NCBI Bookshelf. A service of the National Library of Medicine, National Institutes of Health.
Gliklich RE, Dreyer NA, Leavy MB, editors. Registries for Evaluating Patient Outcomes: A User's Guide [Internet]. 3rd edition. Rockville (MD): Agency for Healthcare Research and Quality (US); 2014 Apr.
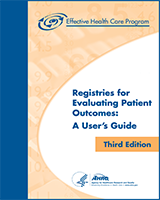
Registries for Evaluating Patient Outcomes: A User's Guide [Internet]. 3rd edition.
Show details1. Introduction
This chapter provides a review and discussion of the analytic challenges faced by studies that use existing administrative databases and patient registries. We provide additional detail and examples of the issues raised in Chapter 13. While that chapter focused on the analysis of registry data in accordance with the registry's purpose and objectives, this chapter tackles the issues and opportunities that arise when using registry data, often in combination with other data sources, to investigate hypotheses or questions that are secondary to the original reason for data collection. Case Examples 42 and 43 provide real-world examples of the analysis of linked registry data sets.
The use of administrative databases and medical registries to provide data for epidemiologic research has blossomed in the last decade,1 fulfilling prophecies that date to the mid-1970s.2 Studies that use data collected for a primary purpose other than research (e.g., administrative databases) or collected for research purposes but used to support secondary studies (e.g., patient registries) have contributed substantial information to the understanding of the incidence, prevalence, outcomes, and other descriptive characteristics of many diseases. For simplicity, this chapter will refer to all such studies as “retrospective database studies.” Retrospective database studies have also contributed information to the understanding of disease etiology, patterns of treatment and disparities in care, adverse effects and late events associated with disease treatments, and the comparative effectiveness of some therapies. Despite these achievements, retrospective database studies sometimes receive criticism because of their potential to yield invalid results.1, 3 Weiss, for example, points out the potential for retrospective database studies to ascertain exposures, outcomes, and potential confounding variables with poor accuracy, or to provide an invalid reference group (the unexposed in a cohort design or controls in a case-control design). Ray1 provides a table of potential pitfalls in “automated database studies,” which includes a similar warning about inaccurate measurement of exposure, outcomes, and covariates, the potential for unmeasured confounding and missing data, and the potential to include immortal person-time.
While these examples and lists of pitfalls provide valuable guidance, none of them is unique to retrospective database studies. Nonrandomized studies of all designs are susceptible to systematic errors arising from mismeasurement of analytic variables,4 unmeasured confounding,5 and poor choice of reference group.6, 7 Also, immortal person-time bias is not limited to retrospective database studies; it has even plagued a secondary analysis of data gathered in a randomized trial.8, 9 Taking a different approach, this chapter begins with a review of the fundamentals of sound study design and analysis. These fundamentals apply to epidemiologic research nested in any study population, but the chapter will focus on and illustrate the topics with examples that use retrospective database studies. In the subsequent sections, important considerations in retrospective database studies will be discussed, with the recognition that studies nested in other study populations may have the same considerations, but perhaps less often or to a lesser degree than retrospective database studies.
2. Fundamentals of Design and Analysis in Retrospective Database Research
2.1. Statement of Objective
Most productive epidemiologic research begins with a clear statement of objective. This objective might be descriptive; for example, to measure the incidence of a particular disease in some population, to characterize the patterns or costs of treatment for a particular disease in some population, or to measure the occurrence of outcomes among patients with a particular disease.
The objective might also involve a comparison; for example, to compare the incidence of a particular disease in two or more subgroups defined by common characteristics (e.g., etiologic research), to compare the cost or quality of care for a particular disease in two or more subgroups (e.g., health services research or disparities research), or to compare the rate of outcomes among two or more subgroups of patients (often defined by different types or levels of treatment) with a particular disease (e.g., clinical research). In all cases, the overarching objective is to obtain an accurate (valid and precise) and generalizable estimate of the frequency of an outcome's occurrence, or its relative frequency compared across groups.10 A valid estimate is one that can be interpreted with little influence by systematic error. A precise estimate is one that can be interpreted with little influence by random error. A generalizable estimate is one that provides information pertinent to the target population, the population for which the study's information provides a basis for potential action, such as a public health or medical intervention. Often times the objective will be accompanied by a specific hypothesis (see Chapter 13), although that is less important than the statement of objective.
2.2. Selection of a Study Population
Once the study's objectives have been stated, the next step in the research plan is to select a study population. Selection of a study population requires identifying potential participants in time and place, including inclusion/exclusion (admissibility) criteria related to the study's objectives and feasibility. Admissibility criteria related to the study's objectives include focusing on a clinically relevant study population of individuals in whom sufficient events will occur to provide adequate precision for the estimates of disease frequency, and in whom the exposure categories will occur with sufficient frequency to provide adequate precision for the estimates of association. These criteria are also used to exclude people with characteristics that can introduce significant bias into the estimates of disease frequency or estimates of association, and that cannot be controlled easily or adequately in the analysis. Precision and validity criteria for admissibility pertain to all studies, regardless of whether they are nested in a health database.
Admissibility criteria related to feasibility center on access to the data. Many ongoing cohort studies have established procedures for data sharing. Similarly, most publicly funded health databases have established procedures for data access. Investigators must ordinarily provide a statement of the study's objective, a protocol for data collection from the database and for data analysis, a list of individuals who will have access to the data, and a study timeline. Some databases charge a fee for data access, although many do not.
An advantage of retrospective database studies is the potential to study associations between rare exposures and rare outcomes in a population large enough to provide sufficient precision, with nearly complete followup, and with few exclusion criteria pertaining to age, comorbidity, or other factors that sometimes limit participation in clinical trials.11, 12 For example, surveillance databases that monitor adverse events potentially associated with pharmaceuticals identified signals suggesting that use of HMG CoA-reductase inhibitors (statins) might increase the risk of amyotrophic lateral sclerosis (ALS).13, 14 The only available epidemiologic evidence came from pooling 41 randomized trials, in which ten ALS cases occurred among 56,352 individuals assigned to placebo and nine ALS cases occurred among 64,602 individuals assigned to the statins arm.14 Using Danish databases, a case-control study identified 556 cases of ALS or other motor neuron syndromes and 5,560 population controls.15 The odds ratio associating disease occurrence with statins use was 0.96 (95% CI, 0.73 to 1.28), thereby rapidly and cost-efficiently providing evidence to counter the drug-monitoring studies and with far greater precision than provided by the pooled clinical trials.
Selection of a study population inevitably involves balancing accuracy and generalizability concerns, as well as cost and feasibility considerations. For example, restriction is one of the most effective strategies for control of confounding through study design.16 If one is concerned about confounding by sex, a simple and effective strategy to control that confounding is to restrict the study population to a single sex. However, such restriction reduces the study's precision by decreasing the sample size, and may also reduce the generalizability of the results (only applicable to half of the target population). An alternative would be to include both sexes and to stratify the analysis by sex. While this approach would improve the generalizability of the results, and allow an evaluation of confounding, the precision of the estimated association would be reduced, and perhaps substantially reduced, if the estimate of effect in men was substantially different from the estimate of effect in women. In this circumstance, the study becomes effectively two studies.
2.3. Definition of Analytic Variables
The protocol for an epidemiological study should provide a clear, unambiguous definition of the outcome being studied, a description of how it will be measured, and a discussion of the accuracy of that measurement. When sensitivity of a dichotomous disease classification is nondifferential and independent of any errors in classification of exposure categories, and there are expected to be few false positives (near perfect specificity), there will usually be little bias of a ratio measure of association.4 This exception to the rule that “nondifferential misclassification biases towards the null” has important design implications. It suggests that retrospective database studies should be designed to optimize specificity; in fact, to ideally make the specificity perfect so there will be no false positives. Such a design might require more stringent criteria applied to the outcome definition than are ordinarily applied in a clinical setting, and therefore more stringent than might be found in a disease registry. For example, the estimated prevalence of dementia in a cohort of men and women aged 65 years or older varied by a factor of 10 depending on the diagnostic criteria that were applied.17 Strategies to reduce inclusion of false-positive cases can include requiring evidence in the patient record of medical procedures (e.g., cholecystectomy for gallstone disease or podiatry examination for diabetes) or interventions (e.g., insulin or glucose lowering medications for diabetes) that provide greater confidence in the validity of the case-finding definition.18 Such an approach often results in fewer included cases and reduced precision, but improved validity.19
If the study objective is to compare the frequency of outcome across subgroups, then the protocol should provide a definition of the exposure contrast(s). It is critical that both the index condition (i.e., the “exposed” or “treated” group) and the reference condition (i.e., the “unexposed” or “untreated/placebo” group) are well defined.6, 20 One frequent shortcoming of epidemiologic research is to compare the occurrence of disease in an index group with the occurrence of disease in all others who do not satisfy the index group definition. Studies of this design are easily constructed with retrospective database research, because of the abundance of participants who do not meet the index group definition. This “all others” reference group is therefore usually a poorly defined mixture of individuals.21 For example, if one uses a pharmaceutical registry to compare the incidence of a disease in statins users with the incidence of disease in those who do not use statins, the reference group of nonusers will contain individuals with indications for statin use but who have not been prescribed statins, as well as individuals without indications for statins use. Nonusers also differ from users in the frequency of contact with medical providers, which raises the potential for differential accuracy of ascertainment of health outcomes. It is therefore preferable to first ensure that the reference group of nonusers contains individuals who have indications for use of the treatment,18 and who, if possible, are receiving alternative therapies for the same indication.22 If one has a biologic basis to separate statins into categories, such as hydrophilic and hydrophobic statins, then a comparison of users of hydrophilic statins with users of hydrophobic statins would often be more valid. With these definitions, only individuals with indications for statins, and treated with statins, are included in the analysis, thereby reducing the potential for confounding by indication and differential followup.23
Finally, considerable attention should be given to identifying and accurately measuring potential confounders and effect modifiers.4, 24 The opportunity to examine important etiologic questions with considerable precision has expanded significantly with the availability of large databases, but systematic error due to confounding by unmeasured or poorly measured confounders remains a central concern. Fortunately, databases generally capture inpatient and outpatient clinical events and medication use that can characterize comorbidities and health care resource utilization, which can aid in the control of confounding. As discussed further below, information on behavioral and lifestyle factors (e.g., cigarette smoking, alcohol use, diet) is infrequently captured or is poorly measured in many databases. Thus, retrospective database researchers should carefully consider the available information on confounders before initiating studies. When data on critical confounders cannot be obtained in a database, and cannot be obtained by linking to another data source, an alternative data set might be better suited to accomplish the study's objectives. Alternatively, in the presence of unmeasured confounding, researchers can use bias analysis5, 25 to assess the potential impact of residual confounding on their observed findings.26, 27
2.4. Validation Substudies
The goal of quality study design and analysis is to reduce the amount of error in an estimate of association. With this goal in mind, investigators have an obligation to quantify how far they are from this goal, and bias analysis is one method by which to achieve this goal.5, 25 Bias analysis methods require data to inform the bias model, and these data are obtained from internal or external validation substudies. Retrospective database research is often amenable to collection of internal validation data, for example by medical record review. In addition, many databases have internal protocols that constantly validate at least some aspects of the data. The validation data generated by these protocols can provide an initial indication of the data quality. To facilitate data collection for study-specific internal validation studies, investigators should consider the important threats to the validity of their research while designing their study, and should allocate project resources accordingly. This consideration should immediately suggest the corresponding bias analyses, which will then inform the data collection required to complete the bias modeling.
