Included under terms of UK Non-commercial Government License.
NCBI Bookshelf. A service of the National Library of Medicine, National Institutes of Health.
Sandall J, Murrells T, Dodwell M, et al. The efficient use of the maternity workforce and the implications for safety and quality in maternity care: a population-based, cross-sectional study. Southampton (UK): NIHR Journals Library; 2014 Oct. (Health Services and Delivery Research, No. 2.38.)

The efficient use of the maternity workforce and the implications for safety and quality in maternity care: a population-based, cross-sectional study.
Show detailsResearch questions
In order to fulfil the aims and objectives, our research question asked to what degree are maternal quality and safety outcomes explained by characteristics of NHS trusts, staffing levels and skill mix, after adjusting for mothers’ characteristics including clinical risk and sociodemographic factors. We wished to know: How important are staffing levels and skill mix in determining outcomes for women and babies? What is the relationship between maternity workforce staffing levels, quality and safety outcomes and health-care output? To what extent is there an optimal staffing mix? What is the implication of efficiency savings for key quality and safety indicators?
Study design
A cross-sectional analysis was undertaken using multilevel logistic regression to investigate the relationship between a number of quality and safety outcomes and the workforce configuration adjusting for confounding characteristics. A cost analysis using a framework where both outcomes and cost are taken into account when measuring efficiency was also completed.
Patient and public involvement
In order to ensure that service user views were included in the proposed research, a trained childbirth educator, with experience of facilitating learning and discussion with pregnant women and their partners (MD), was involved in the development of the proposal and the design of the research, and named as a joint applicant on the grant proposal. She is also an experienced maternity service user representative, having represented the views of women on a number of maternity projects and committees, including Midwifery 2020, the Maternity Care Working Party and the RCOG Women’s Network. She has been concerned with the experience of NHS patients generally and has served as a lay member on the NICE Guidance Development Group for Patient Experience in Adult NHS Services. Her interest in maternity research is reflected in her role as voluntary research networker for the National Childbirth Trust (NCT).
In addition, she has an interest in how maternity data can be used to inform women making decisions and choices about childbirth. Together with the data analyst on this project (RG), she represented BirthChoiceUK, a voluntary organisation which has, for the last 14 years, helped women to make choices about their maternity care through information provided by the website www.BirthChoiceUK.com.74 It provided information and maternity statistics for each maternity unit in the UK in an accessible format for parents to help them know what questions to ask locally and to decide where to plan to have their baby.
As joint applicant, and a user researcher, MD contributed to the development of the research proposal. This led to the consideration of wider quality outcomes for maternity services rather than a focus primarily on safety, and a more woman-focused approach to the concepts of productivity and efficiency.
It was originally intended that she would only attend and contribute to co-investigator meetings. However, it became clear that her knowledge of HES data and coding, quality metrics and women’s experience of maternity care – all derived from previous service user representative activities and driven from a woman-centred point of view – would be essential and valuable to the project and she therefore joined the project team. In doing so, she was able to have a hands-on role in the project, contributing on a regular basis to the direction of the study, including the choice of indicators to represent quality of maternity care and undertaking part of the research herself, developing a way of identifying women who had clinical risk factors relevant to birth.
She has already had, and will continue to have, a role in dissemination, having given both oral and poster presentations on the project and its interim findings at a number of conferences. Findings from this project will also inform future developments of BirthChoiceUK in its aim to provide high-quality information for parents. For example, HES data, cleaned and stratified by clinical risk and parity using techniques developed in this project, are displayed on the Which? Birth Choice website (www.which.co.uk/birth-choice75), created in partnership with BirthChoiceUK. These data will help women understand differences in outcomes between maternity units in England and contribute to their decision about where to give birth.
Further patient and public involvement (PPI) was contributed by the members of the advisory group (see Appendix 1). The purpose of the advisory group was specifically to advise the co-investigator group on the study questions, analysis and outputs. The advisory group included representatives from the user organisations NCT, the Stillbirth and Neonatal Death Charity (SANDS) and the National Maternity Support Foundation (Jake’s Charity), which were able to comment on the progress and findings of the study at our two advisory group meetings. Their help will be sought for dissemination of the findings.
