important. Relevance requires that evaluations are conducted under real-world conditions, which in turn requires changes in the design of randomised trials (e.g. greater use of pragmatic trials). Intervention design and process evaluation need to explicitly consider issues of implementation to ensure that trial results can be scaled to drive population benefit.
The increasing complexity of interventions and their evaluation threatens the speed and efficiency of the evaluation, and the ability to meet the needs of decision-makers for rapid results. Innovations such as the more effective use of electronic health records have significant potential to facilitate rapid trial delivery.
Introduction
Attempts to improve health outcomes or tackle public health problems increasingly make use of complex interventions. These are commonly defined as interventions that contain several interacting components.1 Additional dimensions of complexity include the number of different behaviours required by those delivering or receiving the intervention; the range of stakeholders or structures targeted (patients, professionals, systems of care); and the degree of variation when the intervention is delivered in different contexts.
Complex interventions present a number of specific problems for evaluators. Many of these relate to the difficulty of standardising such interventions; their sensitivity to features of local context; and the length and complexity of the causal chains linking the intervention with outcome.1 Complexity exists not only in the interactions of the different components of the intervention2 but also in its interaction with the wider system in which the intervention is embedded.
Although a range of experimental and non-experimental approaches to evaluation have been proposed, randomised controlled trials (RCTs) remain the optimal method for obtaining unbiased estimates of effectiveness.3 The UK Medical Research Council (MRC) has set out guidance on developing and evaluating complex interventions in trials, originally published in 2000, and revised and extended in 2008.1 Others have further extended our understanding of relevant research methods in this area.4
As the pace and scope of change in health services has increased, there is also a growing need to evaluate not just complex interventions, but also large-scale transformations of services. These have been defined as ‘interventions aimed at coordinated, system wide change affecting multiple organisations and care providers, with the goal of significant improvements in the efficiency of health care delivery, the quality of patient care, and population-level patient outcomes’.5 ‘Integrated care’ for long-term conditions represents an excellent exemplar of such a transformation, involving complex packages of care as well as more profound changes in workforce configuration, service organisation and funding. The rigorous evaluation of such change represents a new level of challenge. The issues of scale and complexity in the evaluation of services are further discussed in Essay 6 in this volume.
Evaluators also face additional pressures. Designing, delivering and understanding the operation of a complex intervention may require complex, multimethod research designs to both provide rigorous assessment and assist in the interpretation of results. Classic RCTs are traditionally long, complicated and expensive exercises and are, therefore, under significant scrutiny in an era of financial restrictions in research funding and major concerns about research waste.6 New, more pragmatic RCT designs are receiving increasing attention.
There is pressure from policy-makers and service managers for rapid evaluation to support short- and medium-term decision-making in areas where they face pressing problems of demand and supply. While methodological rigour remains critical, increasing importance is placed on the speed with which research findings are delivered and translated into practice. Developing and testing innovations to enable implementation at ‘pace and scale’ is considered crucial to ensure reach and long-lasting and replicable effects. This requires greater attention to the balance between internal and external validity, building consideration of later implementation into the design of interventions at an early stage, and may set limits around the cost, time scales and sophistication of research designs.
In this essay, we first describe recent methodological developments in relation to RCTs for the evaluation of complex interventions and large-scale transformation. We review current knowledge about available approaches, comparing and contrasting methods for different scenarios. We then go on to outline issues and practical challenges that could usefully be addressed by those who conduct, fund and regulate such research.
Randomised methods
In a conventional RCT, individuals are randomly allocated between alternatives. However, complex interventions and large-scale transformations often target levels other than the individual participant. For example, whole communities may be targeted by public health programmes; other interventions may be aimed at groups of health professionals, or organisations such as general practices.
Contamination of the control group, leading to biased estimates of effect size, is a drawback of RCTs of population-level interventions.7 This is likely to occur in situations in which different public health promotion interventions are tested within the same community, or the same clinician delivers different types of health care to patients.8
Cluster randomised controlled trials
Cluster randomised controlled trials (cRCTs) have emerged partly as a means of addressing such problems. The units randomised are pre-existing, natural or self-selected clusters. Cluster members all have an identifiable feature in common (such as patients in a single general practice), and outcomes are measured in all or in a representative sample. The clusters can vary widely depending on the setting, and range in size from families to entire communities.
Methodological discussion of the cRCT appears to have begun in the field of education in the 1940s,8 but the design saw only sparse use before the 1980s. However, the last half-century has seen a steady increase in cRCTs published in the medical literature: from one per year in the 1960s to over 120 in 2008.8 The main implication of the cRCT design is that the outcomes of individuals within the same cluster tend to be correlated, which must be accounted for in the analysis. The statistical measure of correlation is known as the intracluster correlation coefficient (ICC), defined as the proportion of variation in the outcome that can be explained by the variation between clusters. Sample size estimates need to account for this, taking into account both the size of the clusters and the ICC. Reliable estimates of ICCs are essential for robust sample size calculations. There is increased understanding of the factors that affect clustering and therefore the likely magnitude of an ICC. For example, Campbell et al.9 demonstrated that ICCs are sensitive to a number of trial-related factors, particularly the setting and the type of outcome. Analysing a range of data sets, mainly from the UK, they found that ICCs were significantly higher for process than outcome variables and for outcomes in specialist settings compared with primary care. The effects of disease prevalence and trial size were less clear-cut.9 Other studies, meanwhile, suggest that ICC is associated with prevalence for binary outcome measures.10
There is also growing understanding of how to calculate sample sizes for cRCTs, including scenarios in which the size of individual clusters varies.11 This has been incorporated into standard statistical packages, and relevant online tools are also available.12
The third development has been the publication of reporting guidelines for cRCTs. These guidelines highlight that it is important to indicate the level at which the interventions were targeted, hypotheses were generated, randomisation was done and outcomes were measured.13 In 2012, specific guidance was published, based on the 2010 version of the Consolidated Standards of Reporting Trials (CONSORT) statement and the 2008 CONSORT statement for the reporting of abstracts.14 The guidance includes a checklist of items that authors should address when reporting cRCTs, in conjunction with the main CONSORT guidelines.15
There has also been the development of an appropriate ethical framework, The Ottawa Statement on the Ethical Design and Conduct of Cluster Randomised Trials. This sets out 15 recommendations, providing guidance on the justification for a cRCT; the need for ethical review; the identification of research participants; obtaining informed consent; the assessment of benefits and harms; and the protection of vulnerable participants.16
Stepped-wedge trials
The stepped-wedge trial has emerged as an alternative to the standard parallel-group cRCT and is increasing in popularity for evaluating complex interventions and large-scale transformations.17 The design includes an initial period during which no clusters are exposed to the intervention and therefore act as controls. Subsequently, at regular intervals (the ‘steps’), one cluster (or a group of clusters) is randomised to cross over from control to intervention. This process continues until all clusters have crossed over and are exposed to the intervention (Figure 2.1). The appeal of the design is that, at the end of the study, there will be a period when all clusters are exposed, but the order of intervention delivery is determined at random. Data collection continues throughout the study, so that each cluster contributes observations during both control and intervention observation periods.19

FIGURE 2.1
Stepped-wedge trial schema. Shaded cells represent intervention periods, blank cells represent control periods and each cell represents a data collection point. Reproduced from Brown and Lilford under the terms of the Creative Commons Attribution Licence (more...)
