NCBI Bookshelf. A service of the National Library of Medicine, National Institutes of Health.
Institute of Medicine (US) Committee on Disability in America; Field MJ, Jette AM, editors. The Future of Disability in America. Washington (DC): National Academies Press (US); 2007.
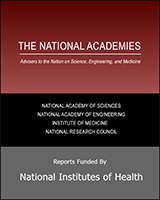
The Future of Disability in America.
Show detailsDavid J. Knutson *
This paper provides an update on the current status of formal methods of risk adjustment of payments to health plans and an assessment of the implications of these methods for people with disabilities. The paper examines the development, implementation, and impact of risk adjustment methods intended for use by multiple purchasers and payers in the United States. Purchasers and payers include, in particular, public programs, such as Medicare and Medicaid, as well as large and small employer-sponsored group plans and individual insurance plans.
The paper also evaluates the strengths and weaknesses of various risk adjustment methods, emphasizing their adequacy in setting payment for health plans that enroll people with disabilities. Emerging issues and trends related to improving risk assessment methods and their impact on improved access to financing and health care for disabled people are also explored.
Iezzoni’s Risk Adjustment for Measuring Health Care Outcomes, the foundation reference on risk adjustment, provides a comprehensive introduction to the concepts and techniques of risk adjustment as a generic tool (Iezzoni, 2003). This paper focuses on one particular purpose of risk adjustment: setting payments for health plans. Risk adjustment is a tool that can be used to improve the fairness of payment by accounting for enrollees’ health when capitated reimbursement levels are set. Improving the fairness of payment is achieved by removing or diminishing economic incentives for avoiding people who might generate high costs because of their underlying health status. Improved risk adjustment methods help reduce information asymmetry among stakeholders and support transparency for managing and regulating risk pools to achieve equitable and efficient health care financing policy objectives.
For this application of risk adjustment, the risk is the medical expenditure level and the time frames involved with typical insurance or managed care contracts, which are usually 12 months. The risk adjustment methods addressed here focus on the use of risk factors to predict or explain individual or group variations in medical expenditures for a 12-month period.
INSURANCE PROGRAMS FOR PEOPLE WITH DISABILITIES
Medicare and Medicaid programs fund a large proportion of health care for people with disabilities. The financing mechanism is primarily fee for service; however, both Medicare and state Medicaid programs are attempting to expand managed care for people with disabilities. The Medicare Advantage program, authorized by the Medicare Modernization Act of 2003, continues Medicare’s 1985 authority to contract with managed care plans. The legislation also authorized, through December 2008, new special needs plans (SNPs) that can disproportionately or exclusively enroll Medicare beneficiaries with special needs: institutionalized individuals, individuals dually eligible for Medicaid and Medicare, and individuals with high-cost chronic diseases.
The vast majority of SNP proposals received through the end of 2005 were for beneficiaries dually eligible for Medicaid and Medicare. Most SNPs chose to enroll exclusively populations with special needs instead of a greater percentage of individuals with special needs in a traditional plan. To date, more than 400 SNPs have been approved. Of these 37 are for institutionalized individuals, 13 are for individuals with chronic diseases, and the remainder is for individuals dually eligible for Medicaid and Medicare. These plans will compete with traditional Medicare Advantage plans, and their capitation payments will be based on the same risk adjustment method used by typical Medicare Advantage plans.
SNPs that selectively or exclusively enroll high-risk or disabled ben eficiaries want the most accurate risk adjustment methodology possible to be used so that they will be assured of adequate funding. Unfortunately, as will be described here, even the best risk adjustment methods in use today significantly underestimate the actual cost of health care for individuals with major chronic illnesses and disabilities.
When the Medicare managed care program began in the mid-1980s, the demographic methods used for the risk adjustment of payments were not very accurate in allocating capitation payments on the basis of the relative needs of the beneficiary. The Centers for Medicare and Medicaid Services (CMS) has now adopted a state-of-the-art diagnosis-based risk adjuster for Medicare managed care plan payment, has added a frailty adjuster (a population sample, survey-based, functional status risk factor) to improve payment accuracy for specific demonstration programs for disabled people, and is considering using this functional status risk factor for all Medicare Advantage plans.
For many years state Medicaid programs, through federal waiver authorizations, have contracted with managed care plans to provide health care coverage for Medicaid beneficiaries. In nearly every case, managed care plans have been paid a risk-adjusted capitation. The major risk adjustment concern for the coverage of disabled populations covered by Medicaid, as it is for coverage by Medicare Advantage, is the accuracy of the methods used to adjust the payments to health plans on the basis of risk and its economic impact on health plans that enroll people with disabilities. As discussed below, many states do not use state-of-the-art risk adjustment methods.
Medicaid demonstration projects that serve individuals dually eligible for Medicare and Medicaid must contract with Medicare SNPs by 2008 if they wish to integrate Medicare and Medicaid payments. From the standpoint of the accuracy of risk-based payment rates, there may be less concern for the Medicaid side of managed care financing for people dually eligible for Medicaid and Medicare now that the implementation of Medicare Part D (coverage of prescription drugs) has shifted a major cost item from Medicaid to Medicare. For managed care programs financed exclusively by the Medicaid program, the level of concern for implementing the most accurate form of risk adjustment is even greater than it is for Medicare.
The central issue of risk adjustment and its implications for people with disabilities are relevant to most purchasers who offer more than one health plan to their beneficiaries and who provide the primary coverage for medical care. In other words, wherever risk selection occurs, the need for risk adjustment becomes critical to provide adequate capitation payments on behalf of people with high-cost conditions, including disabilities that require costly medical care.
MEDICAL COSTS AND POPULATIONS
Medical costs are highly skewed in any population. A relatively small proportion of the population (15 to 20 percent) accounts for two-thirds or more of the total health care expenditures for the entire population. Another way to characterize the skew is that about 80 percent of the population typically has medical costs below the mean per capita medical cost for the population. With no risk adjustment, a capitation payment system would pay far too much for 80 percent of the population and significantly underpay for the 20 percent whose costs are above the mean. If enrollment were randomly distributed across the population, the over- and underpayments would average to the right amount.
This skewed cost distribution is found in the general population and also in insurance programs exclusively for people with disabilities, even though the average cost per capita varies substantially between the two populations. A skewed distribution is also found in the Medicaid population. Ireys and colleagues (1997) found that in a pediatric Medicaid population, the medical costs for beneficiaries with selected chronic illnesses ranged from 10 to 29 times the mean for all children in the population. Specific examples include cardiopulmonary disease, the costs for which were 10 times greater than the mean for all children; cystic fibrosis, the costs for which were 14 times greater; chronic respiratory disease, the costs for which were 20 times greater, muscular dystrophy, the costs for which were 17 times; and, spina bifida, the costs for which were 11 times greater.
When one considers the distribution of medical costs for people with disabilities, the relationship between functional status and diagnosis becomes complex. Whereas the average cost for populations with disabilities is higher than that for the general population, the risk distribution is similar to that for the general population. For example, for Medicare beneficiaries over age 65 years enrolled in a Medicare Advantage health plan, CMS currently includes a risk factor indicator of whether a Medicare beneficiary was originally eligible for Medicare because of a disability. The average cost for this “ever disabled” population over age 65 years is nearly 50 percent higher than for the Medicare population over age 65 years as a whole. However, not all people with disabilities have medical costs that are greater. The specific diagnoses must be taken into account. Most of the difference in cost between a population with disabilities and a general population is explained by the type and the number of diagnoses recorded. Functional status, which may be considered a more direct measure of disability, in fact, adds only a modest improvement in the ability of current risk adjustment models to predict medical costs.
Kronick and colleagues (1995) reported that for a number of state Medicaid programs designed exclusively for people with disabilities (non institutionalized individuals and individuals not dually eligible for Medicaid and Medicare), 30 percent of the people with disabilities had no diagnosis of a chronic illness and their medical costs were similar to those for the general population. However, those with major chronic conditions had medical costs that were many times greater. For example, individuals with a diagnosis of multiple sclerosis had costs eight times greater than those with no diagnosis of a chronic disease. (The analysis included prescription drug costs.) Individuals with cardiovascular disease had costs 10 times greater than for those with no chronic disease, and those with quadriplegia had costs ranging from 3 to 34 times greater than for those with no chronic disease. This range also demonstrates that what would seem to be a direct risk factor (i.e., disability status), without reference to the presence of a chronic health condition, can fail to capture the high degrees of variability in health status and health care costs among people with disabilities. Pope and colleagues (2004) identified five conditions that predict higher medical costs (ranging from $2,200 to $9,700 in additional payments) for the disabled population over age 65 years enrolled in Medicare (over 12 percent of all beneficiaries). The conditions include some blood diseases, chemical dependency and related psychoses, and opportunistic infections.