Data sources
We used the following data sets:
- HES from 143 NHS trusts in England, admitted patients (including the ‘maternity and baby tail’) for the NHS year 2010–11. Specifically, the population is women who delivered in an obstetric or maternity unit based in a NHS trust in England 2010–11.
- NHS Workforce Statistics, England: 2010–11 from the Health and Social Care Information Centre, which includes staff in post including bank staff.
- CQC Maternity Survey of Maternity Provider Trusts 2007 and survey of women’s experiences, 2007 and 2010, from the UK Data Archive.
- Office for National Statistics (ONS) Number of Maternities by Establishment, 2001/02 to 2010/11.
- The BirthChoiceUK database, held by Rod Gibson Associates Ltd and containing information on NHS trusts and maternity units by location and type (e.g. OU, FMU, AMU).
- Reference cost data by NHS trust – NHS reference costs 2010/11 were available under Open Government Licence v2.0 (URL: www.nationalarchives.gov.uk/doc/open-government-licence/version/2/).76
Data storage, governance and ethics
The data were stored in a MySQL database [MySQL version 5.5. This is an open source program (www.mysql.com) that is overseen by Oracle Corporation, Redwood Shores, CA, USA]. The flat HES data files were reorganised into a relational database to facilitate faster processing. To further speed processing, the mothers’ delivery records were separated from the general inpatient records as were mothers’ non-delivery inpatient records. A similar split was carried out for babies’ birth records. The loading, organisation and cleaning of data as well as the calculation of indicators were all performed by routines written in Python, thus ensuring reproducibility. The number of deliveries by communal establishment code for 2010/11 was obtained from the ONS under Open Government Licence v1.0 (URL: www.nationalarchives.gov.uk/doc/open-government-licence/). These were matched with communal establishment place using a list obtained from NHS Connecting for Health. Communal establishment place was matched to NHS trust using the BirthChoiceUK database. Advice regarding ethical approval was sought from King’s College Research Ethics Committee, which advised that ethics approval was not required because the team planned to undertake secondary analysis of existing anonymised data. Careful measures were taken to safeguard the source data, in line with College research governance policies and procedures.
The HES data set available to the study consisted of:
- HES Inpatient Records 2000/01 to 2010/11
- HES Outpatient records 2003/04 to 2010/11
- HES accident and emergency (A&E) records 2007/08 to 2010/11
- ONS-HES Linked Mortality Statistics (including neonatal deaths) 2000/01 to 2010/11.
In summary, a mother has a single HES delivery record with fields available to record a limited amount of information on nine babies born in a single episode. Each baby has its own HES birth record, but this is not linked to the mother’s delivery record. Only the year 2010/11 was of direct relevance to the project. However, using anonymised unique patient identifiers in the HES records, women delivering babies could be linked to previous inpatient and previous delivery records for years back to 2000/01. This allowed a more complete picture of a woman’s obstetric history to be built; for example events such as stillbirth or a caesarean in a previous pregnancy which were considered risk factors for the delivery recorded in 2010/11.
Data cleaning and quality
The quality of HES data is improving. Murray et al.77 examined the range and completeness of birth information recorded in HES and tested an approach for minimising the effect of hospital-level variations by selecting hospitals with high completeness of recording (90%) for key fields. They found that the proportion of missing data in key birth record fields has been decreasing annually, such as gestational age and birthweight (from 46.2% and 43.9% in 2005/06 to 18.1% and 16.9% in 2009/10 respectively). They compared the important characteristics such as size and access to specialist neonatal care between 71 high-coding and 85 low-coding hospitals and found no significant differences, suggesting hospitals with high birth record completeness may be generalisable and representative of all hospitals. Knight et al.78 found that analyses using HES data were affected by the completeness and consistency of data. They found that different analysis rules had a small effect on the statistics at a national level but the effect could be substantial for individual NHS trusts.
Thus, we have drawn upon the guidelines developed by Sinha et al.79 for reporting on data cleaning and quality. Appendix 2 provides an account of full data cleaning conducted for the study, prior to data analysis. The study restricted the records to NHS hospital deliveries resulting in a registrable birth. Duplicate delivery records were removed from the mother’s records. The babies’ birth records also contained duplicate records. These duplicates were not removed, as the majority of the project’s work concentrated on the mother’s delivery record and the resources were not available to clean both. We consulted the CQC regarding its Maternity Data Quality – Indicator specifications for maternity-related measures included within its surveillance programme, and developed a scoring system that was used to select records with the largest amount of most useful and relevant data to the project.