The rationale for using this approach, as well as specific methodological considerations, has been described in more detail elsewhere.19,20 However, the principal advantage is that it may more adequately reconcile the differing needs and priorities of policy-makers and service managers on the one hand, and evaluators on the other. It has intuitive appeal, as each cluster eventually receives the intervention and acts as its own control. As a consequence, it is particularly suited to interventions in which there is a lack of evidence of effectiveness but a strong belief that they will do more good than harm,21 such as efforts to improve hand hygiene. It also promotes the phased implementation of novel interventions, permitting time for staff training, if this is required. In addition, the researcher may study the effect of time on an intervention, such as whether or not adoption or learning is maintained.
Nevertheless, because data collection usually takes place at each time point in the study, the data burden is potentially significant. It may also take time before every cluster receives the intervention. In addition, the analysis is more complex than that required for a conventional cRCT. Sample size calculations and analysis must make allowance for both the clustered design and the confounding effect of time.20 In a stepped-wedge design, more clusters are exposed to the intervention towards the end of the study than in its early stages. Consequently, the effect of the intervention might be confounded by any underlying temporal trends if, for example, there has been a general move towards improved patient outcomes, external to the study.19 Of course, judging whether or not an intervention is likely to do more good than harm is complex, especially when considering wider issues such as opportunity costs. A stepped wedge may mean that all clusters are exposed to an ineffective intervention if initial estimates of effect prove unfounded.
Selecting a randomised approach
Stepped-wedge trials and cRCTs both offer a means of evaluating complex interventions and large-scale transformations. In what situation should one be preferred? Consider a hypothetical study, involving a group-based weight loss programme with internet support. Early pilot investigations suggest that the intervention is effective, with a primary outcome of weight loss after 6 months. There is interest locally in implementing the programme; eight obesity clinics have provisionally agreed to participate.
In this example, we assume a minimum duration of follow-up of 6 months, and monthly introduction of the intervention into new clusters. In the event that the intervention is effective, the final results would be available in 14 months using a stepped-wedge design. By that stage, all the study clusters would have at least 6 months’ experience of using the intervention, and have been reaping the benefits. In contrast, if a parallel-arm cRCT were used, this result would have been known within 6 months. Four of the clusters would have been delivering the programme since the start of the trial. However, it would now be necessary to ‘fast track’ the intervention in the four control clusters.
On the other hand, consider if the intervention were not effective. With a stepped-wedge design, the final results would again be available in 14 months. However, by that point, all of the clusters would have been exposed to an intervention that does not work, and all would have been delivering it to patients for at least 6 months. Consequently, ethical scrutiny of this type of trial is important. In addition, because data collection usually takes place sequentially, the researchers would have collected a full set of trial data on 14 separate occasions (although a smaller number of data collection periods could be considered). If a cRCT were used, the results would be known within 6 months. Only half of the clusters would have been exposed to an intervention that did not work, and trial data would have been collected only once.
Key questions to be addressed before commencing such a trial include:
- What is the strength of the evidence that the intervention is more likely to do more good than harm? Considerations of costs and opportunity costs may be relevant.
- As well as effectiveness, what other issues are being assessed by the trial, such as learning and possible decay of the intervention effect?
- How quickly could an effective intervention be rolled out to control clusters if a cRCT were conducted?
The optimal conditions for a stepped wedge are likely to be when there is a lack of evidence of effectiveness but a strong belief that the intervention will do more good than harm.20 It may also be relevant when measurement of the effect of the intervention over time is important, including evaluation of staff learning and the effect of the service ‘bedding down’. In contrast, a cRCT may be more appropriate when the evidence of effectiveness is not clear-cut and rapid implementation in control clusters is possible. cRCTs have the added advantage that half of the study sample is ‘protected’, should the intervention not work, and the data collection burden is minimised.
In many situations, the concerns of those commissioning the evaluation may outweigh methodological issues. The constraints under which policy-makers and service managers operate may mean that a stepped wedge is the only way to gain agreement to randomisation, because all clusters will receive the intervention during the research. As a consequence, stepped-wedge designs may be particularly useful when policy dictates that an initiative will be rolled out widely, and there is scope to do this in a stepped way to enhance evaluation.
There are examples of successful cRCTs of complex policy interventions, which have successfully negotiated issues around randomisation and implementation, such as a cRCT of a scheme to provide free healthy breakfasts in Welsh primary schools.22 Of the 608 schools invited to participate, 111 agreed to be randomised and participate in data collection activities. Stepped-wedge designs have the potential to appear more attractive to stakeholders and may encourage higher levels of participation.
Pragmatic and explanatory attitudes to trial design
The PRagmatic-Explanatory Continuum Indicator Summary 2 (PRECIS-2) tool is designed to support trial design decisions consistent with the intended purpose of a planned RCT. This tool is especially useful if the intention is to design a RCT that will have results widely applicable within usual care at whatever level of the health service this may be, from primary to tertiary care settings. PRECIS-2 consists of nine domains: eligibility criteria, recruitment, setting, organisation, flexibility (delivery), flexibility (adherence), follow-up, primary outcome and primary analysis (Figure 2.2). These are discussed until a consensus is reached that that particular element of the trial is designed in a way which promotes the goal of the trial, be it a real-world applicability or research designed purely to understand efficacy. The process makes research teams more aware of the range of opinions and can help to ensure that design decisions are matched to the needs of those who will ultimately use the results of the trial.23
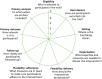
FIGURE 2.2
The PRECIS-2 wheel. Reproduced from The PRECIS-2 tool: designing trials that are fit for purpose, Loudon K, Treweek S, Sullivan F, Donnan P, Thorpe KE, Zwarenstein M, vol. 350, p. h2147, 2015, with permission from BMJ Publishing Group Ltd.