Certain constellations of chronic illness diagnoses may serve as indicators of disability. These diagnosis-related risk factors are used by risk assessment models currently in the vanguard of methods for adjusting health plan payment. Most models rely exclusively on claims data—namely, data on diagnoses rather than functional status—and therefore the adequacy of the performance of risk adjustment methods for people with disabilities will be similar to the adequacy of their performance for populations with any high-cost chronic condition.
RISK SEGMENTATION IN INSURANCE MARKETS AND THE NEED FOR HEALTH-BASED RISK ADJUSTMENT
The need for health care can be influenced by disease, functional status, and socioeconomic factors. Risk selection is defined as the set of processes leading to the selective enrollment of individuals in health plans, which produces risk segmentation. Risk segmentation results from the uneven enrollment of higher- or lower-risk individuals across competing health plans or physician practices, so that some groups attract a disproportionate enrollment of higher-risk individuals. Segmentation is a problem if the payment system does not adjust payment on the basis of enrollee risk.
Risk selection occurs primarily when consumers have a choice of insurance plans or a choice of whether and when to buy health insurance. When individuals can choose among competing insurance plans, those who expect to use a lot of health care services will tend to be attracted to the more gen erous health plans, even when the premium is higher; and individuals with no chronic health care needs will be more likely to choose the lowest-cost plan, even if the benefits are relatively sparse or restrictive. The health plan characteristics that produce this selective attractiveness include benefit design (e.g., coverage of home health care services or mental health services), cost-sharing requirements (e.g., deductibles or co-payments when services are used), and ease of access to specialized services (e.g., no requirements for prior review for the use of specialized services or no requirement to use preferred specialty providers in the provider network) (Batavia and DeJong, 2001). Studies have found evidence of risk selection in a number of settings, including the benefit program for federal employees.
It is conventional wisdom that plans avoid being the only plan in a particular market with an academic medical center in its network, the best mental health benefits, open access to specialists, or richer prescription drug benefits (Knutson and Wrightson, 2002; Kronick et al., 1996). Each of these features is expected to attract those who use health care services more often. The magnitude and effects of risk selection in health insurance have been debated for many years. Although it is undeniable that risk selection occurs, the primary debates are related to its effects and to the means of addressing related problems, primarily those concerning efficiency and equity. Many debates are limited to issues within the bailiwick of particular disciplines. Economists discuss the efficiency and asymmetry of information. For actuaries, advising clients on detecting and avoiding adverse selection is a primary issue. Without summarizing each discipline’s own take on the topic, it is apparent, to those who consider social and health policy, that risk selection in insurance markets generates a high potential for inequity for individuals with chronic and disabling conditions, whose future health care costs will be significantly and predictably higher than those for the general population (Batavia and DeJong, 2001; Kronick et al., 2000).
Therefore, in a market in which health plans compete for individual enrollees and payments to plans are not adjusted to reflect the risk of the enrollees, those plans that attract the healthiest enrollees will generally be more profitable and plans that consistently attract less healthy individuals may not be able to survive. Risk adjustment of payments to health plans is intended to protect individuals from this dynamic.
Medicare Example
Individuals become eligible for Medicare when they reach the age of 65 years or 29 months after they qualify for Social Security Disability Insurance benefits. In traditional Medicare, acute-care services are paid for on a fee-for-service basis, unless the beneficiary chooses to enroll in one of a number of Medicare Advantage plans. These plans operate as insurance plans and receive a monthly premium (capitated payment) for each beneficiary enrolled. Between 1985 and 2000, the premium was based on the U.S. average per capita cost for beneficiaries, for whom the risk is adjusted by age, gender, institutional status, medical enrollment status, and county of residence.
Most studies have found that, in comparison with the established fee-for-service system, the managed care plans enrolled healthier individuals. Although earlier studies—which used limited methods and measures to assess the risk presented by beneficiaries—found little or no risk selection (Eggers and Prihoda, 1982; Hill et al., 2002), later studies, including those that used more direct and accurate risk assessment methods, found strong evidence of positive selection between Medicare health plans and traditional Medicare fee-for-service plans.
For example, Lubitz and colleagues (1985) found that 106 of the 108 health plans studied experienced favorable selection. More recent studies have further reinforced the findings of positive selection (Greenwald, 2000; Greenwald et al., 2000; Zaslavsky and Buntin, 2002). A study by CMS, using the best available version of a claims-based risk assessment tool, found that the relative risk (compared with that for the traditional fee-for-service Medicare population) was “favorable” for all but three plans. Their enrollees ranged from approximately 3 percent to 15 percent favorable selection. Table C-1 presents the average risk for the beneficiaries of a health maintenance organization (HMO) compared with the risk for fee-for-service plan beneficiaries in the same counties.
TABLE C-1
Distribution of Difference Between HMO and Fee-for-Service Mean Risk Factors for Medicare Beneficiaries in 428 U.S. Counties.
Because the premium rates were normalized on the basis of the rates for the Medicare fee-for-service population and because the demographic risk adjustment methods in use during the early years of the Medicare managed care program were poor predictors of risk (r2 = 1 to 3 percent), plans were significantly overpaid compared with the payments expected from budget assumptions. This prompted CMS to introduce a risk adjuster that was based on health data rather than or in addition to demographic data.
The health plan industry resisted the transition for a number of reasons. What was clear was that the vast majority of plans in the program at that time, once CMS used a more accurate risk adjustment, would see their payments reduced (Blumenthal et al., 2005; Weissman et al., 2005).
How did the risk selection dynamic involve Medicare beneficiaries with disabilities? Disabled beneficiaries under age 65 years were eligible for both managed care and the traditional fee-for-service program. Actual enrollment distributions between managed care and the traditional fee-for-service program were similar by age group, but the population over age 65 years originally eligible for Medicare because of a disability was, on average, less prevalent in managed care plans than in fee-for-service plans, although the difference was not large. Although the beneficiary risk levels were much lower for Medicare managed care plans than for the fee-for-service Medicare program, for the vast majority of managed care plans, the prevalence of those enrolled who were eligible for Medicare because of a disability did not differ from that for the fee-for-service program (Greenwald et al., 2000). One explanation for this apparent inconsistency is that the plans attracted the least costly segment of those with disabilities, just as they had with the general Medicare population.
Outside the Medicare program, the Risk Adjustment Impact Study (RAIS) (which was funded by the Robert Wood Johnson Foundation) also found evidence of risk selection. The study found that relative risk scores across competing plans in one state Medicaid program ranged from 0.70 to 1.36. Significant selection bias was found in all programs or markets studied. Had the plans’ payment rates been determined by using traditional demographic risk adjusters rather than the more accurate adjustment method actually used, the plan with the highest risk group of enrollees would have been seriously underpaid and the other plans would have been overpaid. Table C-2 presents the findings of selection bias across competing plans from RAIS.
TABLE C-2
Percent Relative Risk Difference Between High- and Low-Risk Participating Health Plans.
Relevance for Large and Small Employers
Large employer-sponsored health insurance programs offering employees a choice of health plans also introduce the opportunity for risk selection. In their plan designs and in their marketing to employers and to their employees, plans strategically position themselves to avoid a disproportionate share of high-risk enrollees. In response, employers and consultants have devised a number of mechanisms that employers can use to reduce the opportunities for and effects of risk selection.
Keenan and colleagues (2001) also found that only a handful of large employers adopted HBRA between 1998 and 2000. Some economists have commented on the continuing low demand for formal risk adjustment among large employers (Glazer and McGuire, 2000). Many have also expressed the view that formal risk adjustment, such as that used by Medicare and many state Medicaid programs, may not be needed in the employer insurance market. Others suggest that employers may deliberately use the risk selection dynamic to get rid of options with more generous benefits. That is, they let adverse selection operate to price these plans out of existence.
Some employers self-fund their employees’ health care and, through the Employee Retirement Income Security Act, avoid most insurance regulations. Selection issues are less important for these employer plans, unless a self-funded product is offered along with an insured plan. In that case, the issues become similar to those associated with offering more than one insured plan. Specifically, the employer may overpay if the insured plan attracts lower-risk enrollees than the self-insured plan.