Study size and bias
We used the full census of 656,969 women’s deliveries in HES, so there was no bias caused by non-response. Any biases would therefore be caused by missing data, poorly recorded data or omitted variables from the risk adjustment model. Sample sizes for some indicators were reduced by the choice of denominator to create the indicator.
For example, healthy mother and healthy baby indicators (n = 518,698; 79%) were limited to records for which there was known gestational age, birth status, birthweight and postnatal duration. Normal birth (n = 548,272; 83%) was limited to records for which there was known onset of labour, mode of delivery and anaesthetic, and intact perineum (n = 493,449; 75%) was limited to women who did not have a caesarean. The final five indicators (delivery with bodily integrity, spontaneous vaginal delivery, elective caesarean, emergency caesarean and all caesareans) all had high levels of completeness (n = 656,135; 99.9%). Missing postcode information meant that a further 5462 delivery records (1%) were lost from the analysis, as were 68,482 records (10%) with mother’s age missing.
A pre-specified decision was taken not to include any trust where fewer than 80% of women could be coded for a particular indicator. Combining this with missing postcode data meant that the percentages of delivery records used in the multilevel models were as follows: healthy mother and healthy baby indicators (n = 431,391; 66%), normal birth (n = 467,022; 71%), intact perineum (n = 439,730; 67%, or 89% of women who had a vaginal delivery), and delivery with bodily integrity, spontaneous vaginal delivery, elective caesarean, emergency caesarean and all caesareans (n = 584,435; 89%).
Derivation of process and outcome indicators
The Institute of Medicine’s Crossing the Quality Chasm report11 identified large gaps between health care that is, and that could and should be delivered in the United States. The report identified six aims for quality improvement that have been widely adopted internationally; thus health care should be safe, effective, patient centred, timely, efficient and equitable. Some outcomes are also influenced by factors outside health care, for example by social and structural determinants of health. Some neonatal outcomes are influenced by quality of care in the neonatal sector. Boxes 1 and 2 detail the result of a review of quality indicators used in maternity care. Sources include AHRQ,30 UK Policy and guidelines,6,80 CQC,81 CQUIN,18,19 ONS,82 NHS Operating Framework 2012/13,83 RCOG,25 Midwifery 2020,13 Safer Childbirth: Minimum Standards for the Organisation and Delivery of Care in Labour,7 Information Centre Indicators for quality improvement 201320 and the Australian Council on Healthcare.84
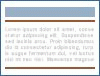
BOX 1
Process indicators Women who have seen a midwife or a maternity health-care professional, for health and social care assessment of needs, risks and choices by 12 weeks and 6 days of pregnancy.
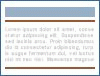
BOX 2
Outcome indicators Women’s experience of maternity services CQC questions.
Indicators used in analysis
The selection of indicators was decided in consultation with the advisory group and informed by needing to have a balance of positive and negative indicators, the importance to women, costs, and the availability and quality of coding within the HES data set. Three indicators were derived to indicate a healthy mother and healthy baby, thus reflecting a concept of harm-free care, avoidance of longer-term morbidity and a positive outcome. The mode of birth indicators were chosen to compare important processes and outcomes across trusts and with other studies. Ten final indicators (some were composites) that measured maternal and infant outcomes were developed and are outlined in Table 1.
TABLE 1
Outcome indicators used in analysis: numbers achieving the indicator and percentages of eligible total
The subcomponents for each composite indicator are shown in Tables 2–5. A full description of each composite outcome is also provided; please see Chapter 3, Outcome indicators.
TABLE 2
Delivery with bodily integrity: composite indicator components
TABLE 5
Healthy baby: composite indicator components
TABLE 3
Normal birth: composite indicator components
TABLE 4
Healthy mother: composite indicator components
Independent variables
We included mother’s characteristics measured at the individual level that are known to affect the outcomes of interest. These included age, parity, clinical risk at the end of pregnancy as measured by the NICE guideline for intrapartum care,80 ethnicity, area socioeconomic deprivation as measured by the Index of Multiple Deprivation (IMD),85 geographical location (urban/rural) and region.