Non-randomised methods
Policy-makers overseeing the rollout of a new programme may not be willing to accept any form of randomisation. Even a lack of control over the order of entry of clusters into a stepped wedge may be unacceptable. This may be for political reasons, as we have described, or may occur when the intervention is already in widespread use, or key decisions about how it will be implemented have already been taken.1 In this scenario, the best evidence may come from a study design further down the conventional evidence hierarchy, such as a controlled before-and-after study or an interrupted time series. The revised MRC guidance cites a range of studies that have successfully evaluated complex interventions using non-randomised methods, including bans on smoking in public places,24 the impact of advice about sleep position on the incidence of sudden infant deaths25 and the impact of financial incentives on quality.26
Non-randomised methods that attempt to deal with the effects of selection, allocation and other biases include matching, conventional covariate adjustment using regression models and propensity score methods.27 A propensity score is defined as the conditional probability of an individual receiving an intervention, given the observed covariates. It can be used to balance the covariates between study groups, and therefore reduce the risk of bias.
This approach is potentially useful for the evaluation of complex interventions where randomisation is not feasible or desirable. However, further work is required to enable better adjustment for case-mix differences. For example, confounding by indication offers challenges to the analyst. In this situation, allocation to treatment is not otherwise ignorable but instead is subject to some latent (unrecognised or unmeasured) process associated with those who are treated, for example when skilled clinicians use their expert judgement to decide whether or not to treat a patient. This judgement may include criteria describing illness severity or patient frailty not included in the propensity score or, more likely, not formally measured. Methods such as propensity scores account only for known and observed patient characteristics.28 Methods that are better able to deal with unobserved factors include differences-in-differences analysis, instrumental variable analysis and regression discontinuity designs.29 There is further discussion of non-randomised designs in Essay 3 in this volume.
Of course, selection bias is only one form of bias in evaluations of complex interventions and large-scale transformations. There are other aspects of good RCT design and delivery that can mitigate other sources of bias (such as protocols, pre-specified primary outcomes and analytic plans) which can be applied to both randomised and non-randomised designs.30
Many non-randomised designs use routine data, which can have significant limitations in terms of accuracy and scope. Such data may lack patient-oriented outcomes such as quality of life and patient experience. A recent assessment of a primary care policy initiative in the Northwest to improve access to care31 was able to use routinely collected patient experience data from the General Practice Patient Survey to provide a more rounded assessment of outcomes, alongside admissions and health-care utilisation data.
Wider practical issues
In the final section of this essay, we outline practical issues of relevance to those seeking to implement RCTs of complex interventions and large-scale transformations.
Challenges in intervention design
As the revised MRC complex interventions guidance highlights, best practice is to develop complex interventions systematically and then to test them via pilot studies, before moving on to an exploratory and then a definitive evaluation.1 In practice, however, policy implementation often does not follow this model, leaving researchers with less opportunity to influence intervention design or delivery. An example from England would be the government’s population-wide prevention programme for cardiovascular disease (the NHS Health Check programme), which was rolled out despite a number of uncertainties about the evidence base underpinning this approach and how best to encourage the necessary uptake among patients to achieve the expected gains.32 Ideally, all intervention development should begin with identification of a relevant evidence base. This will often need to be supplemented by the application of appropriate theory, which may lead to greater probability of an effective intervention than a purely empirical or pragmatic approach.33
Even in cases where researchers cannot influence the design and implementation of an intervention, they can usefully help decision-makers to develop a theoretical understanding of the likely process of change by articulating the rationale behind it. This includes the changes that might be expected, and how change is to be achieved. A ‘logic model’ can help clarify causal assumptions, which may be drawn from behavioural, social science or organisational theory. Making explicit the causal assumptions and mechanisms of effect can allow external scrutiny of its plausibility and help evaluators decide which aspects of the intervention or its context to prioritise for investigation.3
Increasing attention is being paid to the possibility of researchers and decision-makers working together to design and subsequently evaluate interventions. One such example is the Practical Approach to Lung Health in South Africa (PALSA) trial, conducted in South Africa.34 From the start, researchers worked with front-line clinicians and managers to develop a guideline for the management of respiratory disease, which was tested and refined prior to implementation and evaluation. This approach is not without controversy, not least because decision-makers typically have a commitment to making an intervention work, which may impact on the evaluation and interpretation. For example, a metaregression describing features of effective computerised clinical decision support systems demonstrated that studies conducted by the system developers were more likely to show benefit than those conducted by a third party.35
Importance of process evaluation
Randomised controlled trials remain the best method for making causal inference and providing a reliable basis for decision-making. However, the basic RCT design cannot assess how or why an intervention does or does not achieve outcomes. To understand this, process evaluations should be routinely included in all evaluations of complex interventions to explain discrepancies between expected and observed outcomes, to understand how context influences outcomes and to provide insights to aid further implementation.1
Process evaluation can usefully investigate how the intervention was delivered, providing decision-makers with information about how it might be replicated. Issues considered may include training and support, communication and management structures, and how these structures interact with attitudes and circumstances of staff to shape the intervention. Process evaluations also commonly investigate the reach of interventions, for example whether or not the intended audience comes into contact with the intervention, and how.3 On the other hand, interventions may work, but in ways that were not expected or predicted.34 Qualitative or quantitative process evaluation may allow modification or updating of ‘logic models’ to account for the new data and plan further research. Further consideration is given to the role of qualitative methods in evaluative research in Essay 7.
The recently published MRC guidance on process evaluation of complex interventions provides a general framework for conducting and reporting this type of study.3 Grant et al.36 also provide a framework for design and reporting which is specific to cRCTs and encourages researchers to consider both the processes involving individuals in the target population on whom outcome data are collected and the processes relating to the clusters within which individuals are nested.
‘Realist’ evaluation methods purport to go beyond conventional evaluations to consider not just ‘what works’, but ‘what works, for whom, and in what circumstances?’ In order to answer these questions, realist evaluators aim to develop and continually update relevant programme theory, to identify the underlying generative mechanisms that explain ‘how’ the outcomes were caused and the influence of context.