For example, in the study of statin use related to ALS and neurodegenerative diseases described above,15 the ICD-10 code used to identify cases (G12.2) corresponded to diagnoses of ALS or other motor neuron syndromes. The investigators therefore selected a random sample of 25 individuals from among all those who satisfied the case definition, and a clinician investigator reviewed their discharge summaries. The proportion of these 25 who did not have ALS (32 percent) was used to inform a bias analysis to model the impact of these false-positive ALS diagnoses. Assuming a valid bias model, the bias analysis results showed that the null association was unlikely to result from the nondifferential misclassification of other diseases as ALS.
In this example, there was no effort to validate that non-cases of ALS were truly free of the disease. Non-cases are seldom validated, because false-negative cases, especially of rare diseases, occur very rarely. Furthermore, validating the absence of disease often requires a study-supported medical examination of the non-case patients, an expensive, time-consuming, and invasive procedure. Prevalent diseases with a lengthy preclinical period and relatively simple diagnostic tests, such as diabetes, are more amenable to validation of non-cases. The ALS example also illustrates that an internal validation study requires protocol planning and allocation of study resources to collect the validation data. A protocol should be written that specifies how participants in the validation sample will be selected from the study population. Participation in the validation substudy might require informed consent to allow medical record review, whereas the database data itself might be available without individual informed consent. These aspects should be resolved in the planning stage, and the analytic plan should include a section devoted to bias modeling and analysis.5
3. Important Considerations
Once an investigator decides to pursue a research objective using a retrospective database study, there are a number of important considerations to evaluate before undertaking the study. These considerations mostly pertain to the quality and completeness of the database,28, 29 and especially to the potential for systematic errors in the database to affect the validity of the study's result.
3.1. Structural Framework for Data Collection
Health databases collect data for various primary purposes30 and can be categorized as follows: (1) data collected for the purpose of reimbursing health care providers; (2) data collected for the purpose of monitoring care provided to beneficiaries of an integrated health care system; (3) data collected for the purpose of surveillance regarding a particular disease or disease category; (4) data collected for the purpose of surveillance for individuals with a specific exposure; and (5) data collected on individuals with a single admission-defining disease or medical procedure. Each type has strengths and limitations (presented in Table 18–1) to consider when evaluating the database for use in studies.
Table 18–1
Types of databases used for retrospective database studies, and their typical advantages and disadvantages.
Databases that collect information for reimbursement (e.g., Medicare, Medicaid, or Ingenix), which are sometimes called “claims” or “administrative” databases, are quite useful for understanding health care costs and can provide important surveillance information on clinical practices and outcomes. However, they may be susceptible to systematic errors if data entries are manipulated by the data generators to affect (likely increase) their reimbursement. For instance, certain clinical conditions with high reimbursement rates may be preferentially reported on claims for patients who have those conditions but who present in the hospital or outpatient setting with other clinical issues, particularly if the presenting conditions are reimbursed at lower rates. The accuracy of some claims data sets have been questioned for diagnoses and procedures including dialysis,31 weight management,32 neutropenia,33 heart failure,34 diabetes,35 and functional outcomes after prostatectomy,36 as examples. On the other hand, the accuracy of registered diagnoses can be quite good.37 The accuracy of the claims data for its intended objective should therefore be considered, and preferably estimated quantitatively by an internal validation substudy.38, 39 Alternatively, estimates of the data's accuracy may be available from an analogous study population from the same or a similar claims data set; an example is an external validation study. Claims data often lack important information on laboratory parameters, diagnostic test results, and behavioral and lifestyle characteristics, which may limit their utility for research in some topic areas.
The second type of database collects information on the health care provided to beneficiaries within an integrated health care system. This system can be a health insurer (e.g., Kaiser Permanente), a benefits program provided to selected individuals (e.g., Veteran's Health Administration), or a national health care system (e.g., the United Kingdom's Clinical Practice Research Database40). These databases typically use an integrated electronic health records system to capture health care information directly from physicians' offices, hospitals, pharmacies, and other sites where care is provided (e.g., infusion centers, surgical centers). The granularity and quality of data captured in these databases is quite good and includes demographic and clinical characteristics, medication use, major clinical events including death, and importantly, results of diagnostic tests and laboratory assays. As with many epidemiological studies, some databases are limited in their geographic coverage and in the demographic characteristics of their patient populations. This lack of representativeness may affect the generalizability of results from studies nested in them.
The intended purpose of a third set of databases is surveillance of the incidence and outcomes related to a particular disease or disease category. These databases, or surveillance registries, often pertain to infectious diseases, cancer, and end-stage renal disease (ESRD). Surveillance for infectious diseases sometimes recognizes that only a proportion of cases will be reported, but assumes that the sensitivity and specificity of reporting remain constant over time, so that changes in the relative frequency of reported incidence provides a signal regarding the true incidence in the population. Thus, although the data quality is high, the completeness may be low. In contrast, both the data quality and completeness in most cancer registries are quite high, and the motivation for manipulation to influence reimbursement does not exist because the registry data are not used for that purpose. For example, the U.S. Cancer Surveillance, Epidemiology, and End Results (SEER) registry has a history of quality control and improvement dating to its inception in 1973 and has been linked to the Medicare administrative database to provide data on cancer treatments and outcomes. In the United States and some other countries, patients with ESRD (patients receiving chronic dialysis or who are transplant recipients) are guaranteed coverage of all dialysis services including medications, procedures, and hospitalizations. These benefits extend throughout the patient's life and require significant resources. Consequently such countries have established surveillance programs like the United States Renal Data System to monitor the health care provided to these patients and the costs associated with their health care.
The fourth type of database collects data on patients with a common exposure, and is commonly used as part of a postmarketing pharmacovigilance program related to a biologic or pharmaceutical product or a medical device. This type of database is typically designed to monitor the incidence of adverse events related to the exposure. These databases are often patient registries.
A last type of database is a clinical patient registry of individuals with a single admission-defining disease or medical procedure. In fact, the first known health-related registry was the Leprosy Registry in Norway, initiated in 1856. In keeping with this history, many of the current clinical registries are found in Scandinavia. For example, the Danish government supports clinical databases used for quality assurance and research (e.g., breast cancer, colorectal cancer, hip arthroplasty, and rheumatologic diseases), as well as disease registries (e.g., the multiple sclerosis registry) used for monitoring and research.41 In fact, a central objective of disease-specific registries may be to provide an infrastructure for clinical trials pertaining to treatments for the disease. The main advantage of these registries and databases is the quality of data on disease characteristics, received treatments, and outcomes related to the disease. The main disadvantage is that they are difficult to use for studies of the etiology of the disease that initiates membership in the registry, since the registry includes only individuals with the disease.
3.2. Changes in Coding Conventions Over Time
A common problem with retrospective database research is the impact of changes in coding conventions over the lifetime of the database. These changes can take the form of diagnostic drift,42 changes in discharge coding schemes, changes in the definition of grading of disease severity, or even variations in the medications on formulary in one region but not others at different points in time. For example, the Danish National Registry of Patients (DNRP) is a database of patient contacts at Danish hospitals. From 1977 to 1993, discharge diagnoses were coded according to ICD-8, and from 1994 forward discharge diagnoses were coded according to ICD-10. ICD-10 included a specific code for chronic obstructive pulmonary disease (J44), whereas ICD-8 did not [ICD-8 496 (COPD not otherwise specified) did not appear in the DNRP]. In addition, from 1977 to 1994 the DNRP registered discharge diagnoses for only inpatient admissions, but from 1995 forward discharge diagnoses from outpatient admissions and emergency room contacts were also registered. COPD patients seen in outpatient settings before 1995 were therefore not registered; this excluded patients who likely had less severe COPD on average. The change in ICD coding convention in 1994 and the exclusion of outpatient admissions before 1995 presented a barrier to estimating the time trend for incidence of all admissions for COPD in any period that overlapped these two changes to the DNRP.43
The General Practice Research Database (GPRD) was a medical records database capturing information on approximately 5 percent of patients in the United Kingdom44 (as of March 2012, the GPRD became the Clinical Practice Research Database). Information was directly entered into the database by general practitioners trained in standardized data entry. When the GPRD was initiated in 1987, diagnoses were recorded using Oxford Medical Information Systems (OXMIS) codes, which were similar to ICD-9 codes. In 1995, the GPRD adopted the Read coding system, a more detailed and comprehensive system that groups and defines illnesses using a hierarchical system. Without knowledge of this shift in coding and how to align codes for specific conditions across the different coding schemes, studies using multiple years of data could produce spurious findings.