Trust-level characteristics included size measured by number of deliveries, teaching status, maternity configuration drawing on the Birthplace in England86 typology of whether or not AMUs and FMUs are part of trust provision and staffing variables. These included staffing levels (FTE obstetric medical staff, midwives and maternity support staff per 100 maternities, FTE all staff per 100 maternities) and skill mix (doctor/midwife and midwife/support worker ratio).
Allocating women to risk categories using data contained within Hospital Episode Statistics records
Although no pregnancy can be considered entirely risk-free, by the same token, none are entirely ‘risky’. Women with recognised medical conditions and complications face greater risk of adverse outcomes and morbidities than those without. Each condition may have a spectrum of risk, and different associated adverse outcomes. Clinically, risks and plans are tailored to the individual. For the purposes of this research, some women are regarded as having ‘higher-risk’ pregnancies because of pre-existing medical conditions, a complicated previous obstetric history or conditions that develop during pregnancy. These women and their babies may have different outcomes from women regarded as at ‘lower risk’. Trusts may have differing proportions of higher-risk women and this could affect the outcomes of maternity services. A system of allocating women retrospectively to lower- and higher-risk status at the end of pregnancy, using information contained in their HES records, allowed us to make necessary adjustments to take this into account.
Methodology
The criteria for determining a higher-risk pregnancy
The Birthplace in England86 study compared safety of birth in different settings for women judged to be at low risk of complications at labour onset. For this study, women were classified as low risk if, immediately prior to the onset of labour, they were not known to have any of the medical conditions or situations listed in the NICE intrapartum care guideline80 that result in increased risk for the woman or baby during or shortly after labour, where care in an OU would be expected to reduce this risk. A few additional conditions not listed in the guidelines which might also be expected to confer increased risk were also used to determine the risk status.
The NICE guidelines themselves have two categories of higher risk: those women who have factors which indicate an increased risk of complications (e.g. sickle cell disease), and those women who require individual assessment when choosing place of birth (e.g. sickle cell trait). In this study, the NICE intrapartum care guideline80 was used to determine the risk status of women, which produced three categories of NICE risk status: low risk, requires individual assessment and at increased risk.
Matching higher-risk conditions with data items contained in Hospital Episode Statistics records
Conditions listed in both the NICE intrapartum care guidelines were matched by one of the project team (MD) to relevant four-alphanumeric digit ICD-10 codes, of which there are about 12,000. Initial matching largely used the existing grouping of ICD-10 codes by chapter and block (e.g. O30 multiple gestation, I10–I15 hypertensive diseases). This included a number of codes for which there were few or no women diagnosed with a particular condition. For certain conditions, other types of codes were matched, such as OPCS-4 or HES Data Dictionary data items, for example to identify breech presentation or multiple pregnancy.
Matching of codes was checked by another member of the project team who is an obstetrician (SB) and disagreements were resolved by consensus. In order to verify that all significant increased risk codes had been included, the numbers of women with a HES delivery record in 2010/11 with each of the four-digit ICD-10 codes were identified. Any codes remaining unallocated, with more than 10 women diagnosed with the condition, were individually checked by both MD and SB to see if they:
- had been missed, and should have been included as being on one of the lists categorising women as being at increased risk of complications
- had not specifically been included on the NICE list of conditions but nonetheless were considered to constitute a diagnosis which would place a women at increased risk of complications sufficient to recommend hospital delivery (‘additional codes’).
Each ICD-10 code and other relevant codes were allocated a number or letter determining the level of risk that the diagnosis would confer according to the NICE guideline, as follows in Table 6.
TABLE 6
Risk classification
For the NICE guideline list, the condition could either be pre-existing or have arisen during the current pregnancy so that it was present by the end of pregnancy or onset of labour (i.e. in time to advise a woman on obstetric referral or place of birth). Some conditions related to events arising in a previous pregnancy or delivery. For these, previous linked records were searched to determine if a woman had a factor increasing her risk of complications. For example, a woman who had had eclampsia following a previous birth could be identified using code O152 (eclampsia in the puerperium) in a previous delivery record. Pre-existing conditions not related to pregnancy (e.g. cardiac disease) were also searched for in any previous linked inpatient record.