Although realist methods are often contrasted with conventional RCTs and systematic reviews, the differences may not be so acute.2 For example, quantitative methods linked to RCTs exist for the analysis of mediation (to explore ‘how’) and moderation (to assess ‘for whom’), which can usefully complement qualitative work.37 Bonell et al.2 also propose that ‘realist’ aims can be examined in the context of RCTs. Such ‘realist’ RCTs should aim to examine the effects of intervention components separately and in combination, for example using multiarm studies and factorial designs; explore mechanisms of change, for example how pathway variables mediate intervention effects; use multiple RCTs to test how intervention effects vary with context; draw on complementary qualitative and quantitative data; and be oriented towards building and validating ‘mid-level’ programme theories which would set out how interventions interact with context to produce outcomes.2 ‘Realist’ RCTs, which not only provide an unbiased average effect estimate but also test and refine programme theory, are a potentially important extension of existing pragmatic RCT methods. Examples where this approach has been used successfully include a cRCT assessing the impact of school fruit ‘tuck shops’ on children’s consumption of fruit and snacks in deprived areas in south Wales and South West England38 and an evaluation of a peer-led intervention that aimed to prevent smoking uptake in secondary schools in England and Wales.39
The addition of process evaluations to RCTs does highlight potential tensions between quality and depth of evaluation, and its speed and efficiency. Process evaluations may require detailed qualitative and observational work, which may add significantly to the costs of the research and its duration. There is also a tension between an ‘independent’ process evaluation designed to make sense of the eventual trial results, and the desire for feedback from the evaluation mid-stream to deal with issues that may be limiting effectiveness.3
Designing for implementation
To successfully improve health care and population health, we need complex interventions with a degree of flexibility, resilient to contextual variation and therefore more likely to be implemented widely. We also require a clear understanding of contextual dependencies (such as target group, resources, system requirements) and system impacts. As we have outlined, attention to implementation issues in intervention design and understanding how or why an intervention works in the trial setting are both crucial to determining whether or not the intervention can be reliably disseminated to other settings.
The traditional linear ‘phases of research’ model remains influential. However, this may not be optimal for complex interventions, because it assumes that real-world effectiveness research naturally follows from successful efficacy research. As we outlined at the start of this essay, complex interventions interact with the context in which they are delivered. Contextual factors that may moderate the effectiveness of the intervention across settings and populations may not be adequately considered in design, which means that an intervention proven to be efficacious in one context may fail to demonstrate effectiveness when implemented in contexts for which it has not been designed.
Work in implementation science seeks to challenge the linear model of translation. It has been suggested that the characteristics which cause an intervention to be successful in efficacy research (e.g. intensive, complex, highly standardised) are fundamentally different from, and often at odds with, programmes that succeed in population-based effectiveness settings (e.g. having broad appeal, being adaptable for both participants and intervention agents).40 The Reach, Effectiveness, Adoption, Implementation, Maintenance (RE-AIM) model proposed for evaluating complex interventions is intended to refocus priorities on wider issues, and give balanced emphasis to internal and external validity.41 The model seeks to determine the characteristics of interventions that can reach large numbers of people, especially those who can most benefit; be widely adopted by different settings; be consistently implemented by staff with moderate levels of training and expertise; and produce replicable and long-lasting effects (and minimal negative impacts) at reasonable cost. An alternative model, normalisation process theory, highlights a series of constructs representing different types of work that people do in implementation that could inform intervention design, and linking it to ideas about agency, social and cognitive mechanisms, and collective action from sociology and psychology.42 A range of other relevant frameworks and models have been reviewed.43,44 For further discussion of current issues in implementation, see Essay 8 in this volume.
Greater attention needs to be paid to planning and documenting intervention reach, adoption, implementation and maintenance. One solution is to extend feasibility and pilot studies. Such studies would not only optimise evaluation methods, and further develop underlying theories or logic models, but also allow detailed consideration of how interventions would be adopted, implemented and maintained in real-world contexts. However, such methods may increase the cost and duration of evaluation and may not always be feasible.
Trial speed and efficiency
As noted in Selecting a randomised approach, a key limitation of current RCTs is their lack of speed and high cost, and there is significant interest in ways of making RCT delivery more efficient.
Randomised controlled trials rely on patients, staff and organisations to volunteer to take part. However, patient recruitment and retention remains a significant problem45 and the evidence base for recruitment and retention is very sparse.46,47 Many health organisations are disengaged with the research production infrastructure, which has implications for the generalisability of findings. To maximise replicability, studies need to be conducted in a wide range of settings to avoid the clear gaps between the populations in research and those to which the results are expected to apply.
There is an urgent need for research to support the more efficient delivery of RCTs.48 This might involve use of qualitative research to explore delivery49 or patient and public involvement to design trials more sensitive to the needs of patients and other stakeholders.50 More consistent outcome measurement and reporting51 might avoid excessive measurement burden. Methodological studies may be usefully embedded in existing trials to grow the evidence-base for efficient delivery.52,53 The cohort multiple RCT uses routine measurement of patients in a cohort to facilitate both recruitment and outcome measurement. Routine measurement of patients in the cohort eases the identification of patients for RCTs, while the cohort may provide a recruitment process which more naturally replicates clinical practice.
There are exemplars for more efficient trial delivery. The Ontario Printed Education Message Trials demonstrate the possibility of conducting large-scale, randomised studies at relatively low cost.54 General practitioners in Ontario, Canada, were randomised by practice to receive a range of reminders about retinal screening in diabetic patients. The reminders were mailed to each physician in conjunction with a widely read professional newsletter. All general practitioners in the province were included in the study, and hence the result provides both a pragmatic and a reliable assessment of the intervention in that context. Alongside a study of the effectiveness of the various interventions,54 the authors also conducted a theory-based process evaluation to assess physicians’ intentions related to referring patients for retinopathy screening, as well as their attitude, subjective norm and perceived behavioural control.55
Medical care and associated research is undergoing something of a paradigm shift with the introduction and use of electronic health records. These provide major opportunities owing to their scope and amenability to analysis. The idea of a ‘learning health-care system’ is based in part on better use of these data to support rapid evaluation (including RCTs and other quasi-experiments) and system-wide learning.56 The potential power of electronic health records in the context of RCTs is beginning to receive attention, particularly the potential to study large samples at relatively low cost, driving improvements in efficiency.57 In the USA, Clinical Data Research Networks supported by PCORnet (National Patient-Centered Clinical Research Network) build capacity for conducting both RCTs and observational effectiveness studies by collating data in a compatible format from a range of different health-care providers, including integrated delivery systems, academic medical centres and safety net clinics.58
Similarly in the UK, cRCTs have been implemented using electronic health records to evaluate the effectiveness of computer-delivered decision support tools in reducing antibiotic prescribing for respiratory tract infections in primary care59 and improving risk factor control after first stroke.60 Both studies made use of electronic health records available via the Clinical Practice Research Datalink (CPRD), which includes anonymised data for approximately 7–8% of UK general practices, linked to a range of other data including mortality information and cancer registrations.61 This experience provides compelling evidence that RCTs may be performed efficiently in large samples of this kind. Process evaluation may also be carried out alongside this type of study.62
However, there are significant challenges. Those who contribute information to data sets such as CPRD are skilled at collecting data, and not necessarily representative of their peers. The geographical dispersal of sites in RCTs using electronic health records may present a challenge for research governance and intervention implementation.63 For example, in a cRCT which used electronic records to study antibiotic prescribing, because the practices contributing to the CPRD are anonymous, permission had to be sought from 155 separate commissioning organisations for the English general practices alone. Further permissions were required in Scotland, Wales and Northern Ireland. The process took almost a year to complete before the RCT could commence.63 Such requirements make it difficult to realise the potential efficiency gains of such trials.