3.3. Other Data Quality Considerations
3.3.1. Selection of Registered Population
An important advantage of some retrospective database research is that it is population based, and therefore provides good representativeness for the target population. However, not all retrospective database research provides this advantage. For example, the U.S. Veterans Health Administration databases provide an important resource retrospective database research. A recent analysis of individuals receiving Veterans Health Administration services in fiscal years 2004 and 2005 reported a mortality rate due to accidental poisoning of about 20 per 100,000 person-years.45 However, this database includes only U.S. military veterans, a selected subpopulation of the U.S. population, with a higher proportion of men than the overall population, and probably an unrepresentative proportion of other characteristics as well. The rate of accidental poisonings was thus almost twice that of the U.S. general population, after adjusting for differences in the age and sex distributions. Similarly, the Medicare administrative database provides an important resource for retrospective database research, including its links with the SEER cancer registry mentioned above. However, the former includes only Medicare recipients, almost all of whom are 65 years of age or older, and many variables are unavailable for members of this population who participate in managed health care plans. Whether the lack of representativeness in these two examples, and others like them, affects inference made to the target population depends on the particular topic.
3.3.2. Probability of Registration in Relation to Disease Severity
A second type of incomplete data arises at the level of registered individuals, rather than afflicting the whole database. In an earlier example, cases of COPD were registered in the Danish National Registry of Patients in reference to ICD-8 before 1994 and in reference to ICD-10 thereafter. Only inpatient diagnoses of COPD were registered in the DNRP before 1995; inpatient, outpatient, and emergency department contacts were registered thereafter. At no time has the DNRP registered COPD cases diagnosed and treated only by a Danish General Practitioner. The least severe cases of this progressive disease are, therefore, missing from the DNRP throughout its history,46 and patients treated as outpatients are missing from the DNRP before 1995. Similar problems occur with hospital databases of other progressive diseases such as diabetes, Alzheimer's disease, or Parkinson's disease. Patients treated by their general practitioners will often eventually appear in the hospital database with the proper discharge diagnosis, since these progressive diseases become more severe over time. The less severe cases do not appear in hospital discharge databases, and their absence presents a barrier to studies of population-based incidence or prevalence, as well as to the accurate determination of whether exposure to a potential etiologic agent preceded the disease diagnosis,47 since neither the date of first diagnosis by the general practitioner nor the date of symptom onset is recorded.
Databases often lack accurate measurements of lifestyle and behavioral factors, such as tobacco use, alcohol drinking, exercise habits, and diet. Some databases can provide proxy measurements of these behavioral factors. For example, poor lung function or diagnosis of COPD is a proxy marker for tobacco smoking history, alcohol-related diseases such as cirrhosis or prescriptions for disulfiram can be used as proxy markers for alcohol abuse, and medically diagnosed obesity may be a proxy marker for poor diet and lack of exercise. None of these proxies provides a reliable measure of the actual concept, however.
Other methods of estimation may add information. For diseases that can be identified by use of specific medications, one could compare the incidence of that medication use with the incidence in the hospitalization database to estimate the proportion of total cases that are registered. Comparison of the date of onset of the medication use with the date of first outpatient or inpatient diagnosis of the disease would provide an estimate of the typical delay between diagnosis by a general practitioner and progression of the disease to a severity level treated in the outpatient or inpatient setting.
3.3.3. Missing Data
Item nonresponse and missing data at the level of an individual record are often less of a problem for retrospective database research than for comparable cohort studies. Cohort studies that rely on participation by study subjects are subject to attrition and nonresponse. Attrition occurs when participants early in the cohort's followup stop replying to regularly mailed surveys, telephone interviews, or emailed data collection instruments. These losses to followup are sometimes related to exposure characteristics and health outcomes, which introduces a form of selection bias,48 even if subjects rejoin the study at a later time.49 Item nonresponse occurs when a participant answers a survey or interview, but does not provide a response for one or more of the data fields. Item nonresponse can also occur when data on an exposure or outcome are collected by other methods, such as when a biospecimen is unavailable to provide tissue for an assay of a genetic or protein biomarker. This missing data may also be related to exposure and disease characteristics, and can introduce a bias, although reliable methods have been developed to resolve bias from item non-response (missing data) in many circumstances.50 Likewise, inverse probability weighting can sometimes be used to address selection bias and loss to followup,51 although it has seldom been implemented to date.
Retrospective database research ordinarily uses data collected for a primary purpose other than research. Item nonresponse (one form of missing data) is also often less of a concern, since the databases often have inherent quality control methods to assure high data completeness. Other forms of missing data can, however, plague retrospective database research in other ways. For example, left truncation is sometimes an important problem in retrospective database research, and is basically a missing data problem (although it can also be conceptualized as an information bias).52
Left truncation occurs when information required to characterize prevalent exposures, covariates, or diseases precedes the establishment of the database. With left truncation, unexposed individuals (e.g., nonusers of a medication) may have been users before the database was established, and apparently incident cases of a disease may have been diagnosed before the database was established, which would make them prevalent cases. Furthermore, covariate information collected at the inception of the database might have been affected by the medical history before the database was established. For example, blood pressure measured soon after a database began might be affected by blood pressure medications prescribed before the database began. Characterizing this initial measurement as baseline (i.e., preceding the first recorded prescription for blood pressure medications) would fail to account for the effect of the prevalent prescription for blood pressure medications, which was prescribed during the left truncation period.
As a second example, in a study of the association between metformin use and the occurrence of breast cancer, the prescription database used to ascertain use of metformin among diabetic patients was not established until after the medication came to market.53 Data on use of metformin were therefore left-truncated, which can be conceptualized as a missing data problem for time-varying characterization of metformin use in the years preceding the database. (See Ibrahim and colleagues54 for a review of methods to model time-varying data.) Alternatively, this distortion can be conceptualized as the more general problem of having poor sensitivity of ever/never classification of metformin use.
Left truncation is a common problem whenever prevalent conditions may have preceded the establishment of a database. For example, many etiologic epidemiology and clinical epidemiology studies exclude prevalent cases of the outcome at the inception of followup. However, some cases of disease may have occurred before followup began and even before the database's inception, and these prevalent cases would be impossible to identify unless they also appeared in the database after its inception but before the followup time began. For many prevalent diseases with good survival, contact with the medical system is frequent, so most prevalent cases should be identifiable after the database is 5 to 10 years old. However, the potential for left truncation to mask some prevalent cases of the disease under study should be considered as a question specific to the research topic.
Right censoring can also occur in retrospective database research. For example, studies that use birth registries to ascertain congenital defects usually fail to detect defects that are diagnosed later in life, such as congenital heart anomalies. These defects are usually never recorded in the birth registry, so must be ascertained by some other method. Without such continued followup, the measurement of the outcome is right censored at the date of last followup by the birth registry.
Left truncation and right censoring are specific examples of the more general problem of data gaps. Data gaps occur when databases pertain only to a particular subgroup of the larger population, and membership in that subgroup is dynamic. Examples include individuals covered by Medicaid and members enrolled in managed care plans. In both examples, the databases pertain to participants in a health insurance program, and membership in those programs can change frequently. Data are collected only while the participants are members. If membership is lost and restored again later, there will be a data gap. Importantly, membership in these plans might be related to other characteristics that affect health, such as socioeconomic status or employment.55 Similar problems can arise when there are gaps in residency and the database is based on national health care data, or when individuals have health insurance from more than one source.