It was decided to exclude induction of labour from the list of conditions that put women at increased risk according to NICE, as this is a procedure rather than a diagnosis. While a woman having an induction would have to give birth in an OU, her risk status at the end of pregnancy should be determined by the diagnosis of a condition rather than the decision to induce. This also enabled the distinction between documented medical and ‘social’ indications for induction.
Determining the risk status of women
Each woman’s HES records for the past 10 years were searched for codes that were tagged to be 4, 43, 2, 23.
If any of a woman’s records contained a 4 or 43, she was retrospectively categorised as ‘NICE increased risk’.
Any woman with no records containing a 4 or 43 type code, but containing a 2 or 23 code was categorised as needing ‘NICE individual assessment’.
If none of a woman’s records contained any codes labelled 4, 43, 2 or 23, she was categorised at ‘NICE low risk’.
Methodological limitations
Because of the large number of ICD-10 codes, not every code was allocated to a NICE risk category. However, all codes with a count of over 10 women were allocated, as were 22% of codes with a count of under 10 (but at least one) women. Because of this, the proportion of higher-risk women may have been underestimated. However, as conditions were coded initially using chapters and blocks of ICD-10 codes, it was unlikely that unallocated codes would relate to conditions on the NICE guideline list.
There was not always a direct match for conditions, and in these circumstances a clinical judgement was made. In some cases it was possible to obtain further information using other codes. For example, a large number of women were diagnosed with ‘unspecified’ asthma (J459, n = 36,000) and it was not possible to determine which of these were mild or at severe increased risk of complications. Those with verified severe asthma were identified by looking for records of women who had previously been hospitalised with asthma as their main diagnosis according to previous inpatient records (n = 4000); they were classified as ‘increased risk’ whereas the rest were classified as being at low risk of complications in the absence of any other risk factors. Nonetheless, this was still not a perfect match, as it was not possible to determine how many women had ‘required an increase in treatment’ as specified by the NICE guideline.
In some cases it was not possible to determine whether the diagnosis was antenatal, intrapartum or postpartum and a judgement had to be made, always erring on the side of caution. In some circumstances other codes could be used to inform that judgement. For example, where a woman was diagnosed with anaemia, this could be antepartum, putting her at increased risk of complications during the birth, or postpartum, which would not be relevant for allocation to risk category. Women diagnosed as anaemic who had had an intrapartum or postpartum haemorrhage were excluded from the increased risk category in the absence of any other risk factors.
Incomplete or inaccurate coding also affected the ability to assign a risk status to women. Trusts which were poor at coding would tend to show more lower-risk women than trusts which were good at coding.
Overall, there were a number of limitations to this method. However, initial testing suggested that it was a useful tool which distinguished well between the groups of women. However, because of unallocated codes which may have been clinically significant on an individual basis, it was considered to be of use only in retrospective analysis rather than in a clinical context prospectively categorising women.
Use in regression analyses
For the regression analyses, women categorised as ‘NICE increased risk’ or ‘NICE individual assessment’ were subsequently combined into a single category, ‘higher risk’. Women categorised here as ‘NICE low risk’ were subsequently referred to as ‘lower risk’.
Tables 7–10 are reproduced from the NICE intrapartum care guideline80 and show medical conditions and other factors that should be taken into consideration when planning place of birth.
TABLE 7
Medical conditions indicating increased risk suggesting planned birth at an OU
TABLE 10
Other factors indicating individual assessment when planning place of birth
TABLE 8
Other factors indicating increased risk suggesting planned birth at an OU
TABLE 9
Medical conditions indicating individual assessment when planning place of birth
Statistical methods
Multilevel logistic regression models, where mothers (deliveries) were nested within trust, were fitted to the 10 dichotomous maternity indicators using the Statistical Analysis System (SAS) version 9.3 (SAS Institute Inc., Cary, NC, USA) Generalised Mixed Models procedure (GLIMMIX). An unstructured variance–covariance matrix parameterised via the matrix’s Cholesky root was used. The root is squared to give the residual variance (σ2). We present the residual σ in findings. This parameterisation has good computational and statistical properties (it guarantees that the variance–covariance matrix is at least positive semidefinite), and is often preferred to an unstructured variance–covariance matrix. Other simper forms of the variance–covariance matrix are available but they require certain assumptions to be made, so a decision was taken not to impose a predetermined structure. The independent variables used in the model are shown in Table 11.
