NCBI Bookshelf. A service of the National Library of Medicine, National Institutes of Health.
Methods Guide for Effectiveness and Comparative Effectiveness Reviews [Internet]. Rockville (MD): Agency for Healthcare Research and Quality (US); 2008-.
This publication is provided for historical reference only and the information may be out of date.
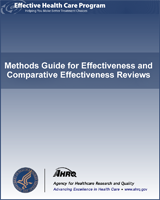
Methods Guide for Effectiveness and Comparative Effectiveness Reviews [Internet].
Show detailsIntroduction
In quantitative synthesis of randomized clinical trials (RCTs) for a comparative effectiveness review, continuous outcomes are usually less straightforward to analyze than binary outcomes. Continuous outcomes are often measured at both baseline and followup time points. Results of continuous data can be reported as means, mean differences, or differences in change score from baseline, and measures of precision are reported as standard deviation (SD), standard error (SE), or confidence intervals. The distribution of the data is not always symmetric, and journal publications may not report all of the information required for meta-analysis.
The original quantitative synthesis chapter of the “Methods Guide for Effectiveness and Comparative Effectiveness Reviews” has a very brief continuous outcomes section that provides limited guidance on using mean difference versus standardized mean difference, but the section does not provide guidance on a number of other issues relating to meta-analysis of continuous outcomes. To fill this gap, this report updates the guidance on quantitative synthesis of continuous outcomes measured in RCTs.
Accordingly, we address the following topics applicable to quantitative synthesis of continuous outcomes measured in RCTs: choice of effect measures of continuous outcomes, choice of estimates for mean difference and baseline imbalance; calculation of SD and SE, how to handle missing data and skewed data, use and interpretation of the standardized mean difference (SMD) and of the ratio of means (RoM) as an alternative measure, and dichotomization of continuous outcomes in meta-analyses.
For each of the topics related to quantitative synthesis of continuous outcomes, we searched for relevant methodological or applied methodological papers in the Effective Health Care Program Methods Library and in Ovid Medline, Current Index to Statistics, and Scopus databases (Appendix A). Recommendations for each topic were then developed based on current knowledge of the literature along with group discussion and consensus. A draft report of the workgroup’s key conclusions and recommendations was circulated for comment to peer reviewers and Agency for Healthcare Research and Quality officers, and those comments were considered by the team in preparing this report. The summary of final key points and recommendations are presented in Table 2 at the end of this chapter.
Table 2
Summary of key points and recommendations for quantitative synthesis of continuous outcomes in comparative effectiveness reviews.
Effect Measures for Continuous Outcomes
The two effect measures most often used for continuous outcomes are mean difference and standardized mean difference (SMD). The choice of effect measure is determined primarily by the scale of the available data: Investigators can combine mean differences if multiple trials report results using the same or similar scales, but SMD is typically used when the outcome is measured using different scales. RoM,1, 2 a recently proposed measure, is an alternative to SMD for outcomes measured using different scales and allows evaluation of the percentage change of a continuous outcome. This section and the next focus on different estimates of mean difference and choice of estimates for mean difference related to baseline imbalance. SMD and RoM are discussed in detail in subsequent sections.
There are several ways to calculate mean difference for continuous outcomes measured at both baseline and followup in randomized clinical trials:
- Use the followup score only to calculate a mean difference between intervention groups.
- Calculate the mean change score from baseline to followup for each intervention group and use the difference in the mean change scores between the intervention groups as the effect measure.
- Use the followup score as the dependent variable in an analysis of covariance (ANCOVA) model to estimate the difference between the intervention groups as the effect measure.
- Use the change score from baseline to followup as the dependent variable in an ANCOVA model to estimate the difference between the intervention groups as the effect measure.
In both options 3 and 4, the variable for the intervention groups is an independent variable in the ANCOVA model, and the baseline score enters the model as a covariate. The coefficient for the variable of the intervention groups provides the estimate for the effect measure, that is, the difference between the two intervention groups. Options 3 and 4 are equivalent statistically in terms of estimating the effect measure. When the variance of the baseline score equals the variance of the followup score, an ANCOVA estimate is the weighted sum of the two estimates from options 1 and 2, and the weight is the correlation between baseline and the followup score.3 If the correlation is greater than 0.5, the difference in change in score from option 2 has more weight; otherwise, the difference between followup scores has more weight. Note that the correlation between baseline and the followup score is generally positive.
It is possible that the observed variance at baseline is very different from the variance of the followup score, and an ANCOVA estimate is not exactly a weighted sum of the two measures; however, the ANCOVA estimate usually lies between the estimates from options 1 and 2. For example, in a study evaluating glycemic control in patients with type 2 diabetes,4 patients randomized to the metformin group have a mean level of hemoglobin A1c of 6.79 percent, and the mean level for the patients randomized to the metformin plus glimepiride group is 6.42 percent. After 20 weeks, the mean level of hemoglobin A1c is 6.86 percent in the metformin group, and 5.68 percent in the metformin plus glimepiride group. For the mean difference between the two groups, options 1 and 2 provide an estimate of 1.18 percent and 0.81 percent, respectively; the ANCOVA estimate is 0.92 percent, located between the above two estimates. The correlation between baseline and the followup score is about 0.6.
Choice of Estimate for Mean Difference and Baseline Imbalance
For an adequately randomized RCT, on average, distribution of baseline characteristics should be similar among intervention groups. However, baseline imbalance often occurs for one or more characteristics. This imbalance could be due to chance, especially in small trials,5 or due to selection bias, often caused by inadequate randomization concealment.6
Assessment of Baseline Balance
Should Investigators Assess Baseline Balance of Included Trials in Quantitative Synthesis?
In the process of quality rating, the balance of baseline scores is one of the factors usually assessed to check the adequacy of randomization, but little attention has been paid to baseline balance in quantitative synthesis. A meta-analysis may have different results depending on whether we adjust for baseline imbalance.7 Here we distinguish between two types of baseline variables. The first reflects the usual patient characteristics and important prognostic factors for the medical condition under study, and the second type reflects the baseline measurements of continuous variables that are specified as outcomes. Both types should be incorporated in quality rating, but the second is more relevant in quantitative synthesis. Quality should be downgraded if the balance of important prognostic factors and outcome variables is not achieved and this imbalance is not addressed in the included studies.
For the second type of baseline variables, investigators should also assess the baseline balance for each continuous outcome and take any imbalance into consideration when conducting quantitative synthesis.
How To Assess Whether the Baseline Scores are Balanced
Though alternative opinion exists,8 for both types of baseline variables the use of statistical testing for baseline difference is generally not recommended for individual studies.9–14 Some argue that such statistical testing “is a test of a null hypothesis that is known to be true,”14 and that it “assesses the probability of something having occurred by chance when we know that it did occur by chance.”12 Even if the statistical tests are not significant, imbalance of important prognostic factors could affect results, and the unadjusted estimates could be biased.
Current practices of using statistical testing for baseline difference vary. In a study of published RCTs in leading medical journals, unadjusted estimates of treatment effects were reported more frequently than adjusted estimates.15 Of the 110 included RCTs, 42 used statistical testing to compare baseline differences. In a systematic review, investigators should base assessments of the baseline distribution on the potential clinical importance of the actual differences between groups and the direction of the imbalance, not on the p-values of tests. An imbalance that favors the control group may have less serious consequences than an imbalance favoring the treatment group. When the decision is not clear cut, we recommend that the investigators take a conservative approach and consider the baseline scores to be imbalanced.
If the baseline scores of the continuous variables specified as outcomes are not reported, investigators should not assume they are comparable even if they consider reported baseline patient characteristics and important prognostic factors to be comparable. If possible, investigators should also consider how attrition may impact imbalances in continuous outcome variables for the subsample with outcome data. For trials with high attrition, the baseline balance may not be maintained in the subsample with outcome data.16 If baseline scores are not reported with sufficient detail to judge whether they are comparable, the investigators should not assume that they are comparable, and this should be appropriately accounted for in quality rating.
If the baseline score imbalance is only by chance, meta-analysis of baseline score differences between treatment groups of included studies should provide a combined estimate close to zero (given no publication bias).7 Investigators are encouraged to do such an analysis.
Choice of Estimate for Mean Difference
When the baseline scores are balanced, options 1, 2, or 3 would provide unbiased estimates of mean difference. The ANCOVA approach (option 3) provides a more efficient estimator with more precision.10, 17, 18 When the baseline scores are imbalanced, options 1 and 2 produce biased effect estimates of mean difference—option 1 simply ignores baseline imbalance, and option 2, contrary to common belief, does not control for the baseline imbalance. The change score is negatively associated with the baseline score and patients with a worse baseline score are more likely to experience a high change score (regression to the mean). For instance, suppose that a trial has an intervention and a placebo group and the intervention group has a worse baseline score. The treatment effect size from the intervention will be underestimated using option 1 and overestimated using option 2.19 When baseline imbalance occurs by chance, the ANCOVA has been shown to be a better method to control for this imbalance, and the estimates from ANCOVA are less biased. When baseline scores are correlated to followup scores, adjusting for baseline using ANCOVA has been shown to remove conditional bias in treatment group comparisons due to chance imbalances11 and to improve efficiency over unadjusted comparisons.11, 18
Choice of Estimate for Mean Difference When There is No or Only Minimal Baseline Imbalance
Estimates from options 1, 2, or 3 could be combined in one single meta-analysis to obtain a combined mean difference. When there is little or no baseline imbalance, we recommend the following for the choice of estimates for mean difference:
- If reported, use an ANCOVA estimate —it is an unbiased and more efficient estimator. When a study does not report ANCOVA estimates, it is possible to calculate them if the studies report: (1) means and SDs at baseline and followup for both intervention and control groups, (2) means and SDs of change for both intervention and control groups, and (3) sample size of both intervention and control groups. However, we recognize that studies rarely report such detailed data and calculating ANCOVA estimates is not usually a practical option.