There are always challenges concerning the accuracy of records, and these records also provide a potentially partial view of outcomes. For example, such records rarely include patient-centred outcomes such as self-reported quality of life or patient satisfaction. Sometimes, the lack of patient-oriented outcomes is less of a problem (e.g. where the focus is on professional behaviour), but there is a danger that the efficiency of RCTs using electronic health records means that patient-oriented outcomes are de-emphasised compared with ‘harder’ measures such as mortality, or policy-maker and manager priorities such as quality of care and health-care utilisation (especially hospital admissions).
Working with decision-makers
Finally, although the purpose of evaluation is to inform decision-making by policy-makers and service providers, there is currently something of a disconnect between those who commission research and those who deliver it. As we have outlined, challenges may arise because policy-makers are often keen to pilot a new intervention in favourable circumstances, and there may be an unwillingness to evaluate at all, in case the programme is not effective. Others argue that coproduction of research with decision-makers results in a loss of researcher independence. However, there are innovative examples of attempts to bridge this gap, such as the National Institute for Health Research Collaborations for Leadership in Applied Health Research and Care in England. Evaluability assessments have also been trialled in Scotland, encouraging early dialogue between researchers and decision-makers to establish whether or not and how to evaluate programmes and policies.64
One of the principal challenges, however, is managing potentially conflicting time scales. Decision-makers typically require a swift answer, which may be challenging to deliver using rigorous methods. This may be compounded where funding and regulatory approvals must be secured before an evaluation can commence, which may make it difficult to conduct baseline assessments prior to an intervention. On the other hand, in health systems in repeated flux, managerial staff may not be in post for extended periods of time, making it challenging for researchers to establish long-term relationships with key decision-makers. In these circumstances, service needs may over-rule methodological concerns, and researchers may have to use evaluation methods that would provide the best possible answer in the time available.
Conclusions
There is significant demand from decision-makers for evaluations and many complex interventions and service transformations remain unevaluated. Evaluation methodology has progressed significantly in the past 10 years. cRCTs are now well established, stepped-wedge designs show great promise, and there are an increasing array of non-randomised methods available. There is increasing acceptance of the importance of theory in intervention design, and of the role of process evaluation in helping to interpret why interventions succeed and fail.
Nevertheless, we have identified important tensions which face the evaluation community. Development of interventions which are efficacious is no longer sufficient. There is now a demand for interventions which deliver better outcomes, can achieve those benefits in different contexts and can be implemented at pace and scale to ensure that local trial impacts translate into population health impact. At the same time, competition for research funding remains acute, and funders and decision-makers demand faster, more efficient evaluations that can better meet their needs and timelines. These demands will place a premium on continued methodological development to ensure that evaluation keeps pace with innovation in health services.
Acknowledgements
We thank Martin Gulliford for helpful feedback.
Contributions of authors
Helen Barratt (Senior Clinical Research Associate, Health Services Research) and Peter Bower (Professor, Health Services Research) wrote the first draft of the essay.
Marion Campbell (Professor, Health Services Research), Laurence Moore (Professor, Public Health Research) and Merrick Zwarenstein (Professor, Family Medicine) commented on the draft and provided additional material.
References
- 1.
- Craig P, Dieppe P, MacIntyre S, Michie S, Nazareth I, Petticrew M. Developing and Evaluating Complex Interventions: New Guidance. London: MRC; 2008. [PMC free article: PMC2769032] [PubMed: 18824488]
- 2.
- Bonell C, Fletcher A, Morton M, Lorenc T, Moore L. Realist randomised controlled trials: a new approach to evaluating complex public health interventions. Soc Sci Med 2012;75:2299–306. 10.1016/j.socscimed.2012.08.032. [PubMed: 22989491] [CrossRef]
- 3.
- Moore GF, Audrey S, Barker M, Bond L, Bonell C, Hardeman W, et al. Process evaluation of complex interventions: Medical Research Council guidance. BMJ 2015;350:h1258. 10.1136/bmj.h1258. [PMC free article: PMC4366184] [PubMed: 25791983] [CrossRef]
- 4.
- Richards D, Hallberg I. Complex Interventions in Health: An Overview of Research Methods. Oxford: Routledge; 2015.
- 5.
- Best A, Greenhalgh T, Lewis S, Saul JE, Carroll S, Bitz J. Large-system transformation in health care: a realist review. Milbank Q 2012;90:421–56. 10.1111/j.1468-0009.2012.00670.x. [PMC free article: PMC3479379] [PubMed: 22985277] [CrossRef]
- 6.
- Macleod MR, Michie S, Roberts I, Dirnagl U, Chalmers I, Ioannidis JPA, et al. Biomedical research: increasing value, reducing waste. Lancet 2014;383:101–4. 10.1016/S0140-6736(13)62329-6. [PubMed: 24411643] [CrossRef]
- 7.
- Ukoumunne OC, Gulliford MC, Chinn S, Sterne JA, Burney PG, Donner A. Methods in health service research. Evaluation of health interventions at area and organisation level. BMJ 1999;319:376–9. 10.1136/bmj.319.7206.376. [PMC free article: PMC1126996] [PubMed: 10435968] [CrossRef]
- 8.
- Moberg J, Kramer M. A brief history of the cluster randomised trial design. J R Soc Med 2015;108:192–8. 10.1177/0141076815582303. [PMC free article: PMC4484210] [PubMed: 26022551] [CrossRef]
- 9.
- Campbell MK, Fayers PM, Grimshaw JM. Determinants of the intracluster correlation coefficient in cluster randomized trials: the case of implementation research. Clin Trials 2005;2:99–107. 10.1191/1740774505cn071oa. [PubMed: 16279131] [CrossRef]
- 10.
- Gulliford MC, Adams G, Ukoumunne OC, Latinovic R, Chinn S, Campbell MJ. Intraclass correlation coefficient and outcome prevalence are associated in clustered binary data. J Clin Epidemiol 2005;58:246–51. 10.1016/j.jclinepi.2004.08.012. [PubMed: 15718113] [CrossRef]
- 11.
- Eldridge SM, Ashby D, Kerry S. Sample size for cluster randomized trials: effect of coefficient of variation of cluster size and analysis method. Int J Epidemiol 2006;35:1292–300. 10.1093/ije/dyl129. [PubMed: 16943232] [CrossRef]
- 12.