Small employers face a particularly difficult set of issues. They are too small to bear the risk of self-insurance and so must purchase products that offer full insurance. Their premiums are determined through a risk evaluation of each employee and dependent by using either past insurance claims information from various sources or questionnaires, or both. A small-group plan is a product offered to a group usually without individual exclusions; that is, the plan usually cannot refuse to insure a given employee within an employee group; however, unlike large-group plans, the rates for small groups are based on the individually projected risk for each covered individual. The group is either accepted or denied by the health plan. (Some states sponsor high-risk pools that accept individuals who have been denied coverage in the market). The total premium for the group is usually the sum of the individual rates for each covered individual in the group. Thus, a very-high-cost employee or dependent can have a major impact on the total premium or on even whether the employer receives an offer of coverage.
Various strategies have been used to create small-employer insurance purchasing groups or pools both to spread the risk across a larger population and to reduce administrative costs. Such pools, many of which have used a form of risk adjustment, have been difficult to maintain. They have been vulnerable to “cherry picking” by health plans, which happens when those plans selectively offer their own small-group products to low-risk employers and attract them out of the larger risk pool. The small-group pools usually welcome all employers, but individual health plans can choose the small groups to which they will offer insurance. In some cases, an insurer will offer its health benefit product to businesses that are also participating in the small-group pool at a rate lower than that of the same product offered by the pool because the pool’s risk may be higher than that of a given employer. This process removes the lower risk employers from the employer pool, thus forcing the pool to raise prices. This selection dynamic often leads to a “death spiral” during which the lower-risk employers increasingly abandon the pool and premiums for the remaining members increase until a sustainable risk pool no longer exists. One major example of such a situation was the demise of PacAdvantage, a small-group insurance pool in California.
Consumer-Driven Plans
In addition, with the advent of so-called consumer-driven products, such as health savings accounts or health reimbursement accounts, combined with health plans with high deductibles, employers are reducing their financial contribution for health insurance and shifting more of the cost of care to employees, primarily by increasing the deductible thresholds for standard benefit programs
When products with high deductibles and health savings accounts are offered to employees along with traditional products with richer benefits, the risk selection opportunity appears to be raised substantially. In making these changes, employers are usually not taking into account the differences in their employees’ health care needs and other circumstances. Studies show some indication that individuals with higher incomes disproportionately selected these less expensive, less rich health plans (Parente et al., 2004). In their longitudinal study of consumer-driven health plans, Parente and colleagues did not detect significant adverse selection or utilization cost dif ferences, but they were quick to point out that the consumer-driven health plan experience is in the early phase and that more research is needed.
One can see that risk selection for employers is ultimately determined by the combination of whom they employ and of the differences in the generosity of the health insurance benefits that they offer their employees. If an employer manages to hire and retain only healthy employees, risk selection is less of a problem. Risk selection is also less of a problem if an employer offers only one plan, although an employer who has more employees with health problems will pay more for insurance (other things being equal) than an employer with few such employees. Concerns about discrimination in the hiring and retention of employees (for example, by the use of health plan data to target employees for layoffs) enter the domain of the Americans with Disabilities Act.
The RAIS study, in addition to surveys of purchasers and health plans, included a survey of all U.S. members of the health section of the Society of Actuaries (SOA) to evaluate the implementation and impact of formal health-based risk adjustment (HBRA) methods that were based primarily on diagnoses (Knutson, 2003).The actuary survey included actuaries who consulted with purchasers and those who worked for or consulted with insurers. It found that actuaries reported that they believed that the use of formal risk adjustment is far less important for employers than for Medicaid or Medicare managed care programs. However, actuarial consultants have reported that the rate of use of these tools is growing as consultants use them to evaluate health plan performance and advise employers on health plan selection and oversight.
Non-Group Insurance
Small employers are dropping health insurance as fringe benefits at the same time that non-group-insurance options are, once again, being considered as a way of extending insurance to those who are not eligible for either public or employer-based insurance. Health plans competing in this market are particularly vulnerable to risk selection problems, for example, when people wait until they develop health problems to buy insurance. As described below, health plans have created a variety of mechanisms to protect themselves.
What happens in this individual insurance market to people with a greater need for health care? Hadley and Reschovsky (2003) reported that the probability of purchasing non-group insurance was 50 percent lower for people in fair to poor health and that premiums were 43 to 50 percent higher for this group than for beneficiaries in excellent health. In some cases, no insurer will cover the former group of individuals, and in other cases, the premium will be unaffordable.
Preventing and Managing Risk Selection
Purchasers and policymakers have devised a number of tools and strategies to try to prevent selection or to mitigate the problems it creates. For example, a study by Palsbo and Post (2003) reported on strategies that state Medicaid programs employ with competing managed care plans that serve Medicaid beneficiaries. These include the use of risk corridors, risk pools, stop-loss reinsurance, and risk classification (risk adjustment). The authors reported that only 12 of the states studied used the same type of state-of-the-art risk adjuster that CMS uses for the Medicare Advantage program. Since publication of the study, however, state policymakers appear to be showing renewed interest in implementing health-based payment for managed care plans.
In addition to risk management strategies, purchasers rely on regulation of health plan rate setting and enrollment to reduce or manage selection in the small group and individual market. Still, most health economists conclude that the best means of addressing risk selection is appropriate risk adjustment.
The views of actuaries on health-based risk adjustment are also informative. Actuaries assess risk and advise on premiums on behalf of purchasers or health plans. The RAIS actuary survey reported that actuaries judged that HBRA reduced the financial impact of selection bias on health plans. Based on a scale of 0 (no financial problem) to 10 (extremely serious financial problem), the rating dropped from 6.8 without HBRA to 3.5 with HBRA.
Half of all actuary respondents thought that the profession would establish a HBRA-related standard of actuarial practice within 5 years. Those with HBRA experience were more likely to believe this (54 percent) than those without HBRA experience (43 percent). More than two-thirds of the actuary respondents (76 percent with direct HBRA experience, 56 percent without experience) thought that HBRA has advanced the actuarial field, and over half (67 percent with experience, 46 percent without experience) thought that more purchasers should implement HBRA. Actuaries with and without HBRA experience now widely believe that HBRA is a useful tool and that it will become a standard of practice.
The RAIS survey of U.S. actuaries included a series of questions about the need for purchaser implementation of HBRA. The actuaries were also asked to imagine that they were advising large employers offering a choice of plans, including Medicaid managed care programs for the disabled, and then indicate how strongly they would advocate the implementation of HBRA for determining payments to health plans or for determining adjustments to employee premium contributions in defined-contribution plans. Sixty-six percent reported that they would “very or somewhat strongly” recommend the use of HBRA for the Medicaid programs, with 21 percent “not sure.” Forty-two percent would “very or somewhat strongly” recommend HBRA for large employers, with 16 percent “not sure.” Thirty-six percent would “very or somewhat strongly” recommend HBRA for employers adjusting employee premium contributions, with 19 percent “not sure.”
The actuary responses were also analyzed for the subset of respondents employed by prominent health plans. Seventy-four percent of actuaries employed by health plans and 40 percent of consultant actuaries would “very or somewhat strongly” recommend the implementation of HBRA in Medicaid programs for the disabled, with 16 and 13 percent, respectively, “not sure,” whereas only 45 percent of actuaries employed by health plans and 47 percent of managed care consulting actuaries would “very or somewhat strongly” recommend the implementation of HBRA for determining payments to plans to a large employer, with 14 and 7 percent, respectively, “not sure.” Thirty-six percent of actuaries employed by managed care organizations and 45 percent of managed care consulting actuaries would “very or somewhat strongly” recommend to a large employer the implementation of HBRA for determining adjustments to employee premium contributions.
LESSONS FROM PURCHASERS WHO HAVE IMPLEMENTED HBRA FOR HEALTH PLAN PAYMENT
What can we learn from the experience of those purchasers who have implemented HBRA for payment? The earlier-cited RAIS study included Medicare Advantage, six state Medicaid programs, a small-employer-group pool, and two state employee benefit programs. The following key findings are highlighted: All purchasers indicated that HBRA had contributed to fairer payments, that HBRA was needed, and that the implementation of HBRA had been worth the effort. All purchasers have had to address health plan resistance to the risk adjustment of their payments, and as one would expect, the resistance came primarily from plans whose members presented a lower-than-average risk.