- If an ANCOVA estimate is not reported and the study directly reported the mean difference or reported enough data to calculate mean difference based on both options 1 and 2, use the estimate with the smaller SE. Option 2, difference in change score, produces a small SE when correlation between baseline and post treatment is high (> 0.5 when variance is equal at baseline and post intervention). Otherwise, option 1, difference between post scores, produces a small SE. There is evidence to show that the correlation between baseline and post score is often greater than 0.5.20 This correlation is often not reported, and Section “Dealing with Missing Data” provides more information on handling the missing correlation.
- If the study reported neither the mean difference nor enough data to calculate the mean difference based on both options 1 and 2, use either the reported estimate or whichever estimate can be calculated from the reported data. Sometimes data needed to include the study in the meta-analysis are missing from the report but can be calculated or imputed from the reported data. For more guidance on handling such situations, see the sections “Calculating Standard Deviation and Standard Error When They Are Not Directly Reported” and “Dealing With Missing Data,” below.
- Since all options provide unbiased estimates, it is appropriate for investigators to use the same estimate across trials. In practice, this advice is limited to options 1 and 2, since ANCOVA estimates are usually not reported consistently. In such cases, some assumptions about missing data are usually needed to obtain an estimate of the same effect measure for all trials. For example, when the change score between baseline and followup needs to be calculated, the correlation between baseline and the followup score is often not known and an assumption about the correlation is needed in order to calculate the SE of change score. For more information about handling such situations, see “Calculating Standard Deviation and Standard Error When They Are Not Directly Reported” and “Dealing With Missing Data,” below.
Choice of Estimate for Mean Difference When There is Baseline Imbalance
When there is baseline imbalance, ANCOVA estimates are preferred over other options as they provide the least biased estimate with more precision. Options 1 and 2 would provide biased estimates. However, trials that are otherwise appropriate for inclusion but lack ANCOVA estimates should not be excluded from the quantitative synthesis, since they still provide valuable information about the study effect. For the choice of estimates for mean difference for each study, we recommend:
- Use ANCOVA estimates if reported (more precision and less bias).
- If ANCOVA estimates are not reported, conduct analyses using both estimates from options 1 and 2 and report the more conservative combined estimate, usually the one with a smaller absolute effect size. Since ANCOVA estimates lie between the estimates from options 1 and 2, the more conservative combined estimate is likely an underestimate compared with the ANCOVA estimate and therefore a better choice for guarding against type I error. If the results from the two estimates do not agree, investigators may also present both combined estimates and clearly explain that the combined estimates are sensitive to the choice of estimate for mean difference. A meta-regression approach7 has been suggested to adjust for baseline imbalance, though its performance has not been fully studied. Investigators may choose this approach as an additional sensitivity analysis.
- If enough trials in a meta-analysis report ANCOVA estimates, investigators are encouraged to conduct subgroup analyses to compare results from ANCOVA versus non-ANCOVA estimates as an additional sensitivity analysis.
Calculating Standard Deviation and Standard Error When They Are Not Directly Reported
Commonly used meta-analysis packages (e.g., Review Manager [RevMan], Stata) require three parameters from each of the intervention groups in order to calculate a weighted mean difference: the mean, the SD, and the sample size. The mean could be the mean change score from baseline or the mean score at followup based on the choice of estimate for mean difference. If any of these are missing, the study will be omitted from the meta-analysis. Alternatively, investigators could use the mean difference between the intervention groups and its associated SE directly in meta-analysis.
Frequently, precision parameters such as SD and SE are not reported directly but may be calculated from other reported statistics. Investigators should always look for reported data that could be used to conduct exact algebraic calculation of these parameters. In this section, we present formulas for calculating SD and SE using other reported statistics. We also briefly discuss the issue of incorporating correlation into calculation of SD for crossover and cluster randomization trials.
Calculation of Standard Deviation and Standard Error Using Available Data
When SD is not directly reported, it can be computed (assuming both mean and sample size are given) from other reported data: SEs, confidence intervals, z- or t-statistics, or exact parametric p-values using available formulas.21 These other reported data could be available for either the mean between baseline and followup from each intervention group or for the mean difference between two intervention groups.
Available Data for One Intervention Group and the Change Scores
In this section, all calculations apply to obtaining the SD for the change score (i.e., the difference between baseline and followup from any one intervention group) when conducting a meta-analysis using three parameters from each intervention group.
If given an SE of the mean change score of one intervention group in a trial of sample size n, the SD for that group can be computed as:
If given a 95% normal confidence interval in the form of (lower confidence bound [LCB], upper confidence bound [UCB]) around the mean, we can compute the SE using the formula:
Formula (1) can then be used to compute SD. If a 90% confidence interval is given rather than a 95% confidence interval, the divisor in formula (2) should be changed to 3.29. If the 95% confidence interval was based on t-distribution, the denominator in the formula must be replaced with the appropriate inverse percentile of the t-distribution multiplied by 2. This could easily be done in Microsoft Excel® by typing in any cell “= tinv(0.05,n-1)” where n is the sample size of the intervention group. If the confidence interval is 90% instead of 95%, replace 0.05 with 0.1.
If given a z-statistic or a t-statistic, for the instance of the change score from baseline in each intervention group, the SE can be computed using the change score:
Again, formula (1) can then be used to determine the SD.
If an exact p-value is reported for testing whether the followup score is significantly different from baseline in each intervention group, the p-value can be converted to a z-statistic first, using the inverse normal value. The easiest way to obtain the z-statistic is by entering “= normsinv(1-p/2)” in any cell, where p is the reported p-value. For example, if the given p-value is 0.03, enter “=normsinv(0.985)”, which returns the z-statistic of 2.17. If the sample size is small and the study obtained the p-value using a paired t-test, then the t-statistic could be obtained by entering “=tinv (p,df)”, where p is the reported p-value and df is the degree of freedom for the t-test and equals n-1, where n is the sample size of the intervention group. Then formula (3) can be used to calculate SE.
If an upper-bound p-value (e.g., p<0.05) is given, then this upper bound can be used with the same formulas to obtain a conservative estimate of the SD.
For calculating SD for the change score, if the SD at baseline (SDb) and followup (SDf) are reported, SD for the change score can also be calculated as:
where r is the correlation between baseline and the followup score. Information about r is often not available and needs to be imputed. For more information on handling missing data for r, see the section “Dealing with Missing Data.”
Available Data for the Mean Difference between Two Groups
If a confidence interval, a z-statistic, or a t-statistic is given for the difference of means between two intervention groups, variations on formulas (2) and (3) can be used to calculate the SE for the mean difference between groups. For formula (3), replace the change score with the mean difference. If an exact p-value for a mean difference is given, it can be converted to a z-statistic using the same Excel “normsinv(1-p/2)” function. If the sample size is small and the study obtained the p-value using a two-sample t-test, then the t-statistic could be obtained by using the Excel function “tinv(p,df)” where p is the reported p-value, but df equals n1 + n2 -2 in this case, where n1 and n2 are the sample size of each intervention group. If an upper-bound p-value (e.g., p<0.05) is given, then the same formulas can be used to obtain a conservative estimate of the SE for mean difference.
In some cases, when the SDs for each intervention group (SDT and SDC for treatment and control groups, respectively) are reported, SE for the mean difference between intervention and control can be calculated as:
where nT and nC are the sample sizes of the two intervention groups. If the estimates of SDT and SDC are similar, one can also use:
Unlike formula (4), there is no need to consider correlation since the intervention groups are independent in a parallel design.
If the individual standard deviations are not given but the SE of the mean difference is presented, this SE can be used directly in the meta-analysis. While this SE is sufficient to determine the precision of the mean difference, some meta-analysis software packages (e.g., RevMan) require the user to input the individual standard deviations. In this case, the simplifying assumption could be made that treatment SD is equal to the control SD, and this computed SD can then be used for both intervention and control groups. This assumption will not affect the final result since the precision of the estimate is determined solely by the given SE, and the estimated SD is only used to re-compute this given SE for the specific software package. The common SD can be estimated as:
Direct use of the SE of the difference in means between groups (and the mean difference) in the meta-analysis or computing the SD of each of the trial group will give the same result. Usually the choice of method depends on the type of data reported in the included trials and the meta-analysis package used.