- Campbell MK, Thomson S, Ramsay CR, MacLennan GS, Grimshaw JM. Sample size calculator for cluster randomized trials. Comput Biol Med 2004;34:113–25. 10.1016/S0010-4825(03)00039-8. [PubMed: 14972631] [CrossRef]
- 13.
- Campbell MK, Elbourne DR, Altman DG, CONSORT group. CONSORT statement: extension to cluster randomised trials. BMJ 2004;328:702–8. 10.1136/bmj.328.7441.702. [PMC free article: PMC381234] [PubMed: 15031246] [CrossRef]
- 14.
- Campbell MK, Piaggio G, Elbourne DR, Altman DG. CONSORT 2010 statement: extension to cluster randomised trials. BMJ 2012;345:e5661. 10.1136/bmj.e5661. [PubMed: 22951546] [CrossRef]
- 15.
- Schulz KF, Altman DG, Moher D, CONSORT Group. CONSORT 2010 statement: updated guidelines for reporting parallel group randomised trials. BMJ 2010;340:c332. 10.1136/bmj.c332. [PMC free article: PMC2844940] [PubMed: 20332509] [CrossRef]
- 16.
- Weijer C, Grimshaw JM, Eccles MP, McRae AD, White A, Brehaut JC, et al. The Ottawa Statement on the Ethical Design and Conduct of Cluster Randomized Trials. PLOS Med 2012;9:e1001346. 10.1371/journal.pmed.1001346. [PMC free article: PMC3502500] [PubMed: 23185138] [CrossRef]
- 17.
- Beard E, Lewis JJ, Copas A, Davey C, Osrin D, Baio G, et al. Stepped wedge randomised controlled trials: systematic review of studies published between 2010 and 2014. Trials 2015;16:353. 10.1186/s13063-015-0839-2. [PMC free article: PMC4538902] [PubMed: 26278881] [CrossRef]
- 18.
- Brown CA, Lilford RJ. The stepped wedge trial design: a systematic review. BMC Med Res Methodol 2006;6:54. 10.1186/1471-2288-6-54. [PMC free article: PMC1636652] [PubMed: 17092344] [CrossRef]
- 19.
- Hemming K, Haines TP, Chilton PJ, Girling AJ, Lilford RJ. The stepped wedge cluster randomised trial:rationale, design, analysis, and reporting. BMJ 2015;16:350. 10.1136/bmj.h391. [PubMed: 25662947] [CrossRef]
- 20.
- Hargreaves JR, Copas AJ, Beard E, Osrin D, Lewis JJ, Davey C, et al. Five questions to consider before conducting a stepped wedge trial. Trials 2015;16:350. 10.1186/s13063-015-0841-8. [PMC free article: PMC4538743] [PubMed: 26279013] [CrossRef]
- 21.
- Mdege ND, Man M-S, Taylor Nee Brown CA, Torgerson DJ. Systematic review of stepped wedge cluster randomized trials shows that design is particularly used to evaluate interventions during routine implementation. J Clin Epidemiol 2011;64:936–48. 10.1016/j.jclinepi.2010.12.003. [PubMed: 21411284] [CrossRef]
- 22.
- Murphy S, Moore GF, Tapper K, Lynch R, Clarke R, Raisanen L, et al. Free healthy breakfasts in primary schools: a cluster randomised controlled trial of a policy intervention in Wales, UK. Public Health Nutr 2011;14:219–26. 10.1017/S1368980010001886. [PubMed: 20602868] [CrossRef]
- 23.
- Loudon K, Treweek S, Sullivan F, Donnan P, Thorpe KE, Zwarenstein M. The PRECIS-2 tool: designing trials that are fit for purpose. BMJ 2015;350:h2147. 10.1136/bmj.h2147. [PubMed: 25956159] [CrossRef]
- 24.
- Semple S, Creely KS, Naji A, Miller BG, Ayres JG. Secondhand smoke levels in Scottish pubs: the effect of smoke-free legislation. Tob Control 2007;16:127–32. 10.1136/tc.2006.018119. [PMC free article: PMC2598470] [PubMed: 17400951] [CrossRef]
- 25.
- Fleming PJ, Blair PS, Bacon C, Bensley D, Smith I, Taylor E, et al. Environment of infants during sleep and risk of the sudden infant death syndrome: results of 1993–5 case–control study for confidential inquiry into stillbirths and deaths in infancy. Confidential enquiry into stillbirths and deaths regional coordinators and researchers. BMJ 1996;313:191–5. 10.1136/bmj.313.7051.191. [PMC free article: PMC2351639] [PubMed: 8696193] [CrossRef]
- 26.
- Sutton M, Nikolova S, Boaden R, Lester H, McDonald R, Roland M. Reduced mortality with hospital pay for performance in England. N Engl J Med 2012;367:1821–8. 10.1056/NEJMsa1114951. [PubMed: 23134382] [CrossRef]
- 27.
- D’Agostino RB. Propensity score methods for bias reduction in the comparison of a treatment to a non-randomized control group. Stat Med 1998;17:2265–81. 10.1002/(SICI)1097-0258(19981015)17:19<2265::AID-SIM918>3.0.CO;2-B. [PubMed: 9802183] [CrossRef]
- 28.
- Freemantle N, Marston L, Walters K, Wood J, Reynolds MR, Petersen I. Making inferences on treatment effects from real world data: propensity scores, confounding by indication, and other perils for the unwary in observational research. BMJ 2013;347:f6409. 10.1136/bmj.f6409. [PubMed: 24217206] [CrossRef]
- 29.
- Greenland S. An introduction to instrumental variables for epidemiologists. Int J Epidemiol 2000;29:722–9. 10.1093/ije/29.4.722. [PubMed: 10922351] [CrossRef]
- 30.
- Craig P, Cooper C, Gunnell D, Haw S, Lawson K, Macintyre S, et al. Using natural experiments to evaluate population health interventions: new Medical Research Council guidance. J Epidemiol Community Health 2012;66:1182–6. 10.1136/jech-2011-200375. [PMC free article: PMC3796763] [PubMed: 22577181] [CrossRef]
- 31.
- National Institute for Health Research CLAHRC Greater Manchester. NHS Greater Manchester Primary Care Demonstrator Evaluation: Final Report NIHR CLAHRC Greater Manchester; 2015.URL: http://clahrc-gm
.nihr .ac.uk/wp-content/uploads /PCDE-final-report-full-final.pdf(accessed 21 April 2016). - 32.
- Abdalrahman B, Soljak M. NHS health checks: an update on the debate and program implementation in England. J Ambul Care Manage 2015;38:5–9. 10.1097/JAC.0000000000000070. [PMC free article: PMC4272224] [PubMed: 25340472] [CrossRef]
- 33.