TABLE 11
Independent variables used in statistical models
Two models were fitted to the data using the mothers’ characteristics, sociodemographics and trust-level variables with either set 1 or set 2 of the staffing variables. Note we use the abbreviation FTE for full-time equivalent staff. Some of the categories do not appear in the statistical models because they were treated as missing data. Often these are related to the fact that the woman’s postcode was missing (e.g. for rural/urban classification, if a woman’s postcode was missing then she would not have a code for IMD).
The results from the 10 multilevel models were summarised in three tables (see Chapter 3, Tables 23–25).
Specifically for this study, symbols have been used to indicate the magnitude of each model effect using the relative chi-squared (chi-squared/degrees of freedom) value in a heuristic way to help summarise the results and provide a sense of which variables were important in predicting particular outcomes. The relative chi-squared values for all statistically significant effects were categorised as follows: minor (1–99), moderate (100–999), strong (1000–9999) or dominant (≥ 10,000).
The ↑ has been used to indicate a positive monotonic relationship (i.e. rises upwards in a linear or non-linear way) between a continuous predictor and the outcome, ↓ to indicate a negative monotonic relationship, * for non-monotonic relationships (e.g. increases with parity and then declines from parity 2 onwards) and ↔ for relationships involving categorical variables [e.g. ethnicity, Strategic Health Authority (SHA)]. So, for positive continuous relationships the symbols ↑, ↑↑, ↑↑↑, ↑↑↑↑ were used for minor, moderate, strong and dominant effects respectively. Where a variable was close to statistical significance (i.e. p > 0.05 but ≤ 0.10), this has been placed in parentheses in the report table (see Tables 23–25). Note the relative chi-squared value for each of the variables is presented in a separate table in the results section (see Table 22).
Sometimes outcomes were achieved more often as deprivation increased. This was represented by an upwards arrow ↑. Conversely, when they happened less often this was indicated by a downwards arrow ↓.
Independent variables were added in blocks starting with mother-level variables, then sociodemographics, trust-level and finally staffing variables. There were two different staffing variable models: the first used FTE per 100 maternities for the three staffing groups (doctors, midwives and support staff) and the second model used FTE (all) staff per 100 maternities alongside two ratio variables – doctor-to-midwife ratio and support worker-to-midwife ratio – to test the effect of substitution of one staff group with another.
The intercept was the only parameter allowed to vary between trusts, to ensure that clustering of mothers and babies within trusts was properly accounted for in the estimation of the parameter estimate standard errors (SEs). Funnel plots of the unadjusted and adjusted proportions (y-axis) against the number of known deliveries for each indicator (x-axis) were plotted. In Appendix 3, the funnel plots for the intercept-only model (unadjusted proportions) and the model that used set 1 of the staffing variables (adjusted proportions) are presented, noting that the plots for the two staffing models were very similar.
We did not attempt to adjust the funnel plot limits for overdispersion,89 which would happen if more trusts fell outside the control limits than would normally be expected. The main purpose of the plots was to judge what impact the model independent variables had upon the variation in the indicator rates across trusts rather than to attempt to identify outlier trusts, which is best achieved using adjusted funnel plots. Typically, model independent variables reduce the amount of variation between units of analysis (e.g. trusts) although it is possible for the variation to increase: for example, after the addition of the variables representing mothers’ characteristics into the model the variation increased for 8 of the 10 indicators.
Three sensitivity analyses were performed by fitting the statistical models to (1) trusts which only had a single OU (n = 50), (2) replacing the dichotomous clinical risk grouping with a three-category variable (NICE lower risk, individual assessment, NICE higher risk) and (3) testing the following interactions (multiplicative effects):
- parity × FTE doctors per 100 maternities
- parity × FTE midwives per 100 maternities
- parity × FTE support workers per 100 maternities
- clinical risk × FTE doctors per 100 maternities
- clinical risk × FTE midwives per 100 maternities
- clinical risk × FTE support workers per 100 maternities.