Occasionally trial authors may confuse standard deviation and standard error. The formulas in this section can be used to verify the values if the study has reported confidence intervals or p-values in addition to the SDs or SEs. In a meta-analysis, if one study has an SD that is much smaller than that of all the other trials and has a disproportionally high weight in the meta-analysis, this can be a red flag that an SE was misreported as an SD.
A Worked Example
Suppose that a parallel study with 15 patients in each group reports the following: “The mean systolic blood pressure in the treatment group was 122.4 mmHG while in the control group it was 134.5 mmHG. This difference was not statistically significant (p=0.24).” If this p-value was computed from a z-statistic, how would we compute the SD?
- Mean difference = 134.5 – 122.4 = 12.1.
- 1−p/2 = 1−0.24/2 = 0.88. Entering “=normsinv(0.88)” in an Excel cell gives a z-statistic of 1.175. Note: If the t-distribution had been used, then the t-statistic = tinv(0.24, 28) = 1.201 where 28 = 15+15−2.
- SE = 12.1/1.175 = 10.298. This number could be used directly in the meta-analysis, or if one is using a software package that requires the SD in each group, it can be computed from this SE:
- This SD can be entered for both treatment and control groups.
Crossover Trials
For trials with a parallel design, the intervention groups are independent of each other, and there is no need to consider correlation between intervention groups when calculating SE for mean difference. A crossover design is one where the participants, in sequence, receive both the intervention and the control and thus all patients are included in both arms of the trial. When a crossover trial is included in a meta-analysis, in most cases, using the methods of a parallel design to calculate SE for mean difference will give an SE that is too large because the positive correlation associated with using the same patients in both the treatment and control groups lowers the variance of the mean difference. The formula to compute the pooled SE for a crossover trial is:
where r is the within-patient correlation coefficient and SEd, SET, and SEC are the difference, treatment, and control SEs respectively. For a parallel trial the value of r is always 0, thus the last term becomes 0. For a crossover study, however, the value of r is usually not reported from the trial and needs to be estimated in order to properly compute the correct SE. See Section “Dealing With Missing Data” for methods for calculating or imputing r.
Cluster Randomized Trials
Cluster randomized trials are similar to crossover trials in that formula (5) or (6) will not provide the correct SE for mean difference. Data among patients within a cluster are usually positively correlated. However, unlike in crossover trials, ignoring this correlation in cluster randomized trials will produce an SE of the mean difference between intervention groups that is too small. If a cluster randomized trial reported an SE that failed to account for this correlation, the simplest way to account for this discrepancy is to compute a design effect (DE) as:
where m is the average cluster size and ICC is the intra-class correlation coefficient. The ICC is defined as the proportion of the total variance (the within-cluster variance plus the between-cluster variance) that is attributed to the between-cluster variance. The square root of the design effect can then be multiplied by the standard error of the regular mean difference (computed as if it were parallel) to produce the adjusted SE. This new adjusted variance will appropriately reflect the loss of precision due to the cluster randomization design.
A Worked Example
For a cluster randomized trial, suppose that the SE of the mean difference is calculated to be 2.4 using the methods for a parallel design. If the average cluster size was 10 and the ICC was estimated to be 0.03, we can adjust the SE for the design effect as:
SEadj = sqrt(DE)*SE=sqrt(1.27)*2.4=2.7
Therefore, 2.7 is the standard error that should be used in the meta-analysis.
The ICC will generally be quite low (less than 0.1) in cluster randomized trials, but it can still have a fairly large effect on the trial variance, particularly when the average cluster size is quite large. Usually this ICC is not reported from the published trials and the investigators need to assume a plausible value to calculate the SE. Investigators should always conduct sensitivity analyses by assuming several values of ICC and checking how robust the results are in comparison with the assumed ICC values. In addition, databases for ICC estimates are available for some outcomes,22–25 and investigators may refer to the relevant literature to check whether the typical magnitudes of ICC for the type of outcome under study have been reported and make assumptions around the typical estimates.
Dealing With Missing Data
Missing data is a common issue in meta-analysis and often leads to biased estimates. Missing data can take many forms: missing studies, missing outcomes, missing summary data, missing individual, and missing study-level characteristics. Missing studies and missing outcomes are complex issues that are not specific to continuous data and will not be discussed here. This section focuses on the issue of missing summary data, which is most relevant to continuous data in the meta-analysis. The issues of missing individuals and missing study-level data will be discussed briefly.
How Are the Missing Data Distributed?
Missing data can be categorized into one of three types based on missing mechanism: missing completely at random (MCAR), missing at random (MAR), or missing not at random (MNAR).26 Data are said to be MCAR if being missing does not depend on observed or unobserved measurements. MAR means that, given the observed data, the reason data are missing does not depend on unobserved data. Data are MNAR if they are neither MCAR nor MAR. Missing data that are MCAR or the more reasonable MAR are considered ignorable in a systematic review. There is no bias in simply performing the meta-analysis without the missing data, and the combined estimate only suffers from less precision.27 Unfortunately, missing data are usually suspected to be MNAR and must be considered. Simply omitting trials with data that are MNAR will lead to biased results.26
Missing Summary Data
If a study is missing data elements that are required in a meta-analysis and these data cannot be calculated from reported data, it is often a good idea to contact the authors to obtain the missing values before conducting the analysis. If it is not possible to obtain the missing values, investigators need to either exclude the study or impute the missing data in some way. Both omitting a study and imputing for missing values can result in bias and under-precision, but it is generally accepted that omitting studies should be avoided when possible.
Standard deviation is the most commonly missing parameter. We recommend that studies missing only SDs should not be excluded, as this could lead to a biased combined estimate. For example, studies with nonsignificant results were more likely to omit standard deviations.
Imputation of Standard Deviation
If the data are not available in an alternative form that allow direct calculation, imputation of missing values is often recommended, based on results from simulation studies.28 Several simple methods have been suggested for directly imputing missing SDs, including direct substitution using the largest SD of the included studies, arithmetic means,29 linear regression,30 coefficient of variation,31 and imputation from correlation.28 We demonstrate some of these methods using the following example, taken from a review comparing asthma patients using long-acting beta agonist (LABA) and inhaled corticosteroid (ICS) in combination versus using ICS alone.32 The outcome is pulmonary function in L/min.
The studies labeled Strand and SAM40036 are missing their SD and are not counted in the final meta-analysis (Figure 1).32 A direct substitution of the largest SD shows that the largest SD in the LABA/ICS group is 52.14 and in the ICS group is 49.64 (Figure 2).
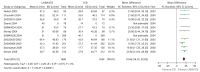
Figure 1
Results of meta-analysis of pulmonary function without including studies with missing data.
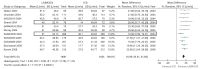
Figure 2
Results of meta-analysis of pulmonary function, imputing missed data using direct substitution.
Alternatively, investigators could use the arithmetic means of the SDs in each group. That is, for the LABA/ICS group, take (46.2 + 51.14 + 45.8 + … + 40.58)/9 = 45.28. This results in 43.47 for the ICS group. Using these values for the two missing studies yields similar results to imputing using the maximum (Figure 3).

Figure 3
Results of meta-analysis of pulmonary function, imputing missed data using arithmetic means.
To use average coefficient of variation (CV) to impute, investigators need to first calculate a CV for each study. CV is defined as SD/mean. For example, for the Nelson study, CV=46.2/51.5 = 0.897. Computing CV for each study and then taking the average gives 0.921 for the LABA/ICS group and 1.527 for the ICS group. To estimate the SD for studies with a missing SD, use these values and the formula SD = CV*mean. In this case, for the Strand study, the mean is 40 in the LABA/ICS group, and the estimate of SD is 40*0.921 = 36.84. Using this method gives similar results to the previous two methods (Figure 4).
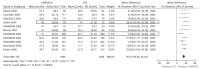
Figure 4
Results of meta-analysis of pulmonary function, imputing missed data using coefficient of variation.
More complex methods for calculating a weighted mean difference directly in the presence of missing SD data include sample size weights,33 bootstrap methods,34 multiple imputation methods,35, 36 the interval method,37 and the prognostic method.37 These methods are complex and don’t permit the creation of a standard forest plot. While these methods may yield more accurate accounting of the true variance in the meta-analysis, this has yet to be fully evaluated. Other work has been done taking into account the uncertainty of the SD when it is imputed.38, 39 A full accounting of these methods is beyond the scope of this paper and investigators are encouraged to look more into each of these methods themselves. There is not yet enough evidence to indicate the relative performance of the various approaches, though there is some evidence that the method chosen may not make a meaningful difference.21, 40
To summarize, investigators should always try to contact authors to request exact estimates. Studies missing only SDs should not be excluded as this may lead to a biased combined estimate when studies with nonsignificant findings are more likely to omit SDs. If exact estimates cannot be obtained, imputation using one of the methods described above should be conducted. Direct substitution using the largest SD is the simplest method and the most likely to lead to a conservative estimate. However, if one is comfortable with one of the more complex methods, using it may lead to a more accurate estimate of precision parameter and is encouraged. No method has been shown to be absolutely superior to any other, so it is most important that the reviewer choose a valid method with which they are comfortable. Investigators may choose to use alternative imputation methods in a sensitivity analysis to determine how robust the results are with respect to the different imputation methods. It is also recommended that investigators report which studies had imputed SDs and which method(s) was used to perform the imputations.