- Albarracín D, Gillette JC, Earl AN, Glasman LR, Durantini MR, Ho M-H. A test of major assumptions about behavior change: a comprehensive look at the effects of passive and active HIV-prevention interventions since the beginning of the epidemic. Psychol Bull 2005;131:856–97. 10.1037/0033-2909.131.6.856. [PMC free article: PMC2713787] [PubMed: 16351327] [CrossRef]
- 34.
- Fairall LR, Zwarenstein M, Bateman ED, Bachmann M, Lombard C, Majara BP, et al. Effect of educational outreach to nurses on tuberculosis case detection and primary care of respiratory illness: pragmatic cluster randomised controlled trial. BMJ 2005;331:750–4. 10.1136/bmj.331.7519.750. [PMC free article: PMC1239979] [PubMed: 16195293] [CrossRef]
- 35.
- Roshanov PS, Fernandes N, Wilczynski JM, Hemens BJ, You JJ, Handler SM, et al. Features of effective computerised clinical decision support systems: meta-regression of 162 randomised trials. BMJ 2013;346:f657. 10.1136/bmj.f657. [PubMed: 23412440] [CrossRef]
- 36.
- Grant A, Treweek S, Dreischulte T, Foy R, Guthrie B. Process evaluations for cluster-randomised trials of complex interventions: a proposed framework for design and reporting. Trials 2013;14:15. 10.1186/1745-6215-14-15. [PMC free article: PMC3600672] [PubMed: 23311722] [CrossRef]
- 37.
- Emsley R, Dunn G, White IR. Mediation and moderation of treatment effects in randomised controlled trials of complex interventions. Stat Methods Med Res 2010;19:237–70. 10.1177/0962280209105014. [PubMed: 19608601] [CrossRef]
- 38.
- Moore L, Tapper K. The impact of school fruit tuck shops and school food policies on children’s fruit consumption: a cluster randomised trial of schools in deprived areas. J Epidemiol Community Health 2008;62:926–31. 10.1136/jech.2007.070953. [PubMed: 18483061] [CrossRef]
- 39.
- Campbell R, Starkey F, Holliday J, Audrey S, Bloor M, Parry-Langdon N, et al. An informal school-based peer-led intervention for smoking prevention in adolescence (ASSIST): a cluster randomised trial. Lancet 2008;371:1595–602. 10.1016/S0140-6736(08)60692-3. [PMC free article: PMC2387195] [PubMed: 18468543] [CrossRef]
- 40.
- Glasgow RE, Lichtenstein E, Marcus AC. Why don’t we see more translation of health promotion research to practice? Rethinking the efficacy-to-effectiveness transition. Am J Public Health 2003;93:1261–7. 10.2105/AJPH.93.8.1261. [PMC free article: PMC1447950] [PubMed: 12893608] [CrossRef]
- 41.
- Glasgow RE, Vogt TM, Boles SM. Evaluating the public health impact of health promotion interventions: the RE-AIM framework. Am J Public Health 1999;89:1322–7. 10.2105/AJPH.89.9.1322. [PMC free article: PMC1508772] [PubMed: 10474547] [CrossRef]
- 42.
- May C. Towards a general theory of implementation. Implement Sci 2013;8:18. 10.1186/1748-5908-8-18. [PMC free article: PMC3602092] [PubMed: 23406398] [CrossRef]
- 43.
- Tabak RG, Khoong EC, Chambers D, Brownson RC. Bridging research and practice. Am J Prevent Med 2012;43:337–50. 10.1016/j.amepre.2012.05.024. [PMC free article: PMC3592983] [PubMed: 22898128] [CrossRef]
- 44.
- Moullin JC, Sabater-Hernández D, Fernandez-Llimos F, Benrimoj SI. A systematic review of implementation frameworks of innovations in healthcare and resulting generic implementation framework. Health Res Policy Syst 2015;13:16. 10.1186/s12961-015-0005-z. [PMC free article: PMC4364490] [PubMed: 25885055] [CrossRef]
- 45.
- Sully BGO, Julious SA, Nicholl J. A reinvestigation of recruitment to randomised, controlled, multicenter trials: a review of trials funded by two UK funding agencies. Trials 2013;14:166. 10.1186/1745-6215-14-166. [PMC free article: PMC3691846] [PubMed: 23758961] [CrossRef]
- 46.
- Brueton VC, Tierney JF, Stenning S, Meredith S, Harding S, Nazareth I, et al. Strategies to improve retention in randomised trials: a Cochrane systematic review and meta-analysis. BMJ Open 2014;4:e003821. 10.1136/bmjopen-2013-003821. [PMC free article: PMC3918995] [PubMed: 24496696] [CrossRef]
- 47.
- Treweek S, Lockhart P, Pitkethly M, Cook JA, Kjeldstrøm M, Johansen M, et al. Methods to improve recruitment to randomised controlled trials: Cochrane systematic review and meta-analysis. BMJ Open 2013;3:e002360. 10.1136/bmjopen-2012-002360. [PMC free article: PMC3586125] [PubMed: 23396504] [CrossRef]
- 48.
- Treweek S, Altman DG, Bower P, Campbell M, Chalmers I, Cotton S, et al. Making randomised trials more efficient: report of the first meeting to discuss the Trial Forge platform. Trials 2015;16:261. 10.1186/s13063-015-0776-0. [PMC free article: PMC4475334] [PubMed: 26044814] [CrossRef]
- 49.
- Donovan JL, Paramasivan S, de Salis I, Toerien M. Clear obstacles and hidden challenges: understanding recruiter perspectives in six pragmatic randomised controlled trials. Trials 2014;15:5. 10.1186/1745-6215-15-5. [PMC free article: PMC3892115] [PubMed: 24393291] [CrossRef]
- 50.
- Ennis L, Wykes T. Impact of patient involvement in mental health research: longitudinal study. Br J Psychiatry 2013;203:381–6. 10.1192/bjp.bp.112.119818. [PubMed: 24029538] [CrossRef]
- 51.
- Gargon E, Gurung B, Medley N, Altman DG, Blazeby JM, Clarke M, et al. Choosing important health outcomes for comparative effectiveness research: a systematic review. PLOS ONE 2014;9:e99111. 10.1371/journal.pone.0099111. [PMC free article: PMC4059640] [PubMed: 24932522] [CrossRef]
- 52.
- Rick J, Graffy J, Knapp P, Small N, Collier DJ, Eldridge S, et al. Systematic techniques for assisting recruitment to trials (START): study protocol for embedded, randomized controlled trials. Trials 2014;15:407. 10.1186/1745-6215-15-407. [PMC free article: PMC4230578] [PubMed: 25344684] [CrossRef]
- 53.