The survey of participating health plans found that one-third of the plans received increased payments under HBRA, one-third received decreased payments, and one-third received the same payments. The plans were evenly distributed when they were asked if HBRA had a strong positive or a strong negative financial impact on product viability. The plans expressed concern about data issues, such as the quality of the data and the cost of data collection, the financial viability of the product, the financial effects of selection bias without HBRA, and the effort required to implement risk adjustment compared with the benefit. The nature of these concerns generally reflected whether a plan’s revenue had increased, decreased, or remained the same. A significant minority (31 percent) reported that HBRA enabled program participation by some health plans that financially could not have done so without HBRA. A similar number (28 percent) reported that HBRA had caused some low-risk plans to drop the product. Overall, however, the responses of health plans that have experienced payment based on HBRA are positive—even for plans that experienced negative financial results. Health plans reported that HBRA
- was more accurate than traditional methods of rate setting (87 percent),
- produced a fairer payment system (81 percent),
- did not require the collection of unnecessary and costly data (77 percent),
- significantly reduced concern about the negative financial impact of adverse selection (decreased concern by two-thirds), and
- should be part of public health policy for plan payment (79 percent).
Many health plans reported that they used HBRA internally, mostly for provider profiling (46 percent) and product management (66 percent).
The researchers reported that state officials and administrators believed that the process improved the efficiency of the payment programs and gave health plans more incentive to focus on providing quality care as opposed to selection strategies. In February 2002, CMS, in conjunction with the Center for Health Program Development and Management at the University of Maryland—Baltimore Campus distributed a questionnaire to CMS regional offices to assess states’ experiences with HBRA. The questionnaire was distributed to the 40 states that had comprehensive Medicaid managed care programs or primary care case management programs that were not currently using HBRA. Of the 35 states that responded, 28 states (80 percent) reported that they were interested in learning more about risk adjustment, and 23 states (67 percent) reported that they had considered using risk adjustment for their Medicaid programs.
CMS has withstood significant plan resistance to changes in risk-adjustment methods, but it did make some compromises. For example, CMS implemented the Selected Significant Condition risk adjustment model in 2004. CMS is also using this new risk adjustment method in the financing formulas for most of the recent demonstration projects that it has initiated in the traditional Medicare program and in rate setting for its managed care program.
The author is not aware of surveys that have explored the views or experiences of employees or health plan members related to risk adjustment as such, and as far as the author knows, public and private purchasers who use the method do not mention its use in materials provided to employees or plan members. This presumably reflects, among other concerns, the complexity of the methods and the circumstances that prompt their use.
ADEQUACY OF CAPITATION PAYMENT FOR PEOPLE WITH DISABILITIES
A perfect risk adjuster would pay a capitated payment to health plans that exactly fits the actual cost along the entire cost distribution for the population. In other words, there should be no systemic bias; there should be only random error based on sample size. Achieving this would, in principle, make health plan managers less anxious about the risk of enrollees. In fact, such a perfect fit might very well lead managers to desire high-cost enrollees if they could enroll a stable actuarial number because these enrollees would bring much higher revenues per capita; they may also present plans with a greater opportunity to achieve profits by improving efficiency at the health care services level. The incentive for health plans to support selective enrollment strategies is directly related to the extent to which the rate-setting method, with market regulations held constant, overpays for healthy enrollees and underpays for high-cost enrollees. The bigger the gap between paid amounts and actual costs, the more the health plan will be rewarded for focusing on avoiding these high-cost enrollees rather than seeking better ways to provide care for them.
HBRA methods that use direct indicators of disease burden and health status are growing in use. They are much better predictors than traditional demographic methods and have far fewer perverse incentives (e.g., to increase spending in order to increase payments) than rates based on the prior expenditures of an individual or group. However, they as yet may not produce adequate payments to remove plan concern with adverse selection for people with disabilities who have high-cost chronic conditions.
Achieving Payment Accuracy for Populations with Disabilities
For the general population, the predictability of medical expenditures at the individual enrollee level historically has been assumed to be about 6 to 15 percent of the observed variation (Newhouse et al., 1989). Using prior utilization information for Medicaid beneficiaries with disabilities in six states, Kronick and colleagues (2000) produced predictions that ranged from 29 to 51 percent. It is both the large proportion of the total cost accounted for by these beneficiaries and the consistency of health care over time that produces this predictability.
Risk adjustment is important for populations with disabilities (1) when such populations are enrolled in capitated health plans and (2) when the capitated health plan must compete in the mainstream insurance market. Current Medicare policy seems to be moving in this direction; that is, not only toward promoting managed care for people with disabilities, but also toward supporting SNPs that would compete with traditional managed care plans in the mainstream Medicare Advantage market. This trend will expose the limits of even the best risk adjustment methods currently in practical use.
The problem is partly with the current state of the art of risk adjustment. Although current claims and survey-based methods are a vast improvement over traditional rate-setting methods, they do not yet produce the perfect predictor described above. The problem also lies in a political and competitive environment dominated by traditional health plans that have historically enrolled less risky beneficiaries than the Medicare program in general and that have not supported the rapid implementation of improvements in risk adjustment. The SNPs that wish to focus on excelling in attributes that would attract people with chronic illnesses or people who are frail or disabled will want the most accurate risk adjuster possible, whereas medical assistance plans with healthier than average enrollees will receive less revenue with a better risk adjuster because the improved method will reduce overpayments for healthy beneficiaries.
During an examination of the progress of risk adjustment methods, one must be mindful that where risk selection already exists, and given the fact that the vast majority of the population (80 percent) has medical costs below the average per capita cost for the population as a whole, the funding allocation politics can be tricky. On a related theme, it is commonly found in competitive health plan markets that the majority of the plans have relatively positive risk compared with the risk for the pool as a whole, leaving only the one or two health plans with high-cost populations to advocate for better risk adjustment.
Predictive Accuracy of Risk Adjusters
Many evaluations of risk adjustment methods use individual enrollee-level statistical analysis to evaluate the overall predictive performance. Although this approach is valid and useful for the comparison of models, if one is concerned with the adequacy and fairness of health plan payment for people with disabilities, one must look directly at the predictive performance for people with disabilities. It is possible for the risk adjuster to perform materially better for the general population but remain problematic for people with disabilities. This kind of prediction performance evaluation is often represented by predictive ratios that compare predicted payments with actual expenditures for defined subpopulations within a risk pool. A predicted payment ratio of 1.00 is the most accurate. A ratio above that indicates overpayment and ratios below that indicate underpayment.
Evaluations of predictive ratios are of different types, each of which examines the extent to which a method overestimates and underestimates spending for different subpopulations. One approach segments the population by actual medical expenditure levels (by quintiles or deciles) and evaluates the predictive ratio for each segment. Another approach identifies subpopulations, using specific chronic conditions or functional status to evaluate predictive ratios. Finally, some studies create simulated health plans with various proportions of high-risk members (risk selection) and evaluate the predictive accuracy for the simulated plans. In some studies, actual enrollees in actual plans (not simulated) of policy interest have been studied directly (e.g., Program of All-Inclusive Care for the Elderly [PACE] programs). This paper includes findings from all types of evaluations.
Commonly Used Risk Factors Produce Different Levels of Payment Accuracy
Prior Utilization as a Risk Factor
An individual’s prior utilization and related costs have historically been the most predictive of future costs. This has been the basis for what insurers call “experience rating.”
Demographic Measures as Risk Factors
Analysts and purchasers have sought alternatives to simple prior utilization models because payments to health plans on the basis of members’ past use of services creates a potential disincentive for plans to promote the prudent use of health care services. One approach relied on demographic variables, such as age, that are statistically associated with higher levels of use of services. The Medicare example of this approach was the Medicare average adjusted per capita cost (AAPCC). The AAPCC underpaid plans for people with major chronic conditions (who were in the upper quintile of cost) by 50 percent.
Health Status Measures as Risk Factors: Diagnosis Codes and Functional Status
Early work to improve demographic-based methods focused on the use of diagnosis codes combined with age and gender and sometimes included additional risk factors, such as functional status. These early explorations represented a major advance in the field. Some studies found that functional status information alone predicted health care expenditures about half as well as diagnosis data alone. These studies also found that that functional status combined with diagnosis data only modestly improved the predictive performance compared with the predictive performance of models that used diagnosis data only (Fowles et al., 1996; Hornbrook and Goodman, 1996). Even for a Medicare population, functional status data do not add significantly to the overall predictive performance of the model on the basis of diagnosis data.
Diagnosis models included all inpatient diagnoses and the diagnoses for patients in ambulatory care settings and were implemented by a handful of public and private employers as well as some managed care programs and Medicare managed care from 1994 to 2000. The performance of the prominent risk adjusters was evaluated by SOA in 1995 and by other investigators (Dunn et al., 1995; Ellis et al., 1996; Fowles et al., 1996; Hwang et al., 2001; Ingber, 2000; Kronick et al., 1996 Kronick et al., 2000).