Missing Correlations
To calculate the SD for change from baseline when meta-analyzing change from baseline scores, the correlation between baseline and followup scores is required in addition to the SDs for baseline and followup scores. This information is often not available from trial reports and has to be imputed.
The first option for imputation is to use estimates of correlation from other similar studies included in the same meta-analysis. If a study gives the SDs for both individual scores as well as for the change score, one can compute the correlation (r) using the following formula (which is a rearrangement of formula [4]):
where SDb, SDf, and SD represent the SDs for baseline, followup, and change scores, respectively. This correlation can be used as an estimate of the correlation in studies where the SD for change scores is not available but the SDs for baseline and followup scores are available.
If it is not possible to compute a correlation from any of the included studies, one can either estimate it from historical data or use an approximate value. In the latter case, the most common value to use is 0.5.29 This can be considered a conservative estimate when using the change scores from baseline. A recent study20 showed that the median correlation for change from baseline among trials included in systematic reviews was 0.59 (interquartile range [IQR]: 0.40, 0.81). A correlation less than 0.5 would make using followup scores generally more efficient than using the change scores from baseline. Thus if a trial author used the change scores from baseline, we can assume the correlation was at least 0.5. As in the case of missing SDs, investigators can always conduct sensitivity analyses by assuming several values of correlation.
The methods described here can also be used for dealing with crossover studies, in which case r would be calculated by rearranging formula (8).
Missing Individuals and Missing Study-Level Characteristics
Individuals missing from a study due to withdrawal and other reasons create an issue at the study level more than at the meta-analysis level. While missing individuals will also affect the results of meta-analysis, it is very difficult to deal with at the meta-analysis level without access to individual patient data. Nevertheless, three methods have been proposed to account for missing patient data: reweighting by completion rate, incorporation of the completion rate into a Bayesian random-effects model, and inference based on a Bayesian shared-parameter model (including the completion rate).41
Missing study-level characteristics will not affect the overall meta-analysis but can affect or even prevent subgroup analysis and meta-regression. Bayesian methods have been suggested to account for missing study-level data during meta-regression,42 but these issues are complex and do not specifically pertain to continuous data. No particular methods are recommended, and investigators may try the methods outlined above for exploratory purposes.
Dealing with Skewed Data
Most meta-analytic techniques for continuous data are based on the mean of the variable of interest, for example, a clinical outcome and a measure of dispersion. If the variable’s distribution is asymmetric, then the data are classified as skewed.
Meta-analytic methods based on means provide correct inference when the individual studies have sufficiently large sample sizes regardless of the variable’s distribution due to the Central Limit Theorem, or when the variable of interest is at least approximately normally distributed.43 However, if neither the sample size is sufficient nor the variable of interest is approximately normal, ignoring variable skewness or treating skewness inadequately can result in misleading conclusions. We know of no comprehensive survey or simulation study addressing the range of possible results of ignoring skewness. However, several examples are available that demonstrate the effects. For example, Ziguras et al.44 compared two meta-analyses of interventions to reduce alcohol consumption, one of which excluded skewed data and one of which did not. The difference in handling skewed data was discussed as one of the reasons that the two analyses produced different results. Shen et al.45 provided an example regarding the relationship between hospital ownership and financial performance in which disregarding skewness produced misleading results.
Typically, an individual study would report nonparametric summaries such as the median and interquartile range if the variable’s distribution is not symmetric. However, the variable of interest may be suspected to be skewed and yet an individual study will report parametric summaries, that is, the mean and SD (or SE or variance). Alternatively, for variables with a skewed distribution, an individual study may transform the data and present either summary statistics on the transformed scale or different statistics, for example, the geometric mean, on the raw (original) scale.
Assessing Skewness
When nonparametric summaries are reported in individual studies, the study authors often have evidence of skewness in the data. Thus, prior to beginning analysis, we recommend that the meta-analyst carefully consider the distribution of each variable of interest and assess whether the distribution may be skewed. This assessment should be based on substantive knowledge of the variable and prior data, if available. For example, utilization and cost variables are often skewed due to a subpopulation of users with no use, and thus no cost, and a few individuals with very high use and hence high cost. When median (or mean) with IQR or range are reported, some idea about the distribution usually can be gained. The two end points of IQR and range are not symmetric around median (or mean) if the distribution of the data is skewed. Altman and Bland46 also provide two useful checks for skewness. If the mean is smaller than twice the SD in each intervention group, the data are likely to be skewed. If there are data from several groups of individuals, and the SD increases as the mean increases across these groups, this indicates that the data are positively skewed. However, data needed for the second check for skewness often may not be reported in the individual studies.
Using Nonparametric Summaries Assuming Symmetry
If symmetry is assumed, nonparametric statistics like medians, ranges, and interquartile ranges can be used to estimate both means and SDs. These nonparametric summaries are only estimates of the true parameters, unlike the direct calculations in the section “Calculating Standard Deviation and Standard Error When They Are Not Directly Reported.” Depending on sample size, different nonparametric summary methods have been used to obtain means from either the median or the range and SDs from either the range or the interquartile range.21, 23, 47
The median is similar to the mean when the variable distribution is symmetric. Thus, if an individual study reports the median for a variable of interest, the median can be used in place of the mean to calculate the mean difference. Most past analyses have used a simple direct substitution of median, but there is a recent study 47 showing that if the range (i.e., the minimum [a] and maximum [b] values) are given, a better estimate of the mean for sample sizes less than 25 is:
while the median itself remains the best estimator for sample sizes greater than 25.
For estimating SD, the most common practice has been to simply compute it from the range or IQR. IQR indicates the length of the interval between the 25th percentile and 75th percentile in which the central 50 percent of the sample values of the variable lie. In these situations, SD can be estimated as IQR/1.35 or as range/4. Hozo47 suggested that range/4 should be used for sample sizes between 15 and 70, while range/6 should be used for sample sizes greater than 70. For sample sizes smaller than 15, the formula below can be used to estimate SD:
Since range is inherently dependent upon sample size, Wiebe21 suggests that the table below reproduced from Pearson48 (see Table 1) should be used to impute SD from range. The SD can be determined simply by dividing the range by the given divisor (which represents the percentage limit for the distribution of the range in a normal population).
Look up the sample size on Table 1 and use the given divisor. For example, if the sample size is 22, then SD could be estimated as range/3.819. It should be noted that Table 1 assumes that the sample data is drawn from a normal distribution. Investigators should use it only when the distribution of data is at least symmetric.
Dealing with Skewness
If skewness is suspected, and individual studies present nonparametric summaries, one can estimate the mean and SD and proceed with usual meta-analysis methods using the resulting estimates. This approach works if the skewness is at most moderate, for example, when the variable of interest has a symmetric distribution in most included studies but shows some skewness in others. However, in the case of significant skewness, for example, when the distribution of the variable of interest is consistently skewed across studies, we recommend transforming the summary statistics of the variable of interest to reduce skewness. An additional advantage of such a transformation can be increased clinical interpretability.43 Generally a logarithmic transformation is used, particularly when the data are economic in nature. Some studies may report summaries on the logarithmic scale; the antilog of the mean of the log data is the geometric mean. Alternatively, study may present the geometric mean on the raw (original) scale alongside its confidence interval or SE. Investigators cannot combine summaries on the raw scale with summaries on the transformed scale. Higgins et al.43 present methods for transforming between different scales which allow the meta-analyst to determine whether to conduct the meta-analysis on the raw scale or on the log-transformed scale as appropriate. Issues to take into consideration when choosing the scale include, for example, which scale was most commonly used across the individual studies.
Some recent research focuses on conducting nonparametric meta-analysis. For example, Ma et al.37 discussed a nonparametric method that utilizes U-statistic theory. Such nonparametric approaches would obviate the need for distributional assumptions, be they normality or symmetry, but may be statistically inefficient. Other authors propose using a ratio of geometric means to analyze skewed continuous data;49 however, the lack of clinician experience with geometric means may make such methods difficult to implement. Investigators may choose to explore these methods and compare them with the results of their primary analysis.
Standardized Mean Difference
For continuous outcomes, different studies in a meta-analysis may use a variety of instruments on different scales to assess the same outcome. For example, included trials might use the Beck Depression Inventory, the Geriatric Depression Scale, and the Center for Epidemiologic Studies Depression scale to measure depression. If these instruments are sufficiently similar to suggest that they are truly measuring the same outcome, standardized mean difference (SMD), a measure of effect size, could be used to combine the studies using different scales. In this section, we discuss the choice and interpretation of SMD estimates and offer caveats on using SMD.
Choice of Standardized Mean Difference
Commonly used estimates of SMD include Cohen’s d, Hedges’ g, and Glass’Δ,50, 51 which are all calculated by dividing the mean difference by the SD. The difference between the effect measures lies in the denominator: Glass’ Δ uses the estimate of the SD from the control group:
and the estimated variance for Glass’ Δ is given by
Cohen’s d divides by the maximum likelihood estimate of the common population SD, calculated as:
where T − C is the mean difference between the two intervention groups and SDT and SDC are the standard deviations of the two intervention groups.