- Smith V, Clarke M, Devane D, Begley C, Shorter G, Maguire L. SWAT 1: what effects do site visits by the principal investigator have on recruitment in a multicentre randomized trial? J Evid Based Med 2013;6:136–7. 10.1111/jebm.12049. [PubMed: 24325369] [CrossRef]
- 54.
- Zwarenstein M, Shiller SK, Croxford R, Grimshaw JM, Kelsall D, Paterson JM, et al. Printed educational messages aimed at family practitioners fail to increase retinal screening among their patients with diabetes: a pragmatic cluster randomized controlled trial [ISRCTN72772651]. Implement Sci 2014;9:87. 10.1186/1748-5908-9-87. [PMC free article: PMC4261896] [PubMed: 25098587] [CrossRef]
- 55.
- Grimshaw JM, Presseau J, Tetroe J, Eccles MP, Francis JJ, Godin G, et al. Looking inside the black box: results of a theory-based process evaluation exploring the results of a randomized controlled trial of printed educational messages to increase primary care physicians’ diabetic retinopathy referrals [Trial registration number ISRCTN72772651]. Implement Sci 2014;9:86. 10.1186/1748-5908-9-86. [PMC free article: PMC4261878] [PubMed: 25098442] [CrossRef]
- 56.
- Krumholz HM. Big data and new knowledge in medicine: the thinking, training, and tools needed for a learning health system. Health Aff (Millwood) 2014;33:1163–70. 10.1377/hlthaff.2014.0053. [PMC free article: PMC5459394] [PubMed: 25006142] [CrossRef]
- 57.
- Van Staa T-P, Dyson L, McCann G, Padmanabhan S, Belatri R, Goldacre B, et al. The opportunities and challenges of pragmatic point-of-care randomised trials using routinely collected electronic records: evaluations of two exemplar trials. Health Technol Assess 2014;18(43). 10.3310/hta18430. [PMC free article: PMC4781625] [PubMed: 25011568] [CrossRef]
- 58.
- Clinical Data Research Networks. PCORnet. 2016. URL: www
.pcornet.org/clinical-data-research-networks/ (accessed February 2016). - 59.
- Gulliford MC, Staa T van Dregan A, McDermott L, McCann G, Ashworth M, et al. Electronic health records for intervention research: a cluster randomized trial to reduce antibiotic prescribing in primary care (eCRT study). Ann Fam Med 2014;12:344–51. 10.1370/afm.1659. [PMC free article: PMC4096472] [PubMed: 25024243] [CrossRef]
- 60.
- Dregan A, van Staa TP, McDermott L, McCann G, Ashworth M, Charlton J, et al. Point-of-care cluster randomized trial in stroke secondary prevention using electronic health records. Stroke 2014;45:2066–71. 10.1161/STROKEAHA.114.005713. [PubMed: 24903985] [CrossRef]
- 61.
- Herrett E, Gallagher AM, Bhaskaran K, Forbes H, Mathur R, van Staa T, et al. Data Resource Profile: Clinical Practice Research Datalink (CPRD). Int J Epidemiol 2015;44:827–36. 10.1093/ije/dyv098. [PMC free article: PMC4521131] [PubMed: 26050254] [CrossRef]
- 62.
- McDermott L, Yardley L, Little P, van Staa T, Dregan A, McCann G, et al. Process evaluation of a point-of-care cluster randomised trial using a computer-delivered intervention to reduce antibiotic prescribing in primary care. BMC Health Serv Res 2014;14:594. 10.1186/s12913-014-0594-1. [PMC free article: PMC4260184] [PubMed: 25700144] [CrossRef]
- 63.
- Gulliford MC, van Staa TP, McDermott L, McCann G, Charlton J, Dregan A, et al. Cluster randomized trials utilizing primary care electronic health records: methodological issues in design, conduct, and analysis (eCRT study). Trials 2014;15:220. 10.1186/1745-6215-15-220. [PMC free article: PMC4062282] [PubMed: 24919485] [CrossRef]
- 64.
- Craig P, Campbell M. Evaluability Assessment: A Systematic Approach to Deciding Whether and How to Evaluate Programmes and Policies (Working Paper). What Works Scotland; 2015. URL: http:
//whatworksscotland .ac.uk/wp-content /uploads/2015/07/WWS-Evaluability-Assessment-Working-paper-final-June-2015.pdf (accessed February 2016).
List of abbreviations
- CONSORT
Consolidated Standards of Reporting Trials
- CPRD
Clinical Practice Research Datalink
- cRCT
cluster randomised controlled trial
- ICC
intracluster correlation coefficient
- MRC
Medical Research Council
- PRECIS-2
PRagmatic-Explanatory Continuum Indicator Summary 2
- RCT
randomised controlled trial
- Declared competing interests of authors: Helen Barratt is supported by the National Institute for Health Research (NIHR) Collaboration for Leadership in Applied Health Research and Care North Thames at Bart’s Health NHS Trust. Laurence Moore reports that he is Scientific Advisor to and former Director of Decipher Impact Ltd. The Health Services Research Unit, University of Aberdeen, receives core funding from the Chief Scientist Office (SCO) of the Scottish Government’s Health and Social Care Directorates (CSO). The Medical Research Council (MRC)/CSO Social and Public Health Sciences Unit, University of Glasgow, receives core funding from the CSO (SPHSU14) and the MRC (MC_UU_12017/14). The views expressed are those of the authors and not necessarily those of the NHS, the NIHR or the Department of Health.
- This essay should be referenced as follows: Barratt H, Campbell M, Moore L, Zwarenstein M, Bower P. Randomised controlled trials of complex interventions and large-scale transformation of services. In Raine R, Fitzpatrick R, Barratt H, Bevan G, Black N, Boaden R, et al. Challenges, solutions and future directions in the evaluation of service innovations in health care and public health. Health Serv Deliv Res 2016;4(16). pp. 19–36.
Publication Details
Author Information and Affiliations
Authors
Helen Barratt,1 Marion Campbell,2 Laurence Moore,3 Merrick Zwarenstein,4 and Peter Bower5.Affiliations
Copyright
Included under terms of UK Non-commercial Government License.
Publisher
NIHR Journals Library, Southampton (UK)
NLM Citation
Barratt H, Campbell M, Moore L, et al. Randomised controlled trials of complex interventions and large-scale transformation of services. In: Raine R, Fitzpatrick R, Barratt H, et al. Challenges, solutions and future directions in the evaluation of service innovations in health care and public health. Southampton (UK): NIHR Journals Library; 2016 May. (Health Services and Delivery Research, No. 4.16.) Essay 2. doi: 10.3310/hsdr04160-19