This was performed in order to find out if the effect of increasing/decreasing staffing levels upon outcomes was the same across different parities and clinical risk groups.
Costing analysis
The cost analysis attempted to model maternity services to identify the relationship between inputs and outputs, and more specifically for this study the relationships between the different labour inputs. In particular, we wanted to establish the extent that the different roles are substitutes or complements, i.e. are competing or aiding inputs.
Data sources
The unit of analysis was the trust. The output variable (Q) was the number of deliveries. The staffing (input) data were taken from the National Workforce Dataset, a reference data set of standardised definitions used consistently across the NHS and governed by Information Standards. Before 2010 the staffing data were provided to the NHS Information Centre (NHS IC) by trusts using an annual census survey. From 2010 onwards the staffing data were extracted directly from the Electronic Staff Records system into a central Data Warehouse and updated quarterly. Full data quality information is available on the NHS IC website, but it employs a Workforce Validation Engine to validate data submitted by trusts and to report back errors and quality scores to trusts. The continual updating of the Electronic Staff Records data and records and the time between our census points and the data extraction date should ensure good-quality data.
Descriptive statistics for the staffing data are reported in Tables 12 and 13. The medical staff were separated into two groups of ‘consultant’ and ‘other doctors’, with the latter group consisting of the remaining medical staff. It was not possible to determine the split between obstetric and gynaecological work. The non-medical staff were grouped into three distinct groups: ‘managers’, ‘midwives’ and ‘support staff’.
TABLE 12
Obstetric/gynaecological medical staffing in England 2010
TABLE 13
Non-medical staffing in England 2010
Reference cost data by NHS trust
NHS reference costs 2010/11 were available under Open Government Licence.76 The following data were extracted for each NHS trust and primary care trust (PCT) providing maternity care:
- number of deliveries, based on activity, less excess bed-days
- activity being undertaken, given by currency code and description
- antenatal costs, given by relevant currency codes
- delivery costs, given by delivery currency codes and excluding excess bed-days
- postnatal costs, given by relevant currency codes, including excess bed-days for delivery codes
- total maternity costs – sum of above costs.
Further details of these are given in Appendix 2.
Methodology to explore midwifery staffing levels, outcomes and the cost of providing maternity services for NHS trusts
Data on medical staffing were not detailed enough to ascertain the split between obstetric and gynaecological responsibilities in a trust. Therefore the costing analysis investigated the relationships between midwifery staffing levels, midwifery-related outcome measures and the cost of providing maternity services for NHS trusts in England and draws on an analysis for the RCM. For each NHS trust and PCT providing maternity services, the following were obtained:
- the average maternal age at time of delivery, determined using the HES data field MATAGE
- the percentage of nulliparous women delivering in the trust, determined using the data field NUMPREG and linkage with previous delivery records
- the average ranking of the overall IMD, determined using the data field IMD04RK
- the percentage of women who were deemed to be at increased risk of complications at onset of labour, determined using the risk allocation methodology described earlier.
These were used to create a demographic profile for each NHS trust.
Modelling techniques
The analysis was done using data aggregated at the NHS trust level, rather than at the level of the women’s delivery record. Although a multilevel model working down to the level of individual women’s outcomes would be possible, it was the intention of this investigation to keep the analysis simple and capture the essence of any relationships using trust-level data.
All the data sources were amalgamated in an Access database (Microsoft Access Professional Plus 2010, Microsoft Corporation, Redmond, WA, USA). The statistical package R [R version 3.01 (lm and glm packages), The R Foundation for Statistical Computing, Vienna, Austria] drew the relevant data for the analysis from this database. The standard R regression routines were used to perform the analysis. In order to establish relationships between midwifery staffing, expenditure and maternity outcomes, a number of modelling techniques were explored, including transformation of variables and the use of generalised linear models. These rarely provided much advantage over ordinary linear regression analysis, so whenever possible the simpler approach was preferred.
Reference costs
Throughout this analysis all the costs were converted to costs per delivery. To account for geographical variation in labour and capital costs between trusts, the costs were divided by the Market Forces Factor.
As costs were always positive, a log transformation was considered. However, in practice it was found that the costs were reasonably normally distributed and problems did not arise with predicted negative costs. Therefore, in the interests of simplicity the regression was performed on the non-transformed data.