Hedges’ g uses the pooled sample SD, calculated as:
The estimated variance for Cohen’s d and Hedges’ g is given by
and
respectively.
All three effect measures are biased to estimate the population standardized mean difference, and the bias can be more than trivial when the sample sizes of both intervention groups are small. Durlak51 suggested that the positive bias “amounts to a 4 percent reduction in effect when the total sample size is 20 and around 2 percent when N = 50.” Hedges 52 provided a formula to correct for this small sample bias for Hedges’ g and to serve as an unbiased estimator of the population SMD:
where Γ(.) is the gamma function. The estimated variance for Hedges’ gadj is given by
Under the equal variance assumption, Cohen’s d and Hedges’ g are more precise estimators than Glass’ Δ, and Hedges’ g has smaller sample variance than Cohen’s d.
Hedges’ unbiased estimator should be used whenever possible, especially when the sample sizes are smaller than 20. For sample sizes greater than or equal to 20, Hedges’ g is generally preferred over Cohen’s d or Glass’ Δ. When sample size is large, the difference between Hedges’ g and Cohen’s d is small and they can be used interchangeably. When variance across the groups differs and the control group may be a more accurate estimate of true population variance, Glass’ Δ is preferable. Sensitivity analyses are recommended to check how the results differ between using Hedges’ g and Glass’ Δ.
Interpreting Values of Standard Mean Difference
In theory, SMD can be any number, positive or negative. SMDs of 0.2, 0.5, and 0.8 are suggested corresponding to small, medium, and large effects 53 and widely used, although they are not defined in meaningful clinical contexts. Conclusions about clinical importance of the differences are often not clear using SMDs.
We recommend that investigators consider back-transforming the combined SMD to the original scale to facilitate assessing the clinical importance of combined SMDs and to aid decision making. Back-transforming can be done by multiplying the SMDs by the SD of the original scale derived from the population representative studies. Since data from more than one scale are combined, investigators need to choose an SD for each scale they plan to back-transform. The standard deviation chosen for the back-transformation could be pooled from the individual studies included in the meta-analysis as long as they all use the same original scale, or from representative studies using the same scale. Whichever approach is taken, researchers are cautioned that back-transformation should only occur for the summary estimate of effect size and not for effect size results from individual studies, due to possible differences in variability across studies (Chapter 12, section 6).27 The back-transformed mean difference should be evaluated for clinical importance according to evidence-based definitions of minimum clinically important differences.
A Worked Example To Illustrate Back-Transformation of the Pooled SMD
In a CER looking at the effectiveness of treatment in preschoolers at risk of attention deficit hyperactivity disorder (ADHD),54 a meta-analysis was conducted to summarize the benefit of parent behavior training (PBT) for disruptive behavior disorder (DBD) in eight “good” quality studies. The outcome was the measured change in parent-rated child behavior, and scales used to measure the child disruptive behavior included the Eyberg child behavior inventory (ECBI), parental account of childhood symptoms (PACS), and reports of ADHD symptoms. The meta-analysis yielded a combined SMD of −0.68 (95% CI −0.88, −0.47), which corresponded to a medium effect size and indicated that PBT improved parent-rated child behavior in preschoolers. The original CER did not do back-transformation of SMD.
Four studies included in the meta-analysis used (the intensity subscale of) ECBI, and the SDs for the mean difference between PBT and the control groups were similar across studies, ranging from 33.0 to 36.8. To back-transform the combined SMD to the ECBI scale, as discussed above, the SD could be pooled from these four studies or from a representative study. If we take the second approach and consider the largest study, which has a SD of 36.8, to be a representative study then the back-transformed mean difference is −25.0 (95% CI −32.4, −17.3) on the ECBI scale.
Two studies included in the meta-analysis used PACS. One study had a sample size of 50 with a SD of 6.07 for the mean difference, and the other study had a sample size of 30 with a SD of 7.53 for the mean difference. If we use the pooled SD from the two studies to back-transform the combined SMD, the pooled SD could be calculated as , and the back-transformed mean difference is −4.5 (95% CI −5.9, −3.1) on the PACS scale.
Caveats on Using Standard Mean Difference
Synthesis of multiple scales adds complexity to the use and interpretation of SMD. Here are a few caveats investigators should consider when using SMD.
Sample variance heterogeneity. Some studies have identified bias associated with using SMD in heterogeneous studies and studies with large SD.55 Inverse variance weighted SMD could produce a biased estimate of the mean SMD since the weight is a function of the observed SMD. Because the SMD is greatly influenced by the SD, factors affecting the SD will affect the SMD. Though SDs are not directly comparable when different measurement scales are used, if there are meaningful differences in variance across studies due to factors such as different inclusion criteria (e.g., one study includes only severely depressed participants, while another includes participants with mild, moderate, and severe depression), especially for the subset of studies using the same scale, then these differences in variance due to populations will affect the SMD.
The bias associated with the use of SMD is small when the true variance is small relative to the effect being estimated.55 However, investigators should examine sample variance heterogeneity when combining SMDs across studies and evaluate how these differences could affect the meta-analysis. In studies using the same scale, this can be accomplished by doing subgroup analyses based on the magnitude of the SD. Subgroup analyses can also be done by grouping studies according to inclusion criteria. For example, in each subgroup, only SMDs from homogeneous populations should be combined (e.g., combining all studies limited to severely depressed participants, and comparing results to those from studies including mildly or moderately depressed samples). If subgroup analyses suggest that results differ, then SMDs should not be combined across all studies with heterogeneous populations.
Covariates. Studies may account for the effect of covariates. When combining SMDs, SMDs calculated using the unadjusted mean difference56 should not be combined with SMDs adjusted for covariates if there is heterogeneity between the two sets of SMDs. For SMDs calculated from mean difference adjusted for covariates, investigators should consider combining only results with a similar degree of adjustment (e.g., adjusted for similar covariates) to ensure comparable effect size across studies. Otherwise, the combined estimate may be biased. If a study uses balanced groups based on important covariates (e.g., if it has achieved balance through adequate randomization), and another study adjusts for these same covariates, these two studies could be considered as having a similar degree of adjustment and could be combined in a meta-analysis.
Directionality. Note that the direction of the scale must be consistent across the scales used in the included studies. For example, if in one study a high score indicates depression and in another study a low score indicates depression, then one of the scores must be reverse-coded to account for scale direction differences. Investigators should assure that scales are converted to a consistent direction of effect across all studies when calculating SMD.
Missing standard deviation. Information from the SD is required when calculating SMD. When the SD is missing, investigators can use imputed SD; Furukawa et al.57 showed that studies using imputed SDs produced similar results to studies using known SD values. Furukawa et al. also discussed how imputing SD applies to SMD, and more information on imputing SD is provided in the above section “Dealing with Missing Data.”
Multiplicity of data. Studies often report data from outcomes based on multiple measures from multiple time points, an important source of possible bias in meta-analysis.58 For example, one trial may assess an outcome using five measures assessed at three time points; the results may be published in four separate articles. Investigators should establish a priori inclusion criteria regarding which outcomes and time points should be used in a meta-analysis and make sure that all outcome measures meeting inclusion criteria are included. Outcome measures should not be excluded on the basis of statistical significance, direction of effect, or magnitude of effect, since such exclusions would result in selection bias. Investigators must also make sure that only one outcome measure is included in a single meta-analysis. Sensitivity analyses may be conducted to assess the impact of the different measures (for the same outcome) on the combined estimate. In addition, investigators should note that the multiplicity of data is a potential issue for all continuous outcomes. This applies to other effect measures, including mean difference and RoM.
Ratio of Means
Mean difference or SMD have been the most commonly used measures in meta-analysis for continuous outcomes. Recently, RoM1, 2 was proposed as an alternative. This measure offers the advantage that it can be used regardless of the units used in the individual trials. As with SMD, RoM can be used to combine outcomes that are measured using different scales. RoM can be interpreted in terms of the percentage change of the intervention group from the control group.
The RoM is calculated by dividing the mean outcome value from the intervention (or treatment) group (T) by the mean outcome value from the control group (C). For meta-analysis, the natural logarithm of each trial’s RoM and its SE are calculated using the mean values, number of participants (n), and SD in each group2 as:
Then the natural logarithm transformed ratios are combined across studies using the standard inverse variance method. A combined ratio and its 95% confidence interval could be obtained by back-transforming the combined log-transformed ratio and its 95% confidence interval:
This method can be employed using a free meta-analysis software package called COMPARE2.59
RoM has a straightforward interpretation and expresses the percentage change in the mean value of the intervention group relative to the control group. The results are in a relative form similar to the risk ratio: For example, if the combined RoM is 1.15, it means that the mean of the intervention group is 15 percent higher than the control group; if the combined RoM is 0.85, then the mean of the intervention group is 15 percent lower than the control group.