Data gaps in retrospective database research can also arise when medications are dispensed in the hospital, since many databases do not capture in-hospital medication use, leading to a form of information bias. In drug safety studies examining mortality risk related to the use of a particular medication, missing in-hospital medication use can result in spurious estimates of treatment effects.56 This bias was illustrated in a case-control study examining mortality risk related to inhaled corticosteroid use from the Saskatchewan, Canada, database. Analyses that failed to account for missed corticosteroid use during hospitalization events preceding death or the matched date for controls showed a beneficial effect (RR=0.6; 95% CI, 0.5 to 0.73). The RR estimates changed markedly once the missing in-hospital corticosteroid use was included (RR=0.93; 95% CI, 0.76 to 1.14 and RR=1.35; 95% CI, 1.14 to 1.60).56 This bias has also been observed in studies of injectable medications in dialysis patients where hospitalization events preceding death resulted in spuriously low effect estimates.57
3.4. Confounding by Indication
Confounding by indication may occur in nonrandomized epidemiologic research that compares two treatments (or treatment with no treatment).58 In the absence of randomization, the indications for selecting one treatment in preference to another (or in preference to no treatment) are often also related to the outcome meant to be achieved or prevented by the treatment.59 For example, randomized trials in younger breast cancer patients have shown that chemotherapy prevents breast cancer recurrence.60 However, in a nonrandomized study of older breast cancer patients, those who received chemotherapy had a higher rate of recurrence than those who did not, probably because chemotherapy was offered only to the women with the most aggressive cancers.61 This example is a classic illustration of confounding by indication. Importantly, this study collected complete detailed data on every prognostic marker of recurrence and all of the other breast cancer treatments, yet adjustment for this detailed suite of variables did not resolve the confounding by indication, even using more advanced methods.23
Retrospective database research is as susceptible to confounding by indication as any other design. However, strategies to reduce the strength of this confounding have been proposed21 and may be most successful when used in the large study populations often achievable only in databases.62 Explained here is a special class of confounding by indication, which might arise especially in retrospective database research: time-dependent confounding by indication generated by dynamic dosing.63 Dynamic dosing refers to the clinical situation in which a medication's dose is titrated (increased or decreased) in response to a changing biomarker or clinical measurement on which the medication acts (i.e., a clinical intermediate).63 Examples include diabetes medications titrated in reaction to hemoglobin A1c (HbA1c) measurements, erythropoiesis stimulating agents (ESAs) titrated in reaction to hemoglobin levels, blood pressure medications titrated in reaction to systolic and diastolic blood pressure values, and antiretroviral therapy titrated in reaction to CD4 counts. The clinical intermediate is therefore both a consequence of therapy and a predictor of future therapy. Time-dependent confounding arises when the clinical intermediate is also a prognostic indicator.64 For example, hemoglobin concentration is a time-dependent confounder of the effect of ESA therapy on survival because it is a risk factor for mortality, it predicts future ESA dose, and past ESA therapy predicts future hemoglobin concentration. Dynamic dosing therefore introduces time-dependent confounding of the treatment's association with outcomes in the presence of this structure of confounding by indication.63
It is important to recognize that the structure requires the clinical intermediate to be both a causal intermediate and a confounder. If it is only a confounder, such as baseline comorbidity or time-dependent comorbidity, the confounding can be addressed by conventional analytic methods. However, when the causal structure indicates that the clinical intermediate is both a causal intermediate and a confounder, inverse probability of treatment weighting (IPTW) with marginal structural models (MSMs) has been proposed as one method for valid adjustment.65 Pharmacoepidemiological studies that have used MSMs to address time-dependent confounding have shown significant improvements in confounding control relative to traditional time-dependent analysis.66-68 In a study of the effect of highly active antiretroviral therapy (HAART) on time to AIDS, the hazard ratio using standard time-dependent Cox regression to adjust for time-varying covariates such as CD4 count and HIV RNA level was 0.81 (95% CI, 0.61 to 1.07). Using an MSM, this effect was strengthened substantially (HR=0.54, 95% CI, 0.38 to 0.78), providing stronger evidence of the benefit of HAART.66 Studies examining the effect of titrated ESA doses on mortality risk in dialysis patients that have used MSMs have found hazard ratio estimates at or below the null,67, 68 whereas results from traditional models found substantially elevated hazard ratio estimates.67
3.5. Precision Considerations When Standard Errors Are Small (Over-Powered)
The large size of the study population that can often be included in retrospective database study is both a strength and a limitation. The sample size allows adjustment for multiple potential confounders with little potential for over-fitting or sparse data bias,69 and allows design features such as comparisons of different treatments for the same indication (comparative effectiveness research) to reduce the potential for confounding by indication.21 Nonetheless, systematic errors remain a possibility, and these systematic errors dominate the uncertainty when estimates of association are measured with high precision as a consequence of a large sample size.70 When confidence intervals are narrow, systematic errors remain, and/or inference or policy action will potentially result, investigators have been encouraged to employ quantitative bias analysis to more fully characterize the total uncertainty.25 Bias analysis methods have been used to address unmeasured confounding,27 selection bias,71 and information bias27, 72 in retrospective database research.
A second potential problem is the possibility of overweighting results from retrospective database–based research in a quantitative meta-analysis of an entire body of research on a particular topic. In such meta-analyses, weights are in proportion to the inverse of variance, so large studies carry most of the weight. The variance, however, measures only sampling error; it does not measure systematic error. This problem of large studies dominating the weights pertains to any meta-analysis that includes one or two studies much larger than the others. However, given the large sample sizes often achieved by retrospective database research, the high-weight studies may often come from studies nested in these databases. For example, in a 2004 quantitative meta-analysis of 11 prospective studies of the association between pregnancy termination and incident breast cancer,73 the two retrospective database studies74, 75 accounted for 54 percent of the weight in the meta-analysis, but only 18 percent (2 of 11) of the studies. Random effects meta-analyses76 and other weighting methods77 provide only a partial solution to this potential overweighting, and only in some circumstances. Meta-analysts should therefore consider the potential for retrospective database research to be overweighted in their quantitative summary estimates. A plot of the inverse-normal of rank percentile against the corresponding study's estimate of association and confidence interval provides a visual depiction of the distribution of study results,78 without undue influence by overpowered studies. (See, for example, the aforementioned meta-analysis of the association between pregnancy termination and breast cancer risk.73)
4. Special Opportunities
As noted earlier, retrospective database research runs the gamut of research topics. There are, however, several research areas to which retrospective database research studies are particularly well suited.
4.1. Rapid Response to Emerging Problems, With Prospective Data
Retrospective database research is ordinarily secondary to another primary purpose. While the collected data may not be optimized to a particular research topic, it is often possible to use the collected data for rapid response to emerging research problems.79 The study mentioned above of the association between statins medication and incident ALS is also a suitable example here. Drug surveillance databases had identified a higher-than-expected prevalence of statins medications associated with reports of ALS. A pooled analysis of trials data revealed no association, but was limited by the small number of ALS cases, short duration of followup, and potential for crossover from the placebo arm to statins treatment after the trial finished.80 Thus, there was little evidence to evaluate the potential causal association between this highly effective drug class—which prevents cardiovascular morbidity and mortality81, 82—and the incidence of ALS, a progressive, neurodegenerative, terminal disease.83
The precisely measured null association reported in the case-control study15 provided a rapid and reliable basis to assuage concerns about an etiologic association between statin use and ALS occurrence. Imagine what would have been required for a purposefully designed study to evaluate the association. The pooled trials result had included nearly 120,000 individuals observed over more than 400,000 person-years, yet included only 19 cases of this rare disease. Few existing cohort studies would have had sufficient person-time to expect substantially more cases, and a cohort study designed to evaluate the association would have required a substantial investment of time and financial support.
A case-control study might have been feasible, but imagine the resources required to enroll and interview an equivalent number of ALS cases as were included in the database study (∼550) and their matched controls. Furthermore, a case-control study of this design would likely have been susceptible to recall bias and selection bias.4, 7 The retrospective database research study avoided both of these biases.15 Recall bias was avoided by ascertaining statins use from a prescription database. These prescriptions were recorded before the ALS incidence, so could not have been affected by the subsequent disease occurrence. Selection bias was avoided because all ALS cases in the region during the followup period were included, and controls were selected from the Civil Registration System. Neither case/control status nor use of statins was likely to be associated with participation. Thus, the retrospective database research study on this topic provided a rapid, cost-efficient, and precise result on an important public health topic, which otherwise would have gone unevaluated or would have required a substantial investment of time and finances to achieve an equivalent, or possibly more biased, result. This study provides a good example of the value of retrospective database research in such circumstances.
4.2. Cost-Efficient Hypotheses-Scanning Analyses
Retrospective database research can sometimes evaluate multiple associations with only a marginal increase in cost over the evaluation of a single association. The U.S. Food and Drug Administration's (FDA) Sentinel Initiative will use an active surveillance system within electronic data from health care information holders to monitor the safety of all FDA-regulated products.84 Similarly, the EU-ADR project aims to use clinical data from health databases, combined with prescription databases, to detect adverse drug reactions.85, 86 The project uses text mining, epidemiological, and computational techniques to analyze electronic health records, with the goal of detecting combinations of drugs and adverse events that merit further investigation.
As a second example, Latourelle and colleagues used retrospective database research to evaluate the association between estrogen-related diseases, such as osteoporosis or endometriosis, and the occurrence of Parkinson's disease.87 To be categorized as “exposed” to these diseases, cases or controls had to have them appear as discharge codes in the hospital database before the first discharge code for Parkinson's disease. For relatively little additional cost, the investigators also evaluated the association between 200 other diseases and the subsequent diagnosis of Parkinson's disease as a hypothesis scanning study, with the objective of suggesting new ideas regarding Parkinson's disease etiology.87 The analysis adjusted for multiple comparisons using empirical Bayesian methods designed to reduce the emphasis on potentially false-positive associations.88 This potential for cost-effective hypotheses-scanning studies as an explicit objective of retrospective database research should be viewed as a strength of such research, not a limitation, so long as the objective is appropriately labeled as such. Hypotheses suggested by these types of studies are often further investigated using studies designed specifically for the topic.
4.3. Hybrid Designs
Retrospective database research does not necessarily have to be limited to data collection from secondary data sources. Hybrid designs allow the use of database research for some aspects of data collection, and primary data collection for others. For example, a study of drug-drug and gene-drug interactions that might reduce the effectiveness of tamoxifen therapy began by identifying eligible breast cancer patients using the Danish Breast Cancer Cooperative Group's clinical registry.89 This clinical registry also provided data on prognostic factors such as tumor diameter and lymph node evaluation, and on treatments such as chemotherapy and radiation therapy. Linkage with the Danish Civil Registration System provided data on vital status; linkage with the Danish National Patient Registry provided data on comorbid diseases; and linkage with the Danish National Registry of Medicinal Products provided data on use of prescription medications. Thus, for relatively low cost, a cohort of breast cancer patients with complete medical, prognostic, and breast cancer treatment data was assembled. A case-control study was then nested in this cohort by identifying cases of breast cancer recurrence and then matching controls to them by risk-set sampling.7 Once cases and controls had been identified, their tumor blocks were collected from the Danish National Pathology Registry,90 and these were used for the necessary bioassays. Thus, retrospective database research allowed identification of the source population and selection of cases and controls, and provided all but the bioassay data. These data, which are expensive to collect, were only obtained for about 13 percent of the members of the total cohort. This hybrid design demonstrates that retrospective database research will remain an important contributor, even in the era of personalized medicine.