Midwives
The number of FTE registered midwives per delivery was used as a variable. This is the inverse of the more usually quoted midwife ratio (deliveries per midwife) but was used in preference as it was more in accord with linear regression analysis.
Operative delivery rates, normal birth rates
These outcomes were constrained to be in the range 0% to 100%, so a logistic transformation was used to ensure that the predicted values fell into this range. Standard linear regression techniques were used on the transformed values.
Care Quality Commission scores
These scores (see Appendix 2 for more detail) were constrained to take values between 0 and 10 so a logistic transformation was used to force the constraint on the regression model.
Written complaints
These data had a Poisson distribution with many zero entries. The most appropriate model was a Poisson Generalised Linear model.
Exclusion of data for quality and bias
A number of trusts were excluded from the analysis:
- Two PCTs and two NHS trusts which provided only midwifery services. These have different patterns of care and costs from trusts providing obstetric services.
- Seven NHS trusts which had atypical or inconsistent data, or data which were overly influential in regression analyses. Inclusion of these trusts could lead to spurious conclusions about relationships between variables.
As a result, analyses were performed using data from 134 English NHS trusts and 2 PCTs.
Analysis
Initial analyses were undertaken to establish (1) the validity of the number of deliveries included in the reference cost data and (2) the relationship between trust profiles and outcomes. A number of hypotheses were then tested to determine the relationship between midwifery staffing, costs of maternity care and outcomes.
Economic modelling methodology
In economics, a production function describes the mechanism for converting a vector of inputs (x) into output (y). After selecting the appropriate functional form, econometric estimation of the function’s parameters allows the output elasticities to be calculated and returns to scale to be found. In the absence of data on input prices at the maternity services level of analysis, we adopted a production (i.e. quantity) function approach. Many health-care studies using production functions (as opposed to cost functions) have adopted Reinhardt’s90 specification of the production function, which was the first to include multiple labour inputs (registered nurses, technicians, administrative staff and doctors). However, this function assumes all inputs to be substitutes (solely because of the absence of cross-products). The advance in production function analysis of the 1970s gave rise to two flexible econometric specifications that allow us to relax this overly strict assumption. Berndt and Christensen91 introduced the transcendental-logarithmic (translog) production function and Diewert92 introduced the generalised linear production function (also known as the Allen, McFadden and Samuelson production function).
Using either of these functions would have allowed us to estimate the relationship between the labour inputs because the regression coefficient on the cross-products (interaction effects) can be simply used to calculate the Hicks64 elasticity of complementarity (see Sato and Koizumi93 or Syrquin and Hollender94 for an explanation). However, an advantage of the Diewert92 specification is that it allows zero quantities for some inputs, which may be a more realistic assumption when labour inputs are disaggregated, as they are in our study. This modelling enabled us to examine the output contribution of the different staff inputs (output elasticities) and their influence upon the productivity of other staff inputs (i.e. whether they are complements or substitutes). With these results available, we were able to investigate the input substitution possibilities available to hospitals under different scenarios.
Following Diewert92 we adopted a generalised linear production function defined as:
where in our study K = 4, X = {consultants, other doctors, midwives and support staff} and Y = Q, corresponding to the number of deliveries. This model was estimated using ordinary least squares in Stata (Stata 12, StataCorp LP, College Station).
To examine the q-complementarity (and therefore to answer the question relating to skill mix), we calculated the Hicks elasticity of complementarity,64 ηH defined for any two staffing inputs i, j (i, ≠ j):
where
The elasticities were computed at the means and the SEs using the delta method.
- Research questions
- Study design
- Patient and public involvement
- Data sources
- Data storage, governance and ethics
- Data cleaning and quality
- Study size and bias
- Derivation of process and outcome indicators
- Indicators used in analysis
- Independent variables
- Statistical methods
- Costing analysis
- Methodology to explore midwifery staffing levels, outcomes and the cost of providing maternity services for NHS trusts
- Exclusion of data for quality and bias
- Economic modelling methodology
- Design and methods - The efficient use of the maternity workforce and the implic...Design and methods - The efficient use of the maternity workforce and the implications for safety and quality in maternity care: a population-based, cross-sectional study
Your browsing activity is empty.
Activity recording is turned off.
See more...