In simulation studies,2 RoM has shown comparable statistical performance to mean difference methods in terms of bias, coverage probability, and statistical power. Overall, the data suggest that RoM is a reasonable alternative. Further data from an empirical analysis of 232 clinically diverse published meta-analyses1 have confirmed the findings of simulated data, and this study suggests that, on average, RoM produces similar effect estimates, and SMDs of 0.2, 0.5, and 0.8 corresponded to increases in mean of 8, 22, and 37 percent, respectively. There was less heterogeneity in meta-analyses using RoM compared with mean difference but more compared with SMD.
Several meta-analyses have used RoM.60–63 One study62 utilized the RoM method when included studies reported various units of dosing for analgesics for a meta-analysis of total analgesic used within a postoperative period. Traditional methods would require standardizing all analgesic doses (i.e., conversion to “morphine equivalent”), which was not possible in all cases since not all analgesics have a reliable equivalence ratio. The treatment effect of cumulative analgesics used was therefore expressed as RoM in the experimental versus the control groups.
In summary, RoM appears to be a reasonable alternative to the traditional effect measures of continuous outcomes based on empirical evidence. Therefore, investigators may choose RoM as an effect measure when appropriate. When the outcome is assessed using different scales, RoM is easier to interpret than SMD. RoM has no units and allows for pooling of the studies expressed in different units; RoM also facilitates comparisons regarding relative effect sizes across different interventions. On the other hand, investigators should note that RoM can only be used in scenarios where the mean values of the intervention and control groups are both positive or both negative. Caution is warranted when RoM is used for small trials with large SDs and large effect sizes. Similar to the limitation of SMD for small trials, the combined estimate of RoM biases towards no effect, and this bias is accentuated by high heterogeneity.
Dichotomizing Continuous Outcomes in Meta-Analyses
For some continuous outcomes, a meaningful clinically important change is often defined, and patients achieving such change are considered as “responders.”64 There are methods developed to convert effect measures for continuous outcomes to effect measures of binary outcomes;65, 66 however, understanding the relationship between continuous effect measures and proportion of “response” is not straightforward. The assumptions used to assess such relationships are usually difficult to verify,66 and the results could be sensitive to underlying assumptions.65 Further research is necessary, and we currently recommend against inferring response rate from a combined mean difference.
Conclusion
In this report, we have provided recommendations on relevant topics applicable to quantitative synthesis of continuous outcomes measured in RCTs. The key points and recommendations for each topic are summarized in Table 2. Investigators are encouraged to follow these recommendations to improve the quality, transparency, and consistency of quantitative synthesis. The recommendations will be updated with the development of new research and methods, and new topics will be added when needs arise.
References
- 1.
- Friedrich JO, Adhikari NK, Beyene J. Ratio of means for analyzing continuous outcomes in meta-analysis performed as well as mean difference methods. J Clin Epidemiol. 2011 May;64(5):556–64. [PubMed: 21447428]
- 2.
- Friedrich JO, Adhikari NK, Beyene J. The ratio of means method as an alternative to mean differences for analyzing continuous outcome variables in meta-analysis: a simulation study. BMC Med Res Methodol. 2008;8:32. [PMC free article: PMC2430201] [PubMed: 18492289]
- 3.
- Senn S. Baseline distribution and conditional size. J Biopharm Stat. 1993 Sep;3(2):265–76. [PubMed: 8220409]
- 4.
- Charpentier G, Fleury F, Kabir M, et al. Improved glycaemic control by addition of glimepiride to metformin monotherapy in type 2 diabetic patients. Diabet Med. 2001 Oct;18(10):828–34. [PubMed: 11678974]
- 5.
- Rosenberger W LJ, editor. Randomization in Clinical Trials: Theory and Practice. New York: Wiley; 2002.
- 6.
- Schulz KF, Grimes DA. Allocation concealment in randomised trials: defending against deciphering. Lancet. 2002;359(9306):614–8. [PubMed: 11867132]
- 7.
- Trowman R, Dumville JC, Torgerson DJ, et al. The impact of trial baseline imbalances should be considered in systematic reviews: a methodological case study. J Clin Epidemiol. 2007 Dec;60(12):1229–33. [PubMed: 17998076]
- 8.
- Berger VW, Weinstein S. Ensuring the comparability of comparison groups: is randomization enough? Control Clin Trials. 2004 Oct;25(5):515–24. [PubMed: 15465620]
- 9.
- Roberts C, Torgerson DJ. Understanding controlled trials: baseline imbalance in randomised controlled trials. BMJ. 1999 Jul 17;319(7203):185. [PMC free article: PMC1116277] [PubMed: 10406763]
- 10.
- Senn S. Testing for baseline balance in clinical trials. Stat Med. 1994 Sep 15;13(17):1715–26. [PubMed: 7997705]
- 11.
- Senn SJ. Covariate imbalance and random allocation in clinical trials. Stat Med. 1989 Apr;8(4):467–75. [PubMed: 2727470]
- 12.
- Altman DG. Comparability of randomised groups. Statistician. 1985;34:125–36.
- 13.
- Altman DG, Doré CJ. Randomisation and baseline comparisons in clinical trials. Lancet. 1990;335(8682):149–53. [PubMed: 1967441]
- 14.
- Begg CB. Suspended judgment. Significance tests of covariate imbalance in clinical trials. Control Clin Trials. 1990;(11):223–5. [PubMed: 2171874]
- 15.
- Austin PC, Manca A, Zwarenstein M, et al. A substantial and confusing variation exists in handling of baseline covariates in randomized controlled trials: a review of trials published in leading medical journals. J Clin Epidemiol. 2010 Feb;63(2):142–53. [PubMed: 19716262]
- 16.
- Hewitt CE, Kumaravel B, Dumville JC, et al. Assessing the impact of attrition in randomized controlled trials. J Clin Epidemiol. 2010 Nov;63(11):1264–70. [PubMed: 20573482]
- 17.
- Senn S. Change from baseline and analysis of covariance revisited. Stat Med. 2006 Dec 30;25(24):4334–44. [PubMed: 16921578]
- 18.
- Crager MR. Analysis of covariance in parallel-group clinical trials with pretreatment baselines. Biometrics. 1987 Dec;43(4):895–901. [PubMed: 3427174]
- 19.
- Vickers AJ, Altman DG. Statistics notes: analysing controlled trials with baseline and follow up measurements. BMJ. 2001;323:1123–4. [PMC free article: PMC1121605] [PubMed: 11701584]
- 20.
- Balk EM, Earley A, Patel K, et al. Empirical Assessment of Within-Arm Correlation Imputation in Trials of Continuous Outcomes. Methods Research Report. Rockville, MD: Agency for Healthcare Research and Quality; Nov, 2012. AHRQ Publication No. 12(13)-EHC141-EF. www
.effectivehealthcare .ahrq.gov/reports/final.cfm . [PubMed: 23326900] - 21.
- Wiebe N, Vandermeer B, Platt RW, et al. A systematic review identifies a lack of standardization in methods for handling missing variance data. J Clin Epidemiol. 2006 Apr;59(4):342–53. [PubMed: 16549255]
- 22.
- Health Services Research Unit. Database of ICCs. University of Aberdeen Chief Scientist Office; [Accessed April 18, 2013]. www
.abdn.ac.uk/hsru/research /delivery/behaviour /methodological-research/ - 23.
- Cook JA, Bruckner T, MacLennan GS, et al. Clustering in surgical trials--database of intracluster correlations. Trials. 2012;13:2. [PMC free article: PMC3311136] [PubMed: 22217216]
- 24.
- Ukoumunne OC, Gulliford MC, Chinn S, et al. Methods for evaluating area-wide and organisation-based interventions in health and health care: a systematic review. Health Technol Assess. 1999;3(5):iii-92. [PubMed: 10982317]
- 25.
- Taljaard M, Donner A, Villar J, et al. Intracluster correlation coefficients from the 2005 WHO Global Survey on Maternal and Perinatal Health: implications for implementation research. Paediatr Perinat Epidemiol. 2008 Mar;22(2):117–25. [PubMed: 18298685]
- 26.
- Little RJA, Rubin DB. Statistical Analysis with Missing Data. 2nd ed. Hoboken, NJ: Wiley; 1987.
- 27.
- Higgins JPT, Green S, editors. Cochrane Handbook for Systematic Reviews of Interventions Version 5.1.0. The Cochrane Collaboration; 2011. [Updated March 2011]
- 28.
- Idris N, Robertson C. The effects of imputing the missing standard deviations on the standard error of meta analysis estimates. Communiations in Statistics - Simulaiton and Computation. 2009;38(3):513–26.
- 29.
- Follmann D, Elliott P, Suh I, et al. Variance imputation for overviews of clinical trials with continuous response. J Clin Epidemiol. 1992 Jul;45(7):769–73. [PubMed: 1619456]
- 30.
- Pigott T. Methods for handling missing data in research synthesis. In: Cooper H, Hedges LV, editors. Handbook of Research Synthesis. New York: Sage Publications; 1994. pp. 163–76.
- 31.
- Bracken M. Statistical methods for analysis of effects of treatment in overviews of randomized trials. In: Sinclair JD, Bracken MD, editors. Effective Care of the Newborn Infant. New York: Oxford University Press; 1992. pp. 13–20.
- 32.