In a second example of a hybrid design, survey data collected over the Internet were linked to retrospective database research.91, 92 The objectives of the study were to assess the feasibility and validity of studies that use the Internet to recruit and follow participants, evaluate the relationship between lifestyle and behavioral factors and delayed time to pregnancy among women attempting to conceive, and evaluate the relationship of several exposures to risk of miscarriage and infant birth weight among women who conceived. Participants were recruited by advertisements on Web sites likely to be visited by women who intended to become pregnant. They were directed to the study's Web site, where they completed an enrollment screening questionnaire followed by an interview covering socio-demographics, reproductive and medical history, lifestyle, and other factors. Enrolled participants were then contacted every 2 months by email for 12 months or until they reported that conception had occurred. Data obtained from the Web-based questionnaires were linked to nationwide databases, which allowed collection of additional data on confounders and outcomes, as well as an assessment of the validity of some of the self-reported data, such as prescription drug use. This study again demonstrated that retrospective database research, in combination with primary data collection, can provide a cost-efficient resource for collecting some aspects of the study data. In contrast to the previous cancer treatment example, the cohort in this pregnancy study was enrolled following more typical cohort study strategies, and not by using the databases to identify a source population.
Hybrid designs have also been used to collect data by medical record review for data fields that are available for a subset of participants in a database.93 Thus, the database provides a cost-efficient resource for initial data collection, which is then supplemented as necessary by medical record review or another primary data collection method to complete the data set. Once an investigator is open to the potential for hybrid designs and there are retrospective database resources suitable to the research topic, the opportunities for combining the databases with primary data collection are limited only by the investigator's creativity.
4.4. Ample Data Allows for Novel Designs
As mentioned above, the ample data often available from retrospective database research can lead to overweighting of such studies in quantitative meta-analyses. While this problem may be disadvantageous, a compensating advantage is the opportunity to use retrospective database research to implement novel study designs. For example, confounding by indication and other biases often plague clinical epidemiology,3, 23 even in the era of comparative effectiveness research. However, the ample study size often provided by retrospective database research can overcome these threats to validity in some situations. The large sample size might allow a design with carefully restricted exposure groups,1 for example, new users of a pharmaceutical only,94 whereas conventionally sized cohort studies would not always have sufficient study size to implement such a design. The new user design in turn facilitates other advanced designs, such as propensity score matching and instrumental variable analyses,21 which are intended to further counteract these threats to validity. These and other novel designs can be implemented in studies of any size, but are likely most effective when the study size is large.95
4.5. Data Pooling Methods
Although retrospective database research often provides relatively large study size within a research topic area, a study's power may still be insufficient if the study must be restricted to rare exposure subgroups or if the study outcome is rare. In these cases, data pooling across similar databases may allow sufficient sample size to provide adequate power. Data pooling also provides advantages over conventional meta-analyses because it allows simultaneous and consistent data analyses. However, such pooling projects face substantial challenges.
First among these challenges is harmonization of the data elements. To accommodate a pooled analysis, data collected from different databases must provide analytic variables (exposure, confounders, modifiers, and outcomes) with equivalent categorizations and definitions. Such data harmonization can be quite challenging. Harmonization of data elements categorized differently or differentially available in two or more databases may pose an insurmountable barrier to pooling. For example, one database might include data on behaviors like alcohol and tobacco use, whereas a second database might not. The pooling project would then face the unenviable decision of controlling for these behaviors for some, but not all, data centers (in which case the analysis becomes comparable to a conventional meta-analysis), or abandoning control for these variables at all centers in order to achieve the data harmonization goal. Differences in the conceptual underpinnings of data elements may be more common. Even a variable as conceptually simple as the Charlson comorbidity index96 can present surprising challenges when subject to harmonization considerations. The Charlson index includes 19 comorbid conditions (e.g., diabetes). As mentioned above, some databases might be able to ascertain diabetes diagnosed in all medical settings (e.g., general practitioner, outpatient, and inpatient), whereas others might be able to ascertain diabetes diagnosed in only a subset (e.g., only general practitioner or only outpatient specialty clinics). Diabetes is defined differently in the different databases, which are not strictly harmonious, and therefore contribute differently to the Charlson index. While the definition of the Charlson variable may be harmonious across the pooled databases, the underlying conceptualization is different, and this difference could result in differences in the strength of confounding by the comorbidity variable or in the degree to which it modifies the association between an exposure contrast and outcome.
Ethical and legal constraints, which are often placed on data sharing, present a second important challenge to pooling projects. Pooling of de-identified data sets can sometimes be arranged through data use agreements, but even these arrangements can be quite challenging and time-consuming. Rassen and colleagues compared four methods of pooling de-identified data sets:97 (1) full covariate information, which may violate privacy concerns; (2) aggregated data methods, which aggregate patients into mutually stratified cells with common characteristics, but usually delete cells with low frequency counts that might defeat the privacy protections of large frequency counts; (3) conventional fixed or random effects meta-analysis, which provides only summary estimates of association for pooling; and (4) propensity score-based pooling, for which a propensity score summarizes each individual's covariate information. They reported that the last alternative provided reasonable analytic flexibility and also strong protection of patient privacy, and advocated its use for studies that require pooling of databases, multivariate adjustment, and privacy protection.97
More recently, Wolfson and colleagues proposed a pooling method that requires no transfer of record-level data to a central analysis center.98 Rather, the central analysis center implements statistical computing code over a secure network, accessing record-level data maintained on servers at the individual study centers. Data aggregation occurs through return of anonymous summary statistics from these harmonized individual-level databases, and even iterative regression modeling can be implemented. The advantage is a reduced burden to comply with ethical and legal requirements to protect privacy, since no record-level data are ever transferred. The disadvantages include requirements for strong data harmonization, secure networks that satisfy regulatory oversight, and assurances that no record-level data are transmitted. It is possible that some summary statistics could violate standards for de-identification, but safeguards can be implemented to prevent transmission of such summary statistics.
These new methods for pooling provide exciting opportunities for pooled projects. At the time of this writing, investigators who choose to undertake them should expect delays required to explain these methods to regulators responsible for oversight of data protection, who are not yet familiar with them. In addition, it is likely that implementing the methods for the first few projects will be challenging. With those caveats in mind, the path should be blazed, because once the methods are familiar and reliable, new research opportunities and efficiencies will inevitably arise. Investigator teams without the time, resources, or patience to implement these new methods can ordinarily rely on conventional meta-analysis methods,99 which solve the privacy protection concerns but also have some important disadvantages by comparison.97, 98
5. Summary
Retrospective database research has made important contributions to descriptive epidemiology, public health epidemiology targeted at disease prevention, and clinical epidemiology targeted at improving disease outcomes or estimates of disease prognosis. Investigators who conduct retrospective database research should first focus on the fundamentals of epidemiologic design and analysis, with the goal of achieving a valid, precise, and generalizable estimate of disease frequency or association. Beyond the fundamentals, retrospective database research presents special challenges for design and analysis, and special opportunities as well; researchers should be aware of both in order to optimize the yield from their work.
Case Examples for Chapter 18
Case Example 42Combining de-identified data from multiple registries to study long-term outcomes in a rare disease
Description | Four independent, prospective, observational, and multicenter disease registries participate in an ongoing systematic review of their aggregated data to study pediatric pulmonary arterial hypertension (PAH). The review is intended to describe disease course and long-term outcomes of pediatric PAH in real-world clinical settings. |
---|---|
Sponsor | Actelion Pharmaceuticals Ltd. |
Year Started | 2009 |
Year Ended | Ongoing |
No. of Sites | 4 multicenter registries |
No. of Patients | Approximately 500 |
Challenge
PAH is a rare disease that is poorly described in pediatric populations. Newly developed PAH therapies used in the pediatric population have recently led to improved survival, and patients are now likely to reach adulthood. This increased attention on pediatric PAH patients presents new challenges in both data needs and methodology to evaluate disease history and progression, general development, and clinical and treatment experience.
In 2009, the European Medicines Agency (EMA) approved Actelion's product bosentan for an expanded indication of pediatric PAH. The sponsor then began working with the EMA to determine how best to collect longitudinal treatment and outcomes data on this population.
Proposed Solution
Four existing registries already collected data on pediatric PAH patients: one is global and three are national in scope (in the United States, France, and the Netherlands). The sponsor and the EMA recognized that a compilation of results from these multiple registries within a common systematic review protocol would allow them to examine data from a large number of patients representing a significant proportion of global pediatric PAH patients. After the EMA approved the systematic review study design, the individual registries reviewed the protocol and agreed to participate.
The sponsor contacted the individual registries to evaluate their data collection and analysis practices. As it was not feasible to pool the data due to differences in data collection elements used by the registries, analyses were done by the respective registry data owners using similar methods under the guidance of a common statistical analysis plan. The de-identified summary tables were sent separately to the sponsor to be included in the systematic review reports.
The outcomes of interest are disease course and long-term outcome (e.g., clinical worsening, hospitalization, death) and general development (e.g., height, body mass index, sexual maturation, onset of puberty). The protocol and statistical analysis plan define the study population (all patients enrolled in one of the four registries aged ≤18 years at the time of diagnosis with PAH), observation period, appropriate statistical methods, and standardized procedures for data extraction (including data quality assurance).
Results
Analyses are performed on an annual basis and the same data cutoff date is applied to all registries to define the observation times of analysis (i.e., from Oct 2009 to the annual report's data cut-off date). This effectively creates a new cohort for each annual report, which is a stand-alone document.