With these methodological advances, the underprediction for the upper 20 percent and the overprediction for the low-risk beneficiaries were substantially reduced but not eliminated. In one example, Hwang and colleagues (2001) evaluated the performance of three prominent diagnosis-based risk adjustment methods for predicting the costs for chronic diseases for children enrolled in Medicaid. The diagnosis models significantly outperformed demographic methods overall for children with chronic health conditions. Nonetheless, these models still over-predicted 15 to 31 percent of the costs for children with no chronic conditions. When the percentage of children with chronic conditions reached 20 percent of the enrolled population, the payments to plans overall ranged from 92 to 105 percent of the actual costs. As the proportion of members with chronic disease rises beyond 20 percent, the plan underpayment worsened, dropping to between 84 and 95 percent of the actual costs. If the average cost per member per month (PMPM) for the overall risk pool were $200, with the best-performing diagnosis-based risk adjusters available, the loss per capita for a plan with a disproportionate share of children with chronic conditions would be $10 to $22 PMPM.
In 2001, SOA sponsored another comparison of the predictive performance of prominent diagnosis-based and prescription-based risk adjustment methods used as proxies for diagnosis, using claims and enrollment data from employers (Cumming et al., 2002). Prescription data-only models were found to perform almost as well as diagnosis-based models, and use of the combination of diagnosis and prescription data improved the performance of the model to a limited extent. That study also demonstrated underpayments for the highest-cost segments of the commercially insured population studied. Table C-3 summarizes the findings for commercially insured and Medicare populations.
TABLE C-3
Predictive Ratios for Alternative Risk Adjustment Models.
Many other studies have demonstrated the significant improvement in performance of health-based risk adjusters compared with the performance of previous methods. They have also consistently found a sufficient level of underprediction for the highest-cost segment of multiple populations, including those with disabilities, to raise continued concern about incentives for health plans to avoid high-risk individuals and to create barriers to access or services in mainstream insurance markets. Temkin-Greener and colleagues (2001) found that for a PACE population, the Medicare inpatient diagnosis-based risk adjustment method underpaid for any group from one to five limitations of activities of daily living with predictive ratios ranging from 0.90 to 0.62. Ettner and colleagues (2000) found that for an employer plan population, the diagnosis-based methods underpaid for psychiatric disabilities with predictive ratios of 0.83 to 0.85. Kronick and colleagues (2000) found that even for an exclusively disabled Medicaid population (non-dually eligible and noninstitutionalized), whereas demographic risk adjustment underpaid the upper quintile on the basis of prior costs with a predictive ratio of 0.37, a diagnosis-based model demonstrated substantial improvement but still had a predictive ratio of 0.71.
Ellis and colleagues (1996) concluded that for the Medicare population, a diagnosis-based group of models was a significant improvement over a demography-based model but continued to underpay, on the basis of prior costs, for the upper quintile of individuals. The predictive ratio for a demographic model was 0.48, whereas a model based only on diagnosis improved the accuracy to 0.85, and the addition of prior procedures and hospitalizations to the model left a 10 percent underprediction for individuals in the highest risk quintile.
Fowles and colleagues (1996) found that for a health plan with em ployer and Medicare enrollees, even a modest skewing of the distribution of high-cost enrollees simulating high-, medium-, and low-risk plans resulted in underpayments for the high-risk group by a per capita average of 4.5 percent for individuals under age 65 years and 6.7 percent for individuals over age 65 years when a diagnosis-based risk adjuster was used. Hwang and colleagues (2001) found that for a population of Medicaid children, use of a demographic risk adjuster underpredicted the costs for the upper quintile, with predictive ratios of 0.50, whereas a diagnosis-based model reduced the magnitude of underprediction to between 0.91 and 0.93 of actual costs.
Pope found that the use of a customized model for the Medicare managed care program based on diagnoses resulted in a large improvement in payments over those achieved by use of a previous demographic risk adjustment method. Nonetheless, it still resulted in underpayment for beneficiaries in the upper cost quintile. The predictive ratio for the demographic model was 0.44, and that for the diagnosis model was 0.85.
Mark and colleagues (2003) evaluated the predictive ratios for two diagnosis-based risk adjustment models and for one demographic model for a large employer-sponsored health plan. For the subpopulation of beneficiaries who had diagnoses of chronic conditions associated with a disability (measured by activities of daily living [ADLs]), the demographic model underpredicted costs by 50 percent. The two diagnosis-based models performed very well and provided 1.01 and 1.02 percent of the actual costs.
Thus, for these employers, it appears that the diagnosis risk adjusters significantly reduced the incentive for “cream skimming,” a term that describes plan strategies designed to selectively attract lower-risk enrollees that produce profits.
Medicare Example
The advances in the Medicare risk adjustment method reflect the advances that have been achieved by using health status information in other sectors. In the mid-1980s, a prior hospitalization data-based model for Medicare HMO rate setting was demonstrated. This first demonstration of a diagnosis-based payment system for Medicare used principal inpatient diagnoses and some demographic data (the principal inpatient diagnostic cost group [PIP-DCG method) to replace the demographics-only-based model in use. The demonstration ran from 1986 to 1987 for a Medicare health plan in Minneapolis, Minnesota.
Compared with the AAPCC, the model based on the inpatient diagnosis reduced the underestimation of expenditures for beneficiaries in the top cost quintile from 50 percent to 12 to 15 percent. Temkin-Greener and colleagues (2001) found, however, that although the PIP-DCG model was a major improvement over the AAPCC, it underpredicted the overall costs for PACE programs by 38 percent. That is, it underpaid these plans, which had higher enrollments of individuals with chronic health conditions. For individuals with five limitations in ADLs, the underprediction was 62 percent.
In 2004, CMS introduced a selected condition diagnosis-based risk adjustment model (formally the hierarchical condition category model [the CMS-HCC model]) for health plan payments. This included diagnosis codes from ambulatory care settings and all of the inpatient codes. This selected condition-based model improved the overall payment prediction by about 25 percent, a major gain in overall performance. However, the underprediction for beneficiaries in the upper quintile of expenditures was 12 to 15 percent.
Table C-4 shows the payment accuracy for two capitation methods for Medicare populations in general and also for Medicare beneficiaries with functional disabilities, as measured by the number of ADLs.
TABLE C-4
Medicare Predictive Ratios for Noninstitutional Beneficiaries by Functional Status, Pooled Data, 1991 to 1994.
In 2004, CMS implemented the selected conditions CMS-HCC model, which included up to 3,400 diagnoses from both ambulatory care and inpatient settings. This implementation was achieved despite health plan industry resistance to much of the plan ostensibly over concern with data burden and diagnosis-coding completeness. A compromise reduced the number of diagnosis codes but retained 90 percent of the predictive performance of a full diagnosis-based model. Another critical compromise eliminated the requirement that health plans submit procedure codes. This eliminated CMS’s ability to calibrate the models with managed care data or to determine care patterns of managed care. Therefore, payment models for health plans will continue to be updated and calibrated on the basis of the data for the traditional Medicare fee-for-service program population, and the predictive performance will be somewhat lower than that which would be achieved if all diagnosis codes were included.
To improve the predictive accuracy for frail elderly individuals and for individuals with major chronic conditions, CMS has also evaluated a frailty adjuster for medical assistance plans and for the PACE program using ADLs as risk factors. Kautter and Pope (2004) found that ADLs added an approximately 10 percent improvement in overall prediction when they were combined with diagnosis-based models.
IMPLEMENTATION FACTORS THAT CAN INFLUENCE THE ACCURACY OF RISK SCORES
In addition to the potential level of predictive performance of a risk assessment method, the success or usefulness of a risk assessment method also depends to a great extent on how the model is implemented. Implementation factors that affect the performance of a model include the extent to which the data required are readily available and adequate in quality, whether the chosen model fits the application, how the model was calibrated, and the time lag between diagnosis coding and payment adjustment.
Data Issues
From the perspective of the data used to assess risk, methods can be categorized by their reliance on demographics, prior expenditures, or health data, including self-reported health status. Methods that rely on demographic risk factors, such as age, gender, and program eligibility status, are easy to administer. Data can be collected from eligibility files. In addition, these measures are unrelated to the care process and therefore do not produce incentives to change treatment or coding to maximize the risk scores. Unfortunately, these methods have a poor predictive value at the individual or risk-skewed group level. As stated earlier, an individual’s total prior medical expenditure is a reasonably good predictor of future expenditure. These data can easily be obtained from claims data. However, as noted earlier, the use of past spending to set payments does nothing to encourage the prudent use of health services.