- Bond K, Coyle D, O’Gorman K, et al. Long-Acting Beta2-Agonist and Inhaled Corticosteroid Combination Therapy for Adult Persistent Asthma: Systematic Review of Clinical Outcomes and Economic Evaluation. Ottawa: Canadian Agency for Drugs and Technologies in Health; 2009. [Technology report number 122] www
.cadth.ca/en/products /health-technology-assessment /publication/941 . - 33.
- Sanchez-Meca J, Marin-Martinez F. Weighting by inverse variance or by sample size in meta-analysis: a simulation study. Educ Psychol Meas. 1998;58(2):211–20.
- 34.
- Zhu W. Making bootstrap statistical inferences: a tutorial. Res Q Exerc Sport. 1997 Mar;68(1):44–55. [PubMed: 9094762]
- 35.
- Rubin DB, Schenker N. Multiple imputation in health-care databases: an overview and some applications. Stat Med. 1991 Apr;10(4):585–98. [PubMed: 2057657]
- 36.
- Stevens JW. A note on dealing with missing standard errors in meta-analyses of continuous outcome measures in WinBUGS. Pharm Stat. 2011 Jul-Aug;10(4):374–8. [PubMed: 21394888]
- 37.
- Ma Y, Mazumdar M. Multivariate meta-analysis: a robust approach based on the theory of U-statistic. Stat Med. 2011 Oct 30;30(24):2911–29. [PubMed: 21830230]
- 38.
- White IR, Higgins JP, Wood AM. Allowing for uncertainty due to missing data in meta-analysis--part 1: two-stage methods. Stat Med. 2008 Feb 28;27(5):711–27. [PubMed: 17703496]
- 39.
- White IR, Welton NJ, Wood AM, et al. Allowing for uncertainty due to missing data in meta-analysis--part 2: hierarchical models. Stat Med. 2008 Feb 28;27(5):728–45. [PubMed: 17703502]
- 40.
- Thiessen Philbrook H, Barrowman N, Garg AX. Imputing variance estimates do not alter the conclusions of a meta-analysis with continuous outcomes: a case study of changes in renal function after living kidney donation. J Clin Epidemiol. 2007 Mar;60(3):228–40. [PubMed: 17292016]
- 41.
- Yuan Y, Little RJ. Meta-analysis of studies with missing data. Biometrics. 2009 Jun;65(2):487–96. [PubMed: 18565168]
- 42.
- Hemming K, Hutton JL, Maguire MG, et al. Meta-regression with partial information on summary trial or patient characteristics. Stat Med. 2010 May 30;29(12):1312–24. [PubMed: 20087842]
- 43.
- Higgins JP, White IR, Anzures-Cabrera J. Meta-analysis of skewed data: combining results reported on log-transformed or raw scales. Stat Med. 2008 Dec 20;27(29):6072–92. [PMC free article: PMC2978323] [PubMed: 18800342]
- 44.
- Ziguras SJ, Stuart GW, Jackson AC. Assessing the evidence on case management. Br J Psychiatry. 2002 Jul;181:17–21. [PubMed: 12091258]
- 45.
- Shen YC, Eggleston K, Lau J, et al. Hospital ownership and financial performance: what explains the different findings in the empirical literature? Inquiry. 2007 Spring;44(1):41–68. [PubMed: 17583261]
- 46.
- Altman DG, Bland JM. Detecting skewness from summary information. BMJ. 1996 Nov 9;313(7066):1200. [PMC free article: PMC2352469] [PubMed: 8916759]
- 47.
- Hozo SP, Djulbegovic B, Hozo I. Estimating the mean and variance from the median, range, and the size of a sample. BMC Med Res Methodol. 2005;5:13. [PMC free article: PMC1097734] [PubMed: 15840177]
- 48.
- Pearson ES. The percentage limits for the distribution of range in samples from a normal population (n less than or equal to 100). Biometrika. 1932;24(3–4):404–17.
- 49.
- Friedrich JO, Adhikari NK, Beyene J. Ratio of geometric means to analyze continuous outcomes in meta-analysis: comparison to mean differences and ratio of arithmetic means using empiric data and simulation. Stat Med. 2012 Jul 30;31(17):1857–86. [PubMed: 22438170]
- 50.
- Card NA. Applied Meta-analysis for Social Science Research. 1st ed. New York: The Guilford Press; 2012.
- 51.
- Durlak JA. How to select, calculate, and interpret effect sizes. J Pediatr Psychol. 2009 Oct;34(9):917–28. [PubMed: 19223279]
- 52.
- Hedges LV. Estimation of effect size from a series of independent experiments. Psychological Bulletin. 1982 September;92(2):490–9.
- 53.
- Cohen J. Statistical Power Analysis for the Behavioral Sciences. 2nd ed. Hillsdale, NJ: Lawrence Erlbaum Associates; 1988.
- 54.
- Charach A, Dashti B, Carson P, et al. Attention Deficit Hyperactivity Disorder: Effectiveness of Treatment in At-Risk Preschoolers; Long-Term Effectiveness in All Ages; and Variability in Prevalence, Diagnosis, and Treatment. Comparative Effectivess Review No. 44. Rockville, MD: Agency for Healthcare Research and Quality; Oct, 2011. AHRQ Publication No. 12-EHC003-EF. www
.effectivehealthcare .ahrq.gov/reports/final.cfm . [PubMed: 22191110] - 55.
- Van Den Noortgate W, Onghena P. Estimating the mean effect size in meta-analysis: bias, precision, and mean squared error of different weighting methods. Behav Res Methods Instrum Comput. 2003 Nov;35(4):504–11. [PubMed: 14748494]
- 56.
- Nakagawa S, Cuthill IC. Effect size, confidence interval and statistical significance: a practical guide for biologists. Biol Rev Camb Philos Soc. 2007 Nov;82(4):591–605. [PubMed: 17944619]
- 57.
- Furukawa TA, Barbui C, Cipriani A, et al. Imputing missing standard deviations in meta-analyses can provide accurate results. J Clin Epidemiol. 2006 Jan;59(1):7–10. [PubMed: 16360555]
- 58.
- Tendal B, Nuesch E, Higgins JP, et al. Multiplicity of data in trial reports and the reliability of meta-analyses: empirical study. BMJ. 2011;343:d4829. [PMC free article: PMC3171064] [PubMed: 21878462]
- 59.
- WINPEPI Program COMPARE2 [Internet]. [Accessed April 18, 2013]. www
.brixtonhealth.com/pepi4windows.html . - 60.
- Adhikari NK, Burns KE, Friedrich JO, et al. Effect of nitric oxide on oxygenation and mortality in acute lung injury: systematic review and meta-analysis. BMJ. 2007 Apr 14;334(7597):779. [PMC free article: PMC1852043] [PubMed: 17383982]
- 61.
- Kunz R, Friedrich C, Wolbers M, et al. Meta-analysis: effect of monotherapy and combination therapy with inhibitors of the renin angiotensin system on proteinuria in renal disease. Ann Intern Med. 2008 Jan 1;148(1):30–48. [PubMed: 17984482]
- 62.
- Peng PW, Wijeysundera DN, Li CC. Use of gabapentin for perioperative pain control -- a meta-analysis. Pain Res Manag. 2007 Summer;12(2):85–92. [PMC free article: PMC2670715] [PubMed: 17505569]
- 63.
- Sud S, Sud M, Friedrich JO, et al. High frequency oscillation in patients with acute lung injury and acute respiratory distress syndrome (ARDS): systematic review and meta-analysis. BMJ. 2010;340:c2327. [PubMed: 20483951]
- 64.
- Tubach F, Ravaud P, Baron G, et al. Evaluation of clinically relevant states in patient reported outcomes in knee and hip osteoarthritis: the patient acceptable symptom state. Ann Rheum Dis. 2005 Jan;64(1):34–7. [PMC free article: PMC1755171] [PubMed: 15130902]
- 65.
- Anzures-Cabrera J, Sarpatwari A, Higgins JP. Expressing findings from meta-analyses of continuous outcomes in terms of risks. Stat Med. 2011 Nov 10;30(25):2967–85. [PubMed: 21826697]
- 66.