The first annual report was sent to the EMA in 2010. For this first analysis, the sponsor had to address technical challenges related to differences between the registries. For example, three of the registries used the SAS software package to conduct their analysis, and one used SPSS, which produces a slightly different output. For subsequent reports, the sponsor also spent time in dialogue with the registries to clarify the detailed requirements, definitions, and analyses of the statistical analysis plan to ensure that each registry understood and interpreted it the same way.
Longitudinal analyses will be examined for evidence of improvement or deterioration over the followup period. The method of analysis respects correlations of within-patient measurements and is based on all patients with at least two measurements during the followup period.
Key Point
For rare disease populations where registries already exist, systematic review of registry data sets may be a more feasible way to analyze outcomes data rather than creating a new patient registry. When planning and conducting such a study, close collaboration between the parties is important to develop a detailed statistical analysis plan and clarify expectations for registry-level analyses.
For More Information
Berger RM, Beghetti M, Humpl T, et al. Clinical features of paediatric pulmonary hypertension: a registry study. Lancet. 2012 Feb 11;379(9815):537–46. [PMC free article: PMC3426911] [PubMed: 22240409].
Humbert M, Sitbon O, Chaouat A. Pulmonary arterial hypertension in France: results from a national registry. American journal of respiratory and critical care medicine. 2006;173(9):1023–30. [PubMed: 16456139].
McGoon MD, Krichman A, Farber HW, et al. Design of the REVEAL registry for US patients with pulmonary arterial hypertension. Mayo Clinic proceedings. Mayo Clinic. 2008. pp. 923–31. [PubMed: 18674477].
Muros-Le Rouzic E, Brand M, Wheeler J, et al. Systematic review methods to assess growth and sexual maturation in pediatric population suffering from pulmonary arterial hypertension in real-world clinical settings; 27th International Conference on Pharmacoepidemiology & Therapeutic Risk Management; August 14-17, 2011; Chicago, IL. Abstract 825..
van Loon RL, Roofthooft MT, van Osch-Gevers M, et al. Clinical characterization of pediatric pulmonary hypertension: complex presentation and diagnosis. J Pediatr. 2009 Aug;155(2):176–82.e1. Epub 2009 Jun 12. [PubMed: 19524254].
Case Example 43Understanding baseline characteristics of combined data sets prior to analysis
Description | The Kaiser Permanente Anterior Cruciate Ligament Reconstruction (KP ACLR) Registry was established to collect standardized data on ACLR procedures, techniques, graft types, and types of fixation and implants. The objectives of the registry are to identify risk factors that lead to degenerative joint disease, graft failure, and meniscal failure; determine outcomes of various graft types and fixation techniques; describe the epidemiology of ACLR patients; determine and compare procedure incidence rate at participating sites; and provide a framework for future studies tracking ACLR outcomes. |
---|---|
Sponsor | Kaiser Permanente |
Year Started | 2005 |
Year Ended | Ongoing |
No. of Sites | 42 surgical centers and 240 surgeons |
No. of Patients | 17,000 |
Challenge
The KP ACLR Registry aimed to collaborate with the Norwegian Ligament Reconstruction Registry on a series of studies to proactively identify patient risk factors as well as surgical practices and techniques associated with poor surgical outcomes. The Norwegian registry has been operating since 2004 and contains data on 14,232 patients. Combining data from these two registries would allow for faster identification of certain risk factors and evaluation of low frequency events.
Proposed Solution
The first step was to compare the patient cohorts of the registries and the surgical practices of the two countries. Aggregate data were shared between the registries in tabular form. Analysis was conducted to identify differences that would be important to consider when making inferences about a population other than that covered by the registry. Commonalities were also identified to determine when inferences could be made from each other's analysis and when data do not need to be adjusted.
Results
The analysis found that the registries generally have similar distributions of age, gender, preoperative patient-reported knee function, and knee-related quality of life. Differences were observed between the two registries in race, sports performed at the time of injury, time to surgery, graft use, and fixation type. While these differences should be accounted for in future analyses of combined data sets from both registries, the results indicate that analyses of the combined data sets are likely to produce findings that can be generalized to a wider population of ACLR patients.
Since this comparison was conducted, two hypothesis-driven analyses have begun, investigating questions using the combined registry data sets. Future plans include further collaboration with ACLR registries in additional countries.
Key Point
Combining or pooling registry data can be a valuable approach to achieving a larger sample size for data analysis. However, it is important to identify cohort and practice differences and similarities between registries before making generalizations of registry findings to other populations or sharing data for collaboration projects.
For More Information
http://www.kpimplantregistries.org/Registries/acl.htm
Granan LP, Inacio MC, Maletis GB, et al. Intraoperative findings and procedures in culturally and geographically different patient and surgeon populations. ACTA Orthop. 2012;83:577–82. [PMC free article: PMC3555446] [PubMed: 23116436].
Maletis G, Granan LP, Inacio M, et al. Comparison of a community based anterior cruciate ligament reconstruction registry in the United States and Norway. The Journal of Bone and Joint Surgery. 2011 December;93(Supplement 3):31–6. [PubMed: 22262420].
References for Chapter 18
- 1.
- Ray WA. Improving automated database studies. Epidemiology. 2011 May;22(3):302–4. [PubMed: 21464650]
- 2.
- Federspiel CF, Ray WA, Schaffner W. Medicaid records as a valid data source: the Tennessee experience. Med Care. 1976 Feb;14(2):166–72. [PubMed: 768652]
- 3.
- Weiss NS. The new world of data linkages in clinical epidemiology: are we being brave or foolhardy? Epidemiology. 2011 May;22(3):292–4. [PubMed: 21464647]
- 4.
- Rothman K, Greenland S, Lash TL. Validity in epidemiologic studies. In: Rothman K, Greenland S, Lash TL, editors. Modern Epidemiology. 3rd ed. Philadelphia: Lippincott Williams & Wilkins; 2008. pp. 128–47.
- 5.
- Lash TL, Fox MP, Fink AK. Applying quantitative bias analysis to epidemiologic data. New York, NY: Springer; 2009.
- 6.
- Rothman K, Greenland S, Poole C, et al. Causation and causal inference. In: Rothman K, Greenland S, Lash TL, editors. Modern Epidemiology. 3rd ed. Philadelphia: Lippincott Williams & Wilkins; 2008. pp. 5–31.
- 7.
- Rothman K, Greenland S, Lash TL. Case-control studies. In: Rothman K, Greenland S, Lash TL, editors. Modern Epidemiology. 3rd ed. Philadelphia: Lippincott Williams & Wilkins; 2008. pp. 111–27.
- 8.
- Souhami L, Bae K, Pilepich M, et al. Impact of the duration of adjuvant hormonal therapy in patients with locally advanced prostate cancer treated with radiotherapy: a secondary analysis of RTOG 85-31. J Clin Oncol. 2009 May 1;27(13):2137–43. [PMC free article: PMC2674000] [PubMed: 19307511]
- 9.
- Collette L, Studer UE. Selection bias is not a good reason for advising more than 5 years of adjuvant hormonal therapy for all patients with locally advanced prostate cancer treated with radiotherapy. J Clin Oncol. 2009 Nov 20;27(33):e201–2. author reply e4. [PubMed: 19786659]
- 10.
- Greenland S, Rothman K. Measures of occurrence. In: Rothman K, Greenland S, Lash TL, editors. Modern Epidemiology. 3rd ed. Philadelphia: Lippincott Williams & Wilkins; 2008. pp. 32–50.
- 11.
- Murthy VH, Krumholz HM, Gross CP. Participation in cancer clinical trials: race-, sex-, and age-based disparities. JAMA. 2004 Jun 9;291(22):2720–6. [PubMed: 15187053]
- 12.
- Heiat A, Gross CP, Krumholz HM. Representation of the elderly, women, and minorities in heart failure clinical trials. Arch Intern Med. 2002 Aug 12-26;162(15):1682–8. [PubMed: 12153370]
- 13.
- Edwards IR, Star K, Kiuru A. Statins, neuromuscular degenerative disease and an amyotrophic lateral sclerosis-like syndrome: an analysis of individual case safety reports from vigibase. Drug Saf. 2007;30(6):515–25. [PubMed: 17536877]
- 14.
- Colman E, Szarfman A, Wyeth J, et al. An evaluation of a data mining signal for amyotrophic lateral sclerosis and statins detected in FDA's spontaneous adverse event reporting system. Pharmacoepidemiol Drug Saf. 2008 Nov;17(11):1068–76. [PubMed: 18821724]
- 15.
- Sorensen HT, Riis AH, Lash TL, et al. Statin use and risk of amyotrophic lateral sclerosis and other motor neuron disorders. Circ Cardiovasc Qual Outcomes. 2010 Jul;3(4):413–7. [PubMed: 20530788]
- 16.
- Rothman K, Greenland S, Lash TL. Design strategies to improve study accuracy. In: Rothman K, Greenland S, Lash TL, editors. Modern Epidemiology. 3rd ed. Philadelphia: Lippincott Williams & Wilkins; 2008. pp. 162–82.
- 17.
- Erkinjuntti T, Ostbye T, Steenhuis R, et al. The effect of different diagnostic criteria on the prevalence of dementia. N Engl J Med. 1997 Dec 4;337(23):1667–74. [PubMed: 9385127]
- 18.
- Jick SS, Bradbury BD. Statins and newly diagnosed diabetes. Br J Clin Pharmacol. 2004 Sep;58(3):303–9. [PMC free article: PMC1884569] [PubMed: 15327590]
- 19.
- Brenner H, Savitz DA. The effects of sensitivity and specificity of case selection on validity, sample size, precision, and power in hospital-based case-control studies. Am J Epidemiol. 1990 Jul;132(1):181–92. [PubMed: 2192549]
- 20.