Health status measures, such as diagnoses and prescriptions, are now predictors that are as good as prior use (Ash et al., 2000). They also provide useful medical management information. Plans must obtain diagnostic data from providers either on claims forms or through other means. Once diagnosis-based risk adjustment is introduced, changes in coding patterns are expected. For diagnosis-based methods, the main concern involves diagnoses in the ambulatory care setting. Historically, these codes have not been used as the basis for payment or rate setting. With the advent of HBRA, the result may be an underreporting of diagnoses and can result in underestimated risk-based payments.
Different health plans have different types of data problems. Staff model HMOs, which have historically had limited experience with fee-for-service billing, will have concerns about claims data completeness. Plans with information systems that truncate the number of diagnosis codes per record to less than three diagnoses per encounter may receive less risk credit than they deserve. Data may also be missing because of subcapitation or because of services that may be contracted or “carved” out to a specialty provider whose utilization data may not be integrated with the other claims or encounter data. For example, a common problem is missing mental health data for plans that carve out mental health services.
With HBRA, more complete diagnosis codes or more specific diagnoses can significantly increase the risk score. At the plan level, plans will attempt to capture and retain in their files all codes submitted by providers. At the provider level, when a risk adjustment system is calibrated or normalized on the basis of the existing coding patterns, coding practices may change in the directions of identifying more conditions that can be legitimately coded and providing more specific coding of the severity of conditions. Once a risk adjustment model is implemented, changes in coding practices that produce more complete and more specific coding create the appearance of a higher-risk population compared with the risk for the population used to calibrate the prediction model. The results inflate the total cost for that population. Purchasers will renormalize their models at regular intervals to prevent overpayment due to upcoding and other changes in coding practices.
A particular underreporting coding problem that is likely to disproportionately affect costs for people with disabilities and to result in underestimates is the lack of persistence of recording the diagnosis codes for major and often disability-related chronic conditions in the typical claims histories over time. For example, on the basis of Medicaid claims data from six states for people with disabilities, Kronick (2000) found that for more than 43 percent of those with the code for quadriplegia in Year 1, that important code was not carried over into Year 2, even though the individual had contact with the provider. The lack of year-to-year persistence was found for many major chronic conditions. The code for multiple sclerosis disappeared in Year 2 for 42 percent of individuals with that condition, and the code for ischemic heart disease disappeared for 67 percent of individuals.
Without the diagnosis recorded in the 12-month time frame of the typical risk assessment period applied in risk adjustment, the payment system assumes that no condition exists. Even if there is no direct link between a chronic condition and a high-cost treatment (e.g., quadriplegia), the often high-frequency occurrences of related acute conditions such as urinary tract infections do not get adequate value because, in a typical risk assessment model, without the strong signal (code) indicating the presence of the underlying major condition, these diagnoses of acute conditions do not predict future costs. The incentive for more complete coding produced by diagnosis-based risk adjustment has been shown to begin to alleviate the problem; but to the extent that it remains, it has a significant impact on the risk assessment for people with these major chronic conditions.
Plans with payments that have been adjusted by purchasers by using diagnosis-based risk adjusters, such as those participating in the Colorado and Maryland Medicaid programs, have, in many cases, made significant improvements in collecting the more complete data needed to set plan-level payments accurately.
For some purchasers, diagnosis data availability problems are such a concern that they substitute prediction models that are based on more easily obtained prescription data. Prescription data are timely, relatively accurate and complete for major drugs prescribed in the ambulatory care setting. In addition, these data do not need to be obtained from providers, eliminating a difficult data collection step. However, if prescribing is increased to raise a plan or provider’s risk score, the incentives for efficiency may be poor. Prescription-based risk assessment models generally focus on drugs believed to be nondiscretionary. However, with off-label prescribing and to the extent that discretion remains in prescribing drugs for additional diseases or for less severe or marginal forms of the disease, caution should be exercised when prescription-based models are considered for provider payment applications.
Functional status and perceived health status information derived from surveys have also been evaluated for use as factors for risk adjustment. These studies have shown that significant nonrespondent underestimation bias can influence those results. Gaming or manipulation of surveys is also a concern.
Risk Adjustment Model Design Should Fit the Application
The risk adjustment methods may differ to some extent in the number of conditions that they incorporate. Some use almost all known diseases to assign risk scores. Others exclude minor, acute conditions, under the assumption that these conditions are not relevant to risk selection and do not represent significant per capita costs and that their inclusion may produce a clinically needless proliferation of these codes. If the user of risk adjustment wished to categorize all patients for an evaluation of how primary care providers are managing these frequent minor, acute conditions, for example, then the use of one of the methods that includes these conditions would be preferred.
Another difference is the assignment of disease measures to risk categories. This process may produce categories that are much too heterogeneous for a specific disease of interest. Some conditions are lumped with related, yet clinically quite distinct, diseases with similar costs. A disease such as diabetes, on the other hand, has its own category in most of these methods, and payment is affected by coding for diabetes more specifically. For other conditions, more detailed coding to describe severity will not change the assignment to a risk category, beyond that from the simple identification of the disease.
The approach to assigning individual risk scores also varies. Some methods are additive, with additional payment made for each additional disease category identified. For payment applications, some of these categories may be arranged in hierarchies of related conditions (e.g., pulmonary conditions), with payment made only for the highest-cost category in the hierarchy, the assumption being that the lower-cost categories in the hierarchy indicate complications related to the more significant condition. This approach avoids double counting. Other methods address this relatedness of conditions by assigning individuals to mutually exclusive risk categories derived by interacting all of the individual’s conditions or by identifying the individual’s dominant condition.
The methods have been designed to be as robust as possible to data problems while preserving predictive performance. The models typically require only one occurrence of the diagnosis or prescription in the assessment period to assign risk. The number of times that the same code appears is irrelevant. Discretionary or ill-defined indicators are often excluded or are assigned to risk categories to minimize gaming incentives. This means that data need not be perfectly complete and detailed to be adequate for risk adjustment.
Lag Issues
One concern for the implementation of payment adjustment is the lag between the date that the health problem was coded or that the prescription was ordered and the use of the information for payment adjustment. Lags reduce the accuracy of the payment in two ways: (1) the minimum length of the claims history required to produce a valid risk score will exclude some recently enrolled beneficiaries who do not meet the requirement, and (2) the longer the lag, the greater the loss of predictive power. It is more difficult to accurately predict events in the distant future than those in the proximate future. Lags occur in three ways, including (1) the length of the risk assessment time frame, (2) the time required for claims and enrollment data to be available to a plan or purchaser, and (3) the time required to implement the risk scoring.
A model that uses health data from a prior period to predict payments in a subsequent period will often require a minimum of 6 months of claims history to capture major chronic conditions and comorbidities; therefore, the model requires continuous enrollment throughout the assessment period. In addition, the model will require that the enrollee be enrolled each month in the payment period for the model to perform as well as it was designed to perform. A concurrent model uses health data from the same period to explain costs during that period. This so-called profiling model does not require a lengthy diagnosis history to assign a valid risk score to an enrollee. The prospective approach may not be critical for Medicare, which has a very stable enrollment, but it is relevant for some Medicaid and other programs, in which there is often high enrollment turnover and in which eligibility may terminate after weeks or months because individuals no longer meet the eligibility criteria.
Health plans will have unequal claims run-out periods (periods between the time that an expense is incurred and the time that the claim is processed). It is important to allow sufficient time for all the plans to reach a similar level of data collection completion; otherwise, payments will be biased. For diagnosis data, 4 months or more may be required to be adequate. (Prescription data may lag by only a month or less before they are available to a plan.)
It may require 2 months to receive updates of changes in the eligibility status of plan members from purchasers. For some large employers, the retroactive adjustment for new enrollment, enrollment status changes, or terminations may take even longer.
Purchasers can control how often and how fast they compute and assign risk scores. Combined with the usual claims run-out lag, the range can be from a minimum of 6 months up to a maximum of 24 months.