- Chinn S. A simple method for converting an odds ratio to effect size for use in meta-analysis. Stat Med. 2000 Nov 30;19(22):3127–31. [PubMed: 11113947]
Abbreviations
- ADHD
Attention deficit hyperactivity disorder
- ANCOVA
Analysis of covariance
- DBD
Disruptive behavior disorder
- ECBI
Eyberg child behavior inventory
- EPC
Evidence-based Practice Center
- MCAR
Missing completely at random
- MAR
Missing at random
- MNAR
Missing not at random
- PACS
Parental account of childhood symptoms
- PBT
Parent behavior training
- RCT
Randomized clinical trial
- RoM
Ratio of means
- SD
Standard deviation
- SE
Standard error
- SMD
Standardized mean difference
Appendix A. Search Strategies
Standardized Mean Difference
Ovid Medline (Date Searched 3/8/2012)
1 | (standardized adj1 mean adj1 difference).ti,ab. | 532 |
2 | meta-analysis as topic/ | 12130 |
3 | meta-analys$.ti,ab. | 41814 |
4 | exp statistics as topic/ | 1697404 |
5 | meta-analysis.sh. | 33853 |
6 | 2 or 3 or 5 | 59871 |
7 | 1 and 4 and 6 | 79 |
Current Index to Statistics (Date Searched 2/22/2012)
Keyword search using combinations of standardized mean difference
Baseline Imbalances
Ovid Medline (Date Searched 2/22/2012)
1 | ((imbalance* or balance* or distribution) and (pre-treatment or pretreatment or baseline or pre-intervention or preintervention or covariat*)).ti,ab. | 18981 |
2 | exp clinical trials as topic/ | 255550 |
3 | meta-analysis as topic/ | 12130 |
4 | "review literature as topic"/ | 4314 |
5 | exp "bias (epidemiology)"/ | 45684 |
6 | exp "analysis of variance"/ | 237153 |
7 | ((analys$ adj3 covarian$) or ANCOVA).ti,ab. | 8690 |
8 | data interpretation, statistical/ | 42335 |
9 | 3 or 4 or 5 or 6 or 7 or 8 | 338233 |
10 | 1 and 2 and 9 | 210 |
Current Index to Statistics (Date Searched 2/22/2012)
Keyword search using combinations of (imbalance* or balance* or distribution) and (pre-treatment or pretreatment or baseline or pre-intervention or preintervention or covariat*)
Scopus
Pearling search to identify additional relevant citations from relevant articles already identified.
Meta-analysis of Skewed Data
Ovid Medline (Date Searched: 3/8-20/2012), Current Index to Statistics, Scopus
We took the Higgins article (Higgins, White and Anzures-Cabrera, "Meta-analysis of skewed data: combining results reported on log-transformed or raw scales." Stats in Med 2008; 27:6072–6092.) as a starting point but were unable to define a subject search that worked, so we did a combination of keyword and pearling searches in Ovid Medline, Current Index to Statistics, and Scopus.
Means Ratios in Pooled Analyses and Categorizing for Continuous Outcomes
We searched Ovid MEDLINE(R) <1946 to January Week 4 2012> and PubMed on March 1st 2012 for (Dichotomis* or Dichotomiz*) limited to: Humans, Meta-Analysis, and English. We searched Web of Science for articles citing either of 2 known studies:
- Fu R, Gartlehner G, Grant M, et al. Conducting quantitative synthesis when comparing medical interventions: AHRQ and the Effective Health Care Program. Journal of Clinical Epidemiology. 2011;64(11):1187–97.
- Friedrich JO, Adhikari NK, Beyene J. The ratio of means method as an alternative to mean differences for analyzing continuous outcome variables in meta-analysis: a simulation study. BMC Med Res Methodol. 2008;8:32. PMID: 18492289.) in combination with a known author/expert (Friedrich, JO). Experts and reviewers also recommended references based on experience and reference list checking.
Acknowledgments
The investigators would like to acknowledge the following people for their contributions: our associate editor, Mark Helfand, M.D., M.S., M.P.H., FACP, for his review of the report and meaningful comments; Robin Paynter, M.L.S., for literature searches; and Leah Williams, B.S., and Elaine Graham, M.L.S., for editorial support. We also appreciate the thoughtful comments of the peer reviewers listed below.
Peer Reviewers
Prior to publication of the final evidence report, EPCs sought input from independent Peer Reviewers without financial conflicts of interest. However, the conclusions and synthesis of the scientific literature presented in this report does not necessarily represent the views of individual reviewers.
Peer Reviewers must disclose any financial conflicts of interest greater than $10,000 and any other relevant business or professional conflicts of interest. Because of their unique clinical or content expertise, individuals with potential nonfinancial conflicts may be retained. The TOO and the EPC work to balance, manage, or mitigate any potential nonfinancial conflicts of interest identified.
The list of Peer Reviewers follows:
- Douglas G. AltmanCentre for Statistics in Medicine WolfsonCollege AnnexeOxford, England
- Roger Chou, M.D., FACPPacific Northwest Evidence-based Practice CenterOregon Health & Science UniversityPortland, OR
- Tim Ramsay, M.Sc., Ph.D.Ottawa Hospital Research InstituteUniversity of OttawaOttawa, Canada
- Jan O. Friedrich, M.D., M.Sc., D.Phil., FRCPCKeenan Research Centre of the Li Ka ShingKnowledge InstituteSt Michael’s Hospital and University of TorontoToronto, Canada
- Joanne McKenzie, D.Phil.School of Public Health and PreventiveMedicine, Monash UniversityMelbourne, Australia
- Robert Platt, Ph.D.McGill UniversityMontreal Children’s Hospital Research InstituteMontreal, Canada
This report is based on research conducted by the Oregon Evidence-based Practice Center (EPC) under contract to the Agency for Healthcare Research and Quality (AHRQ), Rockville, MD (Contract No. 290-2007-10057-I). The findings and conclusions in this document are those of the authors, who are responsible for its contents; the findings and conclusions do not necessarily represent the views of AHRQ. Therefore, no statement in this report should be construed as an official position of AHRQ or of the U.S. Department of Health and Human Services.
The information in this report is intended to help health care decisionmakers—patients and clinicians, health system leaders, and policymakers, among others—make well-informed decisions and thereby improve the quality of health care services. This report is not intended to be a substitute for the application of clinical judgment. Anyone who makes decisions concerning the provision of clinical care should consider this report in the same way as any medical reference and in conjunction with all other pertinent information, i.e., in the context of available resources and circumstances presented by individual patients.
This report may be used, in whole or in part, as the basis for development of clinical practice guidelines and other quality enhancement tools, or as a basis for reimbursement and coverage policies. AHRQ or U.S. Department of Health and Human Services endorsement of such derivative products may not be stated or implied.
None of the investigators have any affiliations or financial involvement that conflicts with the material presented in this report.
Suggested citation: Fu R, Vandermeer BW, Shamliyan TA, O’Neil ME, Yazdi F, Fox SH, Morton SC. Handling Continuous Outcomes in Quantitative Synthesis. Methods Guide for Comparative Effectiveness Reviews. (Prepared by the Oregon Evidence-based Practice Center under Contract No. 290-2007-10057-I.) AHRQ Publication No. 13-EHC103-EF. Rockville, MD: Agency for Healthcare Research and Quality. July 2013. www
.effectivehealthcare .ahrq.gov/reports/final.cfm.
- Introduction
- Effect Measures for Continuous Outcomes
- Choice of Estimate for Mean Difference and Baseline Imbalance
- Calculating Standard Deviation and Standard Error When They Are Not Directly Reported
- Dealing With Missing Data
- Dealing with Skewed Data
- Standardized Mean Difference
- Ratio of Means
- Dichotomizing Continuous Outcomes in Meta-Analyses
- Conclusion
- References
- Abbreviations
- Search Strategies
- Acknowledgments
- Peer Reviewers
- Missing data were poorly reported and handled in randomized controlled trials with repeatedly measured continuous outcomes: a cross-sectional survey.[J Clin Epidemiol. 2022]Missing data were poorly reported and handled in randomized controlled trials with repeatedly measured continuous outcomes: a cross-sectional survey.Ren Y, Jia Y, Huang Y, Zhang Y, Li Q, Yao M, Li L, Li G, Yang M, Yan P, et al. J Clin Epidemiol. 2022 Aug; 148:27-38. Epub 2022 Apr 15.
- Review Uptake of methodological advances for synthesis of continuous and time-to-event outcomes would maximize use of the evidence base.[J Clin Epidemiol. 2020]Review Uptake of methodological advances for synthesis of continuous and time-to-event outcomes would maximize use of the evidence base.Freeman SC, Sutton AJ, Cooper NJ. J Clin Epidemiol. 2020 Aug; 124:94-105. Epub 2020 May 12.
- A Unified Continuous Flow Assembly-Line Synthesis of Highly Substituted Pyrazoles and Pyrazolines.[Angew Chem Int Ed Engl. 2017]A Unified Continuous Flow Assembly-Line Synthesis of Highly Substituted Pyrazoles and Pyrazolines.Britton J, Jamison TF. Angew Chem Int Ed Engl. 2017 Jul 17; 56(30):8823-8827. Epub 2017 Jun 20.
- Handling ties in continuous outcomes for confounder adjustment with rank-ordered logit and its application to ordinal outcomes.[Stat Methods Med Res. 2020]Handling ties in continuous outcomes for confounder adjustment with rank-ordered logit and its application to ordinal outcomes.Ning Y, Tan CS, Maraki A, Ho PJ, Hodgins S, Comasco E, Nilsson KW, Wagner P, Khoo EY, Tai ES, et al. Stat Methods Med Res. 2020 Feb; 29(2):437-454. Epub 2019 Apr 3.
- Review Droplet handling.[Adv Biochem Eng Biotechnol. 2010]Review Droplet handling.Torii T. Adv Biochem Eng Biotechnol. 2010; 119:165-77.
- Handling Continuous Outcomes in Quantitative Synthesis - Methods Guide for Effec...Handling Continuous Outcomes in Quantitative Synthesis - Methods Guide for Effectiveness and Comparative Effectiveness Reviews
Your browsing activity is empty.
Activity recording is turned off.
See more...