- Jick H, Garcia Rodriguez LA, Perez-Gutthann S. Principles of epidemiological research on adverse and beneficial drug effects. Lancet. 1998 Nov 28;352(9142):1767–70. [PubMed: 9848368]
- 21.
- Sturmer T, Jonsson Funk M, Poole C, et al. Nonexperimental comparative effectiveness research using linked healthcare databases. Epidemiology. 2011 May;22(3):298–301. [PMC free article: PMC4012640] [PubMed: 21464649]
- 22.
- Jick SS, Kaye JA, Russmann S, et al. Risk of nonfatal venous thromboembolism with oral contraceptives containing norgestimate or desogestrel compared with oral contraceptives containing levonorgestrel. Contraception. 2006 Jun;73(6):566–70. [PubMed: 16730485]
- 23.
- Bosco JL, Silliman RA, Thwin SS, et al. A most stubborn bias: no adjustment method fully resolves confounding by indication in observational studies. J Clin Epidemiol. 2010 Jan;63(1):64–74. [PMC free article: PMC2789188] [PubMed: 19457638]
- 24.
- Greenland S. The effect of misclassification in the presence of covariates. Am J Epidemiol. 1980 Oct;112(4):564–9. [PubMed: 7424903]
- 25.
- Greenland S, Lash TL. Bias analysis. In: Rothman K, Greenland S, Lash TL, editors. Modern Epidemiology. 3rd ed. Philadelphia: Lippincott Williams & Wilkins; 2008. pp. 345–80.
- 26.
- Bradbury BD, Wilk JB, Kaye JA. Obesity and the risk of prostate cancer (United States). Cancer Causes Control. 2005 Aug;16(6):637–41. [PubMed: 16049801]
- 27.
- Lash TL, Schmidt M, Jensen AO, et al. Methods to apply probabilistic bias analysis to summary estimates of association. Pharmacoepidemiol Drug Saf. 2010 Jun;19(6):638–44. [PubMed: 20535760]
- 28.
- Goldberg J, Gelfand HM, Levy PS. Registry evaluation methods: a review and case study. Epidemiol Rev. 1980;2:210–20. [PubMed: 7000537]
- 29.
- Roos LL, Mustard CA, Nicol JP, et al. Registries and administrative data: organization and accuracy. Med Care. 1993 Mar;31(3):201–12. [PubMed: 8450678]
- 30.
- Sorensen HT, Baron JA. Registries and medical databases. In: Trichopoulos D, Olsen JH, Saracci R, editors. Teaching Epidemiology. New York, NY: Oxford University Press; 2010. pp. 455–67.
- 31.
- Clement FM, James MT, Chin R, et al. Validation of a case definition to define chronic dialysis using outpatient administrative data. BMC Med Res Methodol. 2011;11:25. [PMC free article: PMC3055853] [PubMed: 21362182]
- 32.
- Aphramor L. Validity of claims made in weight management research: a narrative review of dietetic articles. Nutr J. 2010;9:30. [PMC free article: PMC2916886] [PubMed: 20646282]
- 33.
- Kim SY, Solomon DH, Liu J, et al. Accuracy of identifying neutropenia diagnoses in outpatient claims data. Pharmacoepidemiol Drug Saf. 2011 Jul;20(7):709–13. [PMC free article: PMC3142869] [PubMed: 21567653]
- 34.
- Quach S, Blais C, Quan H. Administrative data have high variation in validity for recording heart failure. Can J Cardiol. 2010 Oct;26(8):306–12. [PMC free article: PMC2954539] [PubMed: 20931099]
- 35.
- Chen G, Khan N, Walker R, et al. Validating ICD coding algorithms for diabetes mellitus from administrative data. Diabetes Res Clin Pract. 2010 Aug;89(2):189–95. [PubMed: 20363043]
- 36.
- Tollefson MK, Gettman MT, Karnes RJ, et al. Administrative data sets are inaccurate for assessing functional outcomes after radical prostatectomy. J Urol. 2011 May;185(5):1686–90. [PubMed: 21419458]
- 37.
- Thygesen SK, Christiansen CF, Christensen S, et al. The predictive value of ICD-10 diagnostic coding used to assess Charlson comorbidity index conditions in the population-based Danish National Registry of Patients. BMC Med Res Methodol. 2011;11:83. [PMC free article: PMC3125388] [PubMed: 21619668]
- 38.
- Cai S, Mukamel DB, Veazie P, et al. Validation of the Minimum Data Set in identifying hospitalization events and payment source. J Am Med Dir Assoc. 2011 Jan;12(1):38–43. [PMC free article: PMC3052878] [PubMed: 21194658]
- 39.
- Tirschwell DL, Longstreth WT Jr. Validating administrative data in stroke research. Stroke. 2002 Oct;33(10):2465–70. [PubMed: 12364739]
- 40.
- Clinical Practice Research Datalink. [September 30, 2013]. http://www
.cprd.com/intro.asp. - 41.
- Sorenson HT, Christensen T, Schlosser HK, et al. Use of Medical Databases in Clinical Epidemiology. Second ed. Denmark: SUN-TRYK Aarhus Universitet; 2009.
- 42.
- Anderson IB, Sorensen TI, Prener A. Increase in incidence of disease due to diagnostic drift: primary liver cancer in Denmark, 1943-85. BMJ. 1991 Feb 23;302(6774):437–40. [PMC free article: PMC1669338] [PubMed: 2004170]
- 43.
- Lash TL, Johansen MB, Christensen S, et al. Hospitalization rates and survival associated with COPD: a nationwide Danish cohort study. Lung. 2011 Feb;189(1):27–35. [PubMed: 21170722]
- 44.
- Rodriguez LA, Perez-Gutthann S, Jick SS. The UK General Practice Research Database. In: Strom BL, editor. Pharmacopepidemiology. 3rd ed. Chichester, UK: John Wiley & Sons, LTD; 2000. pp. 375–85.
- 45.
- Bohnert AS, Ilgen MA, Galea S, et al. Accidental poisoning mortality among patients in the Department of Veterans Affairs Health System. Med Care. 2011 Apr;49(4):393–6. [PubMed: 21407033]
- 46.
- Hansen JG, Pedersen L, Overvad K, et al. The Prevalence of chronic obstructive pulmonary disease among Danes aged 45-84 years: population-based study. COPD. 2008 Dec;5(6):347–52. [PubMed: 19353348]
- 47.
- Alonso A, Jick SS, Jick H, et al. Antibiotic use and risk of multiple sclerosis. Am J Epidemiol. 2006 Jun 1;163(11):997–1002. [PubMed: 16597708]
- 48.
- Hernan MA, Hernandez-Diaz S, Robins JM. A structural approach to selection bias. Epidemiology. 2004 Sep;15(5):615–25. [PubMed: 15308962]
- 49.
- Clough-Gorr KM, Fink AK, Silliman RA. Challenges associated with longitudinal survivorship research: attrition and a novel approach of reenrollment in a 6-year follow-up study of older breast cancer survivors. J Cancer Surviv. 2008 Jun;2(2):95–103. [PubMed: 18648978]
- 50.
- Donders AR, van der Heijden GJ, Stijnen T, et al. Review: a gentle introduction to imputation of missing values. J Clin Epidemiol. 2006 Oct;59(10):1087–91. [PubMed: 16980149]
- 51.
- Howe CJ, Cole SR, Chmiel JS, et al. Limitation of inverse probability-of-censoring weights in estimating survival in the presence of strong selection bias. Am J Epidemiol. 2011 Mar 1;173(5):569–77. [PMC free article: PMC3105434] [PubMed: 21289029]
- 52.
- Cain KC, Harlow SD, Little RJ, et al. Bias due to left truncation and left censoring in longitudinal studies of developmental and disease processes. Am J Epidemiol. 2011 May 1;173(9):1078–84. [PMC free article: PMC3121224] [PubMed: 21422059]
- 53.
- Bosco JL, Antonsen S, Sorensen HT, et al. Metformin and incident breast cancer among diabetic women: a population-based case-control study in Denmark. Cancer Epidemiol Biomarkers Prev. 2011 Jan;20(1):101–11. [PubMed: 21119073]
- 54.
- Ibrahim JG, Chu H, Chen LM. Basic concepts and methods for joint models of longitudinal and survival data. J Clin Oncol. 2010 Jun 1;28(16):2796–801. [PMC free article: PMC4503792] [PubMed: 20439643]
- 55.
- Riley GF. Administrative and claims records as sources of health care cost data. Med Care. 2009 Jul;47(7 Suppl 1):S51–5. [PubMed: 19536019]
- 56.
- Suissa S. Immeasurable time bias in observational studies of drug effects on mortality. Am J Epidemiol. 2008 Aug 1;168(3):329–35. [PubMed: 18515793]
- 57.
- Bradbury BD, Wang O, Critchlow CW, et al. Exploring relative mortality and epoetin alfa dose among hemodialysis patients. Am J Kidney Dis. 2008 Jan;51(1):62–70. [PubMed: 18155534]
- 58.
- Walker AM. Confounding by indication. Epidemiology. 1996 Jul;7(4):335–6. [PubMed: 8793355]
- 59.
- Miettinen OS. The need for randomization in the study of intended effects. Stat Med. 1983 Apr-Jun;2(2):267–71. [PubMed: 6648141]
- 60.
- NCCN Practice Guidelines in Oncology, Breast Cancer - v.2.2011. Invasive breast cancer, systemic adjuvant treatment. 2010. [August 15, 2012]. [National Comprehensive Cancer Network] http://www
.nccn.org/professionals /physician_gls /f_guidelines.asp. - 61.
- Geiger AM, Thwin SS, Lash TL, et al. Recurrences and second primary breast cancers in older women with initial early-stage disease. Cancer. 2007 Mar 1;109(5):966–74. [PubMed: 17243096]
- 62.