For individual-level prospective models, the enrollee must be continuously eligible for 6 to 12 months in the assessment period, 6 to 18 months in the claims delay period, and 1 to 12 months in the payment period for a health plan to be paid for the risk of that enrollee. This continuous enrollment requirement can remove up to 40 to 50 percent of any currently enrolled Medicaid population from the clinical condition risk assessment (e.g., all new enrollees), thus dramatically reducing the predictive performance of the total capitation system because the rates for enrollees generally must be based on the much poorer predictors of age and gender. Therefore, it is important to know to what extent the delay has reduced the performance of the model compared with its predictive performance from research studies, which often include no delay.
Model Calibration Issues
To determine that the costs for someone with a major chronic condition are some amount above the average for the population, the risk adjustment models must be normalized on the basis of some actual population. It has been assumed that for the most valid payment, relative values could be achieved only by estimating the weights on the basis of the values for the actual insured population for which payments would be applied. Experience has taught that imported relative weights can be sufficiently valid and stable if they are estimated on the basis of a similar population, for example, Medicare, Medicaid, and commercial populations (with similar covered benefits). For some, however, it may be preferable to calculate weights on the basis of the user’s population. This requires both a sufficiently large population and adequate data. Whether a user imports or calculates its own data, weights must be updated at regular intervals to account for changes in practice patterns, coding changes, and significant changes in benefit design. When one wishes the accuracy to be maximized for the highest-risk segment of the population, small numbers can present a problem in developing stable risk weights.
Although many of the diagnosis-based models are calibrated, of necessity, on the basis of fee-for-service data and although experience has taught that these weights are reasonably valid for managed care applications, there is a desire to move, when possible, to weights based on patient encounter data. There may be some gain in validity from encounter-based weights that reflects the clinical and coding practices of a managed care environment. The use of encounter data for the estimation of weights requires the highest standard for completeness. Although duplications of diagnoses can be tolerated in the risk assessment, duplications of charges could cause significant errors when proper weights are established. Another issue to be considered in the development of weights is how to apply charges to encounter data.
Current risk adjustment models do not directly capture the additional costs that would be incurred to reduce barriers for access to health care. For example, a number of investigators have found that risk models that have introduced factors associated with barriers to health care may predict a lower cost for an individual compared with that for another individual with an identical health status profile but no indicator of a barrier to access.
Typical risk adjustment models, like all traditional rate-setting models, establish payment levels on the basis of current practice patterns. If one wishes to provide incentives to improve the quality of care or to reduce existing barriers to access, features must be added to the payment models. Future research is needed to better integrate quality and disparity related incentive features into risk based payment models.
Implementation Environment
Another important consideration is the environmental context in which HBRA is being implemented. One such issue, especially for employees, is the concern with access to private information. A third party may need to collect and analyze the data. In addition, the payment model may require special calibration for the specific application or population. The model may also need to be updated frequently because the relationship between the risk measure and medical expenditures may change rapidly, for example, as prescribing patterns change and new drugs become available or as the availability of generic drugs reduces the costs for drugs that no longer are protected by patent.
Other factors may also need to be considered. If other major purchasers are using a particular approach, it may be less confusing to the market if the same approach is used. Finally, the cost of licensing and maintaining the software should be taken into account. Prices vary, and some in the public domain may require additional outside consultants for successful implementation.
CURRENT ISSUES AND TRENDS
For programs that want to excel or focus exclusively on health care for individuals with many health care needs, the remaining underpayment bias may still present a daunting financial barrier to provider accountability systems, such as tiered networks, competing in the mainstream insurance market. CMS, as was described above, is attempting to mainstream the financing for many demonstration projects and special programs, many of which serve people with disabilities.
A better understanding of both the underestimation bias and the prediction error associated with individuals with higher health care costs is needed. Rarely is this group addressed explicitly by risk adjustment models. The assumption is that plans will enroll enough of the overpaid (lower-cost) beneficiaries to balance out individuals with higher health care costs. Many times the rationale expressed is that, after all, “they are still insurance companies and the pooling of risk is their job.” Here we confront the often implicit illogical application of insurance assumptions under conditions of chronic, and thus predictable, costs and the desire to finance adequate care by using a per capita budget to allow substitution (prevention) and fiscal restraint (not fee for service).
NEXT GENERATION
The widespread implementation of electronic medical records will provide a new source of clinical data that are feasibly obtainable, such as clinical, hematological, radiological, cognitive, psychological, and other laboratory test results. Such data are expected to improve the predictive performance of risk adjusters, especially in further refining the differentiation of the more severe and complex stages of a condition than the current diagnosis codes or coding practices allow. These studies are only now beginning. For example, Luft and Dudley (2004) have identified 100 verifiable, expensive, and predictable conditions that are good predictors, even from claims data, and that account for a large portion of overall medical expenditures. Also promising is that for many of the conditions, the residual unexplained risk due to limitations of diagnosis coding can be further refined by using the results of single diagnostic tests. This has not been considered feasible for insurance applications; however, with the promise of electronic medical records and the need for accuracy for expensive and deserving populations with the greatest needs, such as people with long-term disabilities, the opportunity may present itself to advance risk adjustment in a significant way. This access to new and richer data may also present the promising opportunity of incorporating quality measures with risk factors to begin the promise of building payment models that address both risk and quality differences.
Eventually, risk assessment will use more sophisticated modeling techniques that more closely fit the dynamics of enrollment length and pentup demand; mechanisms of risk selection (Luft and Dudley, 2004); the behaviors of various health problems from a variable, relevant time frame or episode-of-illness perspective and from the perspective of a longer time frame; and financing models that support 3- to 5-year contracts or payment schemes to reduce the effects of externalities that exacerbate active risk selection and the inability to invest in preventive care.
So far, this paper has not addressed long-term care. The long-term care purchasers have developed a sophisticated case mix and risk assessment process to determine the need for institutional and long-term care and community services, as well as the level of care within a long-term care institution. Those who are working on the integration of Medicare and Medicaid for dually eligible individuals are confronting the remaining problems of risk adjusters described earlier in this paper and are increasingly interested in integrating acute-care risk adjustment with long-term care payments. Usually, a theoretically pure premium rate-setting process attempts to avoid the inclusion of setting of care or services because of a concern of reinforcing the overuse of health care services or constraining creative innovation. However, for some applications, such as health plan integration of long-term care with acute care, the inclusion of setting of care or prior utilization markers may improve the accuracy of the rates because the care delivery patterns are so different when a beneficiary is in an institution than in the community. This is in part because long-term care services may substitute for or prevent acute care utilization.
The integration problem remains, however, for people with long-term, significant chronic conditions when long-term care is a substitute for or prevents acute care utilization or vice versa. This problem is usually ad dressed by calibrating the risk adjustment models separately for populations who are dually eligible or who are in a long-term care facility or by adding an indicator to the risk model. The problem becomes especially difficult when an overlap in the coverage of services exists between both payers and the payers disagree over how many health care services are needed. This has been a major problem for mental health services, which do require a licensed physician and are, to some extent, covered by both Medicare and Medicaid and for which, under managed care plans, utilization controls place limits on mental health services that are more restrictive. The variation in mental health costs for the Medicaid-covered portion for dually eligible mental health services is greater than that for other health problems, many of which are driven by Medicare coverage policy. Now that prescription costs for the dually eligible are Medicare’s responsibility, states may experience the need to coordinate Medicaid coverage around a complex set of formularies for Part D. Under these circumstances the coordination of benefits makes risk adjustment and the rate setting that it supports particularly difficult.
CONCLUSIONS
The use of the best risk adjustment methods for multiple applications, including insurance plan payment, is now common practice. These tools not only produce more accurate allocations of resources than traditional actuarial methods and reduce the level of use of perverse incentives but also offer insights for managers and policy makers about who and what is producing real value, that is, cost-effectiveness, as performance is able to be better differentiated from risk.
The problem is that for individuals with the highest-cost medical needs, the current methods still underpredict expenditures to the extent that concerns about the effects of adverse selection remain, particularly for a plan that wishes to excel in care for those with significant long-term chronic conditions.
REFERENCES
- Ash AS, Ellis RP, Pope GC. Using diagnoses to describe populations and predict costs. Health Care Financ Rev. 2000 Spring;21(3):7–28. [PMC free article: PMC4194673] [PubMed: 11481769]
- Batavia AI, DeJong G. Disability, chronic illness, and risk selection. Arch Phys Med Rehab. 2001 Apr;82(4):546–552. [PubMed: 11295020]
- Blumenthal D, Weissman JS, Wachterman M, et al. The who, what, and why of risk adjustment: a technology on the cusp of adoption. J Health Polit Policy Law. 2005 Jun;30(3):453–473. [PubMed: 16089112]
- Cumming R, Knutson DJ, Cameron BA, Derrick B. A Comparative Analysis of Claims-Based Methods of Health Risk Assessment for Commercial Populations. Washington, DC: Society of Actuaries; May 24, 2002.