- Brookhart MA, Wang PS, Solomon DH, et al. Instrumental variable analysis of secondary pharmacoepidemiologic data. Epidemiology. 2006 Jul;17(4):373–4. [PubMed: 16810095]
- 63.
- Bradbury BD, Brookhart MA, Winkelmayer WC, et al. Evolving statistical methods to facilitate evaluation of the causal association between erythropoiesis-stimulating agent dose and mortality in nonexperimental research: strengths and limitations. Am J Kidney Dis. 2009 Sep;54(3):554–60. [PubMed: 19592144]
- 64.
- Weiss NS, Dublin S. Accounting for time-dependent covariates whose levels are influenced by exposure status. Epidemiology. 1998 Jul;9(4):436–40. [PubMed: 9647909]
- 65.
- Hernan MA, Brumback B, Robins JM. Marginal structural models to estimate the causal effect of zidovudine on the survival of HIV-positive men. Epidemiology. 2000 Sep;11(5):561–70. [PubMed: 10955409]
- 66.
- Cole SR, Hernan MA, Robins JM, et al. Effect of highly active antiretroviral therapy on time to acquired immunodeficiency syndrome or death using marginal structural models. Am J Epidemiol. 2003 Oct 1;158(7):687–94. [PubMed: 14507605]
- 67.
- Zhang Y, Thamer M, Cotter D, et al. Estimated effect of epoetin dosage on survival among elderly hemodialysis patients in the United States. Clin J Am Soc Nephrol. 2009 Mar;4(3):638–44. [PMC free article: PMC2653651] [PubMed: 19261818]
- 68.
- Wang O, Kilpatrick RD, Critchlow CW, et al. Relationship between epoetin alfa dose and mortality: findings from a marginal structural model. Clin J Am Soc Nephrol. 2010 Feb;5(2):182–8. [PMC free article: PMC2827587] [PubMed: 20019122]
- 69.
- Greenland S, Schwartzbaum JA, Finkle WD. Problems due to small samples and sparse data in conditional logistic regression analysis. Am J Epidemiol. 2000 Mar 1;151(5):531–9. [PubMed: 10707923]
- 70.
- Greenland S. Randomization, statistics, and causal inference. Epidemiology. 1990 Nov;1(6):421–9. [PubMed: 2090279]
- 71.
- Fink AK, Lash TL. A null association between smoking during pregnancy and breast cancer using Massachusetts registry data (United States). Cancer Causes Control. 2003 Jun;14(5):497–503. [PubMed: 12946045]
- 72.
- Lash TL, Fox MP, Thwin SS, et al. Using probabilistic corrections to account for abstractor agreement in medical record reviews. Am J Epidemiol. 2007 Jun 15;165(12):1454–61. [PubMed: 17406006]
- 73.
- Lash TL, Fink AK. Null association between pregnancy termination and breast cancer in a registry-based study of parous women. Int J Cancer. 2004 Jun 20;110(3):443–8. [PubMed: 15095312]
- 74.
- Melbye M, Wohlfahrt J, Olsen JH, et al. Induced abortion and the risk of breast cancer. N Engl J Med. 1997 Jan 9;336(2):81–5. [PubMed: 8988884]
- 75.
- Goldacre MJ, Kurina LM, Seagroatt V, et al. Abortion and breast cancer: a case-control record linkage study. J Epidemiol Community Health. 2001 May;55(5):336–7. [PMC free article: PMC1731878] [PubMed: 11297654]
- 76.
- Poole C, Greenland S. Random-effects meta-analyses are not always conservative. Am J Epidemiol. 1999 Sep 1;150(5):469–75. [PubMed: 10472946]
- 77.
- Shuster JJ. Empirical vs natural weighting in random effects meta-analysis. Stat Med. 2010 May 30;29(12):1259–65. [PMC free article: PMC3697007] [PubMed: 19475538]
- 78.
- Cunnane C. Unbiased plotting positions - a review. Journal of Hydrology. 1978;37:205–22.
- 79.
- Grady D, Hearst H. Utilizing existing databases. In: Hully SB, Cummings SR, Browner WS, et al., editors. Designing Clinical Research. 3rd ed. Philadelphia: Lippincott Williams & Wilkins; 2007. pp. 207–21.
- 80.
- Sorensen HT, Lash TL. Statins and amyotrophic lateral sclerosis--the level of evidence for an association. J Intern Med. 2009 Dec;266(6):520–6. [PubMed: 19930099]
- 81.
- Thavendiranathan P, Bagai A, Brookhart MA, et al. Primary prevention of cardiovascular diseases with statin therapy: a meta-analysis of randomized controlled trials. Arch Intern Med. 2006 Nov 27;166(21):2307–13. [PubMed: 17130382]
- 82.
- Aronow HD, Topol EJ, Roe MT, et al. Effect of lipid-lowering therapy on early mortality after acute coronary syndromes: an observational study. Lancet. 2001 Apr 7;357(9262):1063–8. [PubMed: 11297956]
- 83.
- Mitchell JD, Borasio GD. Amyotrophic lateral sclerosis. Lancet. 2007 Jun 16;369(9578):2031–41. [PubMed: 17574095]
- 84.
- Rosati K. Using electronic health information for pharmacovigilance: the promise and the pitfalls. J Health Life Sci Law. 2009 Jul;2(4):171, 3–239. [PubMed: 19673181]
- 85.
- Trifiro G, Pariente A, Coloma PM, et al. Data mining on electronic health record databases for signal detection in pharmacovigilance: which events to monitor? Pharmacoepidemiol Drug Saf. 2009 Dec;18(12):1176–84. [PubMed: 19757412]
- 86.
- Coloma PM, Schuemie MJ, Trifiro G, et al. Combining electronic healthcare databases in Europe to allow for large-scale drug safety monitoring: the EU-ADR Project. Pharmacoepidemiol Drug Saf. 2011 Jan;20(1):1–11. [PubMed: 21182150]
- 87.
- Latourelle JC, Dybdahl M, Destefano AL, et al. Estrogen-related and other disease diagnoses preceding Parkinson's disease. Clin Epidemiol. 2010;2:153–70. [PMC free article: PMC2943181] [PubMed: 20865113]
- 88.
- Greenland S, Robins JM. Empirical-Bayes adjustments for multiple comparisons are sometimes useful. Epidemiology. 1991 Jul;2(4):244–51. [PubMed: 1912039]
- 89.
- Lash TL, Cronin-Fenton D, Ahern TP, et al. CYP2D6 inhibition and breast cancer recurrence in a population-based study in Denmark. J Natl Cancer Inst. 2011 Mar 16;103(6):489–500. [PMC free article: PMC3057982] [PubMed: 21325141]
- 90.
- Erichsen R, Lash TL, Hamilton-Dutoit SJ, et al. Existing data sources for clinical epidemiology: the Danish National Pathology Registry and Data Bank. Clin Epidemiol. 2010;2:51–6. [PMC free article: PMC2943174] [PubMed: 20865103]
- 91.
- Mikkelsen EM, Hatch EE, Wise LA, et al. Cohort profile: the Danish Web-based Pregnancy Planning Study—‘Snart-Gravid’ Int J Epidemiol. 2009 Aug;38(4):938–43. [PMC free article: PMC2734065] [PubMed: 18782897]
- 92.
- Huybrechts KF, Mikkelsen EM, Christensen T, et al. A successful implementation of e-epidemiology: the Danish pregnancy planning study ‘Snart-Gravid’ Eur J Epidemiol. 2010 May;25(5):297–304. [PMC free article: PMC2945880] [PubMed: 20148289]
- 93.
- Thwin SS, Clough-Gorr KM, McCarty MC, et al. Automated inter-rater reliability assessment and electronic data collection in a multi-center breast cancer study. BMC Med Res Methodol. 2007;7:23. [PMC free article: PMC1919388] [PubMed: 17577410]
- 94.
- Ray WA. Evaluating medication effects outside of clinical trials: new-user designs. Am J Epidemiol. 2003 Nov 1;158(9):915–20. [PubMed: 14585769]
- 95.
- Brookhart MA, Rassen JA, Schneeweiss S. Instrumental variable methods in comparative safety and effectiveness research. Pharmacoepidemiol Drug Saf. 2010 Jun;19(6):537–54. [PMC free article: PMC2886161] [PubMed: 20354968]
- 96.
- Charlson ME, Pompei P, Ales KL, et al. A new method of classifying prognostic comorbidity in longitudinal studies: development and validation. J Chronic Dis. 1987;40(5):373–83. [PubMed: 3558716]
- 97.
- Rassen JA, Solomon DH, Curtis JR, et al. Privacy-maintaining propensity score-based pooling of multiple databases applied to a study of biologics. Med Care. 2010 Jun;48(6 Suppl):S83–9. [PMC free article: PMC2933455] [PubMed: 20473213]
- 98.
- Wolfson M, Wallace SE, Masca N, et al. DataSHIELD: resolving a conflict in contemporary bioscience--performing a pooled analysis of individual-level data without sharing the data. Int J Epidemiol. 2010 Oct;39(5):1372–82. [PMC free article: PMC2972441] [PubMed: 20630989]
- 99.
- Greenland S, O'Rourke K. Meta-analysis. In: Rothman K, Greenland S, Lash TL, editors. Modern Epidemiology. 3rd ed. Philadelphia: Lippincott Williams & Wilkins; 2008. pp. 652–82.
- Analysis of Linked Registry Data Sets - Registries for Evaluating Patient Outcom...Analysis of Linked Registry Data Sets - Registries for Evaluating Patient Outcomes
- LOC105370673 [Homo sapiens]LOC105370673 [Homo sapiens]Gene ID:105370673Gene
Your browsing activity is empty.
Activity recording is turned off.
See more...