- Dunn DL, Rosenblatt A, Taira DA, et al. A Comparative Analysis of Methods of Health Risk Assessment. Washington, DC: Society of Actuaries; December 21, 1995.
- Eggers PW, Prihoda R. Pre-enrollment reimbursement patterns of Medicare beneficiaries enrolled in “at-risk” HMOs. Health Care Financ Rev. 1982 Sep;4(1):55–73. [PMC free article: PMC4191283] [PubMed: 10309720]
- Ellis RP, Pope GC, Iezzoni L, et al. Diagnosis-based risk adjustment for Medicare capitation payments. Health Care Financ Rev. 1996 Spring;17(3):101–128. [PMC free article: PMC4193604] [PubMed: 10172666]
- Ettner SL, Frank RG, Mark T, Smith MW. Risk adjustment of capitation payments to behavioral health care carve-outs: how well do existing methodologies account for psychiatric disability? Health Care Manag Sci. 2000 Feb;3(2):159–169. [PubMed: 10780284]
- Fowles JB, Weiner JP, Knutson D, Fowler E, Tucker AM, Ireland M. Taking health status into account when setting capitation rates: a comparison of risk-adjustment methods. JAMA. 1996 Oct 23–30;276(16):1316–1321. [PubMed: 8861990]
- Glazer J, McGuire TG. Optimal risk adjustments in markets with adverse selection: an application to managed care. Am Econ Rev. 2000;90:1055.
- Greenwald LM. Medicare risk-adjusted capitation payments: from research to implementation. Health Care Financ Rev. 2000 Spring;21(3):1–5. [PMC free article: PMC4194676] [PubMed: 11481749]
- Greenwald LM, Levy JM, Ingber MJ. Favorable selection in the Medicare+Choice program: new evidence. Health Care Financ Rev. 2000 Spring;21(3):127–134. [PMC free article: PMC4194679] [PubMed: 11481751]
- Hadley J, Reschovsky JD. Health and the cost of nongroup insurance. Inquiry. 2003 Fall;40(3):235–253. [PubMed: 14680257]
- Hill SC, Thornton C, Trenholm C, Wooldridge J. Risk selection among SSI enrollees in TennCare. Inquiry. 2002 Summer;39(2):152–167. [PubMed: 12371569]
- Hornbrook MC, Goodman MJ. Chronic disease, functional health status, and demographics: a multi-dimensional approach to risk adjustment. Health Serv Res. 1996 Aug;31(3):283–307. [PMC free article: PMC1070120] [PubMed: 8698586]
- Hwang W, Ireys HT, Anderson GF. Comparison of risk adjusters for Medicaid-enrolled children with and without chronic health conditions. Ambul Pediatr. 2001 Jul–Aug;1(4):217–224. [PubMed: 11888404]
- Iezzoni LI. Risk Adjustment for Measuring Health Care Outcomes. 3. Chicago, IL: Health Administration Press; 2003.
- Ingber MJ. Implementation of risk adjustment for Medicare. Health Care Financ Rev. 2000 Spring;21(3):119–126. [PMC free article: PMC4194670] [PubMed: 11481750]
- Ireys HT, Anderson GF, Shaffer TJ, Neff JM. Expenditures for care of children with chronic illnesses enrolled in the Washington State Medicaid program, fiscal year 1993. Pediatrics. 1997 Aug;100(2 Pt 1):197–204. [PubMed: 9240799]
- Kautter J, Pope GC. CMS frailty adjustment model. Health Care Financ Rev. 2004 Winter;26(2):1–19. [PMC free article: PMC4194868] [PubMed: 25372243]
- Keenan PS, Buntin MJ, McGuire TG, Newhouse JP. The prevalence of formal risk adjustment in health plan purchasing. Inquiry. 2001;38:245–259. [PubMed: 11761352]
- Knutson D. Health-based risk adjustment: payment and other innovative uses. Presented at Robert Wood Johnson HCFO and Kaiser Permanente IHP May 23, 2003 Invitational Meeting; 2003. pp. 1–12. Unpublished manuscript.
- Knutson D, Wrightson W. Financial Strategy for Managed Care Organizations: Rate Setting, Risk Adjustment and Competitive Advantage. Chicago, IL: Health Administration Press; 2002. The Buyer’s Health Care Action Group’s pricing and payment system; pp. 227–238.
- Kronick R, Zhou Z, Dreyfus T. Making risk adjustment work for everyone. Inquiry. 1995 Spring;32(1):41–55. [PubMed: 7713617]
- Kronick R, Dreyfus T, Lee L, Zhou Z. Diagnostic risk adjustment for Medicaid: the disability payment system. Health Care Financ Rev. 1996 Spring;17(3):7–33. [PMC free article: PMC4193611] [PubMed: 10172665]
- Kronick R, Gilmer T, Dreyfus T, Lee L. Improving health-based payment for Medicaid beneficiaries: CDPS. Health Care Financ Rev. 2000 Spring;21(3):29–64. [PMC free article: PMC4194678] [PubMed: 11481767]
- Lubitz J, Beebe J, Riley G. Improving the Medicare HMO payment formula to deal with biased selection. Adv Health Econ Health Serv Res. 1985;6:101–126. [PubMed: 10301333]
- Luft HS, Dudley RA. Assessing risk-adjustment approaches under non-random selection. Inquiry. 2004 Summer;41(2):203–217. [PubMed: 15449434]
- Mark TL, Ozminkowski RJ, Kirk A, Ettner SL, Drabek J. Risk adjustment for people with chronic conditions in private sector health plans. Med Decis Making. 2003 Sep–Oct;23(5):397–405. [PubMed: 14570297]
- Newhouse JP, Manning WG, Keeler EB, Sloss EM. Adjusting capitation rates using objective health measures and prior utilization. Health Care Financ Rev. 1989 Spring;10(3):41–54. [PMC free article: PMC4192960] [PubMed: 10313096]
- Palsbo SE, Post R. Financial risk reduction for people with disabilities in Medicaid programs. Manag Care Q. 2003;11:1–7. [PubMed: 12968436]
- Parente ST, Feldman R, Christianson JB. Employee choice of consumer-driven health insurance in a multiplan, multiproduct setting. Health Serv Res. 2004 Aug;39(4 Pt 2):1091–1112. [PMC free article: PMC1361055] [PubMed: 15230913]
- Pope GC, Kautter J, Ellis RP, et al. Risk adjustment of Medicare capitation payments using the CMS-HCC model. Health Care Financ Rev. 2004;25:119–141. [PMC free article: PMC4194896] [PubMed: 15493448]
- Temkin-Greener H, Meiners MR, Gruenberg L. PACE and the Medicare+Choice risk-adjusted payment model. Inquiry. 2001 Spring;38(1):60–72. [PubMed: 11381723]
- Weissman JS, Wachterman M, Blumenthal D. When methods meet politics: how risk adjustment became part of Medicare managed care. J Health Polit Policy Law. 2005 Jun;30(3):475–504. [PubMed: 16089113]
- Zaslavsky AM, Buntin MJ. Using survey measures to assess risk selection among Medicare managed care plans. Inquiry. 2002 Summer;39(2):138–151. [PubMed: 12371568]
Footnotes
- *
Director of Health Systems Studies, Park Nicollet Institute for Research and Education, Minneapolis, Minnesota.
- INSURANCE PROGRAMS FOR PEOPLE WITH DISABILITIES
- MEDICAL COSTS AND POPULATIONS
- RISK SEGMENTATION IN INSURANCE MARKETS AND THE NEED FOR HEALTH-BASED RISK ADJUSTMENT
- LESSONS FROM PURCHASERS WHO HAVE IMPLEMENTED HBRA FOR HEALTH PLAN PAYMENT
- ADEQUACY OF CAPITATION PAYMENT FOR PEOPLE WITH DISABILITIES
- IMPLEMENTATION FACTORS THAT CAN INFLUENCE THE ACCURACY OF RISK SCORES
- CURRENT ISSUES AND TRENDS
- NEXT GENERATION
- CONCLUSIONS
- REFERENCES
- Risk Adjustment of Insurance Premiums in the United States and Implications for ...Risk Adjustment of Insurance Premiums in the United States and Implications for People with Disabilities - The Future of Disability in America
Your browsing activity is empty.
Activity recording is turned off.
See more...